- Survey paper
- Open access
- Published: 03 May 2022
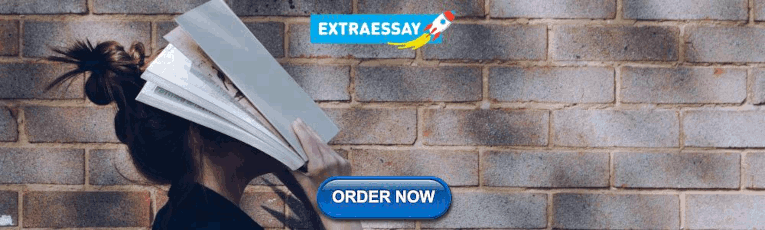
A systematic review and research perspective on recommender systems
- Deepjyoti Roy ORCID: orcid.org/0000-0002-8020-7145 1 &
- Mala Dutta 1
Journal of Big Data volume 9 , Article number: 59 ( 2022 ) Cite this article
80k Accesses
148 Citations
14 Altmetric
Metrics details
Recommender systems are efficient tools for filtering online information, which is widespread owing to the changing habits of computer users, personalization trends, and emerging access to the internet. Even though the recent recommender systems are eminent in giving precise recommendations, they suffer from various limitations and challenges like scalability, cold-start, sparsity, etc. Due to the existence of various techniques, the selection of techniques becomes a complex work while building application-focused recommender systems. In addition, each technique comes with its own set of features, advantages and disadvantages which raises even more questions, which should be addressed. This paper aims to undergo a systematic review on various recent contributions in the domain of recommender systems, focusing on diverse applications like books, movies, products, etc. Initially, the various applications of each recommender system are analysed. Then, the algorithmic analysis on various recommender systems is performed and a taxonomy is framed that accounts for various components required for developing an effective recommender system. In addition, the datasets gathered, simulation platform, and performance metrics focused on each contribution are evaluated and noted. Finally, this review provides a much-needed overview of the current state of research in this field and points out the existing gaps and challenges to help posterity in developing an efficient recommender system.
Introduction
The recent advancements in technology along with the prevalence of online services has offered more abilities for accessing a huge amount of online information in a faster manner. Users can post reviews, comments, and ratings for various types of services and products available online. However, the recent advancements in pervasive computing have resulted in an online data overload problem. This data overload complicates the process of finding relevant and useful content over the internet. The recent establishment of several procedures having lower computational requirements can however guide users to the relevant content in a much easy and fast manner. Because of this, the development of recommender systems has recently gained significant attention. In general, recommender systems act as information filtering tools, offering users suitable and personalized content or information. Recommender systems primarily aim to reduce the user’s effort and time required for searching relevant information over the internet.
Nowadays, recommender systems are being increasingly used for a large number of applications such as web [ 1 , 67 , 70 ], books [ 2 ], e-learning [ 4 , 16 , 61 ], tourism [ 5 , 8 , 78 ], movies [ 66 ], music [ 79 ], e-commerce, news, specialized research resources [ 65 ], television programs [ 72 , 81 ], etc. It is therefore important to build high-quality and exclusive recommender systems for providing personalized recommendations to the users in various applications. Despite the various advances in recommender systems, the present generation of recommender systems requires further improvements to provide more efficient recommendations applicable to a broader range of applications. More investigation of the existing latest works on recommender systems is required which focus on diverse applications.
There is hardly any review paper that has categorically synthesized and reviewed the literature of all the classification fields and application domains of recommender systems. The few existing literature reviews in the field cover just a fraction of the articles or focus only on selected aspects such as system evaluation. Thus, they do not provide an overview of the application field, algorithmic categorization, or identify the most promising approaches. Also, review papers often neglect to analyze the dataset description and the simulation platforms used. This paper aims to fulfil this significant gap by reviewing and comparing existing articles on recommender systems based on a defined classification framework, their algorithmic categorization, simulation platforms used, applications focused, their features and challenges, dataset description and system performance. Finally, we provide researchers and practitioners with insight into the most promising directions for further investigation in the field of recommender systems under various applications.
In essence, recommender systems deal with two entities—users and items, where each user gives a rating (or preference value) to an item (or product). User ratings are generally collected by using implicit or explicit methods. Implicit ratings are collected indirectly from the user through the user’s interaction with the items. Explicit ratings, on the other hand, are given directly by the user by picking a value on some finite scale of points or labelled interval values. For example, a website may obtain implicit ratings for different items based on clickstream data or from the amount of time a user spends on a webpage and so on. Most recommender systems gather user ratings through both explicit and implicit methods. These feedbacks or ratings provided by the user are arranged in a user-item matrix called the utility matrix as presented in Table 1 .
The utility matrix often contains many missing values. The problem of recommender systems is mainly focused on finding the values which are missing in the utility matrix. This task is often difficult as the initial matrix is usually very sparse because users generally tend to rate only a small number of items. It may also be noted that we are interested in only the high user ratings because only such items would be suggested back to the users. The efficiency of a recommender system greatly depends on the type of algorithm used and the nature of the data source—which may be contextual, textual, visual etc.
Types of recommender systems
Recommender systems are broadly categorized into three different types viz. content-based recommender systems, collaborative recommender systems and hybrid recommender systems. A diagrammatic representation of the different types of recommender systems is given in Fig. 1 .
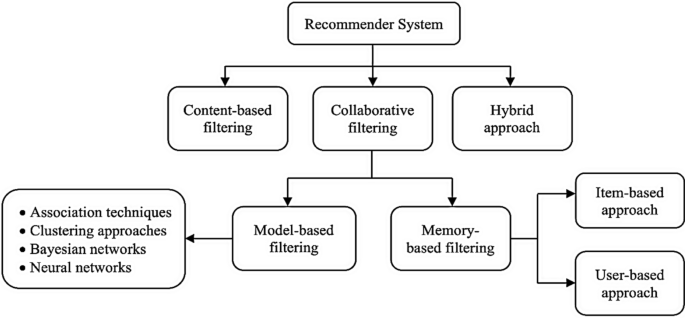
Content-based recommender system
In content-based recommender systems, all the data items are collected into different item profiles based on their description or features. For example, in the case of a book, the features will be author, publisher, etc. In the case of a movie, the features will be the movie director, actor, etc. When a user gives a positive rating to an item, then the other items present in that item profile are aggregated together to build a user profile. This user profile combines all the item profiles, whose items are rated positively by the user. Items present in this user profile are then recommended to the user, as shown in Fig. 2 .
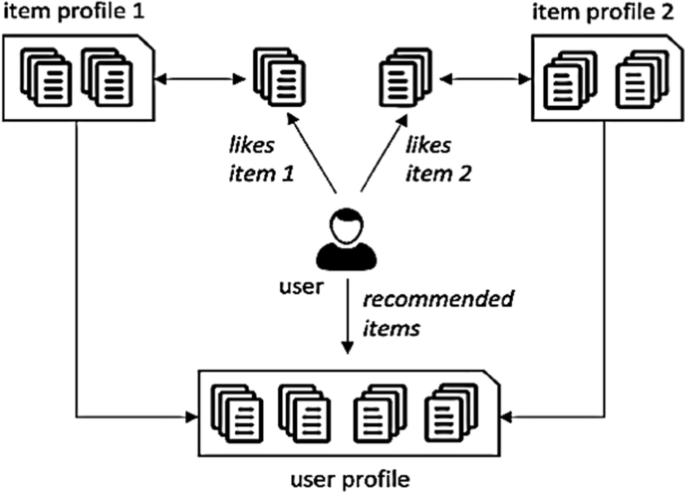
One drawback of this approach is that it demands in-depth knowledge of the item features for an accurate recommendation. This knowledge or information may not be always available for all items. Also, this approach has limited capacity to expand on the users' existing choices or interests. However, this approach has many advantages. As user preferences tend to change with time, this approach has the quick capability of dynamically adapting itself to the changing user preferences. Since one user profile is specific only to that user, this algorithm does not require the profile details of any other users because they provide no influence in the recommendation process. This ensures the security and privacy of user data. If new items have sufficient description, content-based techniques can overcome the cold-start problem i.e., this technique can recommend an item even when that item has not been previously rated by any user. Content-based filtering approaches are more common in systems like personalized news recommender systems, publications, web pages recommender systems, etc.
Collaborative filtering-based recommender system
Collaborative approaches make use of the measure of similarity between users. This technique starts with finding a group or collection of user X whose preferences, likes, and dislikes are similar to that of user A. X is called the neighbourhood of A. The new items which are liked by most of the users in X are then recommended to user A. The efficiency of a collaborative algorithm depends on how accurately the algorithm can find the neighbourhood of the target user. Traditionally collaborative filtering-based systems suffer from the cold-start problem and privacy concerns as there is a need to share user data. However, collaborative filtering approaches do not require any knowledge of item features for generating a recommendation. Also, this approach can help to expand on the user’s existing interests by discovering new items. Collaborative approaches are again divided into two types: memory-based approaches and model-based approaches.
Memory-based collaborative approaches recommend new items by taking into consideration the preferences of its neighbourhood. They make use of the utility matrix directly for prediction. In this approach, the first step is to build a model. The model is equal to a function that takes the utility matrix as input.
Model = f (utility matrix)
Then recommendations are made based on a function that takes the model and user profile as input. Here we can make recommendations only to users whose user profile belongs to the utility matrix. Therefore, to make recommendations for a new user, the user profile must be added to the utility matrix, and the similarity matrix should be recomputed, which makes this technique computation heavy.
Recommendation = f (defined model, user profile) where user profile ∈ utility matrix
Memory-based collaborative approaches are again sub-divided into two types: user-based collaborative filtering and item-based collaborative filtering. In the user-based approach, the user rating of a new item is calculated by finding other users from the user neighbourhood who has previously rated that same item. If a new item receives positive ratings from the user neighbourhood, the new item is recommended to the user. Figure 3 depicts the user-based filtering approach.
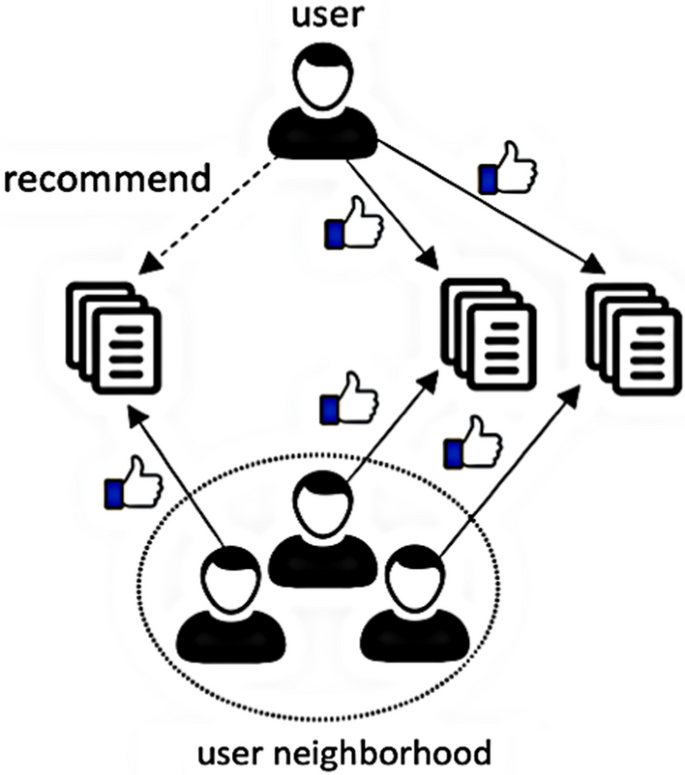
User-based collaborative filtering
In the item-based approach, an item-neighbourhood is built consisting of all similar items which the user has rated previously. Then that user’s rating for a different new item is predicted by calculating the weighted average of all ratings present in a similar item-neighbourhood as shown in Fig. 4 .
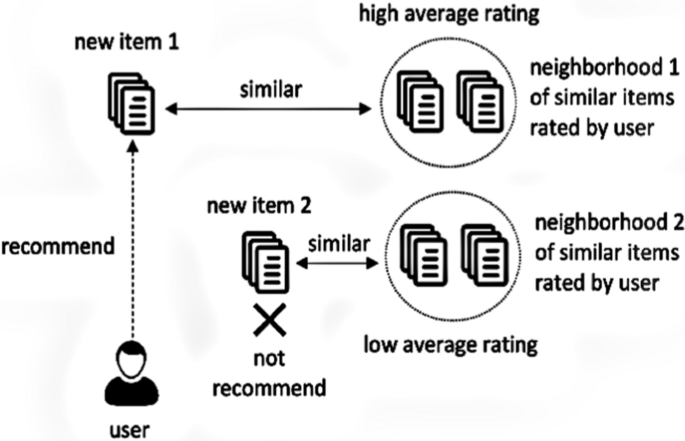
Item-based collaborative filtering
Model-based systems use various data mining and machine learning algorithms to develop a model for predicting the user’s rating for an unrated item. They do not rely on the complete dataset when recommendations are computed but extract features from the dataset to compute a model. Hence the name, model-based technique. These techniques also need two steps for prediction—the first step is to build the model, and the second step is to predict ratings using a function (f) which takes the model defined in the first step and the user profile as input.
Recommendation = f (defined model, user profile) where user profile ∉ utility matrix
Model-based techniques do not require adding the user profile of a new user into the utility matrix before making predictions. We can make recommendations even to users that are not present in the model. Model-based systems are more efficient for group recommendations. They can quickly recommend a group of items by using the pre-trained model. The accuracy of this technique largely relies on the efficiency of the underlying learning algorithm used to create the model. Model-based techniques are capable of solving some traditional problems of recommender systems such as sparsity and scalability by employing dimensionality reduction techniques [ 86 ] and model learning techniques.
Hybrid filtering
A hybrid technique is an aggregation of two or more techniques employed together for addressing the limitations of individual recommender techniques. The incorporation of different techniques can be performed in various ways. A hybrid algorithm may incorporate the results achieved from separate techniques, or it can use content-based filtering in a collaborative method or use a collaborative filtering technique in a content-based method. This hybrid incorporation of different techniques generally results in increased performance and increased accuracy in many recommender applications. Some of the hybridization approaches are meta-level, feature-augmentation, feature-combination, mixed hybridization, cascade hybridization, switching hybridization and weighted hybridization [ 86 ]. Table 2 describes these approaches.
Recommender system challenges
This section briefly describes the various challenges present in current recommender systems and offers different solutions to overcome these challenges.
Cold start problem
The cold start problem appears when the recommender system cannot draw any inference from the existing data, which is insufficient. Cold start refers to a condition when the system cannot produce efficient recommendations for the cold (or new) users who have not rated any item or have rated a very few items. It generally arises when a new user enters the system or new items (or products) are inserted into the database. Some solutions to this problem are as follows: (a) Ask new users to explicitly mention their item preference. (b) Ask a new user to rate some items at the beginning. (c) Collect demographic information (or meta-data) from the user and recommend items accordingly.
Shilling attack problem
This problem arises when a malicious user fakes his identity and enters the system to give false item ratings [ 87 ]. Such a situation occurs when the malicious user wants to either increase or decrease some item’s popularity by causing a bias on selected target items. Shilling attacks greatly reduce the reliability of the system. One solution to this problem is to detect the attackers quickly and remove the fake ratings and fake user profiles from the system.
Synonymy problem
This problem arises when similar or related items have different entries or names, or when the same item is represented by two or more names in the system [ 78 ]. For example, babywear and baby cloth. Many recommender systems fail to distinguish these differences, hence reducing their recommendation accuracy. To alleviate this problem many methods are used such as demographic filtering, automatic term expansion and Singular Value Decomposition [ 76 ].
Latency problem
The latency problem is specific to collaborative filtering approaches and occurs when new items are frequently inserted into the database. This problem is characterized by the system’s failure to recommend new items. This happens because new items must be reviewed before they can be recommended in a collaborative filtering environment. Using content-based filtering may resolve this issue, but it may introduce overspecialization and decrease the computing time and system performance. To increase performance, the calculations can be done in an offline environment and clustering-based techniques can be used [ 76 ].
Sparsity problem
Data sparsity is a common problem in large scale data analysis, which arises when certain expected values are missing in the dataset. In the case of recommender systems, this situation occurs when the active users rate very few items. This reduces the recommendation accuracy. To alleviate this problem several techniques can be used such as demographic filtering, singular value decomposition and using model-based collaborative techniques.
Grey sheep problem
The grey sheep problem is specific to pure collaborative filtering approaches where the feedback given by one user do not match any user neighbourhood. In this situation, the system fails to accurately predict relevant items for that user. This problem can be resolved by using pure content-based approaches where predictions are made based on the user’s profile and item properties.
Scalability problem
Recommender systems, especially those employing collaborative filtering techniques, require large amounts of training data, which cause scalability problems. The scalability problem arises when the amount of data used as input to a recommender system increases quickly. In this era of big data, more and more items and users are rapidly getting added to the system and this problem is becoming common in recommender systems. Two common approaches used to solve the scalability problem is dimensionality reduction and using clustering-based techniques to find users in tiny clusters instead of the complete database.
Methodology
The purpose of this study is to understand the research trends in the field of recommender systems. The nature of research in recommender systems is such that it is difficult to confine each paper to a specific discipline. This can be further understood by the fact that research papers on recommender systems are scattered across various journals such as computer science, management, marketing, information technology and information science. Hence, this literature review is conducted over a wide range of electronic journals and research databases such as ACM Portal, IEEE/IEE Library, Google Scholars and Science Direct [ 88 ].
The search process of online research articles was performed based on 6 descriptors: “Recommender systems”, “Recommendation systems”, “Movie Recommend*”, “Music Recommend*”, “Personalized Recommend*”, “Hybrid Recommend*”. The following research papers described below were excluded from our research:
News articles.
Master’s dissertations.
Non-English papers.
Unpublished papers.
Research papers published before 2011.
We have screened a total of 350 articles based on their abstracts and content. However, only research papers that described how recommender systems can be applied were chosen. Finally, 60 papers were selected from top international journals indexed in Scopus or E-SCI in 2021. We now present the PRISMA flowchart of the inclusion and exclusion process in Fig. 5 .
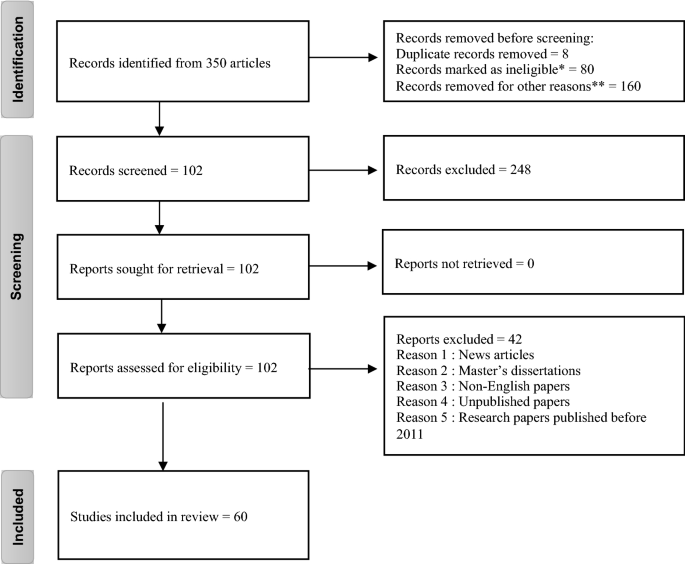
PRISMA flowchart of the inclusion and exclusion process. Abstract and content not suitable to the study: * The use or application of the recommender system is not specified: **
Each paper was carefully reviewed and classified into 6 categories in the application fields and 3 categories in the techniques used to develop the system. The classification framework is presented in Fig. 6 .
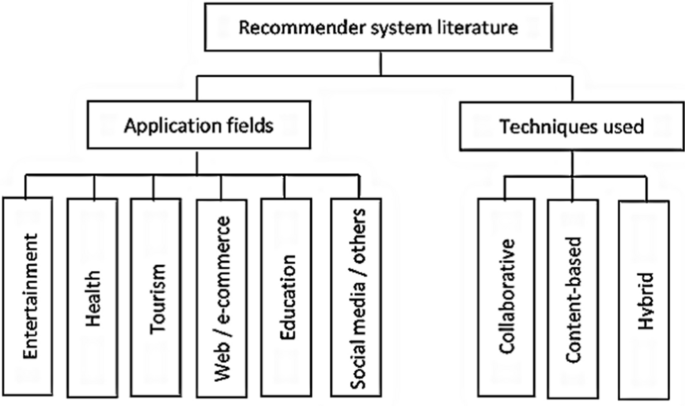
Classification framework
The number of relevant articles come from Expert Systems with Applications (23%), followed by IEEE (17%), Knowledge-Based System (17%) and Others (43%). Table 3 depicts the article distribution by journal title and Table 4 depicts the sector-wise article distribution.
Both forward and backward searching techniques were implemented to establish that the review of 60 chosen articles can represent the domain literature. Hence, this paper can demonstrate its validity and reliability as a literature review.
Review on state-of-the-art recommender systems
This section presents a state-of-art literature review followed by a chronological review of the various existing recommender systems.
Literature review
In 2011, Castellano et al. [ 1 ] developed a “NEuro-fuzzy WEb Recommendation (NEWER)” system for exploiting the possibility of combining computational intelligence and user preference for suggesting interesting web pages to the user in a dynamic environment. It considered a set of fuzzy rules to express the correlations between user relevance and categories of pages. Crespo et al. [ 2 ] presented a recommender system for distance education over internet. It aims to recommend e-books to students using data from user interaction. The system was developed using a collaborative approach and focused on solving the data overload problem in big digital content. Lin et al. [ 3 ] have put forward a recommender system for automatic vending machines using Genetic algorithm (GA), k-means, Decision Tree (DT) and Bayesian Network (BN). It aimed at recommending localized products by developing a hybrid model combining statistical methods, classification methods, clustering methods, and meta-heuristic methods. Wang and Wu [ 4 ] have implemented a ubiquitous learning system for providing personalized learning assistance to the learners by combining the recommendation algorithm with a context-aware technique. It employed the Association Rule Mining (ARM) technique and aimed to increase the effectiveness of the learner’s learning. García-Crespo et al. [ 5 ] presented a “semantic hotel” recommender system by considering the experiences of consumers using a fuzzy logic approach. The system considered both hotel and customer characteristics. Dong et al. [ 6 ] proposed a structure for a service-concept recommender system using a semantic similarity model by integrating the techniques from the view of an ontology structure-oriented metric and a concept content-oriented metric. The system was able to deliver optimal performance when compared with similar recommender systems. Li et al. [ 7 ] developed a Fuzzy linguistic modelling-based recommender system for assisting users to find experts in knowledge management systems. The developed system was applied to the aircraft industry where it demonstrated efficient and feasible performance. Lorenzi et al. [ 8 ] presented an “assumption-based multiagent” system to make travel package recommendations using user preferences in the tourism industry. It performed different tasks like discovering, filtering, and integrating specific information for building a travel package following the user requirement. Huang et al. [ 9 ] proposed a context-aware recommender system through the extraction, evaluation and incorporation of contextual information gathered using the collaborative filtering and rough set model.
In 2012, Chen et al. [ 10 ] presented a diabetes medication recommender model by using “Semantic Web Rule Language (SWRL) and Java Expert System Shell (JESS)” for aggregating suitable prescriptions for the patients. It aimed at selecting the most suitable drugs from the list of specific drugs. Mohanraj et al. [ 11 ] developed the “Ontology-driven bee’s foraging approach (ODBFA)” to accurately predict the online navigations most likely to be visited by a user. The self-adaptive system is intended to capture the various requirements of the online user by using a scoring technique and by performing a similarity comparison. Hsu et al. [ 12 ] proposed a “personalized auxiliary material” recommender system by considering the specific course topics, individual learning styles, complexity of the auxiliary materials using an artificial bee colony algorithm. Gemmell et al. [ 13 ] demonstrated a solution for the problem of resource recommendation in social annotation systems. The model was developed using a linear-weighted hybrid method which was capable of providing recommendations under different constraints. Choi et al. [ 14 ] proposed one “Hybrid Online-Product rEcommendation (HOPE) system” by the integration of collaborative filtering through sequential pattern analysis-based recommendations and implicit ratings. Garibaldi et al. [ 15 ] put forward a technique for incorporating the variability in a fuzzy inference model by using non-stationary fuzzy sets for replicating the variabilities of a human. This model was applied to a decision problem for treatment recommendations of post-operative breast cancer.
In 2013, Salehi and Kmalabadi [ 16 ] proposed an e-learning material recommender system by “modelling of materials in a multidimensional space of material’s attribute”. It employed both content and collaborative filtering. Aher and Lobo [ 17 ] introduced a course recommender system using data mining techniques such as simple K-means clustering and Association Rule Mining (ARM) algorithm. The proposed e-learning system was successfully demonstrated for “MOOC (Massively Open Online Courses)”. Kardan and Ebrahimi [ 18 ] developed a hybrid recommender system for recommending posts in asynchronous discussion groups. The system was built combining both collaborative filtering and content-based filtering. It considered implicit user data to compute the user similarity with various groups, for recommending suitable posts and contents to its users. Chang et al. [ 19 ] adopted a cloud computing technology for building a TV program recommender system. The system designed for digital TV programs was implemented using Hadoop Fair Scheduler (HFC), K-means clustering and k-nearest neighbour (KNN) algorithms. It was successful in processing huge amounts of real-time user data. Lucas et al. [ 20 ] implemented a recommender model for assisting a tourism application by using associative classification and fuzzy logic to predict the context. Niu et al. [ 21 ] introduced “Affivir: An Affect-based Internet Video Recommendation System” which was developed by calculating user preferences and by using spectral clustering. This model recommended videos with similar effects, which was processed to get optimal results with dynamic adjustments of recommendation constraints.
In 2014, Liu et al. [ 22 ] implemented a new route recommendation model for offering personalized and real-time route recommendations for self-driven tourists to minimize the queuing time and traffic jams infamous tourist places. Recommendations were carried out by considering the preferences of users. Bakshi et al. [ 23 ] proposed an unsupervised learning-based recommender model for solving the scalability problem of recommender systems. The algorithm used transitive similarities along with Particle Swarm Optimization (PSO) technique for discovering the global neighbours. Kim and Shim [ 24 ] proposed a recommender system based on “latent Dirichlet allocation using probabilistic modelling for Twitter” that could recommend the top-K tweets for a user to read, and the top-K users to follow. The model parameters were learned from an inference technique by using the differential Expectation–Maximization (EM) algorithm. Wang et al. [ 25 ] developed a hybrid-movie recommender model by aggregating a genetic algorithm (GA) with improved K-means and Principal Component Analysis (PCA) technique. It was able to offer intelligent movie recommendations with personalized suggestions. Kolomvatsos et al. [ 26 ] proposed a recommender system by considering an optimal stopping theory for delivering books or music recommendations to the users. Gottschlich et al. [ 27 ] proposed a decision support system for stock investment recommendations. It computed the output by considering the overall crowd’s recommendations. Torshizi et al. [ 28 ] have introduced a hybrid recommender system to determine the severity level of a medical condition. It could recommend suitable therapies for patients suffering from Benign Prostatic Hyperplasia.
In 2015, Zahálka et al. [ 29 ] proposed a venue recommender: “City Melange”. It was an interactive content-based model which used the convolutional deep-net features of the visual domain and the linear Support Vector Machine (SVM) model to capture the semantic information and extract latent topics. Sankar et al. [ 30 ] have proposed a stock recommender system based on the stock holding portfolio of trusted mutual funds. The system employed the collaborative filtering approach along with social network analysis for offering a decision support system to build a trust-based recommendation model. Chen et al. [ 31 ] have put forward a novel movie recommender system by applying the “artificial immune network to collaborative filtering” technique. It computed the affinity of an antigen and the affinity between an antibody and antigen. Based on this computation a similarity estimation formula was introduced which was used for the movie recommendation process. Wu et al. [ 32 ] have examined the technique of data fusion for increasing the efficiency of item recommender systems. It employed a hybrid linear combination model and used a collaborative tagging system. Yeh and Cheng [ 33 ] have proposed a recommender system for tourist attractions by constructing the “elicitation mechanism using the Delphi panel method and matrix construction mechanism using the repertory grids”, which was developed by considering the user preference and expert knowledge.
In 2016, Liao et al. [ 34 ] proposed a recommender model for online customers using a rough set association rule. The model computed the probable behavioural variations of online consumers and provided product category recommendations for e-commerce platforms. Li et al. [ 35 ] have suggested a movie recommender system based on user feedback collected from microblogs and social networks. It employed the sentiment-aware association rule mining algorithm for recommendations using the prior information of frequent program patterns, program metadata similarity and program view logs. Wu et al. [ 36 ] have developed a recommender system for social media platforms by aggregating the technique of Social Matrix Factorization (SMF) and Collaborative Topic Regression (CTR). The model was able to compute the ratings of users to items for making recommendations. For improving the recommendation quality, it gathered information from multiple sources such as item properties, social networks, feedback, etc. Adeniyi et al. [ 37 ] put forward a study of automated web-usage data mining and developed a recommender system that was tested in both real-time and online for identifying the visitor’s or client’s clickstream data.
In 2017, Rawat and Kankanhalli [ 38 ] have proposed a viewpoint recommender system called “ClickSmart” for assisting mobile users to capture high-quality photographs at famous tourist places. Yang et al. [ 39 ] proposed a gradient boosting-based job recommendation system for satisfying the cost-sensitive requirements of the users. The hybrid algorithm aimed to reduce the rate of unnecessary job recommendations. Lee et al. [ 40 ] proposed a music streaming recommender system based on smartphone activity usage. The proposed system benefitted by using feature selection approaches with machine learning techniques such as Naive Bayes (NB), Support Vector Machine (SVM), Multi-layer Perception (MLP), Instance-based k -Nearest Neighbour (IBK), and Random Forest (RF) for performing the activity detection from the mobile signals. Wei et al. [ 41 ] have proposed a new stacked denoising autoencoder (SDAE) based recommender system for cold items. The algorithm employed deep learning and collaborative filtering method to predict the unknown ratings.
In 2018, Li et al. [ 42 ] have developed a recommendation algorithm using Weighted Linear Regression Models (WLRRS). The proposed system was put to experiment using the MovieLens dataset and it presented better classification and predictive accuracy. Mezei and Nikou [ 43 ] presented a mobile health and wellness recommender system based on fuzzy optimization. It could recommend a collection of actions to be taken by the user to improve the user’s health condition. Recommendations were made considering the user’s physical activities and preferences. Ayata et al. [ 44 ] proposed a music recommendation model based on the user emotions captured through wearable physiological sensors. The emotion detection algorithm employed different machine learning algorithms like SVM, RF, KNN and decision tree (DT) algorithms to predict the emotions from the changing electrical signals gathered from the wearable sensors. Zhao et al. [ 45 ] developed a multimodal learning-based, social-aware movie recommender system. The model was able to successfully resolve the sparsity problem of recommender systems. The algorithm developed a heterogeneous network by exploiting the movie-poster image and textual description of each movie based on the social relationships and user ratings.
In 2019, Hammou et al. [ 46 ] proposed a Big Data recommendation algorithm capable of handling large scale data. The system employed random forest and matrix factorization through a data partitioning scheme. It was then used for generating recommendations based on user rating and preference for each item. The proposed system outperformed existing systems in terms of accuracy and speed. Zhao et al. [ 47 ] have put forward a hybrid initialization method for social network recommender systems. The algorithm employed denoising autoencoder (DAE) neural network-based initialization method (ANNInit) and attribute mapping. Bhaskaran and Santhi [ 48 ] have developed a hybrid, trust-based e-learning recommender system using cloud computing. The proposed algorithm was capable of learning online user activities by using the Firefly Algorithm (FA) and K-means clustering. Afolabi and Toivanen [ 59 ] have suggested an integrated recommender model based on collaborative filtering. The proposed model “Connected Health for Effective Management of Chronic Diseases”, aimed for integrating recommender systems for better decision-making in the process of disease management. He et al. [ 60 ] proposed a movie recommender system called “HI2Rec” which explored the usage of collaborative filtering and heterogeneous information for making movie recommendations. The model used the knowledge representation learning approach to embed movie-related information gathered from different sources.
In 2020, Han et al. [ 49 ] have proposed one Internet of Things (IoT)-based cancer rehabilitation recommendation system using the Beetle Antennae Search (BAS) algorithm. It presented the patients with a solution for the problem of optimal nutrition program by considering the objective function as the recurrence time. Kang et al. [ 50 ] have presented a recommender system for personalized advertisements in Online Broadcasting based on a tree model. Recommendations were generated in real-time by considering the user preferences to minimize the overhead of preference prediction and using a HashMap along with the tree characteristics. Ullah et al. [ 51 ] have implemented an image-based service recommendation model for online shopping based random forest and Convolutional Neural Networks (CNN). The model used JPEG coefficients to achieve an accurate prediction rate. Cai et al. [ 52 ] proposed a new hybrid recommender model using a many-objective evolutionary algorithm (MaOEA). The proposed algorithm was successful in optimizing the novelty, diversity, and accuracy of recommendations. Esteban et al. [ 53 ] have implemented a hybrid multi-criteria recommendation system concerned with students’ academic performance, personal interests, and course selection. The system was developed using a Genetic Algorithm (GA) and aimed at helping university students. It combined both course information and student information for increasing system performance and the reliability of the recommendations. Mondal et al. [ 54 ] have built a multilayer, graph data model-based doctor recommendation system by exploiting the trust concept between a patient-doctor relationship. The proposed system showed good results in practical applications.
In 2021, Dhelim et al. [ 55 ] have developed a personality-based product recommending model using the techniques of meta path discovery and user interest mining. This model showed better results when compared to session-based and deep learning models. Bhalse et al. [ 56 ] proposed a web-based movie recommendation system based on collaborative filtering using Singular Value Decomposition (SVD), collaborative filtering and cosine similarity (CS) for addressing the sparsity problem of recommender systems. It suggested a recommendation list by considering the content information of movies. Similarly, to solve both sparsity and cold-start problems Ke et al. [ 57 ] proposed a dynamic goods recommendation system based on reinforcement learning. The proposed system was capable of learning from the reduced entropy loss error on real-time applications. Chen et al. [ 58 ] have presented a movie recommender model combining various techniques like user interest with category-level representation, neighbour-assisted representation, user interest with latent representation and item-level representation using Feed-forward Neural Network (FNN).
Comparative chronological review
A comparative chronological review to compare the total contributions on various recommender systems in the past 10 years is given in Fig. 7 .
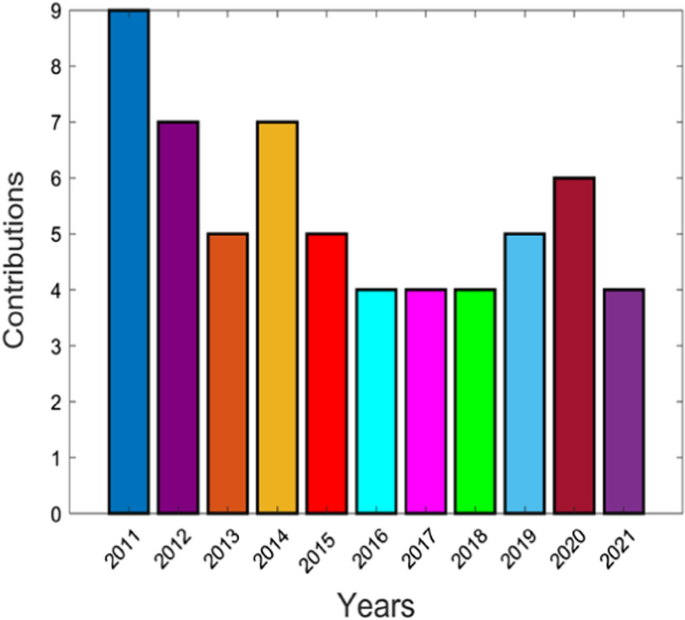
Comparative chronological review of recommender systems under diverse applications
This review puts forward a comparison of the number of research works proposed in the domain of recommender systems from the year 2011 to 2021 using various deep learning and machine learning-based approaches. Research articles are categorized based on the recommender system classification framework as shown in Table 5 . The articles are ordered according to their year of publication. There are two key concepts: Application fields and techniques used. The application fields of recommender systems are divided into six different fields, viz. entertainment, health, tourism, web/e-commerce, education and social media/others.
Algorithmic categorization, simulation platforms and applications considered for various recommender systems
This section analyses different methods like deep learning, machine learning, clustering and meta-heuristic-based-approaches used in the development of recommender systems. The algorithmic categorization of different recommender systems is given in Fig. 8 .
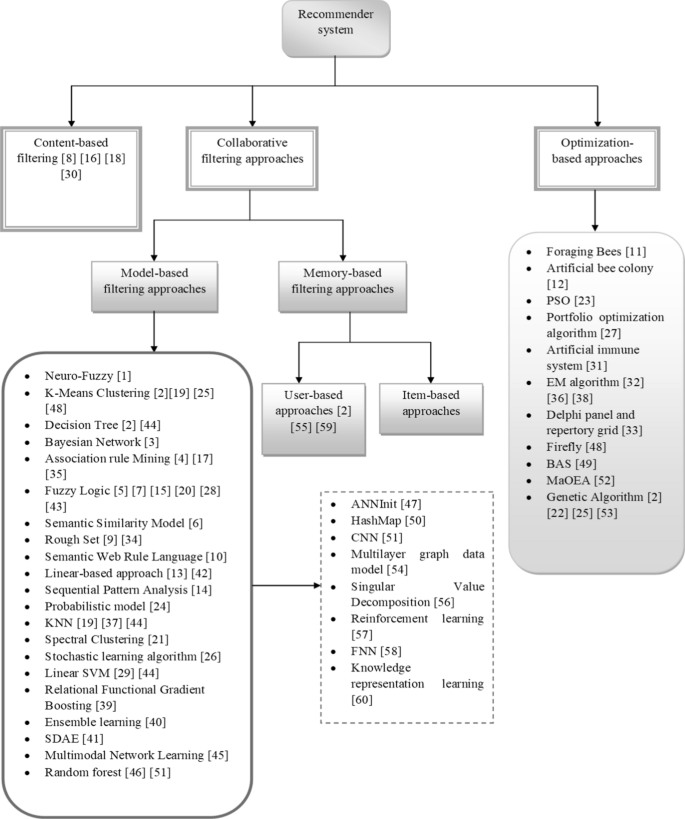
Algorithmic categorization of different recommender systems
Categorization is done based on content-based, collaborative filtering-based, and optimization-based approaches. In [ 8 ], a content-based filtering technique was employed for increasing the ability to trust other agents and for improving the exchange of information by trust degree. In [ 16 ], it was applied to enhance the quality of recommendations using the account attributes of the material. It achieved better performance concerning with F1-score, recall and precision. In [ 18 ], this technique was able to capture the implicit user feedback, increasing the overall accuracy of the proposed model. The content-based filtering in [ 30 ] was able to increase the accuracy and performance of a stock recommender system by using the “trust factor” for making decisions.
Different collaborative filtering approaches are utilized in recent studies, which are categorized as follows:
Model-based techniques
Neuro-Fuzzy [ 1 ] based technique helps in discovering the association between user categories and item relevance. It is also simple to understand. K-Means Clustering [ 2 , 19 , 25 , 48 ] is efficient for large scale datasets. It is simple to implement and gives a fast convergence rate. It also offers automatic recovery from failures. The decision tree [ 2 , 44 ] technique is easy to interpret. It can be used for solving the classic regression and classification problems in recommender systems. Bayesian Network [ 3 ] is a probabilistic technique used to solve classification challenges. It is based on the theory of Bayes theorem and conditional probability. Association Rule Mining (ARM) techniques [ 4 , 17 , 35 ] extract rules for projecting the occurrence of an item by considering the existence of other items in a transaction. This method uses the association rules to create a more suitable representation of data and helps in increasing the model performance and storage efficiency. Fuzzy Logic [ 5 , 7 , 15 , 20 , 28 , 43 ] techniques use a set of flexible rules. It focuses on solving complex real-time problems having an inaccurate spectrum of data. This technique provides scalability and helps in increasing the overall model performance for recommender systems. The semantic similarity [ 6 ] technique is used for describing a topological similarity to define the distance among the concepts and terms through ontologies. It measures the similarity information for increasing the efficiency of recommender systems. Rough set [ 9 , 34 ] techniques use probability distributions for solving the challenges of existing recommender models. Semantic web rule language [ 10 ] can efficiently extract the dataset features and increase the model efficiency. Linear programming-based approaches [ 13 , 42 ] are employed for achieving quality decision making in recommender models. Sequential pattern analysis [ 14 ] is applied to find suitable patterns among data items. This helps in increasing model efficiency. The probabilistic model [ 24 ] is a famous tool to handle uncertainty in risk computations and performance assessment. It offers better decision-making capabilities. K-nearest neighbours (KNN) [ 19 , 37 , 44 ] technique provides faster computation time, simplicity and ease of interpretation. They are good for classification and regression-based problems and offers more accuracy. Spectral clustering [ 21 ] is also called graph clustering or similarity-based clustering, which mainly focuses on reducing the space dimensionality in identifying the dataset items. Stochastic learning algorithm [ 26 ] solves the real-time challenges of recommender systems. Linear SVM [ 29 , 44 ] efficiently solves the high dimensional problems related to recommender systems. It is a memory-efficient method and works well with a large number of samples having relative separation among the classes. This method has been shown to perform well even when new or unfamiliar data is added. Relational Functional Gradient Boosting [ 39 ] technique efficiently works on the relational dependency of data, which is useful for statical relational learning for collaborative-based recommender systems. Ensemble learning [ 40 ] combines the forecast of two or more models and aims to achieve better performance than any of the single contributing models. It also helps in reducing overfitting problems, which are common in recommender systems.
SDAE [ 41 ] is used for learning the non-linear transformations with different filters for finding suitable data. This aids in increasing the performance of recommender models. Multimodal network learning [ 45 ] is efficient for multi-modal data, representing a combined representation of diverse modalities. Random forest [ 46 , 51 ] is a commonly used approach in comparison with other classifiers. It has been shown to increase accuracy when handling big data. This technique is a collection of decision trees to minimize variance through training on diverse data samples. ANNInit [ 47 ] is a type of artificial neural network-based technique that has the capability of self-learning and generating efficient results. It is independent of the data type and can learn data patterns automatically. HashMap [ 50 ] gives faster access to elements owing to the hashing methodology, which decreases the data processing time and increases the performance of the system. CNN [ 51 ] technique can automatically fetch the significant features of a dataset without any supervision. It is a computationally efficient method and provides accurate recommendations. This technique is also simple and fast for implementation. Multilayer graph data model [ 54 ] is efficient for real-time applications and minimizes the access time through mapping the correlation as edges among nodes and provides superior performance. Singular Value Decomposition [ 56 ] can simplify the input data and increase the efficiency of recommendations by eliminating the noise present in data. Reinforcement learning [ 57 ] is efficient for practical scenarios of recommender systems having large data sizes. It is capable of boosting the model performance by increasing the model accuracy even for large scale datasets. FNN [ 58 ] is one of the artificial neural network techniques which can learn non-linear and complex relationships between items. It has demonstrated a good performance increase when employed in different recommender systems. Knowledge representation learning [ 60 ] systems aim to simplify the model development process by increasing the acquisition efficiency, inferential efficiency, inferential adequacy and representation adequacy. User-based approaches [ 2 , 55 , 59 ] specialize in detecting user-related meta-data which is employed to increase the overall model performance. This technique is more suitable for real-time applications where it can capture user feedback and use it to increase the user experience.
Optimization-based techniques
The Foraging Bees [ 11 ] technique enables both functional and combinational optimization for random searching in recommender models. Artificial bee colony [ 12 ] is a swarm-based meta-heuristic technique that provides features like faster convergence rate, the ability to handle the objective with stochastic nature, ease for incorporating with other algorithms, usage of fewer control parameters, strong robustness, high flexibility and simplicity. Particle Swarm Optimization [ 23 ] is a computation optimization technique that offers better computational efficiency, robustness in control parameters, and is easy and simple to implement in recommender systems. Portfolio optimization algorithm [ 27 ] is a subclass of optimization algorithms that find its application in stock investment recommender systems. It works well in real-time and helps in the diversification of the portfolio for maximum profit. The artificial immune system [ 31 ]a is computationally intelligent machine learning technique. This technique can learn new patterns in the data and optimize the overall system parameters. Expectation maximization (EM) [ 32 , 36 , 38 ] is an iterative algorithm that guarantees the likelihood of finding the maximum parameters when the input variables are unknown. Delphi panel and repertory grid [ 33 ] offers efficient decision making by solving the dimensionality problem and data sparsity issues of recommender systems. The Firefly algorithm (FA) [ 48 ] provides fast results and increases recommendation efficiency. It is capable of reducing the number of iterations required to solve specific recommender problems. It also provides both local and global sets of solutions. Beetle Antennae Search (BAS) [ 49 ] offers superior search accuracy and maintains less time complexity that promotes the performance of recommendations. Many-objective evolutionary algorithm (MaOEA) [ 52 ] is applicable for real-time, multi-objective, search-related recommender systems. The introduction of a local search operator increases the convergence rate and gets suitable results. Genetic Algorithm (GA) [ 2 , 22 , 25 , 53 ] based techniques are used to solve the multi-objective optimization problems of recommender systems. They employ probabilistic transition rules and have a simpler operation that provides better recommender performance.
Features and challenges
The features and challenges of the existing recommender models are given in Table 6 .
Simulation platforms
The various simulation platforms used for developing different recommender systems with different applications are given in Fig. 9 .
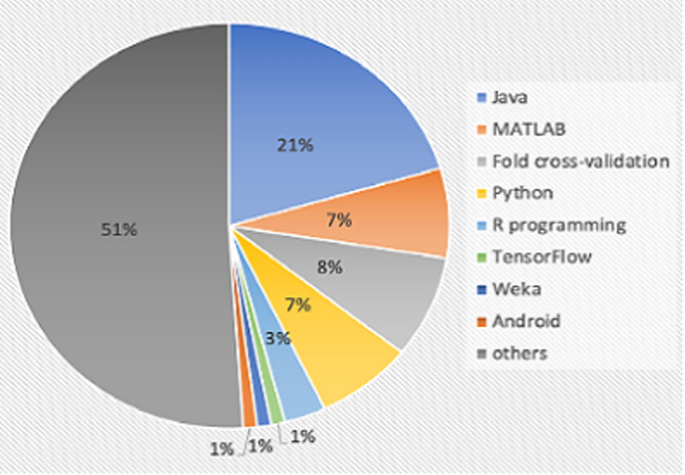
Simulation platforms used for developing different recommender systems
Here, the Java platform is used in 20% of the contributions, MATLAB is implemented in 7% of the contributions, different fold cross-validation are used in 8% of the contributions, 7% of the contributions are utilized by the python platform, 3% of the contributions employ R-programming and 1% of the contributions are developed by Tensorflow, Weka and Android environments respectively. Other simulation platforms like Facebook, web UI (User Interface), real-time environments, etc. are used in 50% of the contributions. Table 7 describes some simulation platforms commonly used for developing recommender systems.
Application focused and dataset description
This section provides an analysis of the different applications focused on a set of recent recommender systems and their dataset details.
Recent recommender systems were analysed and found that 11% of the contributions are focused on the domain of healthcare, 10% of the contributions are on movie recommender systems, 5% of the contributions come from music recommender systems, 6% of the contributions are focused on e-learning recommender systems, 8% of the contributions are used for online product recommender systems, 3% of the contributions are focused on book recommendations and 1% of the contributions are focused on Job and knowledge management recommender systems. 5% of the contributions concentrated on social network recommender systems, 10% of the contributions are focused on tourist and hotels recommender systems, 6% of the contributions are employed for stock recommender systems, and 3% of the contributions contributed for video recommender systems. The remaining 12% of contributions are miscellaneous recommender systems like Twitter, venue-based recommender systems, etc. Similarly, different datasets are gathered for recommender systems based on their application types. A detailed description is provided in Table 8 .
Performance analysis of state-of-art recommender systems
The performance evaluation metrics used for the analysis of different recommender systems is depicted in Table 9 . From the set of research works, 35% of the works use recall measure, 16% of the works employ Mean Absolute Error (MAE), 11% of the works take Root Mean Square Error (RMSE), 41% of the papers consider precision, 30% of the contributions analyse F1-measure, 31% of the works apply accuracy and 6% of the works employ coverage measure to validate the performance of the recommender systems. Moreover, some additional measures are also considered for validating the performance in a few applications.
Research gaps and challenges
In the recent decade, recommender systems have performed well in solving the problem of information overload and has become the more appropriate tool for multiple areas such as psychology, mathematics, computer science, etc. [ 80 ]. However, current recommender systems face a variety of challenges which are stated as follows, and discussed below:
Deployment challenges such as cold start, scalability, sparsity, etc. are already discussed in Sect. 3.
Challenges faced when employing different recommender algorithms for different applications.
Challenges in collecting implicit user data
Challenges in handling real-time user feedback.
Challenges faced in choosing the correct implementation techniques.
Challenges faced in measuring system performance.
Challenges in implementing recommender system for diverse applications.
Numerous recommender algorithms have been proposed on novel emerging dimensions which focus on addressing the existing limitations of recommender systems. A good recommender system must increase the recommendation quality based on user preferences. However, a specific recommender algorithm is not always guaranteed to perform equally for different applications. This encourages the possibility of employing different recommender algorithms for different applications, which brings along a lot of challenges. There is a need for more research to alleviate these challenges. Also, there is a large scope of research in recommender applications that incorporate information from different interactive online sites like Facebook, Twitter, shopping sites, etc. Some other areas for emerging research may be in the fields of knowledge-based recommender systems, methods for seamlessly processing implicit user data and handling real-time user feedback to recommend items in a dynamic environment.
Some of the other research areas like deep learning-based recommender systems, demographic filtering, group recommenders, cross-domain techniques for recommender systems, and dimensionality reduction techniques are also further required to be studied [ 83 ]. Deep learning-based recommender systems have recently gained much popularity. Future research areas in this field can integrate the well-performing deep learning models with new variants of hybrid meta-heuristic approaches.
During this review, it was observed that even though recent recommender systems have demonstrated good performance, there is no single standardized criteria or method which could be used to evaluate the performance of all recommender systems. System performance is generally measured by different evaluation matrices which makes it difficult to compare. The application of recommender systems in real-time applications is growing. User satisfaction and personalization play a very important role in the success of such recommender systems. There is a need for some new evaluation criteria which can evaluate the level of user satisfaction in real-time. New research should focus on capturing real-time user feedback and use the information to change the recommendation process accordingly. This will aid in increasing the quality of recommendations.
Conclusion and future scope
Recommender systems have attracted the attention of researchers and academicians. In this paper, we have identified and prudently reviewed research papers on recommender systems focusing on diverse applications, which were published between 2011 and 2021. This review has gathered diverse details like different application fields, techniques used, simulation tools used, diverse applications focused, performance metrics, datasets used, system features, and challenges of different recommender systems. Further, the research gaps and challenges were put forward to explore the future research perspective on recommender systems. Overall, this paper provides a comprehensive understanding of the trend of recommender systems-related research and to provides researchers with insight and future direction on recommender systems. The results of this study have several practical and significant implications:
Based on the recent-past publication rates, we feel that the research of recommender systems will significantly grow in the future.
A large number of research papers were identified in movie recommendations, whereas health, tourism and education-related recommender systems were identified in very few numbers. This is due to the availability of movie datasets in the public domain. Therefore, it is necessary to develop datasets in other fields also.
There is no standard measure to compute the performance of recommender systems. Among 60 papers, 21 used recall, 10 used MAE, 25 used precision, 18 used F1-measure, 19 used accuracy and only 7 used RMSE to calculate system performance. Very few systems were found to excel in two or more matrices.
Java and Python (with a combined contribution of 27%) are the most common programming languages used to develop recommender systems. This is due to the availability of a large number of standard java and python libraries which aid in the development process.
Recently a large number of hybrid and optimizations techniques are being proposed for recommender systems. The performance of a recommender system can be greatly improved by applying optimization techniques.
There is a large scope of research in using neural networks and deep learning-based methods for developing recommender systems. Systems developed using these methods are found to achieve high-performance accuracy.
This research will provide a guideline for future research in the domain of recommender systems. However, this research has some limitations. Firstly, due to the limited amount of manpower and time, we have only reviewed papers published in journals focusing on computer science, management and medicine. Secondly, we have reviewed only English papers. New research may extend this study to cover other journals and non-English papers. Finally, this review was conducted based on a search on only six descriptors: “Recommender systems”, “Recommendation systems”, “Movie Recommend*”, “Music Recommend*”, “Personalized Recommend*” and “Hybrid Recommend*”. Research papers that did not include these keywords were not considered. Future research can include adding some additional descriptors and keywords for searching. This will allow extending the research to cover more diverse articles on recommender systems.
Availability of data and materials
Not applicable.
Castellano G, Fanelli AM, Torsello MA. NEWER: A system for neuro-fuzzy web recommendation. Appl Soft Comput. 2011;11:793–806.
Article Google Scholar
Crespo RG, Martínez OS, Lovelle JMC, García-Bustelo BCP, Gayo JEL, Pablos PO. Recommendation system based on user interaction data applied to intelligent electronic books. Computers Hum Behavior. 2011;27:1445–9.
Lin FC, Yu HW, Hsu CH, Weng TC. Recommendation system for localized products in vending machines. Expert Syst Appl. 2011;38:9129–38.
Wang SL, Wu CY. Application of context-aware and personalized recommendation to implement an adaptive ubiquitous learning system. Expert Syst Appl. 2011;38:10831–8.
García-Crespo Á, López-Cuadrado JL, Colomo-Palacios R, González-Carrasco I, Ruiz-Mezcua B. Sem-Fit: A semantic based expert system to provide recommendations in the tourism domain. Expert Syst Appl. 2011;38:13310–9.
Dong H, Hussain FK, Chang E. A service concept recommendation system for enhancing the dependability of semantic service matchmakers in the service ecosystem environment. J Netw Comput Appl. 2011;34:619–31.
Li M, Liu L, Li CB. An approach to expert recommendation based on fuzzy linguistic method and fuzzy text classification in knowledge management systems. Expert Syst Appl. 2011;38:8586–96.
Lorenzi F, Bazzan ALC, Abel M, Ricci F. Improving recommendations through an assumption-based multiagent approach: An application in the tourism domain. Expert Syst Appl. 2011;38:14703–14.
Huang Z, Lu X, Duan H. Context-aware recommendation using rough set model and collaborative filtering. Artif Intell Rev. 2011;35:85–99.
Chen RC, Huang YH, Bau CT, Chen SM. A recommendation system based on domain ontology and SWRL for anti-diabetic drugs selection. Expert Syst Appl. 2012;39:3995–4006.
Mohanraj V, Chandrasekaran M, Senthilkumar J, Arumugam S, Suresh Y. Ontology driven bee’s foraging approach based self-adaptive online recommendation system. J Syst Softw. 2012;85:2439–50.
Hsu CC, Chen HC, Huang KK, Huang YM. A personalized auxiliary material recommendation system based on learning style on facebook applying an artificial bee colony algorithm. Comput Math Appl. 2012;64:1506–13.
Gemmell J, Schimoler T, Mobasher B, Burke R. Resource recommendation in social annotation systems: A linear-weighted hybrid approach. J Comput Syst Sci. 2012;78:1160–74.
Article MathSciNet Google Scholar
Choi K, Yoo D, Kim G, Suh Y. A hybrid online-product recommendation system: Combining implicit rating-based collaborative filtering and sequential pattern analysis. Electron Commer Res Appl. 2012;11:309–17.
Garibaldi JM, Zhou SM, Wang XY, John RI, Ellis IO. Incorporation of expert variability into breast cancer treatment recommendation in designing clinical protocol guided fuzzy rule system models. J Biomed Inform. 2012;45:447–59.
Salehi M, Kmalabadi IN. A hybrid attribute–based recommender system for e–learning material recommendation. IERI Procedia. 2012;2:565–70.
Aher SB, Lobo LMRJ. Combination of machine learning algorithms for recommendation of courses in e-learning System based on historical data. Knowl-Based Syst. 2013;51:1–14.
Kardan AA, Ebrahimi M. A novel approach to hybrid recommendation systems based on association rules mining for content recommendation in asynchronous discussion groups. Inf Sci. 2013;219:93–110.
Chang JH, Lai CF, Wang MS, Wu TY. A cloud-based intelligent TV program recommendation system. Comput Electr Eng. 2013;39:2379–99.
Lucas JP, Luz N, Moreno MN, Anacleto R, Figueiredo AA, Martins C. A hybrid recommendation approach for a tourism system. Expert Syst Appl. 2013;40:3532–50.
Niu J, Zhu L, Zhao X, Li H. Affivir: An affect-based Internet video recommendation system. Neurocomputing. 2013;120:422–33.
Liu L, Xu J, Liao SS, Chen H. A real-time personalized route recommendation system for self-drive tourists based on vehicle to vehicle communication. Expert Syst Appl. 2014;41:3409–17.
Bakshi S, Jagadev AK, Dehuri S, Wang GN. Enhancing scalability and accuracy of recommendation systems using unsupervised learning and particle swarm optimization. Appl Soft Comput. 2014;15:21–9.
Kim Y, Shim K. TWILITE: A recommendation system for twitter using a probabilistic model based on latent Dirichlet allocation. Inf Syst. 2014;42:59–77.
Wang Z, Yu X, Feng N, Wang Z. An improved collaborative movie recommendation system using computational intelligence. J Vis Lang Comput. 2014;25:667–75.
Kolomvatsos K, Anagnostopoulos C, Hadjiefthymiades S. An efficient recommendation system based on the optimal stopping theory. Expert Syst Appl. 2014;41:6796–806.
Gottschlich J, Hinz O. A decision support system for stock investment recommendations using collective wisdom. Decis Support Syst. 2014;59:52–62.
Torshizi AD, Zarandi MHF, Torshizi GD, Eghbali K. A hybrid fuzzy-ontology based intelligent system to determine level of severity and treatment recommendation for benign prostatic hyperplasia. Comput Methods Programs Biomed. 2014;113:301–13.
Zahálka J, Rudinac S, Worring M. Interactive multimodal learning for venue recommendation. IEEE Trans Multimedia. 2015;17:2235–44.
Sankar CP, Vidyaraj R, Kumar KS. Trust based stock recommendation system – a social network analysis approach. Procedia Computer Sci. 2015;46:299–305.
Chen MH, Teng CH, Chang PC. Applying artificial immune systems to collaborative filtering for movie recommendation. Adv Eng Inform. 2015;29:830–9.
Wu H, Pei Y, Li B, Kang Z, Liu X, Li H. Item recommendation in collaborative tagging systems via heuristic data fusion. Knowl-Based Syst. 2015;75:124–40.
Yeh DY, Cheng CH. Recommendation system for popular tourist attractions in Taiwan using delphi panel and repertory grid techniques. Tour Manage. 2015;46:164–76.
Liao SH, Chang HK. A rough set-based association rule approach for a recommendation system for online consumers. Inf Process Manage. 2016;52:1142–60.
Li H, Cui J, Shen B, Ma J. An intelligent movie recommendation system through group-level sentiment analysis in microblogs. Neurocomputing. 2016;210:164–73.
Wu H, Yue K, Pei Y, Li B, Zhao Y, Dong F. Collaborative topic regression with social trust ensemble for recommendation in social media systems. Knowl-Based Syst. 2016;97:111–22.
Adeniyi DA, Wei Z, Yongquan Y. Automated web usage data mining and recommendation system using K-Nearest Neighbor (KNN) classification method. Appl Computing Inform. 2016;12:90–108.
Rawat YS, Kankanhalli MS. ClickSmart: A context-aware viewpoint recommendation system for mobile photography. IEEE Trans Circuits Syst Video Technol. 2017;27:149–58.
Yang S, Korayem M, Aljadda K, Grainger T, Natarajan S. Combining content-based and collaborative filtering for job recommendation system: A cost-sensitive Statistical Relational Learning approach. Knowl-Based Syst. 2017;136:37–45.
Lee WP, Chen CT, Huang JY, Liang JY. A smartphone-based activity-aware system for music streaming recommendation. Knowl-Based Syst. 2017;131:70–82.
Wei J, He J, Chen K, Zhou Y, Tang Z. Collaborative filtering and deep learning based recommendation system for cold start items. Expert Syst Appl. 2017;69:29–39.
Li C, Wang Z, Cao S, He L. WLRRS: A new recommendation system based on weighted linear regression models. Comput Electr Eng. 2018;66:40–7.
Mezei J, Nikou S. Fuzzy optimization to improve mobile health and wellness recommendation systems. Knowl-Based Syst. 2018;142:108–16.
Ayata D, Yaslan Y, Kamasak ME. Emotion based music recommendation system using wearable physiological sensors. IEEE Trans Consum Electron. 2018;64:196–203.
Zhao Z, Yang Q, Lu H, Weninger T. Social-aware movie recommendation via multimodal network learning. IEEE Trans Multimedia. 2018;20:430–40.
Hammou BA, Lahcen AA, Mouline S. An effective distributed predictive model with matrix factorization and random forest for big data recommendation systems. Expert Syst Appl. 2019;137:253–65.
Zhao J, Geng X, Zhou J, Sun Q, Xiao Y, Zhang Z, Fu Z. Attribute mapping and autoencoder neural network based matrix factorization initialization for recommendation systems. Knowl-Based Syst. 2019;166:132–9.
Bhaskaran S, Santhi B. An efficient personalized trust based hybrid recommendation (TBHR) strategy for e-learning system in cloud computing. Clust Comput. 2019;22:1137–49.
Han Y, Han Z, Wu J, Yu Y, Gao S, Hua D, Yang A. Artificial intelligence recommendation system of cancer rehabilitation scheme based on IoT technology. IEEE Access. 2020;8:44924–35.
Kang S, Jeong C, Chung K. Tree-based real-time advertisement recommendation system in online broadcasting. IEEE Access. 2020;8:192693–702.
Ullah F, Zhang B, Khan RU. Image-based service recommendation system: A JPEG-coefficient RFs approach. IEEE Access. 2020;8:3308–18.
Cai X, Hu Z, Zhao P, Zhang W, Chen J. A hybrid recommendation system with many-objective evolutionary algorithm. Expert Syst Appl. 2020. https://doi.org/10.1016/j.eswa.2020.113648 .
Esteban A, Zafra A, Romero C. Helping university students to choose elective courses by using a hybrid multi-criteria recommendation system with genetic optimization. Knowledge-Based Syst. 2020;194:105385.
Mondal S, Basu A, Mukherjee N. Building a trust-based doctor recommendation system on top of multilayer graph database. J Biomed Inform. 2020;110:103549.
Dhelim S, Ning H, Aung N, Huang R, Ma J. Personality-aware product recommendation system based on user interests mining and metapath discovery. IEEE Trans Comput Soc Syst. 2021;8:86–98.
Bhalse N, Thakur R. Algorithm for movie recommendation system using collaborative filtering. Materials Today: Proceedings. 2021. https://doi.org/10.1016/j.matpr.2021.01.235 .
Ke G, Du HL, Chen YC. Cross-platform dynamic goods recommendation system based on reinforcement learning and social networks. Appl Soft Computing. 2021;104:107213.
Chen X, Liu D, Xiong Z, Zha ZJ. Learning and fusing multiple user interest representations for micro-video and movie recommendations. IEEE Trans Multimedia. 2021;23:484–96.
Afolabi AO, Toivanen P. Integration of recommendation systems into connected health for effective management of chronic diseases. IEEE Access. 2019;7:49201–11.
He M, Wang B, Du X. HI2Rec: Exploring knowledge in heterogeneous information for movie recommendation. IEEE Access. 2019;7:30276–84.
Bobadilla J, Serradilla F, Hernando A. Collaborative filtering adapted to recommender systems of e-learning. Knowl-Based Syst. 2009;22:261–5.
Russell S, Yoon V. Applications of wavelet data reduction in a recommender system. Expert Syst Appl. 2008;34:2316–25.
Campos LM, Fernández-Luna JM, Huete JF. A collaborative recommender system based on probabilistic inference from fuzzy observations. Fuzzy Sets Syst. 2008;159:1554–76.
Funk M, Rozinat A, Karapanos E, Medeiros AKA, Koca A. In situ evaluation of recommender systems: Framework and instrumentation. Int J Hum Comput Stud. 2010;68:525–47.
Porcel C, Moreno JM, Herrera-Viedma E. A multi-disciplinar recommender system to advice research resources in University Digital Libraries. Expert Syst Appl. 2009;36:12520–8.
Bobadilla J, Serradilla F, Bernal J. A new collaborative filtering metric that improves the behavior of recommender systems. Knowl-Based Syst. 2010;23:520–8.
Ochi P, Rao S, Takayama L, Nass C. Predictors of user perceptions of web recommender systems: How the basis for generating experience and search product recommendations affects user responses. Int J Hum Comput Stud. 2010;68:472–82.
Olmo FH, Gaudioso E. Evaluation of recommender systems: A new approach. Expert Syst Appl. 2008;35:790–804.
Zhen L, Huang GQ, Jiang Z. An inner-enterprise knowledge recommender system. Expert Syst Appl. 2010;37:1703–12.
Göksedef M, Gündüz-Öğüdücü S. Combination of web page recommender systems. Expert Syst Appl. 2010;37(4):2911–22.
Shao B, Wang D, Li T, Ogihara M. Music recommendation based on acoustic features and user access patterns. IEEE Trans Audio Speech Lang Process. 2009;17:1602–11.
Shin C, Woo W. Socially aware tv program recommender for multiple viewers. IEEE Trans Consum Electron. 2009;55:927–32.
Lopez-Carmona MA, Marsa-Maestre I, Perez JRV, Alcazar BA. Anegsys: An automated negotiation based recommender system for local e-marketplaces. IEEE Lat Am Trans. 2007;5:409–16.
Yap G, Tan A, Pang H. Discovering and exploiting causal dependencies for robust mobile context-aware recommenders. IEEE Trans Knowl Data Eng. 2007;19:977–92.
Meo PD, Quattrone G, Terracina G, Ursino D. An XML-based multiagent system for supporting online recruitment services. IEEE Trans Syst Man Cybern. 2007;37:464–80.
Khusro S, Ali Z, Ullah I. Recommender systems: Issues, challenges, and research opportunities. Inform Sci Appl. 2016. https://doi.org/10.1007/978-981-10-0557-2_112 .
Blanco-Fernandez Y, Pazos-Arias JJ, Gil-Solla A, Ramos-Cabrer M, Lopez-Nores M. Providing entertainment by content-based filtering and semantic reasoning in intelligent recommender systems. IEEE Trans Consum Electron. 2008;54:727–35.
Isinkaye FO, Folajimi YO, Ojokoh BA. Recommendation systems: Principles, methods and evaluation. Egyptian Inform J. 2015;16:261–73.
Yoshii K, Goto M, Komatani K, Ogata T, Okuno HG. An efficient hybrid music recommender system using an incrementally trainable probabilistic generative model. IEEE Trans Audio Speech Lang Process. 2008;16:435–47.
Wei YZ, Moreau L, Jennings NR. Learning users’ interests by quality classification in market-based recommender systems. IEEE Trans Knowl Data Eng. 2005;17:1678–88.
Bjelica M. Towards TV recommender system: experiments with user modeling. IEEE Trans Consum Electron. 2010;56:1763–9.
Setten MV, Veenstra M, Nijholt A, Dijk BV. Goal-based structuring in recommender systems. Interact Comput. 2006;18:432–56.
Adomavicius G, Tuzhilin A. Toward the next generation of recommender systems: a survey of the state-of-the-art and possible extensions. IEEE Trans Knowl Data Eng. 2005;17:734–49.
Symeonidis P, Nanopoulos A, Manolopoulos Y. Providing justifications in recommender systems. IEEE Transactions on Systems, Man, and Cybernetics - Part A: Systems and Humans. 2009;38:1262–72.
Zhan J, Hsieh C, Wang I, Hsu T, Liau C, Wang D. Privacy preserving collaborative recommender systems. IEEE Trans Syst Man Cybernet. 2010;40:472–6.
Burke R. Hybrid recommender systems: survey and experiments. User Model User-Adap Inter. 2002;12:331–70.
Article MATH Google Scholar
Gunes I, Kaleli C, Bilge A, Polat H. Shilling attacks against recommender systems: a comprehensive survey. Artif Intell Rev. 2012;42:767–99.
Park DH, Kim HK, Choi IY, Kim JK. A literature review and classification of recommender systems research. Expert Syst Appl. 2012;39:10059–72.
Download references
Acknowledgements
We thank our colleagues from Assam Down Town University who provided insight and expertise that greatly assisted this research, although they may not agree with all the interpretations and conclusions of this paper.
No funding was received to assist with the preparation of this manuscript.
Author information
Authors and affiliations.
Department of Computer Science & Engineering, Assam Down Town University, Panikhaiti, Guwahati, 781026, Assam, India
Deepjyoti Roy & Mala Dutta
You can also search for this author in PubMed Google Scholar
Contributions
DR carried out the review study and analysis of the existing algorithms in the literature. MD has been involved in drafting the manuscript or revising it critically for important intellectual content. Both authors read and approved the final manuscript.
Corresponding author
Correspondence to Deepjyoti Roy .
Ethics declarations
Ethics approval and consent to participate, consent for publication, competing interests.
On behalf of all authors, the corresponding author states that there is no conflict of interest.
Additional information
Publisher's note.
Springer Nature remains neutral with regard to jurisdictional claims in published maps and institutional affiliations.
Rights and permissions
Open Access This article is licensed under a Creative Commons Attribution 4.0 International License, which permits use, sharing, adaptation, distribution and reproduction in any medium or format, as long as you give appropriate credit to the original author(s) and the source, provide a link to the Creative Commons licence, and indicate if changes were made. The images or other third party material in this article are included in the article's Creative Commons licence, unless indicated otherwise in a credit line to the material. If material is not included in the article's Creative Commons licence and your intended use is not permitted by statutory regulation or exceeds the permitted use, you will need to obtain permission directly from the copyright holder. To view a copy of this licence, visit http://creativecommons.org/licenses/by/4.0/ .
Reprints and permissions
About this article
Cite this article.
Roy, D., Dutta, M. A systematic review and research perspective on recommender systems. J Big Data 9 , 59 (2022). https://doi.org/10.1186/s40537-022-00592-5
Download citation
Received : 04 October 2021
Accepted : 28 March 2022
Published : 03 May 2022
DOI : https://doi.org/10.1186/s40537-022-00592-5
Share this article
Anyone you share the following link with will be able to read this content:
Sorry, a shareable link is not currently available for this article.
Provided by the Springer Nature SharedIt content-sharing initiative
- Recommender system
- Machine learning
- Content-based filtering
- Collaborative filtering
- Deep learning
Literature Review on Recommender Systems: Techniques, Trends and Challenges
- Conference paper
- First Online: 25 May 2023
- Cite this conference paper
- Fethi Fkih 15 , 16 &
- Delel Rhouma 15 , 16
Part of the book series: Lecture Notes in Networks and Systems ((LNNS,volume 647))
Included in the following conference series:
- International Conference on Hybrid Intelligent Systems
685 Accesses
Nowadays, Recommender Systems (RSs) have become a necessity especially with the rapid increasing of the numerical data volume. In fact, internet’s users need an automatic system that help them to filter the huge flow of information provided bay websites or even by research engines. Therefore, a Recommender System can be seen as an Information Retrieval system that can respond to an implicit user’s query. The RS draw this implicit user’s query based on a user’s profile that can be created using some semantic or statistic knowledge. In this paper, we provide an in-depth literature review on main RS approaches. Basically, RS’s techniques can be divide into three classes: collaborative Filtering-based, Content-based and hybrid approaches. Also, we show the challenges and the potential trends in this domain.
This is a preview of subscription content, log in via an institution to check access.
Access this chapter
Subscribe and save.
- Get 10 units per month
- Download Article/Chapter or eBook
- 1 Unit = 1 Article or 1 Chapter
- Cancel anytime
- Available as PDF
- Read on any device
- Instant download
- Own it forever
- Available as EPUB and PDF
- Compact, lightweight edition
- Dispatched in 3 to 5 business days
- Free shipping worldwide - see info
Tax calculation will be finalised at checkout
Purchases are for personal use only
Institutional subscriptions
Similar content being viewed by others
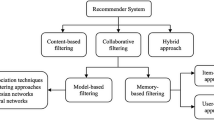
A systematic review and research perspective on recommender systems
Recent Challenges in Recommender Systems: A Survey
A Survey and Classification on Recommendation Systems
Fkih, F., Omri, M.N.: Hybridization of an index based on concept lattice with a terminology extraction model for semantic information retrieval guided by WordNet. In: Abraham, A., Haqiq, A., Alimi, A., Mezzour, G., Rokbani, N., Muda, A. (eds.) Proceedings of the 16th International Conference on Hybrid Intelligent Systems (HIS 2016). HIS 2016. Advances in Intelligent Systems and Computing, vol. 552. Springer, Cham (2017)
Google Scholar
Fkih, F., Omri, M.N.: Information retrieval from unstructured web text document based on automatic learning of the threshold. Int. J. Inf. Retr. Res. (IJIRR) 2 (4) (2012)
Ricci, F., Rokach, L., Shapira, B.: Introduction to recommender systems handbook. In: Recommender Systems Handbook, pp. 1–35. Springer, Boston, MA (2011)
Gandhi, S., Gandhi, M.: Hybrid recommendation system with collaborative filtering and association rule mining using big data. In: 2018 3rd International Conference for Convergence in Technology (I2CT). IEEE (2018)
Lee, S.-J., et al.: A movie rating prediction system of user propensity analysis based on collaborative filtering and fuzzy system. J. Korean Inst. Intell. Syst. 19 (2), 242–247 (2009)
Tian, Y., et al.: College library personalized recommendation system based on hybrid recommendation algorithm. Procedia CIRP 83 , 490–494 (2019)
Schafer, J.B., et al.: Collaborative filtering recommender systems. In: The Adaptive Web. Springer, Berlin, Heidelberg (2007)
Cacheda, F., et al.: Comparison of collaborative filtering algorithms: limitations of current techniques and proposals for scalable, high-performance recommender systems. ACM Trans. Web (TWEB) 5 (1), 2 (2011)
Fkih, F.: Similarity measures for collaborative filtering-based recommender systems: review and experimental comparison. J. King Saud Univ. - Comput. Inf. Sci. (2021)
Resnick, P., et al.: GroupLens: an open architecture for collaborative filtering of netnews. In: Proceedings of the 1994 ACM Conference on Computer Supported Cooperative Work. ACM (1994)
Sarwar, B.M., et al.: Item-based collaborative filtering recommendation algorithms. Www 1 , 285–295 (2001)
Zhu, Y.: A book recommendation algorithm based on collaborative filtering. In: 2016 5th International Conference on Computer Science and Network Technology (ICCSNT). IEEE (2016)
Lin, W., Alvarez, S.A., Ruiz, C.: Collaborative recommendation via adaptive association rule mining. Data Min. Knowl. Disc. 6 , 83–105 (2000)
Article Google Scholar
Sandvig, J.J., Mobasher, B., Burke, R.: Robustness of collaborative recommendation based on association rule mining. In: Proceedings of the 2007 ACM Conference on Recommender systems. ACM (2007)
Sieg, A., Mobasher, B., Burke, R.: Improving the effectiveness of collaborative recommendation with ontology-based user profiles. In: Proceedings of the 1st International Workshop on Information Heterogeneity and Fusion in Recommender Systems. ACM (2010)
Kurmashov, N., Latuta, K., Nussipbekov, A.: Online book recommendation system. In: 2015 Twelve International Conference on Electronics Computer and Computation (ICECCO). IEEE (2015)
Kanetkar, S., et al.: Web-based personalized hybrid book recommendation system. In: 2014 International Conference on Advances in Engineering & Technology Research (ICAETR-2014). IEEE (2014)
Mooney, R.J., Roy, L.: Content-based book recommending using learning for text categorization. In: Proceedings of the Fifth ACM Conference on Digital Libraries. ACM (2000)
Burke, R.: Hybrid web recommender systems. In: The Adaptive Web, pp. 377–408. Springer, Berlin, Heidelberg (2007)
Chandak, M., Girase, S., Mukhopadhyay, D.: Introducing hybrid technique for optimization of book recommender system. Procedia Comput. Sci. 45 , 23–31 (2015)
Ouni, S., Fkih, F., Omri, M.N.: BERT- and CNN-based TOBEAT approach for unwelcome tweets detection. Soc. Netw. Anal. Min. 12 , 144 (2022)
Ouni, S., Fkih, F., Omri, M.N.: Novel semantic and statistic features-based author profiling approach. J. Ambient Intell. Hum. Comput. (2022)
Ouni, S., Fkih, F., Omri, M.N.: Bots and gender detection on Twitter using stylistic features. In: Bădică, C., Treur, J., Benslimane, D., Hnatkowska, B., Krótkiewicz, M. (eds.) Advances in Computational Collective Intelligence. ICCCI 2022. Communications in Computer and Information Science, vol. 1653. Springer, Cham (2022)
Download references
Author information
Authors and affiliations.
Department of Computer Science, College of Computer, Qassim University, Buraydah, Saudi Arabia
Fethi Fkih & Delel Rhouma
MARS Research Laboratory LR17ES05, University of Sousse, Sousse, Tunisia
You can also search for this author in PubMed Google Scholar
Corresponding author
Correspondence to Fethi Fkih .
Editor information
Editors and affiliations.
Faculty of Computing and Data Science, FLAME University, Pune, Maharashtra, India
Ajith Abraham
National University of Kaohsiung, Kaohsiung, Taiwan
Tzung-Pei Hong
Symbiosis International University, Pune, India
Ketan Kotecha
University of Jinan, Jinan, China
Scientific Network for Innovation and Research Excellence, Machine Intelligence Research Labs, Mala, Kerala, India
Pooja Manghirmalani Mishra
Scientific Network for Innovation and Research Excellence, Machine Intelligence Research Labs, Auburn, WA, USA
Niketa Gandhi
Rights and permissions
Reprints and permissions
Copyright information
© 2023 The Author(s), under exclusive license to Springer Nature Switzerland AG
About this paper
Cite this paper.
Fkih, F., Rhouma, D. (2023). Literature Review on Recommender Systems: Techniques, Trends and Challenges. In: Abraham, A., Hong, TP., Kotecha, K., Ma, K., Manghirmalani Mishra, P., Gandhi, N. (eds) Hybrid Intelligent Systems. HIS 2022. Lecture Notes in Networks and Systems, vol 647. Springer, Cham. https://doi.org/10.1007/978-3-031-27409-1_44
Download citation
DOI : https://doi.org/10.1007/978-3-031-27409-1_44
Published : 25 May 2023
Publisher Name : Springer, Cham
Print ISBN : 978-3-031-27408-4
Online ISBN : 978-3-031-27409-1
eBook Packages : Intelligent Technologies and Robotics Intelligent Technologies and Robotics (R0)
Share this paper
Anyone you share the following link with will be able to read this content:
Sorry, a shareable link is not currently available for this article.
Provided by the Springer Nature SharedIt content-sharing initiative
- Publish with us
Policies and ethics
- Find a journal
- Track your research

An official website of the United States government
The .gov means it’s official. Federal government websites often end in .gov or .mil. Before sharing sensitive information, make sure you’re on a federal government site.
The site is secure. The https:// ensures that you are connecting to the official website and that any information you provide is encrypted and transmitted securely.
- Publications
- Account settings
The PMC website is updating on October 15, 2024. Learn More or Try it out now .
- Advanced Search
- Journal List
- Springer Nature - PMC COVID-19 Collection

A systematic literature review on educational recommender systems for teaching and learning: research trends, limitations and opportunities
Felipe leite da silva.
1 Centro de Estudos Interdisciplinares em Novas Tecnologias da Educação, Universidade Federal do Rio Grande do Sul, Porto Alegre, Rio Grande do Sul Brazil
Bruna Kin Slodkowski
Ketia kellen araújo da silva, sílvio césar cazella.
2 Departamento de Ciências Exatas e Sociais Aplicadas, Universidade Federal de Ciências da Saúde de Porto Alegre, Porto Alegre, Rio Grande do Sul Brazil
Associated Data
The datasets generated during the current study correspond to the papers identified through the systematic literature review and the quality evaluation results (refer to Section 3.4 in paper). They are available from the corresponding author on reasonable request.
Recommender systems have become one of the main tools for personalized content filtering in the educational domain. Those who support teaching and learning activities, particularly, have gained increasing attention in the past years. This growing interest has motivated the emergence of new approaches and models in the field, in spite of it, there is a gap in literature about the current trends on how recommendations have been produced, how recommenders have been evaluated as well as what are the research limitations and opportunities for advancement in the field. In this regard, this paper reports the main findings of a systematic literature review covering these four dimensions. The study is based on the analysis of a set of primary studies ( N = 16 out of 756, published from 2015 to 2020) included according to defined criteria. Results indicate that the hybrid approach has been the leading strategy for recommendation production. Concerning the purpose of the evaluation, the recommenders were evaluated mainly regarding the quality of accuracy and a reduced number of studies were found that investigated their pedagogical effectiveness. This evidence points to a potential research opportunity for the development of multidimensional evaluation frameworks that effectively support the verification of the impact of recommendations on the teaching and learning process. Also, we identify and discuss main limitations to clarify current difficulties that demand attention for future research.
Supplementary Information
The online version contains supplementary material available at 10.1007/s10639-022-11341-9.
Introduction
Digital technologies are increasingly integrated into different application domains. Particularly in education, there is a vast interest in using them as mediators of the teaching and learning process. In such a task, the computational apparatus serves as an instrument to support human knowledge acquisition from different educational methodologies and pedagogical practices (Becker, 1993 ).
In this sense, Educational Recommender Systems (ERS) play an important role for both educators and students (Maria et al., 2019 ). For instructors, these systems can contribute to their pedagogical practices through recommendations that improve their planning and assist in educational resources filtering. As for the learners, through preferences and educational constraints recognition, recommenders can contribute for their academic performance and motivation by indicating personalized learning content (Garcia-Martinez & Hamou-Lhadj, 2013 ).
Despite the benefits, there are known issues upon the usage of the recommender system in the educational domain. One of the main challenges is to find an appropriate correspondence between the expectations of users and the recommendations (Cazella et al., 2014 ). Difficulties arise from differences in learner’s educational interests and needs (Verbert et al., 2012 ). The variety of student’s individual factors that can influence the learning process (Buder & Schwind, 2012 ) is one of the challenging matters that makes it complex to be overcome. On a recommender standpoint, this reflects an input diversity with potential to tune recommendations for users.
In another perspective, from a technological and artificial intelligence standpoint, the ERS are likely to suffer from already known issues noted on the general-purpose ones, such as the cold start and data sparsity problems (Garcia-Martinez & Hamou-Lhadj, 2013 ). Furthermore, problems are related to the approach used to generate recommendations. For instance, the overspecialization is inherently associated with the way that content-based recommender systems handle data (Iaquinta et al., 2008 ; Khusro et al., 2016 ). These issues pose difficulties to design recommenders that best suit the user’s learning needs and that distance themselves from user’s dissatisfaction in the short and long term.
From an educational point of view, issues emerge on how to evaluate ERS effectiveness. A usual strategy to measure the quality of educational recommenders is to apply the traditional recommender’s evaluation methods (Erdt et al., 2015 ). This approach determines system quality based on performance properties, such as its precision and prediction accuracy. Nevertheless, in the educational domain, system effectiveness needs to take into account the students’ learning performance. This dimension brings new complexities on how to successfully evaluate ERS.
As ERS topic has gradually increased in attraction for scientific community (Zhong et al., 2019 ), extensive research have been carried out in recent years to address these issues (Manouselis et al. 2010 ; Manouselis et al., 2014 ; Tarus et al., 2018 ; George & Lal, 2019 ). ERS has become a field of application and combination of different computational techniques, such as data mining, information filtering and machine learning, among others (Tarus et al., 2018 ). This scenario indicates a diversity in the design and evaluation of recommender systems that support teaching and learning activities. Nonetheless, research is dispersed in literature and there is no recent study that encompasses the current scientific efforts in the field that reveals how such issues are addressed in current research. Reviewing evidence, and synthesizing findings of current approaches in how ERS produce recommendations, how ERS are evaluated and what are research limitations and opportunities can provide a panoramic perspective of the research topic and support practitioners and researchers for implementation and future research directions.
From the aforementioned perspective, this work aims to investigate and summarize the main trends and research opportunities on ERS topic through a Systematic Literature Review (SLR). The study was conducted based on the last six years publications, particularly, regarding to recommenders that support teaching and learning process.
Main trends referrer to recent research direction on the ERS field. They are analyzed in regard to how recommender systems produce recommendations and how they are evaluated. As mentioned above, these are significant dimensions related to current issues of the area. Specifically for the recommendation production, this paper provides a three-axis-based analysis centered on systems underlying techniques, input data and results presentation.
Additionally, research opportunities in the field of ERS as well as their main limitations are highlighted. Because current comprehension of these aspects is fragmented in literature, such an analysis can shed light for future studies.
The SLR was carried out using Kitchenham and Charters ( 2007 ) guidelines. The SLR is the main method for summarizing evidence related to a topic or a research question (Kitchenham et al., 2009 ). Kitchenham and Charters ( 2007 ) guidelines, in turn, are one of the leading orientations for reviews on information technology in education (Dermeval et al., 2020 ).
The remainder of this paper is structured as follows. In Section 2 , the related works are presented. Section 3 details the methodology used in carrying out the SLR. Section 4 covers the SLR results and related discussion. Section 5 presents the conclusion.
Related works
In the field of education, there is a growing interest in technologies that support teaching and learning activities. For this purpose, ERS are strategic solutions to provide a personalized educational experience. Research in this sense has attracted the attention of the scientific community and there has been an effort to map and summarize different aspects of the field in the last 6 years.
In Drachsler et al. ( 2015 ) a comprehensive review of technology enhanced learning recommender systems was carried out. The authors analyzed 82 papers published from 2000 to 2014 and provided an overview of the area. Different aspects were analyzed about recommenders’ approach, source of information and evaluation. Additionally, a categorization framework is presented and the study includes the classification of selected papers according to it.
Klašnja-Milićević et al. ( 2015 ) conducted a review on recommendation systems for e-learning environments. The study focuses on requirements, challenges, (dis)advantages of techniques in the design of this type of ERS. An analysis on collaborative tagging systems and their integration in e-learning platform recommenders is also discussed.
Ferreira et al. ( 2017 ) investigated particularities of research on ERS in Brazil. Papers published between 2012 and 2016 in three Brazilian scientific vehicles were analyzed. Rivera et al. ( 2018 ) presented a big picture of the ERS area through a systematic mapping. The study covered a larger set of papers and aimed to detect global characteristics in ERS research. Aiming at the same focus, however, setting different questions and repositories combination, Pinho, Barwaldt, Espíndola, Torres, Pias, Topin, Borba and Oliveira (2019) performed a systematic review on ERS. In these works, it is observed the common concern of providing insights about the systems evaluation methods and the main techniques adopted in the recommendation process.
Nascimento et al. ( 2017 ) carried out a SLR covering learning objects recommender systems based on the user’s learning styles. Learning objects metadata standards, learning style theoretical models, e-learning systems used to provide recommendations and the techniques used by the ERS were investigated.
Tarus et al ( 2018 ) and George and Lal ( 2019 ) concentrated their reviews on ontology-based ERS. Tarus et al. ( 2018 ) examined research distribution in a period from 2005 to 2014 according to their years of publication. Furthermore, the authors summarized the techniques, knowledge representation, ontology types and ontology representations covered in the papers. George and Lal ( 2019 ), in turn, update the contributions of Tarus et al. ( 2018 ), investigating papers published between 2010 and 2019. The authors also discuss how ontology-based ERS can be used to address recommender systems traditional issues, such as cold start problem and rating sparsity.
Ashraf et al. ( 2021 ) directed their attention to investigate course recommendation systems. Through a comprehensive review, the study summarized the techniques and parameters used by this type of ERS. Additionally, a taxonomy of the factors taken into account in the course recommendation process was defined. Salazar et al. ( 2021 ), on the other hand, conducted a review on affectivity-based ERS. Authors presented a macro analysis, identifying the main authors and research trends, and summarized different recommender systems aspects, such as the techniques used in affectivity analysis, the source of affectivity data collection and how to model emotions.
Khanal et al. ( 2019 ) reviewed e-learning recommendation systems based on machine learning algorithms. A total of 10 papers from two scientific vehicles and published between 2016 and 2018 were examined. The study focal point was to investigate four categories of recommenders: those based on collaborative filtering, content-based filtering, knowledge and a hybrid strategy. The dimensions analyzed were the machine learning algorithms used, the recommenders’ evaluation process, inputs and outputs characterization and recommenders’ challenges addressed.
Related works gaps and contribution of this study
The studies presented in the previous section have a diversity of scope and dimensions of analysis, however, in general, they can be classified into two distinct groups. The first, focus on specific subjects of ERS field, such as similar methods of recommendations (George & Lal, 2019 ; Khanal et al., 2019 ; Salazar et al., 2021 ; Tarus et al., 2018 ) and same kind of recommendable resources (Ashraf et al., 2021 ; Nascimento et al., 2017 ). This type of research scrutinizes the particularities of the recommenders and highlights aspects that are difficult to be identified in reviews with a broader scope. Despite that, most of the reviews concentrate on analyses of recommenders’ operational features and have limited discussion on crosswise issues, such as ERS evaluation and presentation approaches. Khanal et al. ( 2019 ), specifically, makes contributions regarding evaluation, but the analysis is limited to four types of recommender systems.
The second group is composed of wider scope reviews and include recommendation models based on a diversity of methods, inputs and outputs strategies (Drachsler et al., 2015 ; Ferreira et al., 2017 ; Klašnja-Milićević et al., 2015 ; Pinho et al., 2019 ; Rivera et al., 2018 ). Due to the very nature of systematic mappings, the research conducted by Ferreira et al. ( 2017 ) and Rivera et al. ( 2018 ) do not reach in depth some topics, for example, the data synthesized on the evaluations of the ERS are delimited to indicate only the methods used. Ferreira et al. ( 2017 ), in particular, aims to investigate only Brazilian recommendation systems, offering partial contributions to an understanding of the state of the art of the area. In Pinho et al. ( 2019 ) it is noted the same limitation of the systematic mappings. The review was reported with a restricted number of pages, making it difficult to detail the findings. On the other hand, Drachsler et al. ( 2015 ) and, Klašnja-Milićević et al. ( 2015 ) carried out comprehensive reviews that summarizes specific and macro dimensions of the area. However, the papers included in their reviews were published until 2014 and there is a gap on the visto que advances and trends in the field in the last 6 years.
Given the above, as far as the authors are aware, there is no wide scope secondary study that aggregate the research achievements on recommendation systems that support teaching and learning in recent years. Moreover, a review in this sense is necessary since personalization has become an important feature in the teaching and learning context and ERS are one of main tools to deal with different educational needs and preferences that affect individuals’ learning process.
In order to widen the frontiers of knowledge in the field of research, this review aims to contribute to the area by presenting a detailed analysis of the following dimensions: how recommendations are produced and presented, how recommender systems are evaluated and what are the studies limitations and research opportunities. Specifically, to summarize the current knowledge, a SLR was conducted based on four research questions (Section 3.1 ). The review focused on papers published from 2015 to 2020 in scientific journals. A quality assessment was performed to select the most mature systems. The data found on the investigated topics are summarized and discussed in Section 4 .
Methodology
This study is based on the SLR methodology for gathering evidences related to the research topic investigated. As stated by Kitchenham and Charters ( 2007 ) and Kitchenham et al. ( 2009 ), this method provides the means for aggregate evidences from current research prioritizing the impartiality and reproducibility of the review. Therefore, a SLR is based on a process that entails the development of a review protocol that guides the selection of relevant studies and the subsequent extraction of data for analysis.
Guidelines for SLR are widely described in literature and the method can be applied for gathering evidences in different domains, such as, medicine and social science (Khan et al., 2003 ; Pai et al., 2004 ; Petticrew & Roberts, 2006 ; Moher et al., 2015 ). Particularly for informatics in education area, Kitchenham and Charters ( 2007 ) guidelines have been reported as one of the main orientations (Dermeval et al, 2020 ). Their approach appears in several studies (Petri & Gresse von Wangenheim, 2017 ; Medeiros et al., 2019 ; Herpich et al, 2019 ) including mappings and reviews on ERS field (Rivera et al., 2018 ; Tarus et al., 2018 ).
As mentioned in Section 1 , Kitchenham and Charters ( 2007 ) guidelines were used in the conducted SLR. They are based on three main stages: the first for planning the review, the second for conducting it and the last for the results report. Following these orientations, the review was structured in three phases with seven main activities distributed among them as depicted in Fig. 1 .

Systematic literature review phases and activities
The first was the planning phase. The identification of the need for a SLR about teaching and learning support recommenders and the development of the review protocol occurred on this stage. In activity 1, the search for SLR with the intended scope of this study was performed. The result did not return compatible papers with this review scope. Papers identified are described in Section 2 . In activity 2, the review process was defined. The protocol was elaborated through rounds of discussion by the authors until consensus was reached. The activity 2 output were the research questions, search strategy, papers selection strategy and the data extraction method.
The next was the conducting phase. At this point, activities for relevant papers identification (activity 3) and selection (activities 4) were executed. In Activity 3, searches were carried out in seven repositories indicated by Dermeval et al. ( 2020 ) as relevant to the area of informatics in education. Authors applied the search string into these repositories search engines, however, due to the large number of returned research, the authors established the limit of 600 to 800 papers that would be analyzed. Thus, three repositories whose sum of search results was within the established limits were chosen. The list of potential repositories considered for this review and the selected ones is listed in Section 3.1 . The search string used is also shown in Section 3.1 .
In activity 4, studies were selected through two steps. In the first, inclusion and exclusion criteria were applied to each identified paper. Accepted papers had they quality assessed in the second step. Parsifal 1 was used to manage planning and conducting phase data. Parsifal is a web system, adhering to Kitchenham and Charters ( 2007 ) guidelines, that helps in SLR conduction. At the end of this step, relevant data were extracted (activity 5) and registered in a spreadsheet. Finally, in the reporting phase, the extracted data were analyzed in order to answer the SLR research questions (activity 6) and the results were recorded in this paper (activity 7).
Research question, search string and repositories
Teaching and learning support recommender systems have particularities of configuration, design and evaluation method. Therefore, the following research questions (Table (Table1) 1 ) were elaborated in an effort to synthesize these knowledge as well as the main limitations and research opportunities in the field from the perspective of the most recent studies:
SLR research questions
Id | Research question | Rationale |
---|---|---|
RQ1 | How teaching and learning support recommender systems produce recommendations? | There is a variety of input parameters and techniques used for building recommenders for teaching and learning (Drachsler et al., ; Manouselis et al., ). They have been proposed in an attempt to find the best approach to match users’ expectations and recommendations. Also, they include by design intrinsic limitations of each strategy (Garcia-Martinez & Hamou-Lhadj, ). Currently, studies are dispersed in literature and, as far as the authors are aware, there are not research synthesizing the knowledge about techniques and inputs used to tackle the field issues. Analyzing the last 6 years trends should clarify the current state of the art on how this kind of recommender has been designed and the latest trends in this sense |
RQ2 | How teaching and learning support recommender systems present recommendations? | Complementarily to RQ1, this research question leads to a broad analysis of the architecture of teaching and learning support recommender systems proposed by the scientific community. This research question adds to RQ1 and widens the insights of current state of the art on how ERS have been designed |
RQ3 | How are teaching and learning support recommender systems evaluated? | There are distinct methods aiming on measuring quality dimensions of an educational recommender and a previous study have suggested a growing awareness of the necessity for the elaboration of educational-focused evaluations in the ERS research field (Erdt et al., ). Analyzing the last 6 years trends on teaching and learning support recommender system evaluation topic will shed light on the current state of the art and will uncover insights of what evaluation goals have been prioritized as well as how recommenders’ pedagogical effectiveness has been measured |
RQ4 | What are the limitations and research opportunities related to the teaching and learning support recommender systems field? | As the ERS research area have been developing through the past years, research limitations that hinder advancements in the field have been reported or can be observed in current studies. Also, an in-depth investigation can inform of under-explored topics that need further investigation taking into account their potential to contribute to the advancement of area. As long as the authors are aware, literature lacks on identifying current limitations and opportunities in teaching and learning support recommender system research. With this research question it is intended to reveal them from the perspective of the last 6 years scientific production. Answering this question can clarify the needs for future research in this topic |
Regarding the search strategy, papers were selected from three digital repositories (Table (Table2). 2 ). For the search, “Education” and “Recommender system” were defined as the keywords and synonyms were derived from them as secondary terms (Table (Table3). 3 ). From these words, the following search string was elaborated:
- ("Education" OR "Educational" OR "E-learning" OR "Learning" OR "Learn") AND ("Recommender system" OR "Recommender systems" OR "Recommendation system" OR "Recommendation systems" OR "Recommending system" OR "Recommending systems")
Repositories considered for the SLR
Name | URL | No of papers | Selected? | Rationale |
---|---|---|---|---|
IEEExplore | 310 | yes | Within defined threshold | |
ACM Digital Library | 60 | yes | ||
Science Direct | 386 | yes | ||
Springer Link | 3.613 | no | Several studies returned exceeding defined threshold | |
Engineering Village | 1.205 | no | ||
Scopus | 1.760 | no | ||
Web of Science | 1018 | no |
Keywords and their synonyms used in the search string
Keyword | Synonym |
---|---|
Educational | Education, E-learning, Learn, Learning |
Recommender system | Recommender systems, Recommendation system, Recommendation systems, Recommending system, Recommending systems |
Inclusion and exclusion criteria
The first step for the selection of papers was performed through the application of objective criteria, thus a set of inclusion and exclusion criteria was defined. The approved papers formed a group that comprises the primary studies with potential relevance for the scope of the SLR. Table Table4 4 lists the defined criteria. In the description column of Table Table4, 4 , the criteria are informed and in the id column they are identified with a code. The latter was defined appending an abbreviation of the respective kind of criteria (IC for Inclusion Criteria and EC for Exclusion Criteria) with an index following the sequence of the list. The Id is used for referencing its corresponding criterion in the rest of this document.
Inclusion and exclusion criteria of the SLR
Inclusion criteria | Exclusion criteria | ||
---|---|---|---|
IC1 | Paper published from 2015 to 2020 | EC1 | Paper published before 2015 and after 2020 |
IC2 | Paper published in scientific journals | EC2 | Paper is not published in scientific journals (ex. conference and workshops) |
IC3 | Paper must be in English language | EC3 | Paper is not in English language |
IC4 | Paper must be a full paper | EC4 | Paper is not a full paper |
IC5 | The search string must be in at least one of the following papers metadata: title, abstract or keywords | EC5 | The search string cannot be found in at least one of the following papers metadata: title, abstract or keywords |
IC6 | Paper should focus on the development of a recommendation system and its application in the educational domain as a tool to support teaching or learning | EC6 | Paper does not focus on the development of a recommendation system and its application in the educational domain as a tool to support teaching or learning |
EC7 | Paper does not present the recommendation system evaluation | ||
IC7 | Paper must present the recommendation system evaluation | EC8 | Paper is not a primary study |
Since the focus of this review is on the analysis of recent ERS publications, only studies from the past 6 years (2015–2020) were screened (see IC1). Targeting mature recommender systems, only full papers from scientific journals that present the recommendation system evaluation were considered (see IC2, IC4 and IC7). Also, solely works written in English language were selected, because they are the most expressive in quantity and are within the reading ability of the authors (see IC3). Search string was verified on papers’ title, abstract and keywords to ensure only studies related to the ERS field were screened (see IC5). The IC6, specifically, delimited the subject of selected papers and aligned it to the scope of the review. Additionally, it prevented the selection of secondary studies in process (e.g., others reviews or systematic mappings). Conversely, exclusion criteria were defined to clarify that papers contrasting with the inclusion criteria should be excluded from review (see EC1 to EC8). Finally, duplicate searches were marked and, when all criteria were met, only the latest was selected.
Quality evaluation
The second step in studies selection activity was the quality evaluation of the papers. A set of questions were defined with answers of different weights to estimate the quality of the studies. The objective of this phase was to filter researches with higher: (i) validity; (ii) details of the context and implications of the research; and (iii) description of the proposed recommenders. Research that detailed the configuration of the experiment and carried out an external validation of the ERS obtained higher weight in the quality assessment. Hence, the questions related to recommender evaluation (QA8 and QA9) ranged from 0 to 3, while the others, from 0 to 2. The questions and their respective answers are presented in Table Table7 7 (see Appendix). Each paper evaluated had a total weight calculated according to Formula 1 :
Quality evaluation questions and answers
Id | Questions | Available Answers (Weight) |
---|---|---|
QA1 | Does the paper clearly present the research contributions? | The paper explicitly lists or describes the research contributions. They are clearly connected with the presented results. (2pts) The paper provides a general description of research contributions. They are clearly connected with the presented results. (1pt) The paper provides a general description of research contributions. They are not clearly connected with the presented results. (0.5pts) The paper does not clearly provide a research contribution. If presented, the contributions are not clearly connected with the results of the study. (0) |
QA2 | Does the paper clearly present how research differs from other related works? | The research is compared in detail with related works. Authors provide the strengths and/or weaknesses of each related work and position their research granularly, stating contributions explicitly. (2pts) The research is compared in detail with related works. Authors provide a general description of the related works and they position their research stating contribution explicitly. (1,0pts) The paper provides a general description of related works. A brief introduction to each study or groups of it is introduced without identifying strengths and/or weaknesses. The authors explain how their research stands out without explicitly comparing it with the related works. (0,5pts) The paper does not position the research in relation to other works in the area. The unique contribution of the study is general or not presented explicitly. (0) |
QA3 | Does the paper clearly present the limitations of the research? | The paper lists or describes the limitations of the study. If the evaluation produces any results that are difficult to explain, the challenges are detailed presented. (2pts) The paper presents the study limitations with a general description. If the evaluation produces any results that are difficult to explain, the paper describes the challenges in a general way. (1,0pts) The paper does not explicitly present or list the limitations of the study. Nonetheless, the paper presents some research-related challenges when discussing the results of the experiments or in the conclusion. (0,5pts) The paper does not present the limitations of the study. If the evaluation presents any results that are difficult to explain, the paper does not describe the challenges. (0) |
QA4 | Does the paper clearly present directions for future work? | The paper explicitly lists or describes directions for future work. These are based on experiment results or limitations explicitly discussed. (2pts) The paper explicitly lists or describes directions for future work. Yet, such directions are not linked with experiment results or the paper does not present the motivations or foundations. (1,0pts) The paper presents directions for future work in general. (0,5pts) The paper does not present directions for future work. (0) |
QA5 | Does the paper clearly present the inputs for the recommendation generation process? | The paper explicitly presents the recommender system input parameters and the way it is collected. When the recommender does not produce recommendations based on a user profile, the authors describe the input elements used. When such information cannot be understood directly, the authors describe in detail each element that composes it and how these elements are obtained (2pts) The paper presents the recommender input parameter through a general description or it is noticed a partial omission of some information, for example, through the use of “etc.” at the end of a list. (1,0pts) The paper does not present the recommender input parameter. (0) |
QA6 | Does the paper clearly present the techniques/methods for the recommendation generation process? | The paper describes the techniques and methods used for the recommendation generation process. They are presented in a continuous flow, beginning with an overview followed by specific details of the proposal. Authors may or may not use one or more illustrations to present the iterations and how the proposed ERS functions in detail. (2pts) The paper describes the techniques and methods used for the recommendation generation process. Yet, these elements are not presented in a continuous flow, beginning with an overview followed by specific details of the proposal. Authors may or may not use one or more illustrations to present the iterations and how the proposed ERS functions in detail. (1,0pts) The paper presents the techniques and methods used for the recommendation generation process in general. The presentation does not have a continuous flow that begins with an overview and then the specific details of the proposal. The author does not use illustrations to present the iterations and the functioning of the proposed recommender or uses illustrations that lack important components crucial for their understanding. (0,5pts) The paper does not present the techniques and methods used in the elaboration of the recommender (0) |
QA7 | Does the paper clearly present the target audience of the recommendations? | The paper explicitly presents the recommender target audience, contextualizes how the research addresses or minimizes a specific issue of them and, whenever possible, provides specific characteristics, such as their age range, education level (e.g., students) or teaching level (e.g., professors). (2pts) The paper explicitly presents recommender target audience and contextualizes how their research resolves or minimizes a specific problem of this audience. However, they do not present the specific characteristics of this audience. (1,0pts) The paper presents a general description of the recommender target audience. (0,5pts) The paper does not specify the recommender target audience (e.g., individuals that use the system are identified only as users). (0) |
QA8 | Does the paper clearly present the setting of the experiment? | The paper explicitly describes the settings of the experiment. All of these main elements are listed: 1) Number of users; 2) Number of items to recommend; 3) Kind of recommended items; 4) Source of the data used. (3pts) The paper explicitly describes the settings of the experiment. Still, one of the following elements is not explained: 1) Number of users; 2) Number of items to be recommended; 3) Kind of recommended items; 4) Source of the data used. (1,5pts) The paper provides a general description of the experiment settings. Yet, it is noted that there is a little detail regarding the experiment configuration and more than one of the following key elements is not explained: 1) Number of users; 2) Number of items to be recommended; 3) Kind of recommended items; 4) Source of the data used. (0,75pts) Authors do not describe the experiment settings. (0) |
QA9 | Does the paper clearly present how the recommender was evaluated? | The paper describes the evaluation steps and if the experiment is based on methodologies and/or theories. It also explicitly presents how the evaluation carried out validates that the research offers direct contributions to the educational development of the user or those who relate to him. It was conducted an external validation through an online evaluation or an experiment based on control/experimental groups. (3pts) The paper describes the evaluation steps and if the experiment is based on methodologies and/or theories. It also explicitly presents how the evaluation carried out validates that the research offers direct contributions to the educational development of the user or those who relate to him. It was conducted an internal validation through an offline evaluation followed or not by questionnaire-based user study. (2,5pts) The paper describes the evaluation steps, but it does not explicitly justify the used approach. It also explicitly presents how the evaluation carried out validates that the research offers direct contributions to the educational development of the user or those who relate to him. It was conducted either an internal or external validation. (2,0pts) The paper describes the evaluation steps and if the experiment is based on methodologies and/or theories. It does not explicitly present how the evaluation carried out validates that the research offers direct contributions to the educational development of the user or those who relate to him. (1,0pts) The paper describes evaluation steps; however, they do not explicitly justify the experiment approach. It also does not explicitly present how the evaluation carried out validates that the research offers direct contributions to the educational development of the user or those who relate to him. (0,75pts) The paper generally presents the evaluation and does not explicitly justify ethe xperiment approach. It also does not explicitly present how the evaluation carried out validates that the research offers direct contributions to the educational development of the user or those who relate to him. (0,35pts) The paper does not present the recommender evaluation (0) |
Papers total weight range from 0 to 10. Only works that reached the minimum weight of 7 were accepted.
Screening process
Papers screening process occurred as shown in Fig. 2 . Initially, three authors carried out the identification of the studies. In this activity, the search string was applied into search engines of the repositories along with the inclusion and exclusion criteria through filtering settings. Two searches were undertaken on the three repositories at distinct moments, one in November 2020 and another in January 2021. The second one was performed to ensure that all 2020 published papers in the repositories were counted. A number of 756 preliminary primary studies were returned and their metadata were registered in Parsifal.

Flow of papers search and selection
Following the protocol, the selection activity was initiated. At the start, the duplicity verification feature of Parsifal was used. A total of 5 duplicate papers were returned and the oldest copies were ignored. Afterwards, papers were divided into groups and distributed among the authors. Inclusion and exclusion criteria were applied through titles and abstracts reading. In cases which were not possible to determine the eligibility of the papers based on these two fields, the body of text was read until it was possible to apply all criteria accurately. Finally, 41 studies remained for the next step. Once more, papers were divided into three groups and each set of works was evaluated by one author. Studies were read in full and weighted according to each quality assessment question. At any stage of this process, when questions arose, the authors defined a solution through consensus. As a final result of the selection activity, 16 papers were approved for data extraction.
Procedure for data analysis
Data from selected papers were extracted in a data collection form that registered general information and specific information. The general information extracted was: reviewer identification, date of data extraction and title, authors and origin of the paper. General information was used to manage the data extraction activity. The specific information was: recommendation approach, recommendation techniques, input parameters, data collection strategy, method for data collection, evaluation methodology, evaluation settings, evaluation approaches, evaluation metrics. This information was used to answer the research questions. Tabulated records were interpreted and a descriptive summary with the findings was prepared.
Results and discussion
In this section, the SLR results are presented. Firstly, an overview of the selected papers is introduced. Next, the finds are analyzed from the perspective of each research question in a respective subsection.
Selected papers overview
Each selected paper presents a distinct recommendation approach that advances the ERS field. Following, an overview of these studies is provided.
Sergis and Sampson ( 2016 ) present a recommendation system that supports educators’ teaching practices through the selection of learning objects from educational repositories. It generates recommendations based on the level of instructors’ proficiency on ICT Competences. In Tarus et al. ( 2017 ), the recommendations are targeted at students. The study proposes an e-learning resource recommender based on both user and item information mapped through ontologies.
Nafea et al. ( 2019 ) propose three recommendation approaches. They combine item ratings with student’s learning styles for learning objects recommendation. Klašnja-Milićević et al. ( 2018 ) present a recommender of learning materials based on tags defined by the learners. The recommender is incorporated in Protus e-learning system.
In Wan and Niu ( 2016 ), a recommender based on mixed concept mapping and immunological algorithms is proposed. It produces sequences of learning objects for students. In a different approach, the same authors incorporate the self-organization theory into ERS. Wan and Niu ( 2018 ) deals with the notion of self-organizing learning objects. In this research, resources behave as individuals who can move towards learners. This movement results in recommendations and is triggered based on students’ learning attributes and actions. Wan and Niu ( 2020 ), in turn, self-organization refers to the approach of students motivated by their learning needs. The authors propose an ERS that recommends self-organized cliques of learners and, based on these, recommend learning objects.
Zapata et al. ( 2015 ) developed a learning object recommendation strategy for teachers. The study describes a methodology based on collaborative methodology and voting aggregation strategies for the group recommendations. This approach is implemented in the Delphos recommender system. In a similar research line, Rahman and Abdullah ( 2018 ) show an ERS that recommends Google results tailored to students’ academic profile. The proposed system classifies learners into groups and, according to the similarity of their members, indicates web pages related to shared interests.
Wu et al. ( 2015 ) propose a recommendation system for e-learning environments. In this study, complexity and uncertainties related to user profile data and learning activities is modeled through tree structures combined with fuzzy logic. Recommendations are produced from matches of these structures. Ismail et al. ( 2019 ) developed a recommender to support informal learning. It suggests Wikipedia content taking into account unstructured textual platform data and user behavior.
Huang et al. ( 2019 ) present a system for recommending optional courses. The system indications rely on the student’s curriculum time constraints and similarity of academic performance between him and senior students. The time that individuals dedicate for learning is also a relevant factor in Nabizadeh et al. ( 2020 ). In this research, a learning path recommender that includes lessons and learning objects is proposed. Such a system estimates the learner’s good performance score and, based on that, produces a learning path that satisfies their time constraints. The recommendation approach also provides indication of auxiliary resources for those who do not reach the estimated performance.
Fernandez-Garcia et al. ( 2020 ) deals with recommendations of disciplines through a dataset with few instances and sparse. The authors developed a model based on several techniques of data mining and machine learning to support students’ decision in choosing subjects. Wu et al. ( 2020 ) create a recommender that captures students’ mastery of a topic and produces a list of exercises with a level of difficulty adapted to them. Yanes et al. ( 2020 ) developed a recommendation system, based on different machine learning algorithms, that provides appropriate actions to assist teachers to improve the quality of teaching strategies.
How teaching and learning support recommender systems produce recommendations?
The process of generating recommendations is analyzed based on two axes. Underlying techniques of recommender systems are discussed first then input parameters are covered. Studies details are provided in Table Table5 5 .
Summary of ERS techniques and input parameters used in the selected papers
Research (citation) | Recommendation approach | Main techniques | Main input parameters | Type of data collection strategy | Method for data collection |
---|---|---|---|---|---|
Sergis and Sampson ( ) | Hybrid (collaborative filtering and fuzzy logic) | Neighbors users based on Euclidean distance and fuzzy sets | (i) ICT Competency; (ii) Rating (users' preferences) | Hybrid | (i) Collection of users’ usage data; (ii) User defined |
Tarus et al. ( ) | Hybrid (Collaborative Filtering, sequential pattern mining and knowledge representation) | Neighbors users based on cosine similarities, Generalized Sequential Pattern algorithm and student/learning resource domain ontologies | (i) Learning style; (ii) Learning level; (iii) Item attributes (iv) Rating (users' preferences) | Explicit | (i) Questionnaire; (ii) Online test; (iii) N/A; (iv) User defined |
Nafea et al. ( ) | Collaborative filtering, content-based filtering and Hybrid (combining the last two approaches) | Neighbors users based on Pearson correlation, Neighbors items based on Pearson correlation and cosine similarity | (i) Learning style; (ii) Item attributes; (iii) Rating (users' preferences) | Explicit | (i) Questionnaire; (ii) Specialist defined |
Wan and Niu ( ) | Self-organization based | Self-organization theory | (i) Learning style; (ii) Item attributes; (iii) learning objectives (iv) learners’ behaviors | Hybrid | (i) Questionnaire; (ii) Specialist defined / students’ feedback; (iii) N/A; (iv) Collection of users’ usage data |
Rahman and Abdullah ( ) | Group based | Groupzation algorithm | (i) Academic information; (ii) learners’ behaviors (iii) Contextual information | Implicit | (i) Learning management system records; (ii) Collection of users’ usage data; (iii) Tracking changes in user academic records and behavior |
Zapata et al. ( ) | Hybrid (techniques for group-based recommendation) | Collaborative methodology, voting aggregation strategies and meta- learning techniques | Rating (users' preferences) | Explicit | User defined |
Wan and Niu ( ) | Hybrid (knowledge representation and heuristic methods) | Mixed concept mapping and immune algorithm | (i) Learning styles; (ii) item attributes | Explicit | (i) Questionnaire; (ii) Specialist de- fined / Students’ feedback |
Klašnja- Miliéevié et al. ( ) | Hybrid (Social tagging and sequential pattern mining) | Most popular tags algorithms and weighted hybrid strategy | (i) Tags; (ii) Learners’ behaviors | Hybrid | (i) User defined; (ii) Collection of users’ usage data |
Wu et al. ( ) | Hybrid (Knowledge representation, collaborative filtering and fuzzy logic) | fuzzy tree matching method, neighbors users based on cosine similarity and fuzzy set strategy | (i) Learning activities (ii) Learning objectives; (iii) Academic information; (iv) Rating (users' preferences) | Hybrid | (i) Collection of users’ usage data (ii, iii, iv) User defined |
Ismail et al. ( ) | Hybrid (Graph based and fuzzy logic) | Structural topical graph analysis algorithms and fuzzy set | (i) Learning interests; (ii) Thesaurus | Implicit | (i) Collection of users’ usage data; (ii) Data extraction from another system |
Huang et al. ( ) | Cross-user-domain collaborative filtering | Neighbors users based on cosine similarity | Academic information | Explicit | Input file/Dataset |
Yanes et al. ( ) | Hybrid (machine learning algorithms) | One-vs-All, Binary Relevance, Classifier Chain, Label Powerset, Multil Label, K Nearest-Neighbors | Academic information | Explicit | Input file/Dataset |
Wan and Niu ( ) | Hybrid (fuzzy logic, self-organization and sequential pattern mining) | Intuitionist fuzzy logic, self-organization theory and PrefixSpan algorithm | (i) Learning style (ii) Learning objectives (iii) Tags (iv) Academic information (v) Information from academic social relations | Hybrid | (i, ii) Questionnaire (iii) Extracted from m learners’ learning profiles (iv, v) Extracted from e-learning platform records |
Fernandez- Garcia et al. ( ) | Hybrid (data mining and machine learning algorithms) | Encoding, Feature Engineering, Scaling, Resampling, Random Forest, Logistic Regression, Decision Tree, Support Vector Machine, K Nearest Neighbors, Multilayer Perceptron and Gradient Boosting Classifier | Academic information | Explicit | Input file/Dataset |
Wu et al. ( ) | Hybrid (neural network techniques) | Recurrent Neural Networks and Deep Knowledge Tracing | Answers records | Explicit | Input file/Dataset |
Nabizadeh et al. ( ) | Hybrid (graph based, clustering technique and matrix factorization) | Depth first search, k-means and matrix factorization | (i) Background knowledge; (ii) users’ available time; (iii) Learning score | Implicit | (i) Collection of users’ usage data; (ii, iii) estimated data |
Techniques approaches
Through selected papers analysis is observed that hybrid recommendation systems are predominant in selected papers. Such recommenders are characterized by computing predictions through a set of two or more algorithms in order to mitigate or avoid the limitations of pure recommendation systems (Isinkaye et al., 2015 ). From sixteen analyzed papers, thirteen (p = 81,25%) are based on hybridization. This tendency seems to be related with the support that hybrid approach provides for development of recommender systems that must meet multiple educational needs of users. For example, Sergis and Sampson ( 2016 ) proposed a recommender based on two main techniques: fuzzy set to deal with uncertainty about teacher competence level and Collaborative Filtering (CF) to select learning objects based on neighbors who may have competences similarities. In Tarus et al. ( 2017 ) students and learning resources profiles are represented as ontologies. The system calculates predictions based on them and recommends learning items through a mechanism that applies collaborative filtering followed by a sequential pattern mining algorithm.
Moreover, the hybrid approach that combines CF and Content-Based Filtering (CBF), although a traditional technique (Bobadilla, Ortega, Hernando and Gutiérrez, 2013), it seems to be not popular in teaching and learning support recommender systems research. From the selected papers, only Nafea et al. ( 2019 ) has a proposal in this regard. Additionally, the extracted data indicates that a significant number of hybrid recommendation systems (p = 53.85%, n = 7) have been built based on the combination of methods of treatment or representation of data, such as the use of ontologies and fuzzy sets, with methods to generate recommendation. For example, Wu et al. ( 2015 ) structure users profile data and learning activities through fuzzy trees. In such structures the values assigned to their nodes are represented by fuzzy sets. The fuzzy tree data model and users’ ratings feed a tree structured data matching method and a CF algorithm for similarities calculation.
Collaborative filtering recommendation paradigm, in turn, plays an important role in research. Nearly a third of the studies (p = 30.77%, n = 4) that propose hybrid recommenders includes a CF-based strategy. In fact, this is the most frequent pure technique on the research set. A total of 31.25%( n = 5) are based on a CF adapted version or combine it with other approaches. CBF-based recommenders, in contrast, have not shared the same popularity. This technique is an established recommendation approach that produces results based on the similarity between items known to the user and others recommendable items (Bobadilla et al., 2013 ). Only Nafea et al. ( 2019 ) propose a CBF-based recommendation system.
Also, CF user-based variant is widely used in analyzed research. In this version, predictions are calculated by similarity between users, as opposed to the item-based version where predictions are based on item similarities (Isinkaye et al., 2015 ). All CF-based recommendation systems identified, whether pure or combined with other techniques, use this variant.
The above finds seem to be related to the growing perception, in the education domain, of the relevance of a student-centered teaching and learning process (Krahenbuhl, 2016 ; Mccombs, 2013 ). Recommendation approaches that are based on users’ profile, such as interests, needs, and capabilities, naturally fit this notion and are more widely used than those based on other information such as the characteristics of the recommended items.
Input parameters approaches
In regard to the inputs consumed in the recommendation process, collected data shows that the main parameters are attributes related to users’ educational profile. Examples are ICT competences (Sergis & Sampson, 2016 ); learning objectives (Wan & Niu, 2018 ; Wu et al., 2015 ), learning styles (Nafea et al., 2019 ), learning levels (Tarus et al., 2017 ) and different academic data (Yanes et al., 2020 ; Fernández-García et al., 2020). Only 25% ( n = 4) of the systems apply item-related information in the recommendation process. Furthermore, with the exception of the Nafea et al. ( 2019 ) CBF-based recommendation, the others are based on a combination of items and users’ information. A complete list of the identified input parameters is provided in Table Table5 5 .
Academic information and learning styles, compared to others parameters, features highly on research. They appear, respectively, in 37.5% ( n = 6) and 31.25% ( n = 5) papers. Student’s scores (Huang et al., 2019 ), academic background (Yanes et al., 2020 ), learning categories (Wu et al., 2015 ) and subjects taken (Fernández-García et al.,2020) are some of the academic data used. Learning styles, in turn, are predominantly based on Felder ( 1988 ) theory. Wan and Niu ( 2016 ), exceptionally, combine Felder ( 1988 ), Kolb et al. ( 2001 ) and Betoret ( 2007 ) to build a specific notion of learning styles. This is also used in two other researchers, carried out by the same authors, and has a questionnaire also developed by them (Wan & Niu, 2018 , 2020 ).
Regarding the way inputs are captured, it was observed that explicit feedback is prioritized over others data collection strategies. In this approach, users have to directly provide the information that will be used in the process of preparing recommendations (Isinkaye et al., 2015 ). Half of analyzed studies are based only on explicit feedback. The use of graphical interface components (Klašnja-Milićević et al., 2018 ), questionnaires (Wan & Niu, 2016 ) and manual entry of datasets (Wu et al., 2020 ; Yanes et al., 2020 ) are the main methods identified.
Only 18.75%( n = 3) ERS rely solely on gathering information through implicit feedback, that is, when inputs are inferred by the system (Isinkaye et al., 2015 ). This type of data collection appears to be more popular when applied with an explicit feedback method for enhancing the prediction tasks. Recommenders that combine both approaches occur in 31.25%( n = 5) of the studies. Implicit data collection methods identified are user’s data usage tracking, as access, browsing and rating history (Rahman & Abdullah, 2018 ; Sergis & Sampson, 2016 ; Wan & Niu, 2018 ), data extraction from another system (Ismail et al., 2019 ), users data session monitoring (Rahman & Abdullah, 2018 ) and data estimation (Nabizadeh et al., 2020 ).
The aforementioned results indicate that, in the context of the teaching and learning support recommender systems, the implicit collection of data has usually been explored in a complementary way to the explicit one. A possible rationale is that the inference of information is noisy and less accurate (Isinkaye et al., 2015 ) and, therefore, the recommendations produced from it involve greater complexity to be adjusted to the users’ expectations (Nichols, 1998 ). This aspect makes it difficult to apply the strategy in isolation and can be a factor that produces greater user dissatisfaction when compared to the disadvantage of the acquisition load of the explicit strategy inputs.
How teaching and learning support recommender systems present recommendations?
From the analyzed paper, two approaches for presenting recommendations are identified. The majority of the proposed ERS are based on a listing of ranked items according to a per-user prediction calculation (p = 87.5%, n = 14). This strategy is applied in all cases where the supported task is to find good items that assist users in teaching and learning tasks (Ricci et al., 2015 ; Drachsler et al., 2015 ). The second one, is based on a learning pathway generation. In this case, recommendations are displayed through a series of linked items tied by some prerequisites. Only 2 recommenders use this approach. In them, the sequence is established by learning objects association attributes (Wan & Niu, 2016 ) and by a combination of prior knowledge of the user, the time he has available and a learning score (Nabizadeh et al., 2020 ). These ERS are associated with the item sequence recommendation task and are intended to guide users who wish to achieve a specific knowledge (Drachsler et al., 2015 ).
In a further examination, it is observed that more than a half (62.5%, n = 10) do not present details of how recommendations list is presented to the end user. In Huang et al. ( 2019 ), for example, there is a vague description of a production of predicted scores for students and a list of the top-n optional courses and it is not specified how this list is displayed. This may be related to the fact that most of these recommenders do not report an integration into another system (e.g., learning management systems) or the purpose of making it available as a standalone tool (e.g., web or mobile recommendation system). The absence of such requirements mitigates the need for the development of a refined presentation interface. Only Tarus et al. ( 2017 ), Wan and Niu ( 2018 ) and Nafea et al. ( 2019 ) propose recommenders incorporated in an e-learning system and do not detail the way in which the results are exhibited. In the six papers that provide insights about recommendation presentation, a few of them (33.33%, n = 2), have a graphical interface that explicitly seeks to capture the attention of the user who may be performing another task in the system. This approach highlights recommendations and is common in commercial systems (Beel, Langer and Genzmehr, 2013). In Rahman and Abdullah ( 2018 ), a panel entitled “recommendations for you” is used. In Ismail et al. ( 2019 ), a pop-up box with suggestions is displayed to the user. The other part of the studies exhibits organic recommendations, i.e., naturally arranged items for user interaction (Beel et al., 2013 ).
In Zapata et al. ( 2015 ), after the user defines some parameters, a list of recommended learning objects that are returned similarly to a search engine result. As for the aggregation methods, another item recommended by the system, only the strategy that fits better to the interests of the group is recommended. The result is visualized through a five-star Likert scale that represents the users’ consensus rating. In Klašnja-Milićević et al. ( 2018 ) and Wu et al. ( 2015 ), the recommenders’ results are listed in the main area of the system. In Nabizadeh et al. ( 2020 ) the learning path occupies a panel on the screen and the items associated with it are displayed as the user progresses through the steps. The view of the auxiliary learning objects is not described in the paper. These three last recommenders do not include filtering settings and distance themselves from the archetype of a search engine.
Also, a significant number of researches are centralized on learning objects recommendations (p = 56.25%, n = 9). Other researches recommendable items identified are learning activities (Wu et al., 2015 ), pedagogical actions (Yanes et al., 2020 ), web pages (Ismail et al., 2019 ; Rahman & Abdullah, 2018 ), exercises (Wu et al., 2020 ), aggregation methods (Zapata et al., 2015 ), lessons (Nabizadeh et al., 2020 ) and subjects (Fernández-García et al., 2020). None of the study relates the way of displaying results to the recommended item. This is a topic that needs further investigation to answer whether there are more appropriate ways to present specific types of items to the user.
How teaching and learning support recommender systems are evaluated?
In ERS, there are three main evaluation methodologies (Manouselis et al., 2013 ). One of them is the offline experiment, which is based on the use of pre-collected or simulated data to test recommenders’ prediction quality (Shani & Gunawardana, 2010 ). User study is the second approach. It takes place in a controlled environment where information related to real interactions of users are collected (Shani & Gunawardana, 2010 ). This type of evaluation can be conducted, for example, through a questionnaire and A/B tests (Shani & Gunawardana, 2010 ). Finally, the online experiment, also called real life testing, is one in which recommenders are used under real conditions by the intended users (Shani & Gunawardana, 2010 ).
In view of these definitions, the analyzed researches comprise only user studies and offline experiments in reported experiments. Each of these methods were identified in 68.75% ( n = 11) papers respectively. Note that they are not exclusive for all cases and therefore the sum of the percentages is greater than 100%. For example, Klašnja-Milićević et al. ( 2018 ) and Nafea et al. ( 2019 ) assessed the quality of ERS predictions from datasets analysis and also asked users to use the systems to investigate their attractiveness. Both evaluation methods are carried out jointly in 37.5%( n = 6) papers. When comparing with methods exclusive usage, each one is conducted at 31.25% ( n = 5). Therefore, the two methods seem to have a balanced popularity. Real-life tests, on the contrary, although they are the ones that best demonstrate the quality of a recommender (Shani & Gunawardana, 2010 ), are the most avoided, probably due to the high cost and complexity of execution.
An interesting finding concerns user study methods used in research. When associated with offline experiments, the user satisfaction assessment is the most common ( p = 80%, n = 5). Of these, only Nabizadeh et al. ( 2020 ) performed an in-depth evaluation combining a satisfaction questionnaire with an experiment to verify the pedagogical effectiveness of their recommender. Wu et al. ( 2015 ), in particular, does not include a satisfaction survey. They conducted a qualitative investigation of user interactions and experiences.
Although questionnaires assist in identification of users’ valuables information, it is sensitive to respondents’ intentions and can be biased with erroneous answers (Shani & Gunawardana, 2010 ). Papers that present only user studies, in contrast, have a higher rate of experiments that results in direct evidence about the recommender’s effectiveness in teaching and learning. All papers in this group have some investigation in this sense. Wan and Niu ( 2018 ), for example, verified whether the recommender influenced the academic score of students and their time to reach a learning objective. Rahman and Abdullah ( 2018 ) investigated whether the recommender impacted the time students took to complete a task.
Regarding the purpose of the evaluations, ten distinct research goals were identified. Through Fig. 3 , it is observed that the occurrence of accuracy investigation excelled the others. Only 1 study did not carry out experiments in this regard. Different traditional metrics were identified for measuring the accuracy of recommenders. The Mean Absolute Error (MAE), in particular, has the higher frequency. Table Table6 6 lists the main metrics identified.

Evaluation purpose of recommender systems in selected papers
Summary of ERS evaluation settings, approaches and metrics in selected papers
Research (citation) | Evaluation Methodology | Dataset Size / No. of Subjects | No. Recommendable Items | Highlights of Evaluation Approaches | Highlights of Evaluation Metrics |
---|---|---|---|---|---|
Sergis and Sampson ( ) | Offline | 2005 | 96.196 | Layered evaluation, Dataset split (70% training, 30% test) and comparison with collaborative filtering variations | Jaccard coefficient (for user’s ICT profile elicitation accuracy) and RMSE |
Tarus et al. ( ) | Offline and user study | 50 | 240 | Dataset split (70% training, 30% test), comparison with collaborative filtering variations and questionnaire survey | MAE, Precision, Recall and user’s satisfaction level |
Nafea et al. ( ) | Offline and user study | 80 | At least 300 | Comparison between the proposed algorithms and questionnaire survey | MAE, RMSE and user’s satisfaction level |
Wan and Niu ( ) | User study | 749 | 3043 | A/B test, comparison with Instructors’ suggestions and e-learning recommender systems based on genetic algorithm and Markov chain, and questionnaire survey | Average students’ score, learning time, learning objects utilization, fitness function, learning objects’ hit rate, learning objects’ proportions marked with educational meaning tags, non centralized distribution of learning objects, proportion of new recommended items, user’s satisfaction level and entropy (time to achieve a stable sequence of recommendations) |
Rahman and Abdullah ( ) | User study | 60 | N/A | A/B test and questionnaire survey | Search time for educational materials, quantity of access to recommended items, user’s satisfaction level, level of ease of use and the usefulness of recommender |
Zapata et al. ( ) | Offline and user study | 75 for offline experiment and 63 for questionnaire | N/A | Comparison between rating aggregation methods, analysis of appropriate aggregation method selection and questionnaire survey | RMSE, Top 1 Frequency, Average Ranking, Average Recommenda- tion Error, Mean Reciprocal Rank, user’s satisfaction level and level of ease of use and the usefulness of recommender |
Wan and Niu ( ) | User study | 250 | 235 | A/B test, comparison with Instructors’ suggestions and e-learning recommender systems based on genetic algorithm, particle swarm optimization and ant colony optimization algorithm, and questionnaire survey | Time spent on learning planning, quantity of recommended learning objects, average score, quantity of students who successfully passed the final exam, average recommendation rounds, Average total recommended learning objects for each learner among all recommendation rounds, average time of learning objects recommendation, average evolution time and user’s satisfaction level |
Klašnja- Miliéevié et al. ( ) | Offline and user study | 120 for offline experiment and 65 for questionnaire | 62 | Dataset split (80% training, 20% test), Comparison between tag recommendation methods and questionnaire survey | Precision, Recall, user’s satisfaction level and level of ease of use and the usefulness |
Wu et al. ( ) | Offline and user study | 2213 for offline experiment and 5 for case study | N/A | Dataset split (20%/40%/50% test set), Compared with recommendation approach for e-learning recommender systems proposed by Bobadilla et al. ( ) and case study | MAE |
Ismail et al. ( ) | User study | 100 for comparison and 80 for questionnaire | N/A | A/B test, comparison between the proposed recom- menders with a control group and a baseline approach and questionnaire survey | Mean Average Precision, knowledge level, visited articles, perceived relevance and user’s satisfaction level |
Huang et al. ( ) | Offline | 1166 | 782 | Dataset split (Training and testing dataset divided according to semesters) and comparison of recommender predicted optional courses and the ground- truth optional courses that student has enrolled on | Average hit rate, average accuracy |
Yanes et al. ( ) | Offline | N/A | 9 | Dataset split (70% training, 30% test) and comparison of different machine learning algorithms | Precision, Recall, F1-measure, Hamming Loss |
Wan and Niu ( ) | User study | 1119 | 2386 | A/B test, comparison with Instructors’ suggestions and with variants of the proposed algorithm, and questionnaire survey | Students’ average scores, proportion of students who passed the exam, average learning time, proportion of the resources that learners had visited out of the total number of resources, matching degree between learning objects and learners, diversity of learning objects attributes, proportion of learner’s tags, user’s satisfaction level, level of usefulness and entropy (time to achieve a stable sequence of recommendations) |
Fernandez-Garcia et al. ( | Offline | 323 | 45 | Sequential chain of steps with dataset transformations | Accuracy, F1-score |
Wu et al. ( ) | Offline | 5126 | 19.136 | Dataset split (70% Training, 10% validation, 20% test) and comparison with user-based and item-based collaborative filter, content-based filtering, hybrid recommendation model based on deep collaborative filtering and a knowledge-graph embedding based collaborative filtering | Accuracy, novelty and diversity |
Nabizadeh et al. ( ) | Offline and user study | 205 for offline experiment and 32 for user study | 90 for offline experiment and 59 for user study | Dataset split (Training and testing dataset divided according to a defined observed and unobserved LO classification), algorithms comparison, A/B test, control and experimental groups | AE, number of correctly completed learning objects/lessons by users, time that users spend to get their goals and user’s satisfaction level |
The system attractiveness analysis, through the verification of user satisfaction, has the second highest occurrence. It is present in 62.5% ( n = 10) studies. The pedagogical effectiveness evaluation of the ERS has a reduced participation in the studies and occurs in only 37.5% ( n = 6). Experiments to examine recommendations diversity, user’s profile elicitation accuracy, evolution process, user’s experience and interactions, entropy, novelty and perceived usefulness and easiness were also identified, albeit to a lesser extent.
Also, 81.25% ( n = 13) papers presented experiments to achieve multiple purposes. For example, in Wan and Niu ( 2020 ) an evaluation is carried out to investigate recommenders’ pedagogical effectiveness, student satisfaction, accuracy, diversity of recommendations and entropy. Only in Huang et al. ( 2019 ), Fernandez-Garcia et al. ( 2020 ) and Yanes et al. ( 2020 ) evaluated a single recommender system dimension.
The upper evidence suggests an engagement of the scientific community in demonstrating the quality of the recommender systems developed through multidimensional analysis. However, offline experiments and user studies, particularly those based on questionnaires, are mostly adopted and can lead to incomplete or biased interpretations. Thus, such data also signalize the need for a greater effort to conduct real life tests and experiments that lead to an understanding of the real impact of recommenders on the teaching and learning process. Researches that synthesize and discuss the empirical possibilities of evaluating the pedagogical effectiveness of ERS can help to increase the popularity of these experiments.
Through papers analysis is also find that the results of offline experiments are usually based on a greater amount of data compared to user studies. In this group, 63.64% ( n = 7) of evaluation datasets have records of more than 100 users. User studies, on the other hand, predominate sets of up to 100 participants in the experiments (72.72%, n = 8). In general, offline assessments that have smaller datasets are those that occur in association with a user study. This is because the data for both experiments usually come from the same subjects (Nafea et al., 2019 ; Tarus et al., 2017 ). The cost (e.g., time and money) related to surveying participants for the experiment is possibly a determining factor in defining appropriate samples.
Furthermore, it is also verified that the greater parcel of offline experiments has a 70/30% division approach for training and testing data. Nguyen et al. ( 2021 ) give some insights in this sense arguing that this is the most suitable ratio for training and validating machine learning models. Further details on recommendation systems evaluation approaches and metrics are presented in Table Table6 6 .
What are the limitations and research opportunities related to the teaching and learning support recommender systems field?
The main limitations observed in selected papers are presented below. They are based on articles’ explicit statements and on authors’ formulations. In this section, only those that are transverse to the majority of the studies are listed. Next, a set of research opportunities for future investigations are pointed out.
Research limitations
Research limitations are factors that hinders current progress in the ERS field. Knowing these factors can assist researchers to attempt coping with them on their study and mitigate the possibility of the area stagnation, that is, when new proposed recommenders does not truly generate better outcomes than the baselines (Anelli et al., 2021 ; Dacrema et al., 2021 ). As a result of this SLR, research limitations were identified in three strands that are presented below.
Reproducibility restriction
The majority of the papers report a specifically collected dataset to evaluate the proposed ERS. The main reason for this is the scarcity of public datasets suited to the research’s needs, as highlighted by some authors (Nabizadeh et al., 2020 ; Tarus et al., 2017 ; Wan & Niu, 2018 ; Wu et al., 2015 ; Yanes et al., 2020 ). Such approach restricts the feasibility of experiment reproduction and makes it difficult to compare recommenders. In fact, this is an old issue in the ERS field. Verbert et al. ( 2011 ) observed, in the beginning of the last decade, the necessity to improve reproducibility and comparison on ERS in order to provide stronger conclusions about their validity and generalizability. Although there was an effort in this direction in the following years based on a broad educational dataset sharing, currently, most of the known ones (Çano & Morisio, 2015 ; Drachsler et al., 2015 ) are retired, and the remaining, proved not to be sufficient to meet current research demands. Of the analyzed studies, only Wu et al. ( 2020 ) use public educational datasets.
Due to the fact that datasets sharing play an important role for recommenders’ model reproduction and comparison in the same conditions, this finding highlight the need of a research community effort for the creation of means to supply this need (e.g., development of public repositories) in order to mitigate current reproducibility limitation.
Dataset size / No of subjects
As can be observed on Table Table6, 6 , a few experimental results are based on a large amount of data. Only five studies have information from 1000 or more users. In particular, the offline evaluation conducted by Wu et al. ( 2015 ), despite having an extensive dataset, uses MovieLens records and is not based on real information related to teaching and learning. Another limitation concerns where data comes from, it is usually from a single origin (e.g., class of a college).
Although experiments based on small datasets can reveal the relevance of an ERS, an evaluation based on a large-scale dataset should provide stronger conclusions on recommendation effectiveness (Verbert et al., 2011 ). Experiments based on larger and more diverse data (e.g., users from different areas and domains) would contribute to most generalizable results. On another hand, scarcity of public dataset may be impairing the quantity and diversity of data used on scientific experiments in the ERS field. As reported by Nabizadeh et al. ( 2020 ), the increasement of the size of the experiment is costly in different aspects. If more public dataset were available, researchers would be more likely to find the ones that could be aligned to their needs and, naturally, increasing the size of their experiment. In this sense, they could be favored by reducing data acquisition difficulty and cost. Furthermore, the scientific community would access users’ data out of their surrounding context and could base their experiments on diversified data.
Lack of in-depth investigation of the impact of known issues in the recommendation system field
Cold start, overspecialization and sparsity are some known challenges in the field of recommender systems (Khusro et al., 2016 ). They are mainly related to a reduced and unequally distributed number of users’ feedback or item description used for generating recommendations (Kunaver & Požrl, 2017 ). These issues also permeate the ERS Field. For instance, in Cechinel et al. ( 2011 ) is reported that on a sample of more than 6000 learning objects from Merlot repository was observed a reduced number of users ratings over items. Cechinel et al. ( 2013 ), in turn, observed, in a dataset from the same repository, a pattern of few users rating several resources while the vast number of them rating 5 or less. Since such issues directly impact the quality of recommendations, teaching and learning support recommenders should be evaluated considering such issues to clarify in which extent they can be effective in real life situations. Conversely, in this SLR, we detected an expressive number of papers (43.75%, n = 7) that do not analyze or discuss how the recommenders behave or handle, at least partially, these issues. Studies that rely on experiments to examine such aspects would elucidate more details of the quality of the proposed systems.
Research opportunities
From the analyzed papers, a set of research opportunities were identified. They are based on gaps related to the subjects explored through the research questions of this SLR. The identified opportunities provide insights of under-explored topics that need further investigation taking into account their potential to contribute to the advancement of the ERS field. Research opportunities were identified in three strands that are presented below.
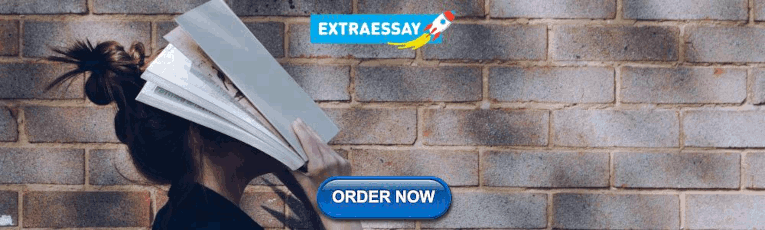
Study of the potential of overlooked user’s attributes
The papers examined present ERS based on a variety of inputs. Preferences, prior knowledge, learning style, and learning objectives are some examples (Table (Table5 5 has the complete list). Actually, as reported by Chen and Wang ( 2021 ), this is aligned with a current research trend of investigating the relationships between individual differences and personalized learning. Nevertheless, one evidence that rises from this SLR also confirms that “some essential individual differences are neglected in existing works” (Chen & Wang, 2021 ). The papers sample suggests a lack of studies that incorporate, in recommendation model, others notably relevant information, such as emotional state and cultural context of students (Maravanyika & Dlodlo, 2018 ; Salazar et al., 2021 ; Yanes et al., 2020 ). This indicates that further investigation is needed in order to clarify the true contributions and existing complexities of collect, measure and apply these other parameters. In this sense, an open research opportunity refers to the investigation of these other users’ attributes in order to explore the impact of such characteristics on the quality of ERS results.
Increase studies on the application of ERS in informal learning situations
Informal learning refers to a type of learning that, typically, occurs out of an education institution (Pöntinen et al., 2017 ). In it, learners do not follow a structured curriculum or have a domain expert to guide him (Pöntinen et al., 2017 ; Santos & Ali, 2012 ). Such aspects influence how ERS can support users. For instance, in informal settings, content can come from multiple providers, as a consequence, it can be delivered without taking into account a proper pedagogical sequence. ERS targeting this scenario, in turn, should concentrate on organizing and sequencing recommendations guiding users’ learning process (Drachsler et al., 2009 ).
Although literature highlight the existence of significative differences on the design of educational recommenders that involves formal or informal learning circumstance (Drachsler et al., 2009 ;Okoye et al, 2012 ; Manouselis et al., 2013 ; Harrathi & Braham, 2021 ), through this SLR was observed that current studies tend to not be explicit in reporting this characteristic. This scenario makes it difficult to obtain a clear landscape of the current field situation in this dimension. Nonetheless, through the characteristics of the proposed ERS, it was observed that current research seems to be concentrated on the formal learning context. This is because recommenders from analyzed papers usually use data that are maintained by institutional learning systems. Moreover, recommendations, predominantly, do not provide a pedagogical sequencing to support self-directed and self-paced learning (e.g., recommendations that build a learning path to lead to specific knowledge). Conversely, informal learning has increasingly gained attention of the scientific community with the emergence of the coronavirus pandemic (Watkins & Marsick, 2020 ).
In view of this, the lack of studies of ERS targeting informal learning settings open a research opportunity. Specifically, further investigation focused on the design and evaluation of recommenders that take into consideration different contexts (ex. location or used device) and that guide users through a learning sequence to achieve a specific knowledge would figure prominently in this context considering the less structured format informal learning circumstances has in terms of learning objectives and learning support.
Studies on the development of multidimensional evaluation frameworks
Evidence from this study shows that the main purpose of ERS evaluation has been to assess recommender’s accuracy and users’ satisfaction (Section 4.4 ). This result, connected with Erdt et al. ( 2015 ) reveals a two decade of evaluation predominantly based on these two goals. Even though others evaluation purposes had a reduced participation in research, they are also critical for measuring the success of ERS. Moubayed et al. ( 2018 ), for example, highlights two e-learning systems evaluation aspects, one is concerned with how to properly evaluate the student performance, the other refers to measuring learners’ learning gains through systems usage. Tahereh et al. ( 2013 ) identifies that stakeholder and indicators associated with technological quality are relevant to consider in educational system assessment. From the perspective of recommender systems field, there are also important aspects to be analyzed in the context of its application in the educational domain such as novelty and diversity (Pu et al., 2011 ; Cremonesi et al., 2013 ; Erdt et al., 2015 ).
Upon this context, it is noted that, although evaluating recommender's accuracy and users’ satisfaction give insights about the value of the ERS, they are not sufficient to fully indicate the quality of the system in supporting the learning process. Other different factors reported in literature are relevant to take in consideration. However, to the best of our knowledge, there is no framework that identifies and organizes these factors to be considered in an ERS evaluation, leading to difficulties for the scientific community to be aware of them and incorporate them in studies.
Because the evaluation of ERS needs to be a joint effort between computer scientists and experts from other domains (Erdt et al., 2015 ), further investigation should be carried out seeking the development of a multidimensional evaluation framework that encompass evaluation requirements based on a multidisciplinary perspective. Such studies would clarify the different dimensions that have the potential to contribute to better ERS evaluation and could even identify which one should be prioritized to truly assess learning impact with reduced cost.
In recent years, there has been an extensive scientific effort to develop recommenders that meet different educational needs; however, research is dispersed in literature and there is no recent study that encompasses the current scientific efforts in the field.
Given this context, this paper presents an SLR that aims to analyze and synthesize the main trends, limitations and research opportunities related to the teaching and learning support recommender systems area. Specifically, this study contributes to the field providing a summary and an analysis of the current available information about the teaching and learning support recommender systems topic in four dimensions: (i) how the recommendations are produced (ii) how the recommendations are presented to the users (iii) how the recommender systems are evaluated and (iv) what are the limitations and opportunities for research in the area.
Evidences are based on primary studies published from 2015 to 2020 from three repositories. Through this review, it is provided an overarching perspective of current evidence-based practice in ERS in order to support practitioners and researchers for implementation and future research directions. Also, research limitations and opportunities are summarized in light of current studies.
The findings, in terms of current trends, shows that hybrid techniques are the most used in teaching and learning support recommender systems field. Furthermore, it is noted that approaches that naturally fit a user centered design (e.g., techniques that allow to represent students’ educational constraints) have been prioritized over that based on other aspects, like item characteristics (e.g., CBF Technique). Results show that these approaches have been recognized as the main means to support users with recommendations in their teaching and learning process and provide directions for practitioners and researchers who seek to base their activities and investigations on evidence from current studies. On the other hand, this study also reveals that highly featured techniques in the major topic of general recommender systems, such as the bandit-based and the deep learning ones (Barraza-Urbina & Glowacka, 2020 ; Zhang et al., 2020 ), have been underexplored, implying a mismatch between the areas. Therefore, the result of this systematic review indicates that a greater scientific effort should be employed to investigate the potential of these uncovered approaches.
With respect to recommendation presentation, the organic display is the most used strategy. However, most of the researches have the tendency to not show details of the used approach making it difficult to understand the state of the art of this dimension. Furthermore, among other results, it is observed that the majority of the ERS evaluation are based on the accuracy of recommenders and user's satisfaction analysis. Such a find open research opportunity scientific community for the development of multidimensional evaluation frameworks that effectively support the verification of the impact of recommendations on the teaching and learning process.
Lastly, the limitations identified indicate that difficulties related to obtaining data to carry out evaluations of ERS is a reality that extends for more than a decade (Verbert et al., 2011 ) and call for scientific community attention for the treatment of this situation. Likewise, the lack of in-depth investigation of the impact of known issues in the recommendation system field, another limitation identified, points to the importance of aspects that must be considered in the design and evaluation of these systems in order to provide a better elucidation of their potential application in a real scenario.
With regard to research limitations and opportunities, some of this study findings indicate the need for a greater effort in the conduction of evaluations that provide direct evidence of the systems pedagogical effectiveness and the development of a multidimensional evaluation frameworks for ERS is suggested as a research opportunity. Also, it was observed a scarcity of public dataset usage on current studies that leads to limitation in terms of reproducibility and comparison of recommenders. This seems to be related to a restricted number of public datasets currently available, and such aspect can also be affecting the size of experiments conducted by researchers.
In terms of limitations of this study, the first refers to the number of datasources used for paper selection. Only the repositories mentioned in Section 3.1 were considered. Thus, the scope of this work is restricted to evidence from publications indexed by these platforms. Furthermore, only publications written in English were examined, thus, results of papers written in other languages are beyond the scope of this work. Also, the research limitations and opportunities presented on Section 4.5 were identified based on the extracted data used to answer this SLR research questions, therefore they are limited to their scope. As a consequence, limitations and opportunities of the ERS field that surpass this context were not identified nor discussed in this study. Finally, the SLR was directed to papers published in scientific journals and, due to this, the results obtained do not reflect the state of the area from the perspective of conference publications. In future research, it is intended to address such limitations.
Electronic supplementary material
Below is the link to the electronic supplementary material.
Table Table7 7
Author contribution
Felipe Leite da Silva: Conceptualization, Methodology approach, Data curation, Writing – original draft. Bruna Kin Slodkowski: Data curation, Writing – original draft. Ketia Kellen Araújo da Silva: Data curation, Writing – original draft. Sílvio César Cazella: Supervision and Monitoring of the research; Writing – review & editing.
Data availability statement
Informed consent.
This research does not involve human participation as research subject, therefore research subject consent does not apply.
Authors consent with the content presented in the submitted manuscript.
Financial and non-financial interests
The authors have no relevant financial or non-financial interests to disclose.
Research involving human participants and/or animals
This research does not involve an experiment with human or animal participation.
Competing interests
The authors have no competing interests to declare that are relevant to the content of this article.
1 http://parsif.al/
Publisher's note
Springer Nature remains neutral with regard to jurisdictional claims in published maps and institutional affiliations.
- Anelli, V. W., Bellogín, A., Di Noia, T., & Pomo, C. (2021). Revisioning the comparison between neural collaborative filtering and matrix factorization. Proceedings of the Fifteenth ACM Conference on Recommender Systems , 521–529. 10.1145/3460231.3475944
- Ashraf E, Manickam S, Karuppayah S. A comprehensive review of curse recommender systems in e-learning. Journal of Educators Online. 2021; 18 :23–35. [ Google Scholar ]
- Barraza-Urbina, A., & Glowacka, D. (2020). Introduction to Bandits in Recommender Systems. Proceedings of the Fourteenth ACM Conference on Recommender Systems , 748–750. 10.1145/3383313.3411547
- Becker F. Teacher epistemology: The daily life of the school. 1. Editora Vozes; 1993. [ Google Scholar ]
- Beel J, Langer S, Genzmehr M. Sponsored vs. Organic (Research Paper) Recommendations and the Impact of Labeling. In: Aalberg T, Papatheodorou C, Dobreva M, Tsakonas G, Farrugia CJ, editors. Research and Advanced Technology for Digital Libraries. Springer Berlin Heidelberg; 2013. pp. 391–395. [ Google Scholar ]
- Betoret F. The influence of students’ and teachers’ thinking styles on student course satisfaction and on their learning process. Educational Psychology. 2007; 27 (2):219–234. doi: 10.1080/01443410601066701. [ CrossRef ] [ Google Scholar ]
- Bobadilla J, Serradilla F, Hernando A. Collaborative filtering adapted to recommender systems of e-learning. Knowledge-Based Systems. 2009; 22 (4):261–265. doi: 10.1016/j.knosys.2009.01.008. [ CrossRef ] [ Google Scholar ]
- Bobadilla J, Ortega F, Hernando A, Gutiérrez A. Recommender systems survey. Knowledge-Based Systems. 2013; 46 :109–132. doi: 10.1016/j.knosys.2013.03.012. [ CrossRef ] [ Google Scholar ]
- Buder J, Schwind C. Learning with personalized recommender systems: A psychological view. Computers in Human Behavior. 2012; 28 (1):207–216. doi: 10.1016/j.chb.2011.09.002. [ CrossRef ] [ Google Scholar ]
- Çano, E., & Morisio, M. (2015). Characterization of public datasets for Recommender Systems. (2015 IEEE 1 st ) International Forum on Research and Technologies for Society and Industry Leveraging a better tomorrow (RTSI) , 249–257.10.1109/RTSI.2015.7325106
- Cazella SC, Behar PA, Schneider D, Silva KKd, Freitas R. Developing a learning objects recommender system based on competences to education: Experience report. New Perspectives in Information Systems and Technologies. 2014; 2 :217–226. doi: 10.1007/978-3-319-05948-8_21. [ CrossRef ] [ Google Scholar ]
- Cechinel C, Sánchez-Alonso S, García-Barriocanal E. Statistical profiles of highly-rated learning objects. Computers & Education. 2011; 57 (1):1255–1269. doi: 10.1016/j.compedu.2011.01.012. [ CrossRef ] [ Google Scholar ]
- Cechinel C, Sicilia M-Á, Sánchez-Alonso S, García-Barriocanal E. Evaluating collaborative filtering recommendations inside large learning object repositories. Information Processing & Management. 2013; 49 (1):34–50. doi: 10.1016/j.ipm.2012.07.004. [ CrossRef ] [ Google Scholar ]
- Chen SY, Wang J-H. Individual differences and personalized learning: A review and appraisal. Universal Access in the Information Society. 2021; 20 (4):833–849. doi: 10.1007/s10209-020-00753-4. [ CrossRef ] [ Google Scholar ]
- Cremonesi P, Garzotto F, Turrin R. User-centric vs. system-centric evaluation of recommender systems. In: Kotzé P, Marsden G, Lindgaard G, Wesson J, Winckler M, editors. Human-Computer Interaction – INTERACT 2013, 334–351. Springer Berlin Heidelberg; 2013. [ Google Scholar ]
- Dacrema MF, Boglio S, Cremonesi P, Jannach D. A troubling analysis of reproducibility and progress in recommender systems research. ACM Transactions on Information Systems. 2021; 39 (2):1–49. doi: 10.1145/3434185. [ CrossRef ] [ Google Scholar ]
- Dermeval, D., Coelho, J.A.P.d.M., & Bittencourt, I.I. (2020). Mapeamento Sistemático e Revisão Sistemática da Literatura em Informática na Educação. Metodologia de Pesquisa Científica em Informática na Educação: Abordagem Quantitativa . Porto Alegre. https://jodi-ojs-tdl.tdl.org/jodi/article/view/442
- Drachsler H, Hummel HGK, Koper R. Identifying the goal, user model and conditions of recommender systems for formal and informal learning. Journal of Digital Information. 2009; 10 (2):1–17. [ Google Scholar ]
- Drachsler H, Verbert K, Santos OC, Manouselis N. Panorama of Recommender Systems to Support Learning. In: Ricci F, Rokach L, Shapira B, editors. Recommender Systems Handbook. Springer; 2015. pp. 421–451. [ Google Scholar ]
- Erdt M, Fernández A, Rensing C. Evaluating recommender systems for technology enhanced learning: A quantitative survey. IEEE Transactions on Learning Technologies. 2015; 8 (4):326–344. doi: 10.1109/TLT.2015.2438867. [ CrossRef ] [ Google Scholar ]
- Felder R. Learning and teaching styles in engineering education. Journal of Engineering Education. 1988; 78 :674–681. [ Google Scholar ]
- Fernandez-Garcia AJ, Rodriguez-Echeverria R, Preciado JC, Manzano JMC, Sanchez-Figueroa F. Creating a recommender system to support higher education students in the subject enrollment decision. IEEE Access. 2020; 8 :189069–189088. doi: 10.1109/ACCESS.2020.3031572. [ CrossRef ] [ Google Scholar ]
- Ferreira, V., Vasconcelos, G., & França, R. (2017). Mapeamento Sistemático sobre Sistemas de Recomendações Educacionais. Proceedings of the XXVIII Brazilian Symposium on Computers in Education , 253-262. 10.5753/cbie.sbie.2017.253
- Garcia-Martinez S, Hamou-Lhadj A. Educational recommender systems: A pedagogical-focused perspective. Multimedia Services in Intelligent Environments. Smart Innovation, Systems and Technologies. 2013; 25 :113–124. doi: 10.1007/978-3-319-00375-7_8. [ CrossRef ] [ Google Scholar ]
- George G, Lal AM. Review of ontology-based recommender systems in e-learning. Computers & Education. 2019; 142 :103642–103659. doi: 10.1016/j.compedu.2019.103642. [ CrossRef ] [ Google Scholar ]
- Harrathi M, Braham R. Recommenders in improving students’ engagement in large scale open learning. Procedia Computer Science. 2021; 192 :1121–1131. doi: 10.1016/j.procs.2021.08.115. [ CrossRef ] [ Google Scholar ]
- Herpich F, Nunes F, Petri G, Tarouco L. How Mobile augmented reality is applied in education? A systematic literature review. Creative Education. 2019; 10 :1589–1627. doi: 10.4236/ce.2019.107115. [ CrossRef ] [ Google Scholar ]
- Huang L, Wang C-D, Chao H-Y, Lai J-H, Yu PS. A score prediction approach for optional course recommendation via cross-user-domain collaborative filtering. IEEE Access. 2019; 7 :19550–19563. doi: 10.1109/ACCESS.2019.2897979. [ CrossRef ] [ Google Scholar ]
- Iaquinta, L., Gemmis, M. de,Lops, P., Semeraro, G., Filannino, M.& Molino, P. (2008). Introducing serendipity in a content-based recommender system. Proceedings of the Eighth International Conference on Hybrid Intelligent Systems , 168-173, 10.1109/HIS.2008.25
- Isinkaye FO, Folajimi YO, Ojokoh BA. Recommendation systems: Principles, methods and evaluation. Egyptian Informatics Journal. 2015; 16 (3):261–273. doi: 10.1016/j.eij.2015.06.005. [ CrossRef ] [ Google Scholar ]
- Ismail HM, Belkhouche B, Harous S. Framework for personalized content recommendations to support informal learning in massively diverse information Wikis. IEEE Access. 2019; 7 :172752–172773. doi: 10.1109/ACCESS.2019.2956284. [ CrossRef ] [ Google Scholar ]
- Khan KS, Kunz R, Kleijnen J, Antes G. Five steps to conducting a systematic review. Journal of the Royal Society of Medicine. 2003; 96 (3):118–121. doi: 10.1258/jrsm.96.3.118. [ PMC free article ] [ PubMed ] [ CrossRef ] [ Google Scholar ]
- Khanal SS, Prasad PWC, Alsadoon A, Maag A. A systematic review: Machine learning based recommendation systems for e-learning. Education and Information Technologies. 2019; 25 (4):2635–2664. doi: 10.1007/s10639-019-10063-9. [ CrossRef ] [ Google Scholar ]
- Khusro S, Ali Z, Ullah I. Recommender Systems: Issues, Challenges, and Research Opportunities. In: Kim K, Joukov N, editors. Lecture Notes in Electrical Engineering. Springer; 2016. pp. 1179–1189. [ Google Scholar ]
- Kitchenham, B. A., & Charters, S. (2007). Guidelines for performing Systematic Literature Reviews in Software Engineering. Technical Report EBSE 2007–001 . Keele University and Durham University Joint Report. https://www.elsevier.com/data/promis_misc/525444systematicreviewsguide.pdf .
- Kitchenham B, Pearl Brereton O, Budgen D, Turner M, Bailey J, Linkman S. Systematic literature reviews in software engineering – A systematic literature review. Information and Software Technology. 2009; 51 (1):7–15. doi: 10.1016/j.infsof.2008.09.009. [ CrossRef ] [ Google Scholar ]
- Klašnja-Milićević A, Ivanović M, Nanopoulos A. Recommender systems in e-learning environments: A survey of the state-of-the-art and possible extensions. Artificial Intelligence Review. 2015; 44 (4):571–604. doi: 10.1007/s10462-015-9440-z. [ CrossRef ] [ Google Scholar ]
- Klašnja-Milićević A, Vesin B, Ivanović M. Social tagging strategy for enhancing e-learning experience. Computers & Education. 2018; 118 :166–181. doi: 10.1016/j.compedu.2017.12.002. [ CrossRef ] [ Google Scholar ]
- Kolb, D., Boyatzis, R., Mainemelis, C., (2001). Experiential Learning Theory: Previous Research and New Directions Perspectives on Thinking, Learning and Cognitive Styles , 227–247.
- Krahenbuhl KS. Student-centered Education and Constructivism: Challenges, Concerns, and Clarity for Teachers. The Clearing House: A Journal of Educational Strategies, Issues and Ideas. 2016; 89 (3):97–105. doi: 10.1080/00098655.2016.1191311. [ CrossRef ] [ Google Scholar ]
- Kunaver M, Požrl T. Diversity in recommender systems – A survey. Knowledge-Based Systems. 2017; 123 :154–162. doi: 10.1016/j.knosys.2017.02.009. [ CrossRef ] [ Google Scholar ]
- Manouselis N, Drachsler H, Vuorikari R, Hummel H, Koper R. Recommender systems in technology enhanced learning. In: Ricci F, Rokach L, Shapira B, Kantor P, editors. Recommender Systems Handbook. Springer; 2010. pp. 387–415. [ Google Scholar ]
- Manouselis N, Drachsler H, Verbert K, Santos OC. Recommender systems for technology enhanced learning. Springer; 2014. [ Google Scholar ]
- Manouselis, N., Drachsler, H., Verbert, K., & Duval, E. (2013). Challenges and Outlook. Recommender Systems for Learning , 63–76. 10.1007/978-1-4614-4361-2
- Maravanyika M, Dlodlo N. An adaptive framework for recommender-based learning management systems. Open Innovations Conference (OI) 2018; 2018 :203–212. doi: 10.1109/OI.2018.8535816. [ CrossRef ] [ Google Scholar ]
- Maria, S. A. A., Cazella, S. C., & Behar, P. A. (2019). Sistemas de Recomendação: conceitos e técnicas de aplicação. Recomendação Pedagógica em Educação a Distância , 19–47, Penso.
- McCombs, B. L. (2013). The Learner-Centered Model: Implications for Research Approaches. In Cornelius-White, J., Motschnig-Pitrik, R. & Lux, M. (eds), Interdisciplinary Handbook of the Person-Centered Approach , 335–352. 10.1007/ 978-1-4614-7141-7_23
- Medeiros RP, Ramalho GL, Falcao TP. A systematic literature review on teaching and learning introductory programming in higher education. IEEE Transactions on Education. 2019; 62 (2):77–90. doi: 10.1109/te.2018.2864133. [ CrossRef ] [ Google Scholar ]
- Moher D, Shamseer L, Clarke M, Ghersi D, Liberati A, Petticrew M, Shekelle P, Stewart LA, PRISMA-P Group Preferred reporting items for systematic review and meta-analysis protocols (PRISMA-P) 2015 statement. Systematic Reviews. 2015; 4 (1):1. doi: 10.1186/2046-4053-4-1. [ PMC free article ] [ PubMed ] [ CrossRef ] [ Google Scholar ]
- Moubayed A, Injadat M, Nassif AB, Lutfiyya H, Shami A. E-Learning: Challenges and research opportunities using machine learning & data analytics. IEEE Access. 2018; 6 :39117–39138. doi: 10.1109/access.2018.2851790. [ CrossRef ] [ Google Scholar ]
- Nabizadeh AH, Gonçalves D, Gama S, Jorge J, Rafsanjani HN. Adaptive learning path recommender approach using auxiliary learning objects. Computers & Education. 2020; 147 :103777–103793. doi: 10.1016/j.compedu.2019.103777. [ CrossRef ] [ Google Scholar ]
- Nafea SM, Siewe F, He Y. On Recommendation of learning objects using Felder-Silverman learning style model. IEEE Access. 2019; 7 :163034–163048. doi: 10.1109/ACCESS.2019.2935417. [ CrossRef ] [ Google Scholar ]
- Nascimento PD, Barreto R, Primo T, Gusmão T, Oliveira E. Recomendação de Objetos de Aprendizagem baseada em Modelos de Estilos de Aprendizagem: Uma Revisão Sistemática da Literatura. Proceedings of XXVIII Brazilian Symposium on Computers in Education- SBIE. 2017; 2017 :213–222. doi: 10.5753/cbie.sbie.2017.213. [ CrossRef ] [ Google Scholar ]
- Nguyen QH, Ly H-B, Ho LS, Al-Ansari N, Le HV, Tran VQ, Prakash I, Pham BT. Influence of data splitting on performance of machine learning models in prediction of shear strength of soil. Mathematical Problems in Engineering. 2021; 2021 :1–15. doi: 10.1155/2021/4832864. [ CrossRef ] [ Google Scholar ]
- Nichols, D. M. (1998). Implicit rating and filtering. Proceedings of the Fifth Delos Workshop: Filtering and Collaborative Filtering , 31–36.
- Okoye, I., Maull, K., Foster, J., & Sumner, T. (2012). Educational recommendation in an informal intentional learning system. Educational Recommender Systems and Technologies , 1–23. 10.4018/978-1-61350-489-5.ch001
- Pai M, McCulloch M, Gorman JD, Pai N, Enanoria W, Kennedy G, Tharyan P, Colford JM., Jr Systematic reviews and meta-analyses: An illustrated, step-by-step guide. The National Medical Journal of India. 2004; 17 (2):86–95. [ PubMed ] [ Google Scholar ]
- Petri G, Gresse von Wangenheim C. How games for computing education are evaluated? A systematic literature review. Computers & Education. 2017; 107 :68–90. doi: 10.1016/j.compedu.2017.01.00. [ CrossRef ] [ Google Scholar ]
- Petticrew M, Roberts H. Systematic reviews in the social sciences a practical guide. Blackwell Publishing. 2006 doi: 10.1002/9780470754887. [ CrossRef ] [ Google Scholar ]
- Pinho PCR, Barwaldt R, Espindola D, Torres M, Pias M, Topin L, Borba A, Oliveira M. Developments in educational recommendation systems: a systematic review. Proceedings of 2019 IEEE Frontiers in Education Conference (FIE) 2019 doi: 10.1109/FIE43999.2019.9028466. [ CrossRef ] [ Google Scholar ]
- Pöntinen S, Dillon P, Väisänen P. Student teachers’ discourse about digital technologies and transitions between formal and informal learning contexts. Education and Information Technologies. 2017; 22 (1):317–335. doi: 10.1007/s10639-015-9450-0. [ CrossRef ] [ Google Scholar ]
- Pu, P., Chen, L., & Hu, R. (2011). A user-centric evaluation framework for recommender systems. Proceedings of the fifth ACM conference on Recommender systems , 157–164. 10.1145/2043932.2043962
- Rahman MM, Abdullah NA. A personalized group-based recommendation approach for web search in E-Learning. IEEE Access. 2018; 6 :34166–34178. doi: 10.1109/ACCESS.2018.2850376. [ CrossRef ] [ Google Scholar ]
- Ricci, F., Rokach, L., & Shapira, B. (2015). Recommender Systems: Introduction and Challenges. I Ricci, F., Rokach, L., Shapira, B. (eds), Recommender Systems Handbook , 1–34. 10.1007/978-1-4899-7637-6_1
- Rivera, A. C., Tapia-Leon, M., & Lujan-Mora, S. (2018). Recommendation Systems in Education: A Systematic Mapping Study. Proceedings of the International Conference on Information Technology & Systems (ICITS 2018) , 937–947. 10.1007/978-3-319-73450-7_89
- Salazar C, Aguilar J, Monsalve-Pulido J, Montoya E. Affective recommender systems in the educational field. A systematic literature review. Computer Science Review. 2021; 40 :100377. doi: 10.1016/j.cosrev.2021.100377. [ CrossRef ] [ Google Scholar ]
- Santos IM, Ali N. Exploring the uses of mobile phones to support informal learning. Education and Information Technologies. 2012; 17 (2):187–203. doi: 10.1007/s10639-011-9151-2. [ CrossRef ] [ Google Scholar ]
- Sergis S, Sampson DG. Learning object recommendations for teachers based on elicited ICT competence profiles. IEEE Transactions on Learning Technologies. 2016; 9 (1):67–80. doi: 10.1109/TLT.2015.2434824. [ CrossRef ] [ Google Scholar ]
- Shani G, Gunawardana A. Evaluating recommendation systems. In: Ricci F, Rokach L, Shapira B, Kantor P, editors. Recommender Systems Handbook. Springer; 2010. pp. 257–297. [ Google Scholar ]
- Tahereh, M., Maryam, T. M., Mahdiyeh, M., & Mahmood, K. (2013). Multi dimensional framework for qualitative evaluation in e-learning. 4th International Conference on e-Learning and e-Teaching (ICELET 2013), 69–75. 10.1109/icelet.2013.6681648
- Tarus JK, Niu Z, Yousif A. A hybrid knowledge-based recommender system for e-learning based on ontology and sequential pattern mining. Future Generation Computer Systems. 2017; 72 :37–48. doi: 10.1016/j.future.2017.02.049. [ CrossRef ] [ Google Scholar ]
- Tarus JK, Niu Z, Mustafa G. Knowledge-based recommendation: A review of ontology-based recommender systems for e-learning. Artificial Intelligence Review. 2018; 50 (1):21–48. doi: 10.1007/s10462-017-9539-5. [ CrossRef ] [ Google Scholar ]
- Verbert K, Manouselis N, Ochoa X, Wolpers M, Drachsler H, Bosnic I, Duval E. Context-aware recommender systems for learning: A survey and future challenges. IEEE Transactions on Learning Technologies. 2012; 5 (4):318–335. doi: 10.1109/TLT.2012.11. [ CrossRef ] [ Google Scholar ]
- Verbert, K., Drachsler, H., Manouselis, N., Wolpers, M., Vuorikari, R., & Duval, E. (2011). Dataset-Driven Research for Improving Recommender Systems for Learning. Proceedings of the 1st International Conference on Learning Analytics and Knowledge , 44–53. 10.1145/2090116.2090122
- Wan S, Niu Z. A learner oriented learning recommendation approach based on mixed concept mapping and immune algorithm. Knowledge-Based Systems. 2016; 103 :28–40. doi: 10.1016/j.knosys.2016.03.022. [ CrossRef ] [ Google Scholar ]
- Wan S, Niu Z. An e-learning recommendation approach based on the self-organization of learning resource. Knowledge-Based Systems. 2018; 160 :71–87. doi: 10.1016/j.knosys.2018.06.014. [ CrossRef ] [ Google Scholar ]
- Wan S, Niu Z. A hybrid E-Learning recommendation approach based on learners’ influence propagation. IEEE Transactions on Knowledge and Data Engineering. 2020; 32 (5):827–840. doi: 10.1109/TKDE.2019.2895033. [ CrossRef ] [ Google Scholar ]
- Watkins KE, Marsick VJ. Informal and incidental learning in the time of COVID-19. Advances in Developing Human Resources. 2020; 23 (1):88–96. doi: 10.1177/1523422320973656. [ CrossRef ] [ Google Scholar ]
- Wu D, Lu J, Zhang G. A Fuzzy Tree Matching-based personalized E-Learning recommender system. IEEE Transactions on Fuzzy Systems. 2015; 23 (6):2412–2426. doi: 10.1109/TFUZZ.2015.2426201. [ CrossRef ] [ Google Scholar ]
- Wu Z, Li M, Tang Y, Liang Q. Exercise recommendation based on knowledge concept prediction. Knowledge-Based Systems. 2020; 210 :106481–106492. doi: 10.1016/j.knosys.2020.106481. [ CrossRef ] [ Google Scholar ]
- Yanes N, Mostafa AM, Ezz M, Almuayqil SN. A machine learning-based recommender system for improving students learning experiences. IEEE Access. 2020; 8 :201218–201235. doi: 10.1109/ACCESS.2020.3036336. [ CrossRef ] [ Google Scholar ]
- Zapata A, Menéndez VH, Prieto ME, Romero C. Evaluation and selection of group recommendation strategies for collaborative searching of learning objects. International Journal of Human-Computer Studies. 2015; 76 :22–39. doi: 10.1016/j.ijhcs.2014.12.002. [ CrossRef ] [ Google Scholar ]
- Zhang S, Yao L, Sun A, Tay Y. Deep learning based recommender system. ACM Computing Surveys. 2020; 52 (1):1–38. doi: 10.1145/3285029. [ CrossRef ] [ Google Scholar ]
- Zhong J, Xie H, Wang FL. The research trends in recommender systems for e-learning: A systematic review of SSCI journal articles from 2014 to 2018. Asian Association of Open Universities Journal. 2019; 14 (1):12–27. doi: 10.1108/AAOUJ-03-2019-0015. [ CrossRef ] [ Google Scholar ]
Exploring the Landscape of Recommender Systems Evaluation: Practices and Perspectives

New Citation Alert added!
This alert has been successfully added and will be sent to:
You will be notified whenever a record that you have chosen has been cited.
To manage your alert preferences, click on the button below.
New Citation Alert!
Please log in to your account
Information & Contributors
Bibliometrics & citations, view options, 1 introduction, 2 material and methods.

2.1 Literature Search

2.2 Data Cleansing and Selection of Papers for the Sample
Papers | Venues | Year |
---|---|---|
Saraswat et al. [ ] | AIML Systems | 2021 |
Jannach [ ] | ARTR | 2023 |
Eftimov et al. [ ] | BDR | 2021 |
Sonboli et al. [ ], Zhu et al. [ ] | CIKM | 2021 |
Ekstrand [ ] | CIKM | 2020 |
Alhijawi et al. [ ], Sánchez and Bellogín [ ], Zangerle and Bauer [ ] | CSUR | 2022 |
Jin et al. [ ] | HAI | 2021 |
Belavadi et al. [ ] | HCII | 2021 |
Peska and Vojtas [ ] | HT | 2020 |
Ostendorff et al. [ ] | ICADL | 2021 |
Afolabi and Toivanen [ ] | IJEHMC | 2020 |
Bellogín et al. [ ] | IRJ | 2017 |
Latifi et al. [ ] | ISCI | 2022 |
Carraro and Bridge [ ] | JIIS | 2022 |
Krichene and Rendle [ ], Li et al. [ ], McInerney et al. [ ] | KDD | 2020 |
Dehghani Champiri et al. [ ] | KIS | 2019 |
Latifi and Jannach [ ] | RecSys | 2022 |
Dallmann et al. [ ], Narita et al. [ ], Parapar and Radlinski [ ], Saito et al. [ ] | RecSys | 2021 |
Cañamares and Castells [ ], Kouki et al. [ ], Sun et al. [ ], Symeonidis et al. [ ] | RecSys | 2020 |
Ferrari Dacrema et al. [ ] | RecSys | 2019 |
Yang et al. [ ] | RecSys | 2018 |
Xin et al. [ ] | RecSys | 2017 |
Ali et al. [ ] | Scientometrics | 2021 |
Diaz and Ferraro [ ], Silva et al. [ ] | SIGIR | 2022 |
Anelli et al. [ ], Li et al. [ ], Lu et al. [ ] | SIGIR | 2021 |
Balog and Radlinski [ ], Mena-Maldonado et al. [ ] | SIGIR | 2020 |
Cañamares and Castells [ ] | SIGIR | 2018 |
Cañamares and Castells [ ] | SIGIR | 2017 |
Chen et al. [ ] | TheWebConf | 2019 |
Al Jurdi et al. [ ] | TKDD | 2021 |
Guo et al. [ ] | TOCHI | 2022 |
Zhao et al. [ ] | TOIS | 2022 |
Ferrari Dacrema et al. [ ], Mena-Maldonado et al. [ ] | TOIS | 2021 |
Anelli et al. [ ] | UMAP | 2022 |
Frumerman et al. [ ] | UMAP | 2019 |
Bellogín and Said [ ] | UMUAI | 2021 |
Said and Bellogín [ ] | UMUAI | 2018 |
Chin et al. [ ], Kiyohara et al. [ ] | WSDM | 2022 |
Cotta et al. [ ] | WSDM | 2019 |
Gilotte et al. [ ] | WSDM | 2018 |
2.3 Review of the Selected Papers in Full Text (Coding)
Types of Contribution | Description |
---|---|
Benchmark | Providing an extensive critical evaluation across a (wide) set of approaches or datasets |
Framework | Introducing a framework for evaluation, which may take the form of a toolkit or a conceptual framework |
Metrics | Analyzing existing or introducing novel metrics of evaluation |
Model | Introducing a novel recommendation or evaluation model |
Survey | A literature survey |
3.1 General Overview

3.2 Type of Contribution

Papers | Details |
---|---|
Anelli et al. [ ] | Reproducibility study. An in-depth, systematic, and reproducible comparison of 10 collaborative filtering algorithms (including approaches based on nearest-neighbors, matrix factorization, linear models, and techniques based on deep learning) using three datasets and the identical evaluation protocol. Provide a guide for future research with respect to baselines and systematic evaluation. |
Dallmann et al. [ ] | Study sampling strategies for sequential item recommendation. Compare four methods across five datasets and find that both, uniform random sampling and sampling by popularity, can produce inconsistent rankings compared with the full ranking of the models. |
Ferrari Dacrema et al. [ ], ] | Reproducibility study. Critical analysis of the performance of 12 neural recommendation approaches with reproducible setup. Comparison against well-tuned, established, non-neural baseline methods. Identification of several methodological issues, including choice of baselines, propagation of weak baselines, and a lack of proper tuning of baselines. |
Kouki et al. [ ] | Compare 14 models (8 baseline and 6 deep learning) for session-based recommendations using 8 different popular evaluation metrics. |
Latifi and Jannach [ ] | Reproducibility study. Benchmark Graph Neural Networks against an effective session-based nearest neighbor method. The conceptually simpler method outperforms the GNN-based method both in terms of Hit Ratio and the MRR. |
Latifi et al. [ ] | Compare the Transformer-based BERT4Rec method [ ] to nearest-neighbor methods for sequential recommendation problems across four datasets using exact and sampled metrics. The nearest-neighbor methods achieve comparable or better performance than BERT4Rec for the smaller datasets, whereas BERT4Rec outperforms the simple methods for the larger ones. |
Sun et al. [ ] | Benchmarks across several datasets, recommendation approaches, and metrics; in addition, it introduces the toolkit daisyRec. |
Zhu et al. [ ] | Open benchmarking for click-through rate prediction with a rigorous comparison of 24 existing models on multiple dataset settings in a reproducible manner. The evaluation framework for CTR (including the benchmarking tools, evaluation protocols, and experimental settings) are publicly available. |
Papers | Details |
---|---|
Al Jurdi et al. [ ] | Classification of natural noise management (NNM) techniques and analysis of their strengths and weaknesses. Comparative statistical analysis of the NNM mechanisms. |
Alhijawi et al. [ ] | Specifically address the objectives: relevance, diversity, novelty, coverage, and serendipity. Reviews the definitions and measures associated with these objectives. Classifies over 100 articles (published from 2015 to 2020) regarding objective-oriented evaluation measures and methodologies. Collect 43 objective-oriented evaluation measures. |
Ali et al. [ ] | Survey on the evaluation of scholarly recommender systems. Analysis suggests that there is a focus on offline experiments, whereby either simple/trivial baselines are used or no baselines at all. |
Chin et al. [ ] | Compare 45 datasets used for implicit feedback based top- recommendation based on characteristics (similarities and differences) and usage patterns across papers. For 15 datasets, they evaluate and compare the performance of five different recommendation algorithms. |
Dehghani Champiri et al. [ ] | Focus on context-aware scholarly recommender systems. Classification evaluation methods and metrics on usage. |
Jannach [ ] | Provide an overview of evaluation aspects as reported in 127 papers on conversational recommender systems. Argue for a mixed methods approach, combining objective (computational) and subjective (perception-oriented) techniques for the evaluation of conversational recommenders, because these are complex multi-component applications, consisting of multiple machine learning models and a natural language user interface. |
Sánchez and Bellogín [ ] | Focus on point-of-interest recommender systems. Systematic review covering 10 years of research on that topic, categorizing the algorithms and evaluation methodologies used. The common problems are that both, the algorithms and the used datasets (statistics), are described in insufficient detail. |
Zangerle and Bauer [ ] | Introduce “Framework for EValuating Recommender systems,” derived from the discourse on recommender systems evaluation. Categorization of the evaluation space of recommender systems evaluation. Emphasis on the required multi-facettedness of a comprehensive evaluation of a recommender system. |
Zhao et al. [ ] | Survey of 93 offline evaluation for top- recommendation algorithms. Provide an overview of aspects related to evaluation metrics, dataset construction, and model optimization. In addition, this work presents a systematic comparison of 12 top- recommendation algorithms (covering both traditional and neural-based algorithms) across eight datasets. |
3.3 Experiment Types

Papers | Online experiment | Offline experiment | User study |
---|---|---|---|
Gilotte et al. [ ] | x | x | |
Narita et al. [ ] | x | x | |
Peska and Vojtas [ ] | x | x | |
Symeonidis et al. [ ] | x | x | |
Frumerman et al. [ ] | x | x | |
Said and Bellogín [ ] | x | x | |
Belavadi et al. [ ] | x | x | |
Kouki et al. [ ] | x | x | x |
3.4 Datasets
Datasets | Papers | # Papers |
---|---|---|
Amazon Beauty [ ] | [ , , ] | 3 |
Amazon Book [ ] | [ ] | 1 |
Amazon Digital Music [ ] | [ , ] | 2 |
Amazon Electronics [ ] | [ , , ] | 3 |
Amazon Home & Kitchen [ ] | [ ] | 1 |
Amazon Instant Video [ ] | [ ] | 1 |
Amazon Kindle Store [ ] | [ ] | 1 |
Amazon Movies & TV [ ] | [ , , ] | 3 |
Amazon Musical Instruments [ ] | [ , ] | 2 |
Amazon Patio, Lawn & Garden [ ] | [ ] | 1 |
Amazon Sports & Outdoors [ ] | [ ] | 1 |
Amazon Toys & Games [ ] | [ , ] | 2 |
Amazon Video Games [ ] | [ , , ] | 3 |
Avazu | [ ] | 1 |
BeerAdvocate [ ] | [ ] | 1 |
Book crossing [ ] | [ ] | 1 |
citeulike-a [ ] | [ , , ] | 3 |
citeULike-t [ ] | [ , , ] | 3 |
Clothing Fit [ ] | [ ] | 1 |
CM100k [ ] | [ , ] | 2 |
CoatShopping [ ] | [ ] | 1 |
Criteo | [ ] | 1 |
epinions [ ] | [ , , , ] | 4 |
Filmtrust [ ] | [ ] | 1 |
Flixster | [ ] | 1 |
Frappe [ ] | [ ] | 1 |
Good Books | [ ] | 1 |
Good Reads | [ ] | 1 |
Gowalla [ ] | [ , ] | 2 |
LastFM [ ] | [ , , , , , , , , ] | 9 |
Library-Thing [ ] | [ ] | 1 |
Million Playlist Dataset | [ ] | 1 |
Million Post Corpus [ ] | [ ] | 1 |
MovieLens 100k [ ] | [ , , ] | 3 |
MovieLens 1M [ ] | [ , , , , , , , , , , , , , , , , , , ] | 19 |
MovieLens 10M [ ] | [ , ] | 2 |
MovieLens 20M [ ] | [ , , , , ] | 5 |
MovieLens 25M [ ] | [ ] | 1 |
MovieLens Latest [ ] | [ ] | 1 |
MovieLens HetRec | [ ] | 1 |
MoviePilot | [ ] | 1 |
NetflixPrize | [ , , , , ] | 5 |
Open Bandit [ ] | [ ] | 1 |
Pinterest [ ] | [ , ] | 2 |
Steam [ ] | [ , ] | 2 |
Ta Feng Grocery Dataset | [ ] | 1 |
Tradesy [ ] | [ ] | 1 |
TREC Common Core 2017 [ ] | [ ] | 1 |
TREC Common Core 2018 | [ ] | 1 |
TREC Deep Learning Document Ranking 2019 [ ] | [ ] | 1 |
TREC Deep Learning Document Ranking 2020 [ ] | [ ] | 1 |
TREC Deep Learning Passage Ranking 2019 [ ] | [ ] | 1 |
TREC Deep Learning Passage Ranking 2020 [ ] | [ ] | 1 |
TREC Robust 2004 | [ ] | 1 |
TREC Web 2009 [ ] | [ ] | 1 |
TREC Web 2010 | [ ] | 1 |
TREC Web 2011 | [ ] | 1 |
TREC Web 2012 [ ] | [ ] | 1 |
TREC Web 2013 | [ ] | 1 |
TREC Web 2014 [ ] | [ ] | 1 |
Webscope R3 [ ] | [ ] | 1 |
Yelp | [ , , , , ] | 5 |
Yahoo R3 (Music) | [ , , , ] | 4 |
Yahoo R4 | [ ] | 1 |
Xing [ ] | [ ] | 1 |
Custom | [ , , , , , , , , , , , , , , ] | 15 |

Datasets | Domains | Feedback | Interactions | Side Information |
---|---|---|---|---|
Amazon Electronics, Products, Video Games [ ] | Products | [1,5] | 20,994,353 (E), 371,345 (B), 2,565,349 (V) | product information (e.g., description, color, product images, technical details), timestamp |
citeulike-a, citeulike-t | Scientific Papers | {0,1} | 204,987 (a), 134,860 (t) | tags, bag-of-words, and raw text for each article, citations between articles |
epinions [ ] | Products | [0,5] | 922,267 | explicit trust relationships among users, timestamps |
LastFM [ ] | Music | {0,1} | 19,150,868 | artist, song name, timestamp |
MovieLens (100k, 1M, 20M) [ ] | Movies | [0,5] | 100,000 (100k)–20,000,000 (20M) | movie metadata (e.g., title, genre), user metadata (e.g., age, gender), rating timestamp |
NetflixPrize | Movies | [1,5] | 100,000,000 | movie metadata (title, release year), rating date |
Yelp | Business | [0,5] | 6,990,280 | business metadata (address, category, etc.), user metadata (user name, user stats (no. of reviews, user votes, etc.)), rating timestamp |
Dataset Combinations | # Papers |
---|---|
{LastFM, ML 1M} | 7 |
{ML 1M, NetflixPrize}, {ML 1M, Yelp} | 5 |
{ML 1M, Yahoo R3}, {LastFM, Yelp}, {LastFM, NetflixPrize}, {LastFM, ML 1M, NetflixPrize}, {LastFM, ML 1M, Yelp} | 4 |
{Amazon Movies & TV, LastFM}, {Amazon Electronics, LastFM}, {Amazon Beauty, ML 20M}, {epinions, ML 1M}, {ML 100k, ML 20M}, {ML 100k, ML 1M}, {ML 1M, ML20M} | 3 |
3.5 Metrics
Metrics | Abbr. | Papers | # |
---|---|---|---|
Area Under Curve | AUC | [ , , , , ] | 5 |
Average Coverage of Long Tail | ACLT | [ ] | 1 |
Average Percentage of Long Tail | APLT | [ ] | 1 |
Average Precision | AP | [ , , , ] | 4 |
Average Recommendation Popularity | ARP | [ ] | 1 |
Binary Preference-based measure | bpref | [ ] | 1 |
Clickthrough rate | CTR | [ , , , ] | 4 |
Conversion rate | CVR | [ ] | 1 |
Coverage (item) | Coverage | [ , , ] | 3 |
Coverage (user) | [ ] | 1 | |
Discounted Cumulative Gain | DCG | [ ] | 1 |
Expected Free Discovery | EFD | [ ] | 1 |
Expected Popularity Complement | EPC | [ , ] | 2 |
Expected Profile Distance | EPD | [ ] | 1 |
F-measure | F1 | [ ] | 1 |
Fallout | [ ] | 1 | |
Gini | [ , ] | 2 | |
Hit Rate | HR | [ , , , , , , , ] | 8 |
Hits | [ ] | 1 | |
Intra-list Diversity | ILD | [ ] | 1 |
Inferred Average Precision | InfAP | [ ] | 1 |
Item Coverage | IC | [ ] | 1 |
Jaccard coefficient | [ ] | 1 | |
Logistic Loss | Logloss | [ ] | 1 |
Mean Absolute Error | MAE | [ ] | 1 |
Mean Average Precision | MAP | [ , , , , , , , ] | 8 |
Mean Reciprocal Rank | MRR | [ , , , , , , , ] | 8 |
Mean Squared Error | MSE | [ , ] | 2 |
normalized Discounted Cumulative Gain | nDCG | [ , , , , , , , , , , , , , , , , , , , ] | 20 |
Novelty | [ ] | 1 | |
Overlap | [ ] | 1 | |
Pearson Correlation Coefficient | PCC | [ ] | 1 |
Popularity | [ ] | 1 | |
Popularity-based Ranking-based Equal Opportunity | PREO | [ ] | 1 |
Popularity-based Ranking-based Statistical Parity | PRSP | [ ] | 1 |
Precision | P | [ , , , , , , , , , , , , , , , , , , , , , ] | 22 |
Recall | R | [ , , , , , , , , , , , , , , , , ] | 17 |
Reciprocal Rank | RR | [ , ] | 2 |
Root Mean Squared Error | RMSE | [ , , , , ] | 5 |
Custom | [ , , , , , , , , , ] | 12 | |

Categories | Metrics |
---|---|
Relevance | AP, AUC, F1, fallout, Hits, HR, InfAP, Logloss, MAP, P, R |
Success Rate | CTR, CVR |
Rating Prediction Accuracy | bpref, MAE, MSE, RMSE |
Ranking | DCG, nDCG, MRR, RR |
Non-accuracy | ACLT, APLT, Coverage, EFD, EPC, EPD, Gini, IC, ILD, Jaccard, Overlap, PCC, Popularity, PREO, PRSP |
Metric combinations | # Papers |
---|---|
{nDCG, P} \(^*\) | 14 |
{nDCG, R} \(^*\) | 13 |
{P, R} | 12 |
{nDCG, P, R} \(^*\) | 10 |
{nDCG, MAP} \(^*\) , {R, MAP}, {nDCG, R, MAP} \(^*\) | 8 |
{nDCG, P, MAP} \(^*\) , {P, MAP}, {nDCG, P, R, MAP} \(^*\) , {nDCG, MRR}, {P, R, MAP} | 7 |
{nDCG, MAP, MRR, R} \(^*\) , {MRR, P, MAP, R} \(^*\) , {nDCG, MRR, MAP} \(^*\) , {MRR, MAP, R} \(^*\) , {MRR, P, MAP} \(^*\) , {nDCG, P, MRR, MAP} \(^*\) , {MRR, P} \(^*\) , {MRR, R} \(^*\) , {MRR, MAP} \(^*\) , {nDCG, HR} \(^*\) , {nDCG, P, MRR, R} \(^*\) , {MRR, HR} \(^*\) , {MRR, P, R} \(^*\) , {nDCG, P, MRR} \(^*\) , {nDCG, MRR, R} \(^*\) , {nDCG, MAP, MRR, P, R} \(^*\) | 6 |
{P, HR}, {nDCG, HR, MRR} \(^*\) | 5 |
{nDCG, P, HR, MAP} \(^*\) , {P, HR, R, MAP}, {nDCG, HR, R} \(^*\) , {nDCG, HR, R, MAP} \(^*\) , {MRR, P, HR, R} \(^*\) , {nDCG, P, HR, MRR} \(^*\) , {nDCG, HR, MRR, R} \(^*\) , {nDCG, P, HR, R} \(^*\) , {MRR, MAP, HR, R} \(^*\) , {MAP, MRR, P, HR, R} \(^*\) , {nDCG, MRR, P, HR, MAP} \(^*\) , {nDCG, MAP, MRR, HR, R} \(^*\) , {nDCG, MAP, P, HR, R} \(^*\) , {nDCG, MRR, P, HR, R} \(^*\) , {nDCG, HR, MRR, MAP} \(^*\) , {nDCG, MRR, P, HR, R, MAP} \(^*\) , {MRR, P, HR, MAP} \(^*\) , {nDCG, P, HR} \(^*\) , {P, HR, R}, {MRR, HR, R} \(^*\) , {MRR, P, HR} \(^*\) , {nDCG, HR, MAP} \(^*\) , {HR, R, MAP}, {P, HR, MAP}, {MRR, HR, MAP} \(^*\) , {HR, R}, {HR, MAP} | 4 |
{Coverage, HR} \(^*\) , {P, AUC}, {AUC, R}, {nDCG, AUC} \(^*\) , {P, Coverage, HR} \(^*\) , {P, Coverage} \(^*\) , {nDCG, AUC, R} \(^*\) , {nDCG, AP} \(^*\) , {AP, R} | 3 |
4 Discussion
5 conclusions, supplementary material.
- Sun A (2024) Beyond Collaborative Filtering: A Relook at Task Formulation in Recommender Systems ACM SIGWEB Newsletter 10.1145/3663752.3663756 2024 :Spring (1-11) Online publication date: 18-Jun-2024 https://dl.acm.org/doi/10.1145/3663752.3663756
- Bauer C Said A Zangerle E (2024) Introduction to the Special Issue on Perspectives on Recommender Systems Evaluation ACM Transactions on Recommender Systems 10.1145/3648398 2 :1 (1-5) Online publication date: 7-Mar-2024 https://dl.acm.org/doi/10.1145/3648398
Index Terms
Human-centered computing
Human computer interaction (HCI)
HCI design and evaluation methods
Information systems
Information retrieval
Evaluation of retrieval results
Retrieval tasks and goals
Recommender systems
Recommendations
Introduction to the special issue on perspectives on recommender systems evaluation.
Evaluation plays a vital role in recommender systems—in research and practice—whether for confirming algorithmic concepts or assessing the operational validity of designs and applications. It may span the evaluation of early ideas and approaches up to ...
Harmonization and Categorization of Metrics and Criteria for Evaluation of Recommender Systems in Healthcare From Dual Perspectives
Researchers' choice of metrics and criteria in evaluating recommender systems depends on what the researcher feels is popular among other researchers, or sometimes based on the objective of the research. There is no harmonized set of criteria and ...
Third Workshop: Perspectives on the Evaluation of Recommender Systems (PERSPECTIVES 2023)
Evaluation is important when developing and deploying recommender systems. The PERSPECTIVES workshop sheds light on the different, potentially diverging or contradictory perspectives on the evaluation of recommender systems. Building on the discussions ...
Information
Published in.

Hong Kong Baptist University, China

University of Klagenfurt, Austria
Association for Computing Machinery
New York, NY, United States
Publication History
Check for updates, author tags.
- systematic literature review
- recommender systems
- Research-article
Funding Sources
- Austrian Science Fund (FWF)
Contributors
Other metrics, bibliometrics, article metrics.
- 2 Total Citations View Citations
- 1,892 Total Downloads
- Downloads (Last 12 months) 1,892
- Downloads (Last 6 weeks) 238
View options
View or Download as a PDF file.
View online with eReader .
Login options
Check if you have access through your login credentials or your institution to get full access on this article.
Full Access
Share this publication link.
Copying failed.
Share on social media
Affiliations, export citations.
- Please download or close your previous search result export first before starting a new bulk export. Preview is not available. By clicking download, a status dialog will open to start the export process. The process may take a few minutes but once it finishes a file will be downloadable from your browser. You may continue to browse the DL while the export process is in progress. Download
- Download citation
- Copy citation
We are preparing your search results for download ...
We will inform you here when the file is ready.
Your file of search results citations is now ready.
Your search export query has expired. Please try again.
- DOI: 10.13088/JIIS.2011.17.1.139
- Corpus ID: 13476303
A Literature Review and Classification of Recommender Systems on Academic Journals
- D. Park , Hyeakyeong Kim , +1 author Jae Kyeong Kim
- Published 1 March 2011
- Computer Science
10 Citations
Movie recommender systems: concepts, methods, challenges, and future directions, recommendation systems on e-learning and social learning: a systematic review, the effect of the personalized settings for cf-based recommender systems, restaurant information extraction (including opinion mining elements) for the recommendation system, an intelligent patent recommender adopting machine learning approach for natural language processing: a case study for smart machinery technology mining, corpus-based information extraction and opinion mining for the restaurant recommendation system, preference-based supply chain partner selection using fuzzy ontology, queuing time computation algorithm for sensor data processing in real-time ubiquitous environment, designing an ai-enabled bundling generator in an automotive case study, designing an ai purchasing requisition bundling generator, 23 references, application of dimensionality reduction in recommender system - a case study, e-commerce recommendation applications, a personalized recommendation procedure for internet shopping support, a personalized recommender system based on web usage mining and decision tree induction, a flexible semantic inference methodology to reason about user preferences in knowledge-based recommender systems, modeling user multiple interests by an improved gcs approach, a group recommendation system for online communities, personalized recommendation over a customer network for ubiquitous shopping, content-independent task-focused recommendation, an open architecture for collaborative filtering of netnews, related papers.
Showing 1 through 3 of 0 Related Papers
To read this content please select one of the options below:
Please note you do not have access to teaching notes, recommender systems: a systematic review of the state of the art literature and suggestions for future research.
ISSN : 0368-492X
Article publication date: 15 March 2018
Issue publication date: 2 May 2018
This paper aims to identify, evaluate and integrate the findings of all relevant and high-quality individual studies addressing one or more research questions about recommender systems and performing a comprehensive study of empirical research on recommender systems that have been divided into five main categories. To achieve this aim, the authors use systematic literature review (SLR) as a powerful method to collect and critically analyze the research papers. Also, the authors discuss the selected recommender systems and its main techniques, as well as their benefits and drawbacks in general.
Design/methodology/approach
In this paper, the SLR method is utilized with the aim of identifying, evaluating and integrating the findings of all relevant and high-quality individual studies addressing one or more research questions about recommender systems and performing a comprehensive study of empirical research on recommender systems that have been divided into five main categories. Also, the authors discussed recommender system and its techniques in general without a specific domain.
The major developments in categories of recommender systems are reviewed, and new challenges are outlined. Furthermore, insights on the identification of open issues and guidelines for future research are provided. Also, this paper presents the systematical analysis of the recommender system literature from 2005. The authors identified 536 papers, which were reduced to 51 primary studies through the paper selection process.
Originality/value
This survey will directly support academics and practical professionals in their understanding of developments in recommender systems and its techniques.
- Recommender system
- Collaborative filtering
- Demographic filtering
Alyari, F. and Jafari Navimipour, N. (2018), "Recommender systems: A systematic review of the state of the art literature and suggestions for future research", Kybernetes , Vol. 47 No. 5, pp. 985-1017. https://doi.org/10.1108/K-06-2017-0196
Emerald Publishing Limited
Copyright © 2018, Emerald Publishing Limited
Related articles
All feedback is valuable.
Please share your general feedback
Report an issue or find answers to frequently asked questions
Contact Customer Support
Information
- Author Services
Initiatives
You are accessing a machine-readable page. In order to be human-readable, please install an RSS reader.
All articles published by MDPI are made immediately available worldwide under an open access license. No special permission is required to reuse all or part of the article published by MDPI, including figures and tables. For articles published under an open access Creative Common CC BY license, any part of the article may be reused without permission provided that the original article is clearly cited. For more information, please refer to https://www.mdpi.com/openaccess .
Feature papers represent the most advanced research with significant potential for high impact in the field. A Feature Paper should be a substantial original Article that involves several techniques or approaches, provides an outlook for future research directions and describes possible research applications.
Feature papers are submitted upon individual invitation or recommendation by the scientific editors and must receive positive feedback from the reviewers.
Editor’s Choice articles are based on recommendations by the scientific editors of MDPI journals from around the world. Editors select a small number of articles recently published in the journal that they believe will be particularly interesting to readers, or important in the respective research area. The aim is to provide a snapshot of some of the most exciting work published in the various research areas of the journal.
Original Submission Date Received: .
- Active Journals
- Find a Journal
- Journal Proposal
- Proceedings Series
- For Authors
- For Reviewers
- For Editors
- For Librarians
- For Publishers
- For Societies
- For Conference Organizers
- Open Access Policy
- Institutional Open Access Program
- Special Issues Guidelines
- Editorial Process
- Research and Publication Ethics
- Article Processing Charges
- Testimonials
- Preprints.org
- SciProfiles
- Encyclopedia
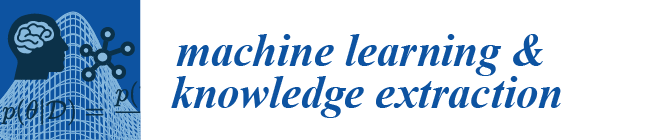
Article Menu

- Subscribe SciFeed
- Google Scholar
- on Google Scholar
- Table of Contents
Find support for a specific problem in the support section of our website.
Please let us know what you think of our products and services.
Visit our dedicated information section to learn more about MDPI.
JSmol Viewer
Systematic review of recommendation systems for course selection.
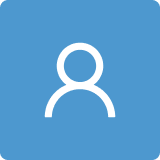
1. Introduction
2. motivation and rationale of the research, 3. research questions, 3.1. questions about the used algorithms.
- What preprocessing methods were applied?
- What recommendation system algorithms were used in the paper?
- What are the applied evaluation metrics?
- What are the performance results of applied evaluation metrics?
3.2. Questions about the Used Dataset
- Is the dataset published or accessible?
- How many records are there in the dataset?
- How many unique student records are there in the dataset?
- How many unique course records are there in the dataset?
- How many features are there in the dataset?
- How many features are used from the existing features?
- How many unique majors are there in the dataset?
- How did the authors split the training and testing set?
3.3. Questions about the Research
- What is the type of comparative produced in the study (algorithm level, preprocessing level, or data level)?
- What is the main aim of the study?
- What are the strong points of the research?
- What are the weak points of the research?
4. Research Methodology
4.1. title-level screening stage.
- The study addresses recommendation systems in the Education sector.
- The study must be primary.
4.2. Abstract-Level Screening Stage
4.3. full-text article scanning stage.
- The study was written in the English language.
- The study implies empirical experiments and provides the experiment’s results.
4.4. Full-Text Article Screening Stage
- Q1: Did the study conduct experiment in the course selection and courses recommendation system?
- Q2: Is there a comparison with other approaches in the conducted study?
- Q3: Were the performance measures fully defined?
- Q4: Was the method used in the study clearly described?
- Q5: Was the dataset and number of training and testing data identified?
4.5. Data Extraction Stage
5. research results, 5.1. the studies included in the slr, 5.1.1. collaborative filtering studies, 5.1.2. content-based filtering studies, 5.1.3. hybrid recommender system studies, 5.1.4. studies based on machine learning, 5.1.5. similarity-based study, 6. key studies analysis, 6.1. discussion of aims and contributions of the existing research works, 6.1.1. aim of studies that used collaborative filtering, 6.1.2. aim of studies that used content-based filtering, 6.1.3. aim of studies that used hybrid recommender systems, 6.1.4. aim of studies that used novel approaches, 6.1.5. aim of studies that used similarity-based filtering, 6.2. description of datasets used in the studies, 6.2.1. dataset description of studies that used collaborative filtering, 6.2.2. dataset description of studies that used content-based filtering, 6.2.3. dataset description of studies that used hybrid recommender systems, 6.2.4. dataset description of studies that used novel approaches.
- Train-test split.
- K-fold cross-validation.
- Nested time series splits.
6.2.5. Dataset Description of the Study That Used Similarity-Based Filtering
6.3. research evaluation, 6.3.1. research evaluation for studies that used collaborative filtering, 6.3.2. research evaluation for studies that used content-based filtering, 6.3.3. research evaluation for studies that used hybrid recommender systems, 6.3.4. research evaluation for studies that used novel approaches, 6.3.5. research evaluation for the study that used similarity-based filtering, 7. discussion of findings, 8. gaps, challenges, future directions and conclusions for (crs) selection, 8.2. challenges, 8.3. future directions, 9. conclusions.
- Making precise course recommendations that are tailored to each student’s interests, abilities, and long-term professional goals.
- Addressing the issue of “cold starts,” wherein brand-new students without prior course experience might not obtain useful, reliable, and precise advice.
- Ensuring that the system is flexible enough to accommodate various educational contexts, data accessibility, and the unique objectives of the advising system.
- Increasing suggestion recall and precision rates.
- Using preprocessing and data-splitting methods to enhance the predefined performance standards of the CRS overall as well as the predefined and measured quality of recommendations.
Author Contributions
Data availability statement, conflicts of interest.
- Iatrellis, O.; Kameas, A.; Fitsilis, P. Academic advising systems: A systematic literature review of empirical evidence. Educ. Sci. 2017 , 7 , 90. [ Google Scholar ] [ CrossRef ] [ Green Version ]
- Chang, P.C.; Lin, C.H.; Chen, M.H. A hybrid course recommendation system by integrating collaborative filtering and artificial immune systems. Algorithms 2016 , 9 , 47. [ Google Scholar ] [ CrossRef ] [ Green Version ]
- Xu, J.; Xing, T.; Van Der Schaar, M. Personalized course sequence recommendations. IEEE Trans. Signal Process. 2016 , 64 , 5340–5352. [ Google Scholar ] [ CrossRef ] [ Green Version ]
- Noaman, A.Y.; Ahmed, F.F. A new framework for e academic advising. Procedia Comput. Sci. 2015 , 65 , 358–367. [ Google Scholar ] [ CrossRef ] [ Green Version ]
- Pizzolato, J.E. Complex partnerships: Self-authorship and provocative academic-advising practices. NACADA J. 2006 , 26 , 32–45. [ Google Scholar ] [ CrossRef ] [ Green Version ]
- Unelsrød, H.F. Design and Evaluation of a Recommender System for Course Selection. Master’s Thesis, Institutt for Datateknikk og Informasjonsvitenskap, Trondheim, Norway, 2011. [ Google Scholar ]
- Kuh, G.D.; Kinzie, J.; Schuh, J.H.; Whitt, E.J. Student Success in College: Creating Conditions That Matter ; John Wiley & Sons: New York, NY, USA, 2011. [ Google Scholar ]
- Mostafa, L.; Oately, G.; Khalifa, N.; Rabie, W. A case based reasoning system for academic advising in Egyptian educational institutions. In Proceedings of the 2nd International Conference on Research in Science, Engineering and Technology (ICRSET’2014), Dubai, United Arab Emirates, 21–22 March 2014; pp. 21–22. [ Google Scholar ]
- Obeidat, R.; Duwairi, R.; Al-Aiad, A. A collaborative recommendation system for online courses recommendations. In Proceedings of the 2019 International Conference on Deep Learning and Machine Learning in Emerging Applications (Deep-ML), Istanbul, Turkey, 26–28 August 2019; pp. 49–54. [ Google Scholar ]
- Feng, J.; Xia, Z.; Feng, X.; Peng, J. RBPR: A hybrid model for the new user cold start problem in recommender systems. Knowl.-Based Syst. 2021 , 214 , 106732. [ Google Scholar ] [ CrossRef ]
- Kohl, C.; McIntosh, E.J.; Unger, S.; Haddaway, N.R.; Kecke, S.; Schiemann, J.; Wilhelm, R. Online tools supporting the conduct and reporting of systematic reviews and systematic maps: A case study on CADIMA and review of existing tools. Environ. Evid. 2018 , 7 , 8. [ Google Scholar ] [ CrossRef ] [ Green Version ]
- Shminan, A.S.; Choi, L.J.; Barawi, M.H.; Hashim, W.N.W.; Andy, H. InVesa 1.0: The Conceptual Framework of Interactive Virtual Academic Advisor System based on Psychological Profiles. In Proceedings of the 2021 13th International Conference on Information & Communication Technology and System (ICTS), Surabaya, Indonesia, 20–21 October 2021; pp. 112–117. [ Google Scholar ]
- Wang, H.; Wei, Z. Research on Personalized Learning Route Model Based on Improved Collaborative Filtering Algorithm. In Proceedings of the 2021 2nd International Conference on Big Data & Artificial Intelligence & Software Engineering (ICBASE), Zhuhai, China, 24–26 September 2021; pp. 120–123. [ Google Scholar ]
- Shaptala, R.; Kyselova, A.; Kyselov, G. Exploring the vector space model for online courses. In Proceedings of the 2017 IEEE First Ukraine Conference on Electrical and Computer Engineering (UKRCON), Kyiv, Ukraine, 29 May–2 June 2017; pp. 861–864. [ Google Scholar ]
- Zhao, X.; Liu, B. Application of personalized recommendation technology in MOOC system. In Proceedings of the 2020 International Conference on Intelligent Transportation, Big Data & Smart City (ICITBS), Vientiane, Laos, 11–12 January 2020; pp. 720–723. [ Google Scholar ]
- Wahyono, I.D.; Asfani, K.; Mohamad, M.M.; Saryono, D.; Putranto, H.; Haruzuan, M.N. Matching User in Online Learning using Artificial Intelligence for Recommendation of Competition. In Proceedings of the 2021 Fourth International Conference on Vocational Education and Electrical Engineering (ICVEE), Surabaya, Indonesia, 2–3 October 2021; pp. 1–4. [ Google Scholar ]
- Elghomary, K.; Bouzidi, D. Dynamic peer recommendation system based on trust model for sustainable social tutoring in MOOCs. In Proceedings of the 2019 1st International Conference on Smart Systems and Data Science (ICSSD), Rabat, Morocco, 3–4 October 2019; pp. 1–9. [ Google Scholar ]
- Mufizar, T.; Mulyani, E.D.S.; Wiyono, R.A.; Arifiana, W. A combination of Multi Factor Evaluation Process (MFEP) and the Distance to the Ideal Alternative (DIA) methods for majors selection and scholarship recipients in SMAN 2 Tasikmalaya. In Proceedings of the 2018 6th International Conference on Cyber and IT Service Management (CITSM), Parapat, Indonesia, 7–9 August 2018; pp. 1–7. [ Google Scholar ]
- Sutrisno, M.; Budiyanto, U. Intelligent System for Recommending Study Level in English Language Course Using CBR Method. In Proceedings of the 2019 6th International Conference on Electrical Engineering, Computer Science and Informatics (EECSI), Bandung, Indonesia, 18–20 September 2019; pp. 153–158. [ Google Scholar ]
- Gan, B.; Zhang, C. Research on the Application of Curriculum Knowledge Point Recommendation Algorithm Based on Learning Diagnosis Model. In Proceedings of the 2020 5th International Conference on Electromechanical Control Technology and Transportation (ICECTT), Nanchang, China, 5–17 May 2020; pp. 188–192. [ Google Scholar ]
- Ivanov, D.A.; Ivanova, I.V. Computer Self-Testing of Students as an Element of Distance Learning Technologies that Increase Interest in the Study of General Physics Course. In Proceedings of the 2018 IV International Conference on Information Technologies in Engineering Education (Inforino), Moscow, Russia, 22–26 October 2018; pp. 1–4. [ Google Scholar ]
- Anupama, V.; Elayidom, M.S. Course Recommendation System: Collaborative Filtering, Machine Learning and Topic Modelling. In Proceedings of the 2022 8th International Conference on Advanced Computing and Communication Systems (ICACCS), Coimbatore, India, 25–26 March 2022; Volume 1, pp. 1459–1462. [ Google Scholar ]
- Sabnis, V.; Tejaswini, P.D.; Sharvani, G.S. Course recommendations in moocs: Techniques and evaluation. In Proceedings of the 2018 3rd International Conference on Computational Systems and Information Technology for Sustainable Solutions (CSITSS), Bengaluru, India, 20–22 December 2018; pp. 59–66. [ Google Scholar ]
- Britto, J.; Prabhu, S.; Gawali, A.; Jadhav, Y. A Machine Learning Based Approach for Recommending Courses at Graduate Level. In Proceedings of the 2019 International Conference on Smart Systems and Inventive Technology (ICSSIT), Tirunelveli, India, 27–29 November 2019; pp. 117–121. [ Google Scholar ]
- Peng, Y. A Survey on Modern Recommendation System based on Big Data. arXiv 2022 , arXiv:2206.02631. [ Google Scholar ]
- Bozyiğit, A.; Bozyiğit, F.; Kilinç, D.; Nasiboğlu, E. Collaborative filtering based course recommender using OWA operators. In Proceedings of the 2018 International Symposium on Computers in Education (SIIE), Jerez, Spain, 19–21 September 2018; pp. 1–5. [ Google Scholar ]
- Mondal, B.; Patra, O.; Mishra, S.; Patra, P. A course recommendation system based on grades. In Proceedings of the 2020 International Conference on Computer Science, Engineering and Applications (ICCSEA), Gunupur, India, 13–14 March 2020; pp. 1–5. [ Google Scholar ]
- Lee, E.L.; Kuo, T.T.; Lin, S.D. A collaborative filtering-based two stage model with item dependency for course recommendation. In Proceedings of the 2017 IEEE International Conference on Data Science and Advanced Analytics (DSAA), Tokyo, Japan, 19–21 October 2017; pp. 496–503. [ Google Scholar ]
- Malhotra, I.; Chandra, P.; Lavanya, R. Course Recommendation using Domain-based Cluster Knowledge and Matrix Factorization. In Proceedings of the 2022 9th International Conference on Computing for Sustainable Global Development (INDIACom), New Delhi, Indi, 23–25 March 2022; pp. 12–18. [ Google Scholar ]
- Huang, L.; Wang, C.D.; Chao, H.Y.; Lai, J.H.; Philip, S.Y. A score prediction approach for optional course recommendation via cross-user-domain collaborative filtering. IEEE Access 2019 , 7 , 19550–19563. [ Google Scholar ] [ CrossRef ]
- Zhao, L.; Pan, Z. Research on online course recommendation model based on improved collaborative filtering algorithm. In Proceedings of the 2021 IEEE 6th International Conference on Cloud Computing and Big Data Analytics (ICCCBDA), Chengdu, China, 24–26 April 2021; pp. 437–440. [ Google Scholar ]
- Ceyhan, M.; Okyay, S.; Kartal, Y.; Adar, N. The Prediction of Student Grades Using Collaborative Filtering in a Course Recommender System. In Proceedings of the 2021 5th International Symposium on Multidisciplinary Studies and Innovative Technologies (ISMSIT), Ankara, Turkey, 21–23 October 2021; pp. 177–181. [ Google Scholar ]
- Dwivedi, S.; Roshni, V.K. Recommender system for big data in education. In Proceedings of the 2017 5th National Conference on E-Learning & E-Learning Technologies (ELELTECH), Hyderabad, India, 3–4 August 2017; pp. 1–4. [ Google Scholar ]
- Zhong, S.T.; Huang, L.; Wang, C.D.; Lai, J.H. Constrained matrix factorization for course score prediction. In Proceedings of the 2019 IEEE International Conference on Data Mining (ICDM), Beijing, China, 8–11 November 2019; pp. 1510–1515. [ Google Scholar ]
- Chen, Z.; Song, W.; Liu, L. The application of association rules and interestingness in course selection system. In Proceedings of the 2017 IEEE 2nd International Conference on Big Data Analysis (ICBDA), Beijing, China, 10–12 March 2017; pp. 612–616. [ Google Scholar ]
- Chen, Z.; Liu, X.; Shang, L. Improved course recommendation algorithm based on collaborative filtering. In Proceedings of the 2020 International Conference on Big Data and Informatization Education (ICBDIE), Zhangjiajie, China, 23–25 April 2020; pp. 466–469. [ Google Scholar ]
- Ren, Z.; Ning, X.; Lan, A.S.; Rangwala, H. Grade prediction with neural collaborative filtering. In Proceedings of the 2019 IEEE International Conference on Data Science and Advanced Analytics (DSAA), Washington, DC, USA, 5–8 October 2019; pp. 1–10. [ Google Scholar ]
- Fernández-García, A.J.; Rodríguez-Echeverría, R.; Preciado, J.C.; Manzano, J.M.C.; Sánchez-Figueroa, F. Creating a recommender system to support higher education students in the subject enrollment decision. IEEE Access 2020 , 8 , 189069–189088. [ Google Scholar ] [ CrossRef ]
- Adilaksa, Y.; Musdholifah, A. Recommendation System for Elective Courses using Content-based Filtering and Weighted Cosine Similarity. In Proceedings of the 2021 4th International Seminar on Research of Information Technology and Intelligent Systems (ISRITI), Yogyakarta, Indonesia, 16–17 December 2021; pp. 51–55. [ Google Scholar ]
- Esteban, A.; Zafra, A.; Romero, C. Helping university students to choose elective courses by using a hybrid multi-criteria recommendation system with genetic optimization. Knowl.-Based Syst. 2020 , 194 , 105385. [ Google Scholar ] [ CrossRef ]
- Emon, M.I.; Shahiduzzaman, M.; Rakib, M.R.H.; Shathee, M.S.A.; Saha, S.; Kamran, M.N.; Fahim, J.H. Profile Based Course Recommendation System Using Association Rule Mining and Collaborative Filtering. In Proceedings of the 2021 International Conference on Science & Contemporary Technologies (ICSCT), Dhaka, Bangladesh, 5–7 August 2021; pp. 1–5. [ Google Scholar ]
- Alghamdi, S.; Sheta, O.; Adrees, M. A Framework of Prompting Intelligent System for Academic Advising Using Recommendation System Based on Association Rules. In Proceedings of the 2022 9th International Conference on Electrical and Electronics Engineering (ICEEE), Alanya, Turkey, 29–31 March 2022; pp. 392–398. [ Google Scholar ]
- Bharath, G.M.; Indumathy, M. Course Recommendation System in Social Learning Network (SLN) Using Hybrid Filtering. In Proceedings of the 2021 5th International Conference on Electronics, Communication and Aerospace Technology (ICECA), Coimbatore, India, 2–4 December 2021; pp. 1078–1083. [ Google Scholar ]
- Nafea, S.M.; Siewe, F.; He, Y. On recommendation of learning objects using felder-silverman learning style model. IEEE Access 2019 , 7 , 163034–163048. [ Google Scholar ] [ CrossRef ]
- Huang, X.; Tang, Y.; Qu, R.; Li, C.; Yuan, C.; Sun, S.; Xu, B. Course recommendation model in academic social networks based on association rules and multi-similarity. In Proceedings of the 2018 IEEE 22nd International Conference on Computer Supported Cooperative Work in Design (CSCWD), Nanjing, China, 9–11 May 2018; pp. 277–282. [ Google Scholar ]
- Baskota, A.; Ng, Y.K. A graduate school recommendation system using the multi-class support vector machine and KNN approaches. In Proceedings of the 2018 IEEE International Conference on Information Reuse and Integration (IRI), Salt Lake City, UT, USA, 6–9 July 2018; pp. 277–284. [ Google Scholar ]
- Jiang, W.; Pardos, Z.A.; Wei, Q. Goal-based course recommendation. In Proceedings of the 9th International Conference on Learning Analytics & Knowledge, Tempe, AZ, USA, 4–8 March 2019; pp. 36–45. [ Google Scholar ]
- Liang, Y.; Duan, X.; Ding, Y.; Kou, X.; Huang, J. Data Mining of Students’ Course Selection Based on Currency Rules and Decision Tree. In Proceedings of the 2019 4th International Conference on Big Data and Computing, Guangzhou, China, 10–12 May 2019; pp. 247–252. [ Google Scholar ]
- Isma’il, M.; Haruna, U.; Aliyu, G.; Abdulmumin, I.; Adamu, S. An autonomous courses recommender system for undergraduate using machine learning techniques. In Proceedings of the 2020 International Conference in Mathematics, Computer Engineering and Computer Science (ICMCECS), Ayobo, Nigeria, 18–21 March 2020; pp. 1–6. [ Google Scholar ]
- Revathy, M.; Kamalakkannan, S.; Kavitha, P. Machine Learning based Prediction of Dropout Students from the Education University using SMOTE. In Proceedings of the 2022 4th International Conference on Smart Systems and Inventive Technology (ICSSIT), Tirunelveli, India, 20–22 January 2022; pp. 1750–1758. [ Google Scholar ]
- Oreshin, S.; Filchenkov, A.; Petrusha, P.; Krasheninnikov, E.; Panfilov, A.; Glukhov, I.; Kaliberda, Y.; Masalskiy, D.; Serdyukov, A.; Kazakovtsev, V.; et al. Implementing a Machine Learning Approach to Predicting Students’ Academic Outcomes. In Proceedings of the 2020 International Conference on Control, Robotics and Intelligent System, Xiamen, China, 27–29 October 2020; pp. 78–83. [ Google Scholar ]
- Verma, R. Applying Predictive Analytics in Elective Course Recommender System while preserving Student Course Preferences. In Proceedings of the 2018 IEEE 6th International Conference on MOOCs, Innovation and Technology in Education (MITE), Hyderabad, India, 29–30 November 2018; pp. 52–59. [ Google Scholar ]
- Bujang, S.D.A.; Selamat, A.; Ibrahim, R.; Krejcar, O.; Herrera-Viedma, E.; Fujita, H.; Ghani, N.A.M. Multiclass prediction model for student grade prediction using machine learning. IEEE Access 2021 , 9 , 95608–95621. [ Google Scholar ] [ CrossRef ]
- Srivastava, S.; Karigar, S.; Khanna, R.; Agarwal, R. Educational data mining: Classifier comparison for the course selection process. In Proceedings of the 2018 International Conference on Smart Computing and Electronic Enterprise (ICSCEE), Shah Alam, Malaysia, 11–12 July 2018; pp. 1–5. [ Google Scholar ]
- Abed, T.; Ajoodha, R.; Jadhav, A. A prediction model to improve student placement at a south african higher education institution. In Proceedings of the 2020 International SAUPEC/RobMech/PRASA Conference, Cape Town, South Africa, 29–31 January 2020; pp. 1–6. [ Google Scholar ]
- Uskov, V.L.; Bakken, J.P.; Byerly, A.; Shah, A. Machine learning-based predictive analytics of student academic performance in STEM education. In Proceedings of the 2019 IEEE Global Engineering Education Conference (EDUCON), Dubai, United Arab Emirates, 8–11 April 2019; pp. 1370–1376. [ Google Scholar ]
- Sankhe, V.; Shah, J.; Paranjape, T.; Shankarmani, R. Skill Based Course Recommendation System. In Proceedings of the 2020 IEEE International Conference on Computing, Power and Communication Technologies (GUCON), Greater Noida, India, 2–4 October 2020; pp. 573–576. [ Google Scholar ]
- Kamila, V.Z.; Subastian, E. KNN and Naive Bayes for Optional Advanced Courses Recommendation. In Proceedings of the 2019 International Conference on Electrical, Electronics and Information Engineering (ICEEIE), Denpasar, Indonesia, 3–4 October 2019; Volume 6, pp. 306–309. [ Google Scholar ]
- Shah, D.; Shah, P.; Banerjee, A. Similarity based regularization for online matrix-factorization problem: An application to course recommender systems. In Proceedings of the TENCON 2017—2017 IEEE Region 10 Conference, Penang, Malaysia, 5–8 November 2017; pp. 1874–1879. [ Google Scholar ]
Click here to enlarge figure
Author | The Study Addresses Recommendation Systems in the Education Sector | Primary Study |
---|---|---|
Shminan et al. [ ] | No | Yes |
Wang et al. [ ] | No | Yes |
Shaptala et al. [ ] | No | Yes |
Zhao et al. [ ] | No | Yes |
ID Wahyono et al. [ ] | No | Yes |
Author | The Study Addresses Recommendation Systems in the Education Sector | Primary Study |
---|---|---|
Elghomary et al. [ ] | No | Yes |
Mufizar et al. [ ] | No | Yes |
Sutrisno et al. [ ] | No | Yes |
Gan et al. [ ] | No | Yes |
Ivanov et al. [ ] | No | Yes |
Author | Reason of Exclusion |
---|---|
Anupama et al. [ ] | Did not imply empirical experiments and did not provide experiments results |
Sabnis et al. [ ] | The full text is not accessible |
Author | Score | Total Score | Included | ||||
---|---|---|---|---|---|---|---|
Q1 | Q2 | Q3 | Q4 | Q5 | |||
Britto et al. [ ] | 1 | 0 | 0.5 | 0.5 | 0.5 | 2.5 | No |
Obeidat et al. [ ] | 0.5 | 1 | 0.5 | 0.5 | 0.5 | 3 | Yes |
Authors and Year | Algorithms Used | Comparative Type |
---|---|---|
A. Bozyiğit et al., 2018 [ ] | • Collaborative filtering; | Algorithm level |
• OWA (ordered weighted average). | ||
B. Mondal et al., 2020 [ ] | • Collaborative filtering. | Algorithm level |
E. L. Lee et al., 2017 [ ] | • Two-stage collaborative filtering; | Algorithm level |
• Personalized Ranking Matrix Factorization (BPR-MF); | ||
• Course dependency regularization; | ||
• Personalized PageRank; | ||
• Linear RankSVM. | ||
I. Malhotra et al., 2022 [ ] | • Collaborative filtering; | Algorithm level |
• Domain-based cluster knowledge; | ||
• Cosine pairwise similarity evaluation; | ||
• Singular value decomposition ++; | ||
• Matrix factorization. | ||
L. Huang et al., 2019 [ ] | • Cross-user-domain collaborative filtering. | Algorithm level |
L. Zhao et al., 2021 [ ] | • Improved collaborative filtering; | Algorithm level |
• Historical preference fusion similarity. | ||
M. Ceyhan et al., 2021 [ ] | • Collaborative filtering; | Algorithm level |
• Correlation-based similarities: (Pearson correlation coefficient, median-based robust correlation coefficient); | ||
• Distance-based similarities: Manhattan and Euclidian distance similarities. | ||
R. Obeidat et al., 2019 [ ] | • Collaborative filtering; | Algorithm level |
• K-means clustering; | ||
• Association rules (Apriori algorithm, sequential, pattern discovery using equivalence classes algorithm). | ||
S. Dwivedi et al., 2017 [ ] | • Collaborative filtering; | Algorithm level |
• Similarity log-likelihood. | ||
S.-T. Zhong et al., 2019 [ ] | • Collaborative filtering; | Algorithm level |
• Constrained matrix factorization. | ||
Z. Chen et al., 2017 [ ] | • Collaborative filtering; | Algorithm level |
• Association rules (Apriori). | ||
Z. Chen et al., 2020 [ ] | • Collaborative filtering; | Algorithm level |
• Improved cosine similarity; | ||
• TF–IDF (term frequency–inverse document frequency). | ||
Z. Ren et al., 2019 [ ] | • Neural Collaborative Filtering (NCF). | Algorithm level |
Authors and Year | Used Algorithms | Comparative Type |
---|---|---|
A. J. Fernández-García et al., 2020 [ ] | • Content-based filtering. | Preprocessing level |
Y. Adilaksa et al., 2021 [ ] | • Content-based filtering; | Algorithm level |
• Weighted cosine similarity; • TF–IDF. |
Authors and Year | Used Algorithms | Comparative Type |
---|---|---|
Esteban, A. et al., 2020 [ ] | • Hybrid recommender system; | Algorithm level |
• Collaborative filtering; | ||
• Content-based filtering; | ||
• Genetic algorithm. | ||
M. I. Emon et al., 2021 [ ] | • Hybrid recommender system; | Algorithm level |
• Collaborative filtering; | ||
• Association rules (Apriori algorithm). | ||
S. Alghamdi et al., 2022 [ ] | • Hybrid recommender system; | Algorithm level |
• Content-based filtering; | ||
• Association rules (Apriori algorithm); | ||
• Jaccard coefficient. | ||
S. G. G et al., 2021 [ ] | • Hybrid recommender system; | Algorithm level |
• Collaborative filtering; | ||
• Content-based filtering; | ||
• Lasso; | ||
• KNN; | ||
• Weighted average. | ||
S. M. Nafea et al., 2019 [ ] | • Hybrid recommender system; | Algorithm level |
• Felder–Silverman learning styles model; | ||
• K-means clustering. | ||
X. Huang et al., 2018 [ ] | • Hybrid recommender system; | Algorithm level |
• Association rules; | ||
• Improved multi-similarity. |
Authors and Year | Used Algorithms | Comparative Type |
---|---|---|
A. Baskota et al., 2018 [ ] | • Forward feature selection; | Algorithm level |
• K-Nearest Neighbor (KNN); | ||
• Multi-class Support Vector Machines (MC-SVM). | ||
Jiang, Weijie et al., 2019 [ ] | • Goal-based filtering; | Algorithm level |
• LSTM recurrent neural network. | ||
Liang, Yu et al., 2019 [ ] | • Currency rules; | Preprocessing level |
• C4.5 decision tree. | ||
M. Isma’il et al., 2020 [ ] | • Support Vector Machine (SVM). | Algorithm level |
M. Revathy et al., 2022 [ ] | • KNN-SMOTE. | Algorithm level |
Oreshin et al., 2020 [ ] | • Latent Dirichlet Allocation; | Algorithm level |
• FastTextSocialNetworkModel; | ||
• Catboost. | ||
R. Verma et al., 2018 [ ] | • Support Vector Machines; | Algorithm level |
• Artificial Neural Networks (ANN). | ||
S. D. A. Bujang et al., 2021 [ ] | • Random forests. | • Algorithm level |
• Preprocessing level | ||
S. Srivastava et al., 2018 [ ] | • Support Vector Machines with radial basis kernel; | Algorithm level |
• KNN. | ||
T. Abed et al., 2020 [ ] | • Naive Bayes. | Algorithm level |
V. L. Uskov et al., 2019 [ ] | • Linear regression. | Algorithm level |
V. Sankhe et al., 2020 [ ] | • Skill-based filtering; | Algorithm level |
• C-means fuzzy clustering; | ||
• Weighted mode. | ||
V. Z. Kamila et al., 2019 [ ] | • KNN; | algorithm level |
• Naive Bayes. |
Authors and Year | Used Algorithms | Comparative Type |
---|---|---|
D. Shah et al., 2017 [ ] | • Similarity-based regularization; | Algorithm level |
• Matrix factorization. |
Authors and Year | Public | Records | Students | Courses | Majors | Features | Used Features | Preprocessing Steps | Data-Splitting Method |
---|---|---|---|---|---|---|---|---|---|
A. Bozyiğit et al., 2018 [ ] | No | N/A | 221 | 76 | N/A | N/A | N/A | N/A | Ten-fold cross-validation. |
B. Mondal et al., 2020 [ ] | No | 300 | 300 | N/A | N/A | 48 | 12 | • Data cleaning: lowercase conversion, removing punctuation, striping white spaces. | N/A |
E. L. Lee et al., 2017 [ ] | No | 896,616 | 13,977 | N/A | N/A | N/A | N/A | • Ignore the students whose 4-year registration records are incomplete. | Nested time-series split cross-validation (class 2008, class 2009 as a training set, and class 2010 as a testing set). |
I. Malhotra et al., 2022 [ ] | No | N/A | 1780 | N/A | 9 | N/A | N/A | N/A | N/A |
L. Huang et al., 2019 [ ] | No | 52,311 | 1166 | N/A | 8 | N/A | N/A | N/A | N/A |
L. Zhao et al., 2021 [ ] | No | N/A | 43,916 | 240 | N/A | N/A | N/A | • Group data based on interest data points, • Eliminate noise by filtering the data noise constrained in 0,1, • Normalize all numerical features. | Five-fold cross-validation. |
M. Ceyhan et al., 2021 [ ] | No | N/A | 1506 | 1460 | N/A | N/A | N/A | • The updated grade is taken into consideration if a student retakes any course. | • Nested time-series split cross-validation, • Train = 91.7% (from 2010/11-F to 2019/20-S), • Test = 8.3% (the whole 2020/21-F). |
R. Obeidat et al., 2019 [ ] | Yes | 22,144 | 10,000 | 16 | N/A | N/A | N/A | • Remove incomplete records • Calculate the order of courses sequences events for each student, • Convert grades to a new grade scale, • Cluster students. | N/A |
S. Dwivedi et al., 2017 [ ] | No | N/A | N/A | N/A | N/A | N/A | N/A | • Data cleaning, • Data discretization (converting low-level concept to high-level concept). | N/A |
S. -T. Zhong et al., 2019 [ ] | No | N/A | N/A | N/A | 8 | N/A | N/A | N/A | N/A |
Z. Chen et al., 2017 [ ] | No | N/A | N/A | N/A | N/A | N/A | N/A | Students’ score categorization (A, B, C). | N/A |
Z. Chen et al., 2020 [ ] | No | 18,457 | 2022 | 309 | N/A | N/A | N/A | N/A | K-fold cross-validation. |
Z. Ren et al., 2019 [ ] | No | N/A | 43,099 | N/A | 151 | N/A | N/A | Used different embedding dimensions for students, courses, and course instructors for different majors. | Nested time-series split cross-validation (data from Fall 2009 to Fall 2015 as a training set, and data from Spring 2016 as a testing set). |
Authors and Year | Public | Records | Students | Courses | Majors | Features | Features Used | Preprocessing Steps | Data-Splitting Method |
---|---|---|---|---|---|---|---|---|---|
A. J. Fernández-García et al., 2020 [ ] | No | 6948 | 323 | N/A | N/A | 10 | 10 | • Feature deletion, • Class reduction, • One-hot encoding, • Creating new features, • Data scaling: MinMax Scaler, Standard Scaler, Robust Scaler, and Normalizer Scaler, • Data resampling: upsample, downsample, SMOTE. | • Train size = 80%, • Test size = 20%. |
Y. Adilaksa et al., 2021 [ ] | No | N/A | N/A | N/A | N/A | N/A | N/A | • Case folding, • Word tokenization, • Punctuation removal, • Stop words removal. | N/A |
Authors and Year | Pub | Recs | Students | Courses | Majors | Features | Used Features | Preprocessing Steps | Data-Splitting Method |
---|---|---|---|---|---|---|---|---|---|
Esteban, A. et al., 2020 [ ] | No | 2500C | 95 | 63 | N/A | N/A | N/A | N/A | Five-fold cross-validation. |
M. I. Emon et al., 2021 [ ] | No | N/A | 250+ | 250+ | 20+ | N/A | N/A | Feature extraction. | N/A |
S. Alghamdi et al., 2022 [ ] | No | 1820 | 38 | 48 | N/A | N/A | 7 | Cluster sets for academic transcript datasets. | Five-fold cross-validation. |
S. G. G et al., 2021 [ ] | No | N/A | ~6000 | ~4000 | 18 | N/A | N/A | N/A | N/A |
S. M. Nafea et al., 2019 [ ] | No | N/A | 80 | N/A | N/A | N/A | N/A | N/A | Student dataset was split into cold-start students, cold-start learning objects, and all students. |
X. Huang et al., 2018 [ ] | Yes | N/A | 56,600 | 860 | N/A | N/A | N/A | N/A | • Train size = 80%, • Test size = 20%. |
Authors and Year | Public | Records | Students | Courses | Majors | Features | Features Used | Preprocessing Steps | Data-Splitting Method |
---|---|---|---|---|---|---|---|---|---|
A. Baskota et al., 2018 [ ] | No | 16,000 | N/A | N/A | N/A | N/A | N/A | • Data cleaning, • Data scaling. | • Train size = 14,000, • Test size = 2000. |
Jiang, Weijie et al., 2019 [ ] | No | 4,800,000 | 164,196 | 10,430 | 17 | N/A | N/A | N/A | Nested time-series split cross-validation (data from F’08 to F’15 as a training set, data in Sp’16 as validation set & data in Sp’17 as test set) |
Liang, Yu et al., 2019 [ ] | No | 35,000 | N/A | N/A | N/A | N/A | N/A | Data cleaning. | N/A |
M. Isma’il et al., 2020 [ ] | No | 8700 | N/A | 9 | N/A | N/A | 4 | • Data cleaning, • Data encoding. | N/A |
M. Revathy et al., 2022 [ ] | No | N/A | 1243 | N/A | N/A | N/A | 33 | • One-hot encoding for categorical features, • Principal Component Analysis (PCA). | • Train size = 804, • Test size = 359. |
Oreshin et al., 2020 [ ] | No | N/A | >20,000 | N/A | N/A | N/A | 112 | • One-hot encoding, • Removed samples with unknown values. | Nested time-series split cross-validation. |
R. Verma et al., 2018 [ ] | No | 658 | 658 | N/A | N/A | 13 | 11 | Data categorization. | Ten-fold cross-validation. |
S. D. A. Bujang et al., 2021 [ ] | No | 1282 | 641 | 2 | N/A | 13 | N/A | • Ranked and grouped the students into five categories of grades, • Applied oversampling SMOTE (Synthetic Minority Over-sampling Technique), • Applied two feature selection methods: Wrapper and filter-based. | Ten-fold cross-validation. |
S. Srivastava et al., 2018 [ ] | No | 1988 | 2890 | N/A | N/A | N/A | 14 | Registration number transformation. | • Train = 1312, • Test = 676. |
T. Abed et al., 2020 [ ] | No | N/A | N/A | N/A | N/A | N/A | 18 | Balanced the dataset using under sampling. | Ten-fold cross-validation. |
V. L. Uskov et al., 2019 [ ] | No | 90+ | N/A | N/A | N/A | 16 | N/A | Data cleaning | • Train = 80%, • Test = 20%. |
V. Sankhe et al., 2020 [ ] | No | N/A | 2000 | 15 | 7 | N/A | N/A | N/A | N/A |
V. Z. Kamila et al., 2019 [ ] | No | N/A | N/A | N/A | N/A | N/A | N/A | N/A | • Train size = 75%, • Test size= 25%. |
Authors and Year | Public | Records | Students | Courses | Majors | Features | Features Used | Preprocessing Steps | Data-Splitting Method |
---|---|---|---|---|---|---|---|---|---|
D. Shah et al., 2017 [ ] | No | N/A | • Dataset 1 = 300 students • Dataset 2 = 84 students | • Dataset 1 = 10 • Dataset 2 = 26 | N/A | N/A | • Student features = 3 • Course features = 30 | N/A | • Train size = 90% • Test size = 10% |
Authors and Year | Evaluation Metrics and Values | Strengths | Weaknesses |
---|---|---|---|
A. Bozyiğit et al., 2018 [ ] | MAE = 0.063. | • Compared the performance of the proposed OWA approach with the performance of other popular approaches. | • The number of features and features used in the dataset is not provided, • The dataset description is not detailed, • Did not use RMSE for evaluation, considered the standard as it’s more accurate, • Mentioned that some preprocessing had been carried out but did not give any details regarding it. |
B. Mondal et al., 2020 [ ] | • MSE = 3.609, • MAE = 1.133, • RMSE = 1.8998089, • Precision, • Recall. | • Used many metrics for evaluation, • The implementation of algorithms is comprehensively explained. | • Did not mention whether they split data for testing or used the training data for testing, • Did not provide the exact measures of precision and recall. |
E. L. Lee et al., 2017 [ ] | AUC = 0.9709. | • Compared the performance of the proposed approach with the performance of other approaches, • Used a very large dataset, • Achieved a very high AUC, • The implementation of algorithms is comprehensively explained. | • Did not provide the percentage of the train-test split, • The number of courses in the dataset is not mentioned (it only mentions course registration records). |
I. Malhotra et al., 2022 [ ] | • MAE = 0.468, • RMSE = 0.781. | • The implementation of algorithms is comprehensively explained with examples, • Used RMSE and MAE for evaluation. | • The dataset description is not detailed, • The method of splitting the training and testing dataset is not provided, • Did not mention whether they have done any preprocessing on the dataset or if it was used as it is, • The proposed approach is not compared to any other approaches in the evaluation section. |
L. Huang et al., 2019 [ ] | • AverHitRate between 0.6538, 1, • AverACC between 0.8347, 1. | • The literature is meticulously discussed, • The implementation is comprehensively explained in detail. | • The method of splitting the training and testing dataset is not provided, • Did not mention whether they have conducted any preprocessing on the dataset or if it was used as it is. |
L. Zhao et al., 2021 [ ] | • Precision, • Recall. | • The implementation is comprehensively explained. | • The exact numbers for the evaluation metrics used in the paper are not provided, • The numbers of features and features used in the dataset are not provided. |
M. Ceyhan et al., 2021 [ ] | • Coverage, • F1-measure, • Precision, • Sensitivity, • Specificity, • MAE, • RMSE, • Binary MAE, • Binary RMSE. | • Used many metrics for evaluation. | • The implemented algorithm and similarities explanation were very brief |
R. Obeidat et al., 2019 [ ] | • Coverage measure (using SPADES | with clustering) = 0.376, 0.28, 0.594, 0.546, • Coverage measure (using Apriori | with clustering) = 0.46, 0.348, 0.582, 0.534. | • Confirmed by experiment that clustering significantly improves the generation and coverage of two association rules: SPADES and Apriori | • The dataset description is not detailed, • The method of splitting the training and testing dataset is not provided, • The implementation is not discussed in detail. |
S. Dwivedi et al., 2017 [ ] | • RMSE = 0.46. | • The proposed system is efficient as it proved to work well with big data, • The implementation of algorithms is comprehensively explained. | • Did not provide any information about the dataset, • The literature review section was very brief. |
S.-T. Zhong et al., 2019 [ ] | • MAE (CS major) = 6.6764 ± 0.0029, • RMSE (CS major) = 4.5320 ± 0.0022. | • Used eight datasets for model training and evaluation, • Dataset description is detailed, • Compared the performance of the proposed approach with the performance of other popular approaches. | • The percentage of train-test splitting is not consistent among the eight datasets. |
Z. Chen et al., 2017 [ ] | • Confidence, • Support. | • The implementation of algorithms is comprehensively explained with examples. | • Did not provide any information about the used dataset, • Did not include any information about the preprocessing of the dataset, • Did not provide useful metrics for evaluation, • The performance of the proposed approach is not compared to other similar approaches. |
Z. Chen et al., 2020 [ ] | • Precision, • Recall, • F1-score. | • Compared the performance of the proposed approach with the performance of other popular approaches: cosine similarity and improved cosine similarity. | • The exact numbers for the evaluation metrics used in the paper are not provided, • The numbers of features and features used in the dataset are not provided. |
Z. Ren et al., 2019 [ ] | • PTA, • MAE. | • Compared the performance of the proposed approach with the performance of other approaches, • The implementation of algorithms is comprehensively explained, • The number of students in the dataset is big. | • The dataset description is not detailed. |
Authors and Year | Evaluation Metrics and Values | Strengths | Weaknesses |
---|---|---|---|
A. J. Fernández-García et al., 2020 [ ] | • Accuracy, • Precision, • Recall, • F1-score. | • Included a section that contains the implementation code, • The literature is meticulously discussed and followed by a table for a summary, • Compared the effect of various preprocessing steps on the final measures of different machine-learning approaches and provided full details about these metrics, • The implementation of each preprocessing step is explained in detail. | • N/A |
Y. Adilaksa et al., 2021 [ ] | • The percentage of recommendation diversity = 81.67%, • Accuracy = 64%. | • The preprocessing steps are discussed in detail, • The implementation is comprehensively explained, • Confirmed by the experiment that using the weighted cosine similarity instead of the traditional cosine similarity significantly increased the accuracy of the course recommendations system. | • Did not provide any information about the used dataset, • The method of splitting the training and testing dataset is not provided, • The accuracy measurement is not specified. |
Authors and Year | Evaluation Metrics and Values | Strengths | Weaknesses |
---|---|---|---|
Esteban, A. et al., 2020 [ ] | • RMSE = 0.971, • Normalized discount cumulative gain (nDCG) = 0.682, • Reach = 100%, • Time = 3.022s. | • The literature is meticulously discussed and followed by a table for a summary, • The implementation of algorithms is comprehensively explained with examples, • Compared the performance of the proposed hybrid approach with other similar approaches, • Used many useful metrics for evaluation. | • Mentioned that some preprocessing had been carried out but did not give any details regarding it, • The number of students in the dataset is relatively low. |
M. I. Emon et al., 2021 [ ] | • Accuracy, • Precision, • Recall, • F1-score. | • Compared the performance of the proposed hybrid approach with the used standalone algorithms. | • The exact numbers for the evaluation metrics used in the paper are not provided, • The dataset description is not detailed, • The method of splitting the training and testing dataset is not provided. |
S. Alghamdi et al., 2022 [ ] | • MAE = 0.772, • RMSE = 1.215. | • The dataset description is detailed, • The implementation of algorithms is clearly explained. | • Other similar approaches are not stated in the literature, • The number of students in the dataset is relatively low. |
S. G. G et al., 2021 [ ] | RMSE = 0.931. | • EDA of the dataset is included in the paper, • Compared the performance of different approaches against the proposed approach, • The implementation is comprehensively discussed and explained. | • The dataset description is not detailed, • The method of splitting the training and testing dataset is not provided, • Similar approaches are not stated in the literature, • Did not mention whether they conducted any preprocessing on the dataset or if it was used as it is. |
S. M. Nafea et al., 2019 [ ] | • MAE for cold students = 0.162, • RMSE for cold students = 0.26, • MAE for cold Learning Objects (Los) = 0.162, • RMSE for cold LOs = 0.3. | • Achieved higher accuracy than standalone traditional approaches mentioned in the paper: collaborative filtering and content-based recommendations, • The implementation is comprehensively explained with examples. | • Mentioned that some preprocessing had been carried out but did not give any details regarding it, • The dataset description is not detailed, • The number of students in the dataset is relatively low. |
X. Huang et al., 2018 [ ] | • Precision, • Recall, • F1-score. | • The implementation of the proposed approach is comprehensively explained with examples, • Compared the performance of the proposed hybrid approach with other similar approaches through testing. | • The dataset description is not detailed, • Did not mention whether they have done any preprocessing on the dataset or if it was used as it is, • The exact numbers for the evaluation metrics used in the paper are not provided. |
Authors and Year | Evaluation Metrics and Values | Strengths | Weaknesses |
---|---|---|---|
A. Baskota et al., 2018 [ ] | • Accuracy = 61.6%, • Precision = 61.2%, • Recall = 62.6%, • F1-score = 61.5%. | • Compared the performance of the proposed approach with the performance of other popular approaches, • Used many evaluation metrics and provided the exact numbers for each metric for the evaluation result. | • The dataset description is not detailed. |
Jiang, Weijie et al., 2019 [ ] | • The A model: accuracy = 75.23%, F-score = 60.24%, • The B model: accuracy = 88.05%, F-score = 42.01%. | • The implementation of algorithms is comprehensively explained with examples, • Included various sets of hyperparameters and carried out extensive testing. | • Did not mention whether they have done any preprocessing on the dataset or if it was used as it is, • Did not mention the number of features in the dataset, • The performance of the proposed approach is not compared to other similar approaches, • Did not mention the exact percentages for splitting data. |
Liang, Yu et al., 2019 [ ] | • Support rate. | • The implementation of algorithms is comprehensively explained. | • The dataset description is not detailed, • A literature review has not been discussed, • The performance of the proposed approach is not compared to other similar approaches, • Did not provide many useful metrics for evaluation and explained that was due to the large number of data sets selected for the experiment. |
M. Isma’il et al., 2020 [ ] | • Accuracy = 99.94%. | • Compared the performance of the proposed machine-learning algorithm with the performance of other algorithms through testing. | • Did not mention the training and test set sizes, • The machine learning algorithms used are not explained, • Only used the accuracy measure for evaluation, • The dataset description is not detailed. |
M. Revathy et al., 2022 [ ] | • Accuracy = 97.59%, • Precision = 97.52%, • Recall = 98.74%, • Sensitivity = 98.74%, • Specificity = 95.56%. | • Used many evaluation metrics and provided the exact numbers for each metric for the evaluation result, • Provided detailed information about the preprocessing steps, • Compared the performance of the proposed approach with the performance of other approaches, • Provided the exact numbers for each metric for the evaluation result. | N/A |
Oreshin et al., 2020 [ ] | • Accuracy = 0.91 ± 0.02, • ROC-AUC = 0.97 ± 0.01, • Recall = 0.83 ± 0.02, • Precision = 0.86 ± 0.03. | • Used many evaluation metrics and provided the exact numbers for each metric for the evaluation result, • Provided detailed information about the preprocessing steps. | • Contains many English grammar and vocabulary errors, • The dataset description is not detailed, • The machine learning algorithms used are not explained, • Did not specify the parameters for the nested time-series split cross-validation. |
R. Verma et al., 2018 [ ] | • Accuracy (SVM) = 88.5%, • Precision, • Recall, • F1-score. | • The implementation of algorithms is comprehensively explained, • Compared the performance of several machine-learning algorithms with the performance of other algorithms through testing and concluded that the best two were SVM and ANN. | • The exact numbers for the evaluation metrics used in the paper are not provided except for the achieved accuracy of SVM. |
S. D. A. Bujang et al., 2021 [ ] | • Accuracy = 99.5%, • Precision 99.5%, • Recall = 99.5%, • F1-score = 99.5%. | • Included all the exact numbers for the evaluation metrics used in the evaluation, • Compared the performance of six machine learning algorithms and concluded that random forests performed the best based on the evaluation metrics, • EDA of the dataset is included in the paper, • The literature is meticulously discussed and followed by a table for a summary, • Provided detailed information about the used dataset. | • The number of courses is very low (only 2). |
S. Srivastava et al., 2018 [ ] | • Accuracy (from 1 cluster to 100) = 99.40%:87.72%. | • Compared the performance of the proposed approach with the performance of other popular approaches, • Provided a confusion matrix for all the used approaches. | • Accuracy is the only metric used for evaluation, • The dataset description is not detailed. |
T. Abed et al., 2020 [ ] | • Accuracy = 69.18%. | • Compared the performance of the proposed approach with the performance of other popular approaches: Random Forest, J48, Naive Bayes, Logistic Regression, Sequential Minimal Optimization, and a Multilayer Perceptron. | • The dataset description is not detailed, • Only used the accuracy measure for evaluation, • Did not include an explanation for the implemented algorithms and why they were initially chosen. |
V. L. Uskov et al., 2019 [ ] | • Average error = 3.70%. | • Through extensive testing of various ML algorithms, they concluded that linear regression was the best candidate for the problem as the data was linear; • The implementation of algorithms is comprehensively explained. | • The dataset description is not detailed, • Only used the accuracy measure for evaluation, • Did not use RMSE for the evaluation of linear regression. |
V. Sankhe et al., 2020 [ ] | • Accuracy = 81.3% | • The implementation of algorithms is comprehensively explained. | • The dataset description is not detailed, • The method of splitting the training and testing dataset is not provided, • Did not mention whether they have conducted any preprocessing on the dataset or if it was used as it is. |
V. Z. Kamila et al., 2019 [ ] | • Accuracy of KNN K = 1:100.00% • Accuracy of Naive Bayes algorithm = 100.00% | • Provided the exact numbers for each metric for the evaluation result. | • The implemented algorithms explanation was very brief, • The performance of the proposed approach is not compared to other similar approaches, • Did not provide any information about the dataset used, • Did not mention whether they have conducted any preprocessing on the dataset or if it was used as it is. |
Authors and Year | Evaluation Metrics | Strengths | Weaknesses |
---|---|---|---|
D. Shah et al., 2017 [ ] | • Normalized mean absolute error (NMAE) = 0.0023, • Computational Time Comparison. | • The implementation of the two compared algorithms is comprehensively explained, • Compared the accuracy of recommendations from both algorithms as well as the speed. | • Did not mention whether they have conducted any preprocessing on the dataset or if it was used as it is, • Similar approaches are not stated in the literature, in addition, the literature was very brief, • Did not use RMSE for evaluation, which is considered the standard as its more accurate. |
The statements, opinions and data contained in all publications are solely those of the individual author(s) and contributor(s) and not of MDPI and/or the editor(s). MDPI and/or the editor(s) disclaim responsibility for any injury to people or property resulting from any ideas, methods, instructions or products referred to in the content. |
Share and Cite
Algarni, S.; Sheldon, F. Systematic Review of Recommendation Systems for Course Selection. Mach. Learn. Knowl. Extr. 2023 , 5 , 560-596. https://doi.org/10.3390/make5020033
Algarni S, Sheldon F. Systematic Review of Recommendation Systems for Course Selection. Machine Learning and Knowledge Extraction . 2023; 5(2):560-596. https://doi.org/10.3390/make5020033
Algarni, Shrooq, and Frederick Sheldon. 2023. "Systematic Review of Recommendation Systems for Course Selection" Machine Learning and Knowledge Extraction 5, no. 2: 560-596. https://doi.org/10.3390/make5020033
Article Metrics
Article access statistics, further information, mdpi initiatives, follow mdpi.
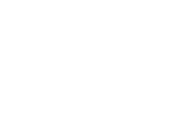
Subscribe to receive issue release notifications and newsletters from MDPI journals
The Infona portal uses cookies, i.e. strings of text saved by a browser on the user's device. The portal can access those files and use them to remember the user's data, such as their chosen settings (screen view, interface language, etc.), or their login data. By using the Infona portal the user accepts automatic saving and using this information for portal operation purposes. More information on the subject can be found in the Privacy Policy and Terms of Service. By closing this window the user confirms that they have read the information on cookie usage, and they accept the privacy policy and the way cookies are used by the portal. You can change the cookie settings in your browser.
- Login or register account
INFONA - science communication portal
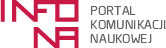
- advanced search
- conferences
- Collections
A literature review and classification of recommender systems research $("#expandableTitles").expandable();
- Contributors
Fields of science
- Bibliography
Expert Systems With Applications > 2012 > 39 > 11 > 10059-10072
Identifiers
journal ISSN : | 0957-4174 |
DOI |
User assignment
Assign to other user | |
Assignment remove confirmation
You're going to remove this assignment. are you sure.
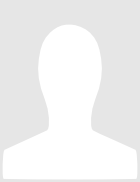
Deuk Hee Park
Hyea kyeong kim, il young choi, jae kyeong kim.
Recommender systems Literature review Data mining technique Classification
Additional information

- Read online
- Add to read later
- Add to collection
- Add to followed

Export to bibliography

- Terms of service
Accessibility options
- Report an error / abuse
Reporting an error / abuse
Sending the report failed.
Submitting the report failed. Please, try again. If the error persists, contact the administrator by writing to [email protected].
You can adjust the font size by pressing a combination of keys:
- CONTROL + + increase font size
- CONTROL + – decrease font
You can change the active elements on the page (buttons and links) by pressing a combination of keys:
- TAB go to the next element
- SHIFT + TAB go to the previous element
Review of the Impact of Auxiliary Information on Recommender Systems
- September 2024
- IEEE Access PP(99):1-1
- CC BY-NC-ND 4.0

- Universiti Teknologi Malaysia
- This person is not on ResearchGate, or hasn't claimed this research yet.

- Kebbi State University of Science and Technology
Abstract and Figures

Discover the world's research
- 25+ million members
- 160+ million publication pages
- 2.3+ billion citations

- Roliana Ibrahim

- Idrees A. Zahid

- Akhmed Kaleel
- ARTIF INTELL REV
- Bjørnar Vassøy

- Shaoqing Wang
- Sultan Alfarhood

- Siti Muslimah Kusuma Haqqu Nurakhmadyavi

- INFORM SCIENCES
- Jianjian Chen

- Xiaoping Lu
- Shuhan Wang
- Nandini Sharma
- Aditi Vaidya
- Shreya Borate

- Xiaodong Li

- Jiangxia Cao

- Recruit researchers
- Join for free
- Login Email Tip: Most researchers use their institutional email address as their ResearchGate login Password Forgot password? Keep me logged in Log in or Continue with Google Welcome back! Please log in. Email · Hint Tip: Most researchers use their institutional email address as their ResearchGate login Password Forgot password? Keep me logged in Log in or Continue with Google No account? Sign up
- Access through your organization
- Purchase PDF
Article preview
Introduction, section snippets, references (55), cited by (20).

Expert Systems with Applications
A literature review for recommender systems techniques used in microblogs.
- • Literature review for recommendations systems used in microblogs.
- • Development of a classification framework for recommender systems used in microblogs.
- • Analyze the research trends on recommender systems used in microblogs.
Related work
Data collection and classification framework, use case of the classification framework.
- • Which users to follow because their contributions are fragmented among various tiny posts.
- • What influences users to follow.
- • The probability that a user recommended to a target user will be accepted.
- • Number of users to follow to obtain
Conclusions
Acknowledgment, followee recommendation based on text analysis of micro-blogging activity, information systems, top-k followee recommendation over microblogging systems by exploiting diverse information sources, future generation computer systems, influential user weighted sentiment analysis on topic based microblogging community, a time-aware spatio-textual recommender system, twilite: a recommendation system for twitter using a probabilistic model based on latent dirichlet allocation, recommender system application developments: a survey, decision support systems, combining tag correlation and user social relation for microblog recommendation, information sciences, a literature review and classification of recommender systems research, dynamic profiles using sentiment analysis for vaa’s recommendation design, procedia computer science, a survey of collaborative filtering based social recommender systems, computer communications, link recommender: collaborative-filtering for recommending urls to twitter users, network-aware recommendations of novel tweets, proceedings of the thirty-ninth international acm sigir conference on research and development in information retrieval, topology-based recommendation of users in micro-blogging communities, journal of computer science and technology, personalized social search based on the user’s social network, proceedings of the eighteenth acm conference on information and knowledge management, social network analysis applied to recommendation systems: alleviating the cold-user problem, proceedings of the international conference on ubiquitous computing and ambient intelligence, content based microblogger recommendation, proceedings of the international conference on privacy, security, risk and trust (passat), and international confernece on social computing (socialcom), hybrid followee recommendation in microblogging systems, science china information sciences, who should i add as a friend: a study of friend recommendations using proximity and homophily, proceedings of the fourth international workshop on modeling social media, personalized microblog recommendation using sentimental features, proceedings of the ieee international conference on big data and smart computing (bigcomp), emtagger: a word embedding based novel method for hashtag recommendation on twitter, ieee international conference on data mining (icdm) acumen workshop, collaborative filtering recommender systems, foundations and trends® in human–computer interaction, transparent user models for personalization, proceedings of the eighteenth acm sigkdd international conference on knowledge discovery and data mining, phrase-based hashtag recommendation for microblog posts, who will you@, proceedings of the twenty-fourth acm international on conference on information and knowledge management, sentiment-based user profiles in microblogging platforms, proceedings of the twenty-sixth acm conference on hypertext & social media, recommending citations: translating papers into references, proceedings of the twenty-first acm international conference on information and knowledge management, from user graph to topics graph: towards twitter followee recommendation based on knowledge graphs, proceedings of the ieee thirty-second international conference on data engineering workshops (icdew), new technique to alleviate the cold start problem in recommender systems using information from social media and random decision forests.
Social media systems are in general an environment where users tend to express themselves in a broad way leaving an enormous digital footprint that could be converted in valuable information [1]. Due to the ever-growing usage of social media and microblogs, used for keeping in touch with people as well as for expressing their opinion about very different topics, a vast amount of information could be extracted and converted into useful knowledge in order to integrate them into a recommender system and generate better estimates [18,26,42,49]. Therefore, this user’s social content could be used and processed with the purpose of elaborating a user’s profile that could help the decision making process [1,3].
Learning peer recommendation using attention-driven CNN with interaction tripartite graph
There has been a notable upsurge in the use of recommendation techniques in online learning [15]. Roughly, recommendation techniques are classified into the three categories: content-based filtering (CBF), collaborative filtering (CF) and hybrid filtering [33]. In early recommendation systems, CBF was widely used to make recommendations to a target learner based on what another learner with similar preferences liked in the past [25], including teaching guidance, adaptive learning and management decisions.
Context-Aware Recommender Systems for Social Networks: Review, Challenges and Opportunities
Using word2vec recommendation for improved purchase prediction, health recommender systems: a state-of-the-art review, current applications of machine learning techniques in crm: a literature review and practical implications.
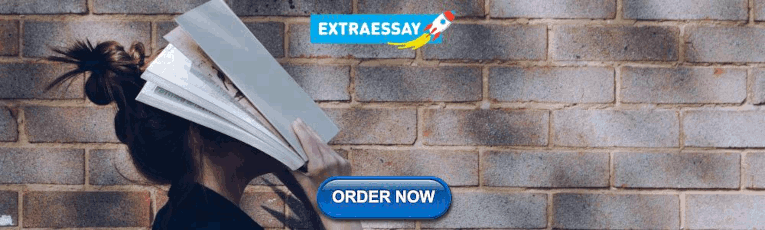
IMAGES
VIDEO
COMMENTS
Recommender systems have become an important research field since the emergence of the first paper on collaborative filtering in the mid-1990s. Although academic research on recommender systems has increased significantly over the past 10 years, there are deficiencies in the comprehensive literature review and classification of that research ...
There is hardly any review paper that has categorically synthesized and reviewed the literature of all the classification fields and application domains of recommender systems. The few existing literature reviews in the field cover just a fraction of the articles or focus only on selected aspects such as system evaluation.
Artificial Intelligence Review. 2020. TLDR. This work proposes a systematic literature review in the field of multicriteria recommender systems, and investigates the domains of application, the exploited evaluation protocols, metrics and datasets, and the most promising suggestions for future works. Expand.
Recommender systems have become an important research field since the emergence of the first paper on collaborative filtering in the mid-1990s. In general, recommender systems are defined as the ...
Although academic research on recommender systems has increased significantly over the past 10 years, there are deficiencies in the comprehensive literature review and classification of that research.
1. Introduction. Recommender systems have become an im-portant research field since the emergence of the first paper on collaborative filtering in the mid- 1990s (Resnick et al., 1994; Shardanand ...
Recommender system is an important research domain which has already attracted a lot of researchers during the period of the last decade. Various approaches and techniques have been proposed by different researchers related to recommender systems. ... In this paper, the classification of the literature review has been done into seven different ...
Findings of this paper indicate that the research field of movie field received most research field in recommender systems researches, and the review and classification framework was independently verified. To understand the trend of recommender system researches by examining the published literature, and to provide practitioners and researchers with insight and future direction on recommender ...
A literature review and classification of recommender systems research. Expert Syst Appl, 39 (11) (2012), pp. 10059-10072. View PDF View article View in Scopus Google Scholar [68] X. Su, T.M. Khoshgoftaar. A survey of collaborative filtering techniques. Adv Artif Intell, 4 (2009), p. 19.
This paper aims to fulfil this significant gap by reviewing and comparing existing articles on recommender systems based on a defined classification framework, their algorithmic categorization, simulation platforms used, applications focused, their features and challenges, dataset description and system per-formance.
Recommender systems have become an important research field since the emergence of the first paper on collaborative filtering in the mid-1990s. Although academic research on recommender systems has increased significantly over the past 10years, there are deficiencies in the comprehensive literature review and classification of that research.
Recommender Systems and Techniques. Recommender techniques are traditionally divided into different categories [12,13] and are discussed in several state-of-the-art surveys [].Collaborative filtering is the most used and mature technique that compares the actions of multiple users to generate personalized suggestions. An example of this technique can typically be found on e-commerce sites ...
evolving field of review-based recommender systems. Contribution of this survey: The key contributions of this survey can be summarized as follows: (1) We systematically explore review-based recommender systems and introduce a classification scheme that considers feature extraction techniques and methodologies used to incorporate reviews into the
In this paper, we provide an in-depth literature review on Recommender system main approaches. The paper is organized as follows: in Sect. 2 we introduce the popular approaches used in the recommendation field. In Sect. 3, we supply a discussion that shows the advantages and disadvantages of each approach.
A systematic literature review on educational recommender systems for teaching and learning: research trends, limitations and opportunities ... a categorization framework is presented and the study includes the classification of selected papers according to it. ... literature lacks on identifying current limitations and opportunities in ...
Recommender systems research and practice are fast-developing topics with growing adoption in a wide variety of information access scenarios. ... As our literature review is concerned with research on the evaluation of recommender ... Despite the absence of a universally accepted classification of metrics in the recommender systems research ...
This research reviewed all articles on recommender systems from 37 journals which were published from 2001 to 2010 to provide trend of recommender system researches by examining the published literature, and provides practitioners and researchers with insight and future direction onRecommender systems. Recommender systems have become an important research field since the emergence of the first ...
Recommender systems have become an important research field since the emergence of the first paper on collaborative filtering in the mid-1990s. Although academic research on recommender systems has increased significantly over the past 10 years, there are deficiencies in the comprehensive literature review and classification of that research ...
Design/methodology/approach. In this paper, the SLR method is utilized with the aim of identifying, evaluating and integrating the findings of all relevant and high-quality individual studies addressing one or more research questions about recommender systems and performing a comprehensive study of empirical research on recommender systems that have been divided into five main categories.
Course recommender systems play an increasingly pivotal role in the educational landscape, driving personalization and informed decision-making for students. However, these systems face significant challenges, including managing a large and dynamic decision space and addressing the cold start problem for new students. This article endeavors to provide a comprehensive review and background to ...
Recommender systems have become an important research field since the emergence of the first paper on collaborative filtering in the mid-1990s. Although academic research on recommender systems has increased significantly over the past 10years, there are deficiencies in the comprehensive literature review and classification of that research. For that reason, we reviewed 210 articles on ...
Systematic literature review of implemented recommender systems for MOOCs. ... This review includes research work over eight years, i.e. from 1st January 2012 to 17th November 2020. ... this is the first systematic literature review based on a classification framework of RSs that have been implemented in MOOCs from January 1st 2012 to 17 ...
This systematic review investigates the impact of incorporating auxiliary information into various types of recommender systems, examining recent advancements, methodologies, datasets, evaluation ...
This growth goes hand in hand with the increase in the amount of research conducted using microblogging data, presented in academic literature (Kefalas, Symeonidis, & Manolopoulos, 2013). The paper is structured as follows: The first section presents related work regarding the design of a conceptual framework for RSs.