Social Network Analysis with NetworkX
Manojit Nandi 2015-07-14 | 7 min read
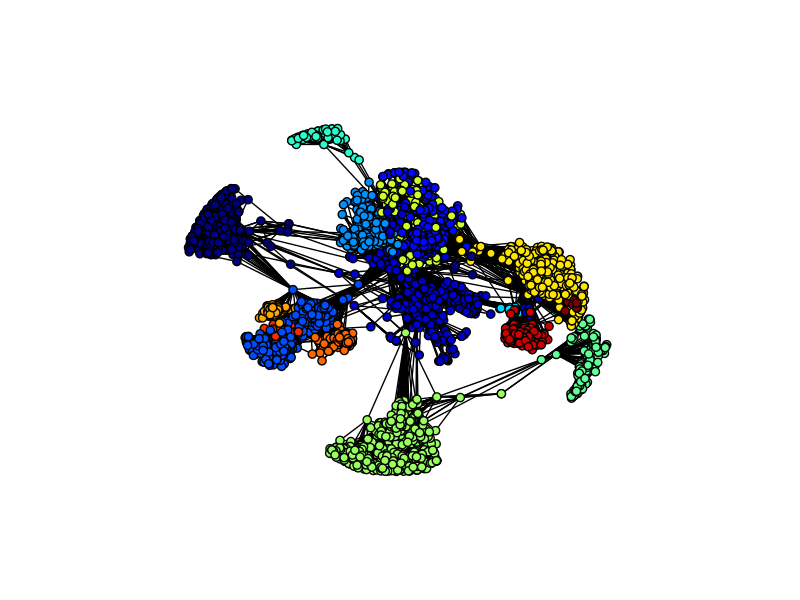
Many types of real-world problems involve dependencies between records in the data. For example, sociologist are eager to understand how people influence the behaviors of their peers; biologists wish to learn how proteins regulate the actions of other proteins. Problems involving dependencies can often be modeled as graphs, and scientists have developed methods for answering these questions called network analysis.
This post describes how to use the Python library NetworkX , to deal with network data and solve interesting problems in network analysis.
- Intro to Graphs
Before we dive into a real-world network analysis, let's first review what a graph is. A graph consists of a set of objects V called vertices and a set of edges E connecting pairs of vertices.
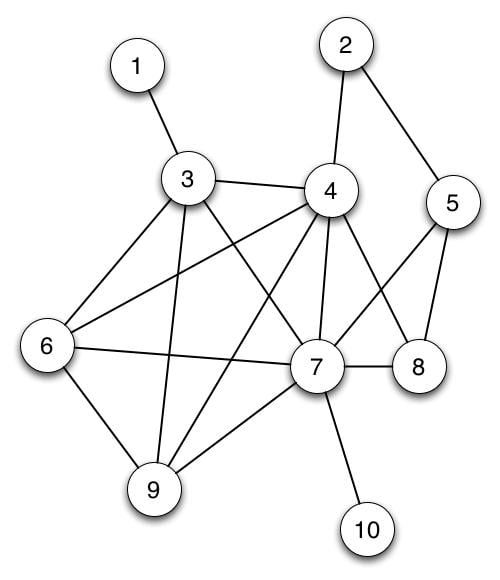
In the above picture, the circles represent the vertices and lines connecting the circles are edges. If this graph represented a social network, the circles would represent people, and an edge between two vertices could signify that those two individuals are friends.
We will look at a special type of network called an ego network. In an ego network, there is a "central" vertex or the ego vertex which the network revolves around.
Ego networks are important in sociology because they allow researchers to study the structure of an individual's relationships with respect to his world.
- Getting started with NetworkX
Domino offers NetworkX as one of its default Python libraries, so all you have to do is import the library with the above statement.
Now, let's consider a real-world dataset: Facebook Ego Networks !
This Facebook combined ego networks datasets contains the aggregated network of ten individuals' Facebook friends list. In this dataset, the vertices represent individuals on Facebook, and an edge between two users means they are Facebook friends. Each ego-network was created by starting with a Facebook user and then mining all of his Facebook friends. The ego-networks are then aggregated by identifying individuals who appear in multiple ego-networks.
Here's some basic information about the network dataset we will be working with:
- Nodes: 4039
- Edges: 88,234
- Average Degree: 43.6910
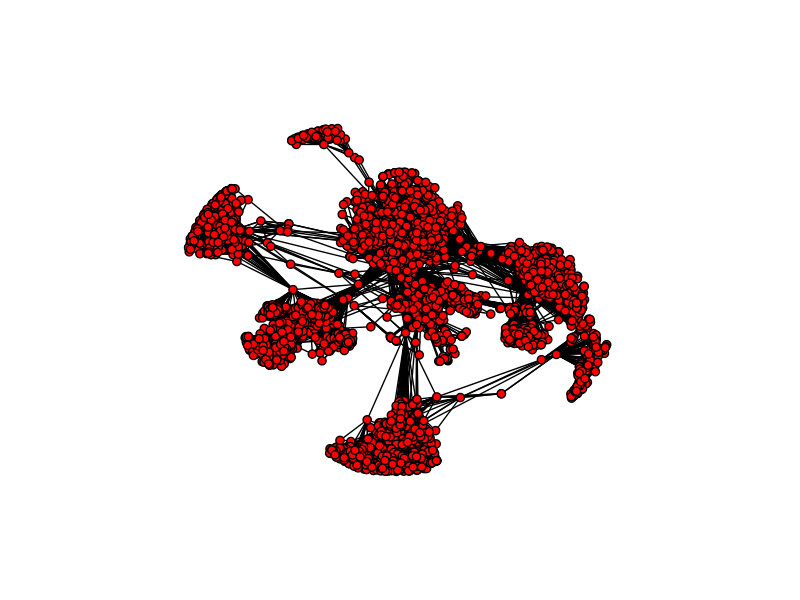
As you can see this is a fairly connected network, and the number of edges in the network is more than 20x the number of nodes, so the network is densely clustered. If you examine the network, you will notice certain hubs of vertices appear. Hubs like these are an important feature of real-world social networks. Dense hubs represent high clustering and are often the result of underlying social mechanisms that are of interest to scientists.
- Parallel Betweenness
How do we determine who is the most "important" individual in the network. Well, that depends on the definition of "important". One way to define "importance" is the individual's betweenness centrality . The betweenness centrality is a measure of how many shortest paths pass through a particular vertex. The more shortest paths that pass through the vertex, the more central the vertex is to the network.
Because the shortest path between any pair of vertices can be determined independently of any other pair of vertices, we can take advantage of Domino's multi-core hardware to compute the betweenness centrality of each vertex in the network using a parallelized algorithm.
To do this, we will use the Pool object from the multiprocessing library and the itertools library. A nice introduction to the multiprocessing library and parallel programming in Python can be found here.
First thing we need to do is partition the vertices of the network into n subsets where n is dependent on the number of processors we have access to. For example, if we use a machine with 32 cores, we partition the Facebook network in 32 chunks with each chunk containing 128 vertices.
Now instead of one processor computing the betweenness for all 4,039 vertices, we can have 32 processors computing the betweenness for each of their 128 vertices in parallel. This drastically reduces the run-time of the algorithm and allows it to scale to larger networks.
Now, let's look at the vertices with the top 10 highest betweenness centrality measures in the network.
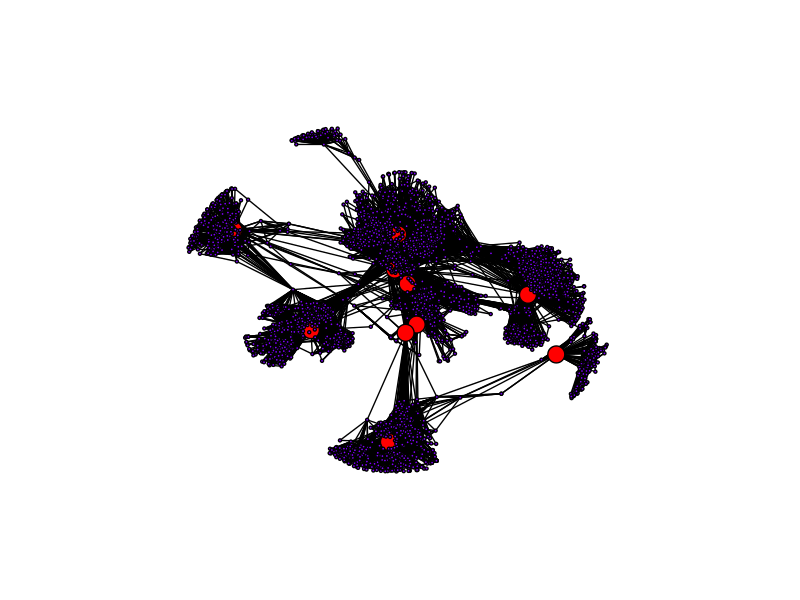
As you can see, vertices that primarily either sit at the center of a hub or acts a bridge between two hubs have higher betweenness centrality. The bridge vertices have high betweenness because all paths connecting the hubs pass through them, and the hub center vertices have high betweenness because all intra-hub paths pass through them.
- Community Detection
For those of you who use Facebook, your Facebook friends probably come from different aspects of your life: Some are your friends from college, others are your co-workers, and maybe some old friends from your hometown.
Because your friends can be broken down into different groups like this, you may wonder if we could identify these different communities in your social network. The answer is yes! Using community detection algorithms, we can break down a social network into different potentially overlapping communities.
The criteria for finding good communities is similar to that for finding good clusters. We want to maximize intra-community edges while minimizing inter-community edges. Formally, the algorithm tries to maximize the modularity of network, or the fraction of edges that fall within the community minus the expected fraction of edges if the edges were distributed by random. Good communities should have a high number of intra-community edges, so by maximizing the modularity, we detect dense communities that have a high fraction of intra-community edges.
While there is no community detection method in NetworkX, a good samaritan has written a community detection library built on top of NetworkX .
This library is easy to use and allows to perform community detection on an undirected graph in less than 3 lines of code!
That's all there is to it!
Now let's look at the different communities.
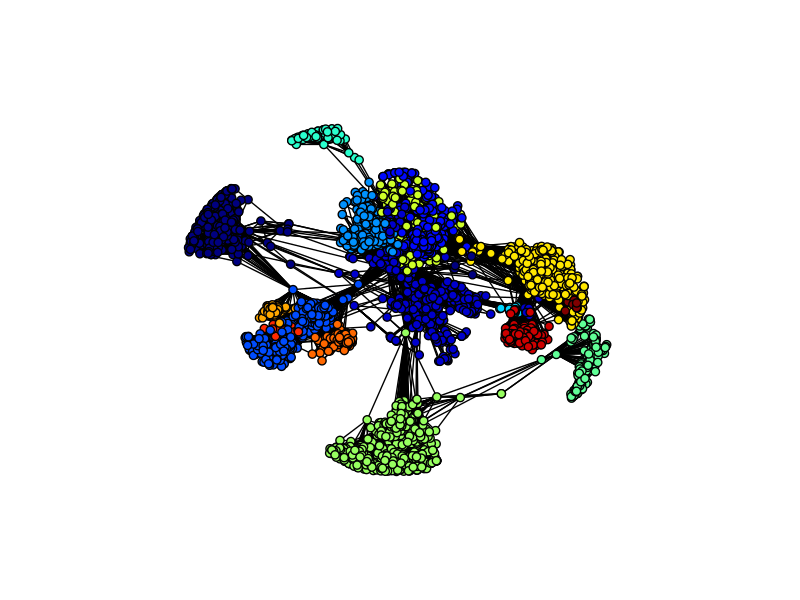
As you can see, the communities closely align with the vertex hubs. Because this dataset was compiled by aggregating the ego network of ten individuals, the different communities most likely reflect the different ego networks.
Graphs and networks are becoming more popular in data science everyday. Neo4j is a database that represents data as a graph, and topological data analysis algorithms and spectral clustering algorithms build upon graphs to identify flexible patterns and sub-structures in data.
You can use Domino to run network algorithms on large hardware to speed up your calculations.
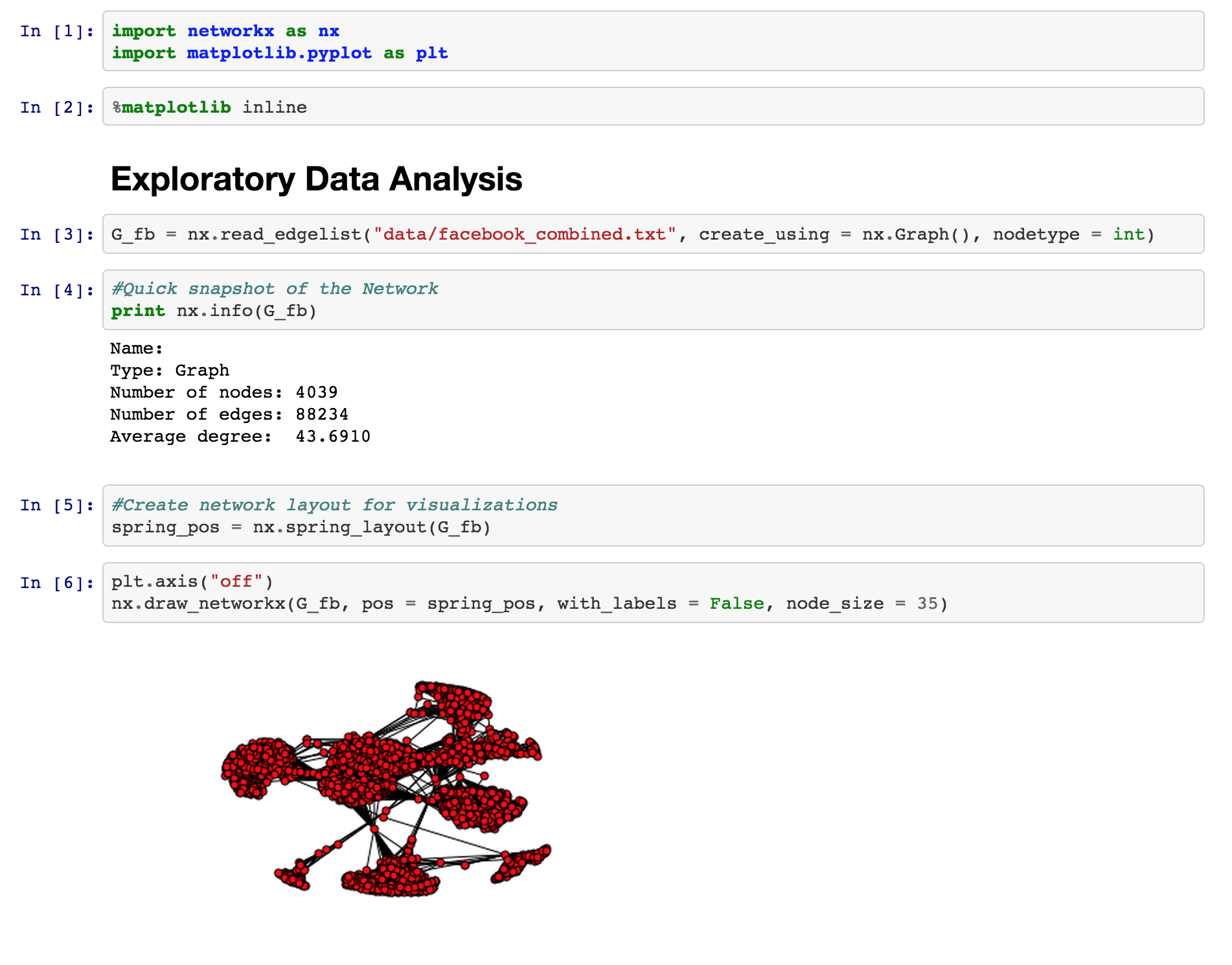
Manojit is a Senior Data Scientist at Microsoft Research where he developed the FairLearn and work to understand data scientist face when applying fairness techniques to their day-to-day work. Previously, Manojit was at JPMorgan Chase on the Global Technology Infrastructure Applied AI team where he worked on explainability for anomaly detection models. Manojit is interested in using my data science skills to solve problems with high societal impact.
RELATED TAGS
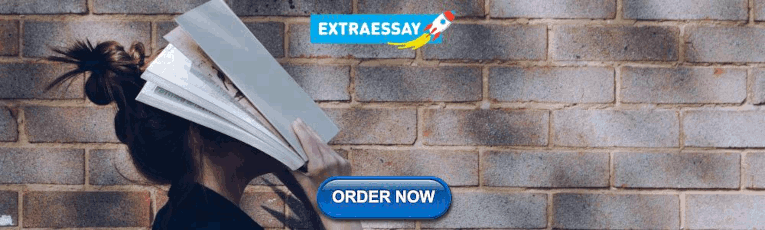
Other posts you might be interested in
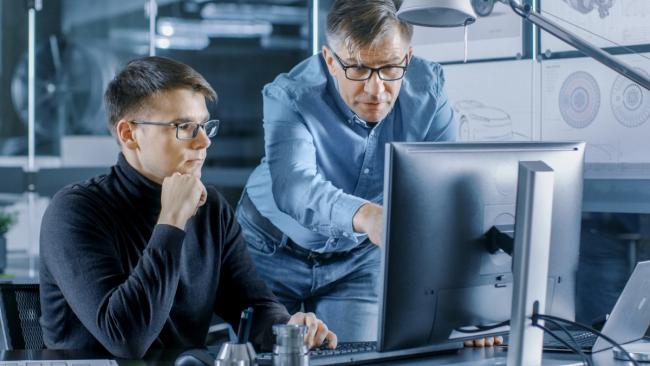
Data Science
Legacy code to open source: Generative AI to the rescue
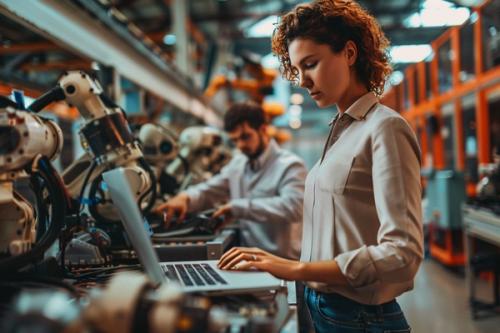
Data Science Platform
Domino expands Generative AI capabilities with AI Gateway and Vector Data Access
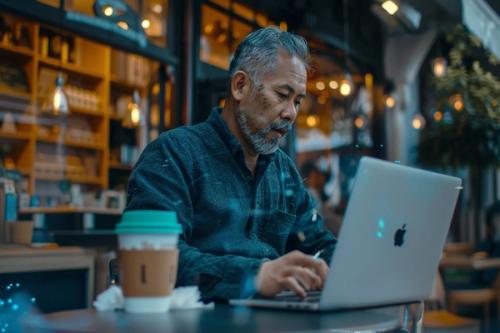
Generative AI
Prompt engineering slowing you down? It’s time to try RAG and here's why.
Digital methods workshop
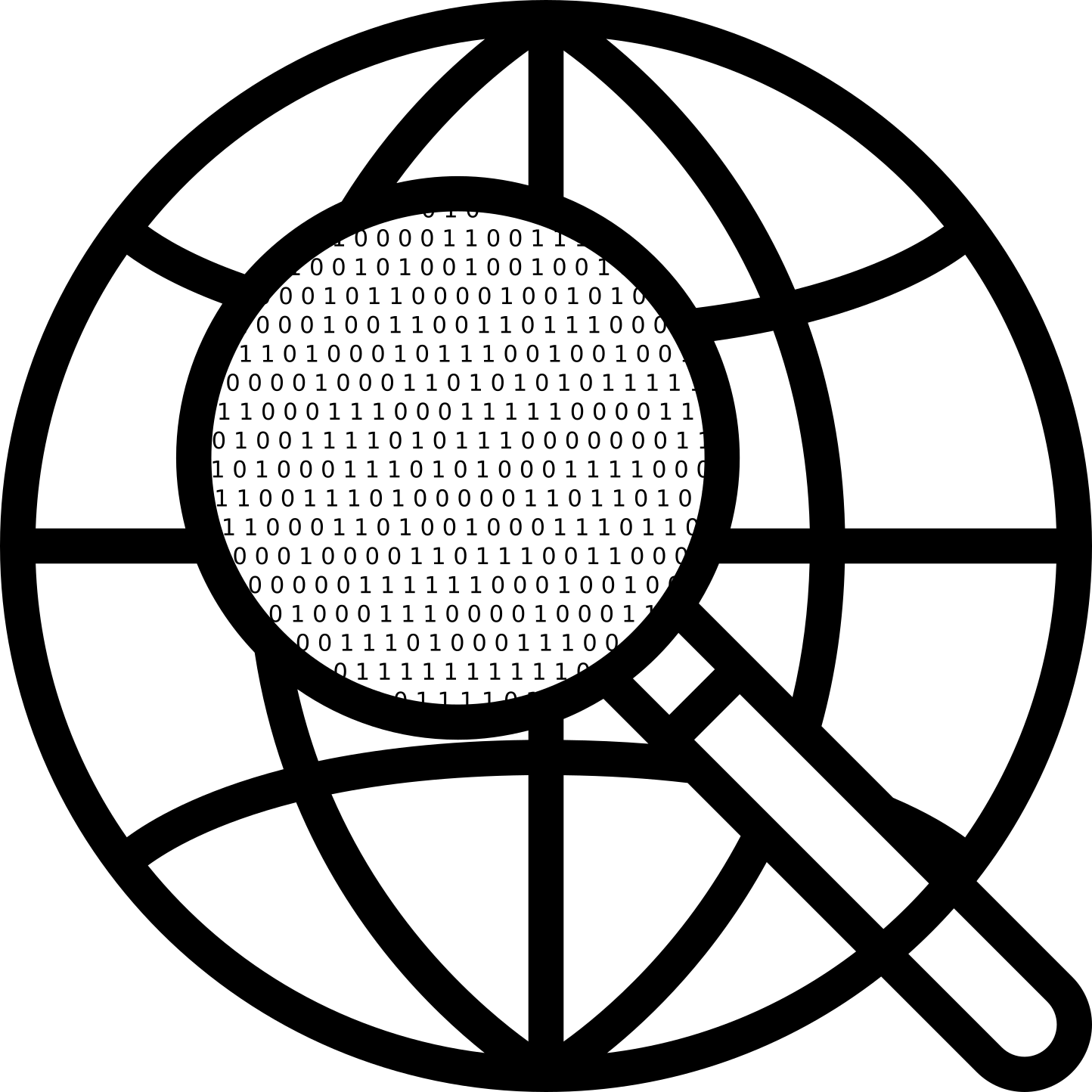
6-7 June 2018 | VSCC Seminar Room 218, The University of Sydney
View the Project on GitHub Digital-Methods-Sydney/ws-201806
A practical introduction to (social) network analysis
Thursday 7 June 2018, 13:00 - 15:00, VSCC Seminar Room 218, The University of Sydney
With Tim Graham, ANU
1. A brief introduction to SNA theory with application examples (Tim)
2. hands-on tutorial with socialmedialab (tim).
You are invited to bring your own laptop to the tutorial. You can prepare for the tutorial by installing R and RStudio on your computer.
To install and get started with R and RStudio
To access the materials of this tutorial
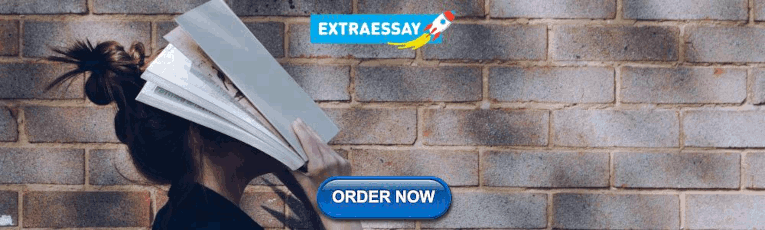
Loading to Python Data Analysis Practice....
Loading to Introduction To Portfolio Construction And Analysis With Python....
Loading to Is Business Analytics A Stem Course....
Navigation Menu
Search code, repositories, users, issues, pull requests..., provide feedback.
We read every piece of feedback, and take your input very seriously.
Saved searches
Use saved searches to filter your results more quickly.
To see all available qualifiers, see our documentation .
- Notifications You must be signed in to change notification settings
Assignment-4
HattanAziz/-Applied-Social-Network-Analysis-in-Python-Assignment-4
Folders and files.
Name | Name | |||
---|---|---|---|---|
2 Commits | ||||
Repository files navigation
-applied-social-network-analysis-in-python-assignment-4.
- Jupyter Notebook 100.0%
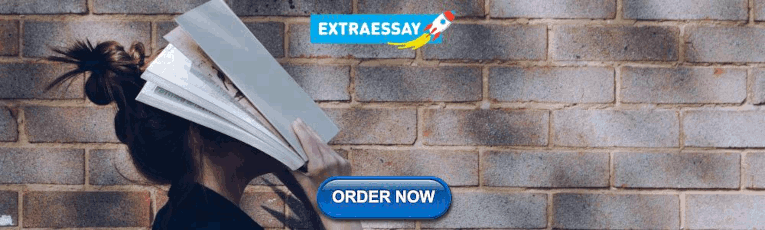
IMAGES
VIDEO
COMMENTS
The final week will explore the evolution of networks over time and cover models of network generation and the link prediction problem. This course should be taken after: Introduction to Data Science in Python, Applied Plotting, Charting & Data Representation in Python, and Applied Machine Learning in Python.
Applied Social Network Analysis in Python. This repository contains a collection of the assignments used in the course Applied Social Network Analysis in Python, part of Applied Data Science using Python Specialization from University of Michigan offered by Coursera. This course will introduce the learner to network analysis through tutorials ...
This repository contains notes, assignments, quizzes and code files from the "Applied Social Network Analysis in Python" course by University of Michigan, on Coursera. - Anacoder1/Applied...
There are 4 modules in this course. This course will introduce the learner to network analysis through tutorials using the NetworkX library. The course begins with an understanding of what network analysis is and motivations for why we might model phenomena as networks. The second week introduces the concept of connectivity and network robustness.
Assignment 1 - Applied Social Network Analysis in Python - Free download as PDF File (.pdf), Text File (.txt) or read online for free. This document contains instructions for an assignment analyzing employee movie preferences and relationships using NetworkX and Pandas. It provides sample code to: 1. Load a bipartite graph from employee-movie choice data into a NetworkX graph.
Source: Huang, Chung-Yuan et al. "Influence of Local Information on Social Simulations in Small-World Network Models."J. Artif. Soc. Soc. Simul. 8 (2005) Small World phenomenon claims that real networks often have very short paths (in terms of number of hops) between any connected network members. This applies for real and virtual social networks (the six handshakes theory) and for ...
The ability to analyze these networks and make informed decisions based on them is a skill that is important for any data analyst. The focus of this tutorial is to teach social network analysis (SNA) using Python and NetworkX, a Python library for the study of the structure, dynamics, and functions of complex networks.
Social network analysis is the process of investigating social structures through the use of networks and graph theory. This article introduces data scientists to the theory of social networks, with a short introduction to graph theory, information spread and influence maximization [6]. It dives into Python code with NetworkX [8] constructing and.
# For the second part of this assignment you will be workking with a company's email network where each node corresponds to a person at the company, and each edge indicates that at least one email has been sent between two people.
The final week will explore the evolution of networks over time and cover models of network generation and the link prediction problem. This course should be taken after: Introduction to Data Science in Python, Applied Plotting, Charting & Data Representation in Python, and Applied Machine Learning in Python.
Social Network Analysis with NetworkX. Many types of real-world problems involve dependencies between records in the data. For example, sociologist are eager to understand how people influence the behaviors of their peers; biologists wish to learn how proteins regulate the actions of other proteins. Problems involving dependencies can often be ...
A practical introduction to (social) network analysis. Thursday 7 June 2018, 13:00 - 15:00, VSCC Seminar Room 218, The University of Sydney. With Tim Graham, ANU. 1. A brief introduction to SNA theory with application examples (Tim) 2. Hands-on tutorial with SocialMediaLab (Tim) You are invited to bring your own laptop to the tutorial.
Python Data Analysis Practice Advantages of eBooks Over Traditional Books 2. Identifying Python Data Analysis Practice Exploring Different Genres Considering Fiction vs. Non-Fiction Determining Your Reading Goals 3. Choosing the Right eBook Platform Popular eBook Platforms Features to Look for in an Python Data Analysis Practice User-Friendly ...
1 - 25 of 453 Reviews for Applied Social Network Analysis in Python. If you are following Dr. Severance's Python course series, expecting a similar experience, be ready for disappointment. Where Dr. Chuck's series is well-taught in-depth information with great examples and explanations, this course series is basically a summary and the ...
network analysis to causal inference. At the same time, there will be an overarching emphasis on how these technical skills can be applied to social questions and problems in a scientific way. I wish each and every one of you great success in your studies here at LSE. Dr Daniel de Kadt MSc Applied Social Data Science Programme Director
Practical Time Series Analysis Dr. Avishek Pal,Dr. PKS Prakash,2017-09-28 Step by Step guide filled with real world practical examples. About This Book Get your first experience with data analysis with one of the most powerful types of analysis—time-series. Find patterns in your data and predict the future pattern based on historical data.
The Use of Python & Machine Learning to Optimise a … main goal of the paper is to construction of an investment portfolio of small cap companies, which uses fundamental analysis to select the top-rated stocks. The analysis A novel portfolio construction strategy based on the core- This section discusses the comparative analysis of portfolio
Offered by University of Michigan. This course will introduce the learner to network analysis through tutorials using the NetworkX library. ... Enroll for free.
This course should be taken after Introduction to Data Science in Python and before the remainder of the Applied Data Science with Python courses: Applied Machine Learning in Python, Applied Text Mining in Python, and Applied Social Network Analysis in Python.
Applied Social Network Analysis in Python is course 5 of 5 in the Applied Data Science with Python Specialization offered on coursera by the University of Michigan. Resources. Readme Activity. Stars. 0 stars Watchers. 0 watching Forks. 0 forks Report repository Releases No releases published.
You signed in with another tab or window. Reload to refresh your session. You signed out in another tab or window. Reload to refresh your session. You switched accounts on another tab or window.
for Business Analytics: Concepts, Techniques, and Applications in Python presents an applied approach to data mining concepts and methods, using Python software for illustration Readers will learn how to implement a variety of popular data mining algorithms in Python (a free and open-source software) to tackle business problems and opportunities.
Star 0. HattanAziz/-Applied-Social-Network-Analysis-in-Python-Assignment-4. This commit does not belong to any branch on this repository, and may belong to a fork outside of the repository. main.