- Remote Sensing Project Topics
As the name suggests, “remote sensing” refers to capturing and monitoring information from the longest distance in wireless mode. For instance: sensing the green vegetation on the earth’s surface from a satellite . For that, it uses electromagnetic waves obtained from sensed objects in the form of diffraction, emission, and reflection . This article is envisioned to provide key Remote Sensing Project Topics along with its research areas, trends, issues, and techniques!!!
From this, we can identify the need and importance of enhancing natural resource management and a green environment . Further, the remote sensing systems or platforms provide below data,
- It is simple and quick to collect data
- It gives unreachable area information
- It enables to compare surveyors’ team which is an inexpensive process
- It quickly generates maps for interpretation
- It gives extremely huge-scale area information
- It acquires area images in constant time duration where the environmental changes can be analysed
In the remote sensing research field, one should know some key terminologies that are widely used among researcher’s interested people . These terms are not only just the terminologies to know but also the principal operations involved in remote sensing . The first term represents the overall main operations involved in remote sensing and the last three terms represent the workflow of remote sensing project topics.
Our professional team of experts has sufficient skill in developing remote sensing projects based on your handhold client’s requirements . Further, we also assist you to provide you development plan to realize your project workflow .
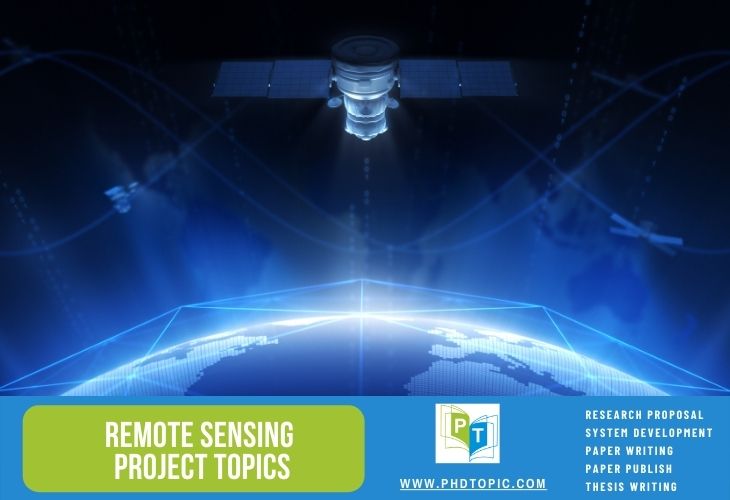
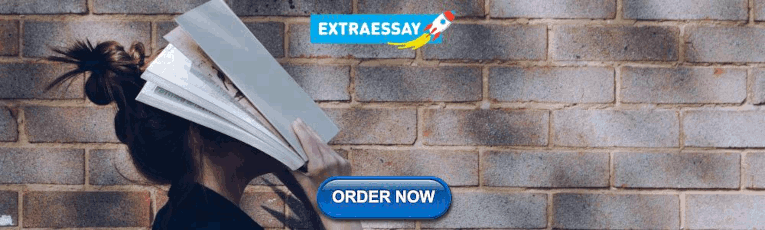
What are the primary terms in remote sensing?
- Addresses entire operations involved in remote sensing space systems
- For instance: data acquisition, analysis, examination, interpretation, and distribution
- Collect data from remote sensors in the space objects
- Transfer collected raw data to earth through telemetry in different forms
- For instance – magnetic tape, electromagnetic signals, photographic film, etc.
- Get the above collected primary data as input
- Process collected data to extract useful information by eliminating unnecessary data
- Get above processed data as input
- Perform required techniques to interpret the processed data and other sources in detail
Now, we can see some significant research challenges involved in remote sensing. Intending to provide innovative research topics based on current scholars’ demands, we work deeply in analysing the recent research journal papers and magazines. So, we can update the recent technological development frequently.
This makes us find the up-to-date research issues that scholars are expecting to solve through advanced methodologies . Moreover, we also provide you with some interesting research ideas to crack the research handpicked research issues/challenges to craft remote sensing project topics . To know the appropriate solutions for the below-specified research issues in remote sensing , approach our team.
What are the important issues in remote sensing?
- Some sensors are lacking in measuring the events in uncertain situations
- Although the inferential techniques are utilized, the sensed data may be inaccurate
- For instance; underwater research
- Although there are several advanced remote sensing tools, lack of data distribution availability may cause a severe impact in the remote sensing field
- Even the data is commercially available, it is very expensive for economically weak researchers
- Specifically, developing countries are largely get affected by this issue
- Since it stops the remote sensing data accessibility for processing and assessment
For illustration, here we have taken the sensor as an example from the above list. Generally, there are numerous sensors are available for the remote sensing process . And, these sensors are differentiated by their characteristics, merits, and demerits. Depending on this information, one should select the appropriate sensor for their project.
Suppose if the sensor attempts to capture images then it should need to consider certain factors such as area coverage, image resolution, flight restriction , etc. For instance: the satellite has a global view, the drone has small and the aircraft has a limited view . Here, we have given you a few most widely used sensor technologies for remote sensing.
What are the 3 remote sensing tools in geography?
- Sound Navigation Ranging (Sonar)
- Sensors, Radiometers and Spectrometers
- Light Detection and Ranging (LiDAR)
From the above, Sonar and LiDAR are used to construct the topographic models. Although these techniques are used for a common purpose, it has one main difference i.e., a supportive environment. In specific, Sonar can be used underwater and LiDAR can be used underground. In addition to topographic models, we can also construct assessment models. Examples of topographic models are delineating watersheds, archaeological sites, and flood risk prediction. Overall, we support you with more number research areas of remote sensing through the above-specified tools. For your reference, here we have given you some important research areas that provide uncountable Remote Sensing Project for research / final year project .
Innovative Remote Sensing Project Topics
- Image Classification
- LiDAR-based Remote Sensing
- Various Imagining Techniques
- Green House Gases Prediction
- PAN and MS Image Merging
- Green Vegetation Land Estimation
- UAV-assisted Sensing in Emergency Situations
In addition, we have also given you some latest research directions on remote sensing . Beyond this list of trends, we also support you in other emerging research perspectives. Further, we also support your interest to develop your idea. Our experts help you to refine your desired idea based on the current research demands and technologies . And, we have framed several Remote Sensing Projects to support following trends and other important trends of remote sensing project topics . We assure you that we provide step-by-step research assistance to support you from the beginning onwards . So, approach us to avail friendly research and development support in your interested remote sensing domain.
Current Trends in Remote Sensing
- Improved Radiometric Resolution for Sensor-centric Characterization
- Encryption of Large-scale Sensed Data over Long Distance
- Environmental Factors Implication on Imaging State
- Employment of Hyperspectral and Multispectral Data for High-Dimension Monitoring
What is the classification of remote sensing data?
Now, we can see the importance of “classification’ in remote sensing. Classification is one of the main processes in digital image processing. While classifying remotely sensed images, it assigns pixel/pixels to the land cover class. If the pixel is bound up with one class, then it is referred to as hard or crisp classification. If the pixel is bound up with multiple classes, then it is referred to as soft or fuzzy classification.
Before implementing the classification process, pre-process method has to be implemented. Since it is necessary to eliminate the artifacts for processing only meaningful information . Here, we have given you a list of preprocessing methods based on artifact types.
- Haze and Low illumination (Histogram Truncation)
- Contextual noise (Difference of Offset Gaussian Filters)
- Speckle noise (Dynamic Thresholding and Image Rationing)
- Contrast Variation (Sub-histogram Equalization and Fuzzy Clustering)
- Blur (Improved S2CVA and Gram-Schmidt Spectral Sharpening)
- Brightness Distortion (Cuckoo Search-based Discrete Wavelet Transform)
- Intensity Variation (Simulated Annealing, Hybrid Ant Colony and Genetic Algorithm)
- Haze (Radiative Transfer-based Detail-Injection Method)
- Irregular Intensity (Alternating Direction Method of Multipliers with Split Bregman Iteration (ADMM-SBI) and Maximum-a-Posterior)
- Brightness and Contrast (BAT algorithm for Histogram Equalized Contrast Enhancement)
Now, we can see the classification process in detail. The input of the classification process is preprocessed data . Generally, the classification is categorized into two types where one is pixel-based classification and the other is object-oriented classification . In pixel-based classification, the whole scene is processed pixel-by-pixel . So, it is also addressed as per-pixel classification. Object-oriented classification, the whole scene is decomposed into several segments/patches using the image segmentation technique . Further, it is used as high-spatial-resolution imagery where the characteristics are subjected to the conventional fuzzy logic .
Deep Learning (DL) is the most widely used technique for performing supervised learning processes as classification . These supervised classification algorithms are used to allocate unknown pixels in the place of m possible classes. Further, the selection of a particular classifier is based on the input data characteristics. Parametric classification assumes that observed vectors in each class and the spectral band are classified as Gaussian . Nonparametric classification will make such an assumption. For your information, here we have classified some extensively used algorithms .
- Matched Filtering
- Linear Spectral Unmixing
- Spectral Angle Mapper
- Spectral Feature Filtering
- Binary Encoding
- Parallepieped
- Neural Network
- 1Dimensional Density Slicing
- Expert System Analysis
- Nearest-neighbor
- Minimum Distance
- Maximum Likelihood
Further, other primary challenges need to be addressed such as environmental state, system trade-off, high-altitude imaging, etc. Since all these challenges may cause an effect on observation quality. Deep learning plays a major role in tackling many important research challenges . Besides, we also support you with other efficient techniques to solve any sort of complicated research issue .
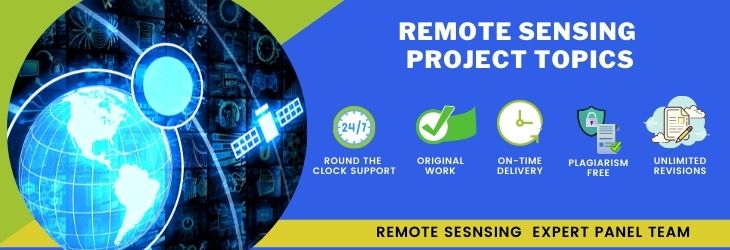
Deep Learning Methods for Remote Sensing
The measurement uncertainties like varieties, complexity, and volume create blockages in the data analysis process. To crack this main obstacle, machine learning was employed. These techniques automate the analysis process and enhance the observation quality . In this, it first removes the unwanted artifacts using deep learning which is a subset of machine learning.
The motive of deep learning techniques is to consider the important factors such as pan-sharping, denoising, fusion, single / multi-band super-resolution, restoration, etc. to enhance the Remote sensing and GIS observation. Some of the other commonly used deep learning-based methods are given as follows,
- DenseNet-201
- DenseNet-121
- Generative Adversarial Networks
- Convolutional Neural Networks
Furthermore, we have also given you the other major concepts that work on the principle of deep learning algorithms . Our developers are adept to handle different input datasets using various advanced techniques to achieve desired research objectives/project goals. Here, we have given you some key concepts with their input data and techniques. Similarly, we also support other primary research concepts of remote sensing project topics with their best-fitting research datasets and techniques .
Important Deep Learning Concepts
- Input – SAR and MS
- Concentrated observation-based CNN
- Input – HS and MS
- Concentrated observation-based 3D-CNN
- 2-stream CNNS-based Fusion
- Input – SAR and RGB
- GAN-based Models\
- CNN sub-networks Fusion
Performance Evaluation in Remote Sensing
Although remote-sensing-driven data are evolving fast, it has some in-built errors from different sources . To reduce the errors, we need the source and perform an accuracy evaluation. For that, first , develop metadata before strategy decision and analysis. And also, it needs GIS layers to support classification. For any kind of application, we use appropriate performance metrics to enhance the efficiency of the system .
- True positives
- False positives
On the whole, we are glad to support you in every stage of research from recent research area identification to code development evaluation . Further, we also provide project documentation/dissertation support. If you are a research scholar, then we extend our services in novel remote sensing project topics , phd guidance , research proposal writing, paper writing, the paper publication with an acceptance guarantee, and thesis writing. Therefore, use this opportunity to utilize all your required services in one place like us.
Related Pages
Services we offer.
Mathematical proof
Pseudo code
Conference Paper
Research Proposal
System Design
Literature Survey
Data Collection
Thesis Writing
Data Analysis
Rough Draft
Paper Collection
Code and Programs
Paper Writing
Course Work
M | T | W | T | F | S | S |
---|---|---|---|---|---|---|
1 | ||||||
2 | 3 | 4 | 5 | 6 | 7 | 8 |
9 | 10 | 11 | 12 | 13 | 14 | 15 |
16 | 17 | 18 | 19 | 20 | 21 | 22 |
23 | 24 | 25 | 26 | 27 | 28 | 29 |
30 |
- BE Projects
- B Tech Projects
- ME Projects
- M Tech Projects
- mca projects
- Mini Projects for CSE
- Mini Projects for ECE
- Mini Projects for IT
- IEEE Projects for CSE
- IEEE Projects for ECE
- Digital Image Processing Projects
- Medical Image Processing Projects
- Matlab Thesis
- Fuzzy Logic Matlab
- Matlab Projects
- Matlab Simulation Projects
- Matlab based Communication Projects
- Medical Imaging Projects
- Biomedical Engineering Projects
- Image Processing Thesis
- Scilab Projects
- OpenCV Projects
- Steganography Projects
- Cryptography Projects
- Cyber Security Projects
- Network Security Projects
- Information Security Projects
- Wireless body area network projects
- Wireless Communication Projects
- Wireless Sensor Networks Projects
- Wireless Network Projects
- Router Projects
- CAN Protocol Projects
- NS2 Projects
- NS3 Projects
- Opnet Projects
- Omnet Projects
- Qualnet Projects
- VANET Projects
- Manet Projects
- LTE Projects
- Ad hoc projects
- Software Defined networking projects
- Peersim Projects
- P2P Live Streaming Projects
- Video Streaming Projects
- Green Radio Projects
- Distributed Computing Projects
- PPI Projects
- Cognitive Radio Projects
- IoT Projects
- m2m projects
- Hadoop Projects
- MapReduce Projects
- Core Java Projects
- Forensics Projects
- Cloudsim Projects
- Cloud Analyst Projects
- Weka Projects
- Pattern Recognition Projects
- Gridsim Projects
- Augmented Reality Projects
- Android Projects
- Rtool Projects
- Software Engineering Projects
- ARM Projects
- Signal Processing Projects
- GPS Projects
- GSM Projects
- RFID Projects
- Embedded System Projects
- LabVIEW Projects
- Microcontroller Projects
- Robotics Projects
- VHDL Projects
- FPGA Projects
- Zigbee Projects
- Simulink Projects
- Power Electronics Projects
- Renewable Energy Projects for Engineering Students
- Writing Phd Thesis
- Cognitive Radio Thesis
- Vanet Thesis
- Manet Thesis
- Mobile Application Thesis
- Neural Network Thesis
- Security system Thesis
- Steganography Thesis
- Software Defined Networking Thesis
- Wireless Network Sensor Thesis
- Computer Science Thesis
- M Tech Thesis
- Phd Projects
- Dissertation Writing Service
Generally, remote sensing is the technology that is intended to observe the earth’s surface with the help of satellites, airplanes, and drones by deploying a camera in them. The sensors in the sensing tools capture the data related to the target then it will be processed as an image for the analytical purpose . In other words, remote sensing is the science technology that is used to gather data of the surface which cannot be manually visualized .
“This article contains the data about the possible Project Topics on GIS and Remote Sensing in wide coverage”
Furthermore, we will see about, how to collect the remote sensing data in real-time . We would like to share the victories about our guidance and project ideas on emerging technology . As we have the benchmark remarks on our guidance in researches and projects for the scholars and the college students we render the best ideologies in the project topics in the current technical requirements.
How to collect the Remote Sensing Data?
- Seaborne – Devices SONAR
- Satellite Sensors – INSAT and Landsat satellites
- Airborne Devices – Air crafts and Drones
- Handheld Devices- Spectrometer, camera, and Transmissometer
These are some of the platforms for acquiring remotely sensed data . We are liable to make the note of our expert’s working flow . They are explained below for your understanding.
Our Experts Working Flow
- Collecting the satellite images and understanding the same
- Pointing the illustration for the ground analysis sample
- Examining and reporting using novel algorithms
- Compare the performance of the remote sensing techniques by more scenarios
- Experiments conducted for different scenarios and also parameters
This is how our experts do the working on remote sensing in general . At this time, research ideas will be resulted in tremendous ways by deploying the right working module. In addition to we are the company with clear ideas and working flows for effective results . The next step is all about an overview of the Project Topics on GIS and Remote Sensing in detail.
Overview of GIS and Remote Sensing
Remote Sensing
- Remote sensing is a tool that is collecting data about the earth’s surface with the help of sensors in satellites or aircraft.
- There are two types of sensors is existing they are active and passive sensors
- Active sensors represent the internal inducements following the earth surface produce energies (laser-beam remote sensing system)
- Passive sensors represent the external inducements that collect the energy produced by the earth surface
- For best instance remote sensing in agriculture will lead to soil management and insects issues and so on
GIS (Geographical Information System)
- The GIS is an effective tool that is integrated with remote sensing for the visualization of the geographic status of the earth
- This is the tool designed for the support of remote sensing in the fields like recording, managing, examining, and visualizing the spatial information
So far we had seen how to collect the remotely sensed images and the overview of the Geographical Information System and the Remote Sensing in detail. Now is the time to know about the working flow of the GIS and remote sensing.
Workflow for GIS and Remote Sensing
- Examining the various (multi) scenes by spectral values
- Acquire data in a geographical location with the use of the following attributes
- Time series
- Multi-image
- Climatic changes
- Same sensors for the spatial and spectral resolution
- This is done based on the multiple scenes that arise in the study
- Collects remote sensing data and gathered it to generate the datasets.
- Next, involves radiometric, atmospheric, and ortho corrections
- The surface is subject to the comparison of the various location, research phases that indulge in more than one scenery capture
- Finally, classify the object or scene for further processing
As of now, we had seen the categorical classifications of the GIS and Remote Sensing in detail. We strongly suggest you have an interaction with our experts for the best outcomes of your research and projects in networking and emerging technologies . Today, we are very happy to transfer our knowledge to you in the sense of research and project guidance. We are always there for you to help in this aspect. Without wasting time let us discuss the current trends on GIS and Remote Sensing .
Current Trends in GIS and Remote Sensing
- This represents the flow of the ocean, temperature, icebergs, and the wavelengths by the observation of the deep seas
- This will help us to maintain the oceans in an effective manner
- This observes the land uses, wildlife environments and point the swamplands
- This will lead us to take safety measures with predetermined methods to protect the environment from pollution
- This will help us to take safety measures in the seashore changes by gathering data on residue transportation through the seas and plotting the sea characteristics
- This is the assessment that identifies the erosions, storms, volcanic activity, and flooding
- The gathered data will be used to take preventive measures before and after exploitations of the hazard events
“Our knowledge hulks are always delighted to assist you in the fields of emerging technology facts and ideologies”
In the emerging technical world, one must know about the programming involved in the Geographic Information System . This is the growing technology; it will enrich one’s career growth abundantly. Every individual can understand this GIS because it doesn’t require a wide knowledge of programming project topics on GIS and remote sensing . We had written this article to the GIS subject matter experts who want to explore more in this field . In this regard, we will see about the programming languages used in remote sensing projects.
Programming languages for Remote Sensing Projects
- Remote Sensing with Python
In the following, an explanation of programming languages is described.
- JavaScript is widely used in every web customization because it is the core language used in it
- Location mapping apps make use of the javascript for the effective results
- The acronym stands for Structured Query Language
- This facilitates to make an influence in the geospatial database
- We can make an influence in the python libraries as they are open-source data that will sink accurately
- It is habitually utilized in the Geographical Information System as its programming language
- C++ and the .NET programming languages are capable of the window-based operating systems instead of Linux and Unix operating systems
- This is a free language which is intended for the examination of the geospatial, visualizations, and arithmetical based computation
- This is the well-developed programming language used in the application creation
- This is the substitute for the above-mentioned languages
This is especially meant for the map servers for instance it is the pillar language used in the GEO map server far, we have discussed the collection of the Remote sensing data, an overview of GIS and remote sensing, workflow for GIS and remote sensing, current trends on GIS and remote sensing, and programming languages for remote sensing projects in detail.
We hope that these ideas and outlines will be very short and crisp for your better perspectives . In addition to every technology needs research for advancement hence the researchers in the industry continuously researching the emerging technology. Usually, it needs an expert’s guidance as we are having the experts who are filtered out. For any queries feel free to approach us for innovative project topics on GIS and Remote Sensing.
“We are a delight to serve you in the fields of research projects that are emerging in recent technology”
Now we will discuss the tools that are available for the GIS and remote sensing projects wide. Without wasting the time we will move to the next phase. They are mentioned by our expert’s experiments in real-time.
Tools for GIS and Remote Sensing projects
- RTool (open-source tool)
- MATLAB (commercial tool)
- Python (open-source tool)
These can be used following their features. Matlab is a commercial remote sensing tool that is specially designed for effective graphical visualizations . R is the open-source and free remote sensing tool used for investigation purposes and python is widely utilized in the GIS allied errands . Every technology owes some systematic processes. The process will effectively enrich the technology . In this regard, let us see the Major process involved in the GIS and remote sensing in detail.
Major 3 Processes in GIS and Remote Sensing
Image Pre-processing
- Radiometric, atmospheric and geometric corrections will showcase the effective perspective
- This also leads the interaction with each field by enrichment of the intensity of the images, and effects
- Comparison of the fields leads to elimination of the misrepresentations
Image Enhancement
- Enriching the interpretability of the pictures produced by the sensors
- Spatial element filtering and enriching the boundaries
- Influence of the multi-images by rationing the bands, aspects, canonical aspects, and vegetation features
Image Classification
- This helps to investigate the earth conditions in the form of human-understandable mode
- Temporal pattern recognition helps to identify the changes aroused in the pixel over the era for the modulation of the features
- Spectral pattern recognition filters the radiance in each pixel for the better understanding
These are the classification of the images that are captured by the sensors . We hope that you will understand the aspects and concepts behind them. Following the ease of your understanding, our experts made this article in different real-time perspectives. Without wasting the time we will move to the theme that how to analyze the GIS and remote sensing data .
Investigation of the technology eminently plays a vital role in the effective execution of the technology!!
How to Analysis the GIS and Remote Sensing Data?
- Discovery of the resources (what are all the resources available and where?)
- Immediacy Analysis(discovering the relevant area that is apt for the choice-making)
- Evaluating the geographical areas, detachments, and route extensions
- Examining the location(for fixing the industry in the right place, warehouse implantation)
- Investigation of the network(location apps for ensuring the earlier arrival)
- Spatio-Temporal Investigation(analysing the changes made to the land in the past 20 years)
- Topography examination(analysing the most vulnerable area which is subject to the natural ruins or disasters)
This is how the developers of the GIS and remote sensing subject matter experts will analyze the research topics on GIS and Remote Sensing in general as we do. Our experts of the concern have mentioned to you the trendy project ideas in GIS and remote sensing technology.
Top 10+ Research Project Topics on GIS and Remote Sensing
- Amalgamated urban studies based on Ontologies
- Consideration of the urban transportations
- Innovative cities and semantic technologies
- Route and operation analysis
- Integration of the multimodal in urban areas
- Location discovery services in urban areas
- Urban development data by the sensors
- Reclamation of the geographic data
- Query handling and optimizing of spatial
- Spatial reasoning and designing
- Structural design of the geographical and middleware
- Spatial and temporal handling of geodata
So far we gave you the overall view of the remote sensing technology and the Geographical Information System in wide coverage. Without a doubt, we hope that this article will ensure the best perspectives in real-time. Reach us if you are interested in implementing research project topics on GIS and Remote Sensing . Thanks for reading.
Happy researching and learning with our effective research and project assistance. We are there for you to transfer our knowledge!!
- Project Topics on GIS and Remote Sensing
Information
- Author Services
Initiatives
You are accessing a machine-readable page. In order to be human-readable, please install an RSS reader.
All articles published by MDPI are made immediately available worldwide under an open access license. No special permission is required to reuse all or part of the article published by MDPI, including figures and tables. For articles published under an open access Creative Common CC BY license, any part of the article may be reused without permission provided that the original article is clearly cited. For more information, please refer to https://www.mdpi.com/openaccess .
Feature papers represent the most advanced research with significant potential for high impact in the field. A Feature Paper should be a substantial original Article that involves several techniques or approaches, provides an outlook for future research directions and describes possible research applications.
Feature papers are submitted upon individual invitation or recommendation by the scientific editors and must receive positive feedback from the reviewers.
Editor’s Choice articles are based on recommendations by the scientific editors of MDPI journals from around the world. Editors select a small number of articles recently published in the journal that they believe will be particularly interesting to readers, or important in the respective research area. The aim is to provide a snapshot of some of the most exciting work published in the various research areas of the journal.
Original Submission Date Received: .
- Active Journals
- Find a Journal
- Proceedings Series
- For Authors
- For Reviewers
- For Editors
- For Librarians
- For Publishers
- For Societies
- For Conference Organizers
- Open Access Policy
- Institutional Open Access Program
- Special Issues Guidelines
- Editorial Process
- Research and Publication Ethics
- Article Processing Charges
- Testimonials
- Preprints.org
- SciProfiles
- Encyclopedia
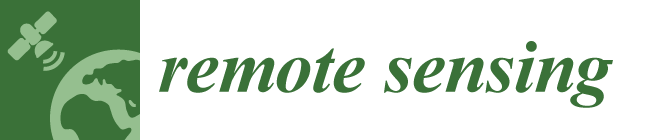
Article Menu
- Subscribe SciFeed
- Google Scholar
- on Google Scholar
- Table of Contents
Find support for a specific problem in the support section of our website.
Please let us know what you think of our products and services.
Visit our dedicated information section to learn more about MDPI.
JSmol Viewer
Remote sensing and geospatial approaches for studying the environment affected by human activities.

1. Introduction
2. overview of the published contributions, 3. conclusions, conflicts of interest.
- Yue, F.; Li, X.; Huang, Q.; Li, D. A Framework for the Construction of a Heritage Corridor System: A Case Study of the Shu Road in China. Remote Sens. 2023 , 15 , 4650. [ Google Scholar ] [ CrossRef ]
- Wang, W.; Tang, J.; Zhang, N.; Wang, Y.; Xu, X.; Zhang, A. Spatiotemporal Pattern of Invasive Pedicularis in the Bayinbuluke Land, China, during 2019–2021: An Analysis Based on PlanetScope and Sentinel-2 Data. Remote Sens. 2023 , 15 , 4383. [ Google Scholar ] [ CrossRef ]
- Li, X.; Zhou, J.; Huang, Y.; Wang, R.; Lu, T. Quantifying Water Impoundment-Driven Air Temperature Changes in the Dammed Jinsha River, Southwest China. Remote Sens. 2023 , 15 , 4280. [ Google Scholar ] [ CrossRef ]
- Jiang, Y.; Liao, L.; Luo, H.; Zhu, X.; Lu, Z. Multi-Scale Response Analysis and Displacement Prediction of Landslides Using Deep Learning with JTFA: A Case Study in the Three Gorges Reservoir, China. Remote Sens. 2023 , 15 , 3995. [ Google Scholar ] [ CrossRef ]
- Chen, Q.; Zhang, H.; Xu, B.; Liu, Z.; Mao, W. Accessing the Time-Series Two-Dimensional Displacements around a Reservoir Using Multi-Orbit SAR Datasets: A Case Study of Xiluodu Hydropower Station. Remote Sens. 2022 , 15 , 168. [ Google Scholar ] [ CrossRef ]
- Wang, Y.; He, Y.; Li, J.; Jiang, Y. Evolution simulation and risk analysis of land use functions and structures in ecologically fragile watersheds. Remote Sens. 2022 , 14 , 5521. [ Google Scholar ] [ CrossRef ]
- Yang, Z.; Zou, L.; Xia, J.; Qiao, Y.; Cai, D. Inner dynamic detection and prediction of water quality based on CEEMDAN and GA-SVM models. Remote Sens. 2022 , 14 , 1714. [ Google Scholar ] [ CrossRef ]
- Li, J.; Qin, T.; Zhang, C.; Zheng, H.; Guo, J.; Xie, H.; Zhang, C.; Zhang, Y. A New Method for Quantitative Analysis of Driving Factors for Vegetation Coverage Change in Mining Areas: GWDF-ANN. Remote Sens. 2022 , 14 , 1579. [ Google Scholar ] [ CrossRef ]
- Fu, J.; Zhang, Q.; Wang, P.; Zhang, L.; Tian, Y.; Li, X. Spatio-temporal changes in ecosystem service value and its coordinated development with economy: A case study in Hainan Province, China. Remote Sens. 2022 , 14 , 970. [ Google Scholar ] [ CrossRef ]
- Chen, X.; Zhao, W.; Chen, J.; Qu, Y.; Wu, D.; Chen, X. Mapping large-scale forest disturbance types with multi-temporal CNN framework. Remote Sens. 2021 , 13 , 5177. [ Google Scholar ] [ CrossRef ]
- Li, Q.; Guo, J.; Wang, F.; Song, Z. Monitoring the characteristics of ecological cumulative effect due to mining disturbance utilizing remote sensing. Remote Sens. 2021 , 13 , 5034. [ Google Scholar ] [ CrossRef ]
- Chen, C.; Chen, H.; Liang, J.; Huang, W.; Xu, W.; Li, B.; Wang, J. Extraction of water body information from remote sensing imagery while considering greenness and wetness based on Tasseled Cap transformation. Remote Sens. 2022 , 14 , 3001. [ Google Scholar ] [ CrossRef ]
The statements, opinions and data contained in all publications are solely those of the individual author(s) and contributor(s) and not of MDPI and/or the editor(s). MDPI and/or the editor(s) disclaim responsibility for any injury to people or property resulting from any ideas, methods, instructions or products referred to in the content. |
Share and Cite
Zhang, C.; Li, J.; Shen, X.; Wu, Q. Remote Sensing and Geospatial Approaches for Studying the Environment Affected by Human Activities. Remote Sens. 2024 , 16 , 3364. https://doi.org/10.3390/rs16183364
Zhang C, Li J, Shen X, Wu Q. Remote Sensing and Geospatial Approaches for Studying the Environment Affected by Human Activities. Remote Sensing . 2024; 16(18):3364. https://doi.org/10.3390/rs16183364
Zhang, Chengye, Jun Li, Xinyi Shen, and Qiusheng Wu. 2024. "Remote Sensing and Geospatial Approaches for Studying the Environment Affected by Human Activities" Remote Sensing 16, no. 18: 3364. https://doi.org/10.3390/rs16183364
Article Metrics
Article access statistics, further information, mdpi initiatives, follow mdpi.
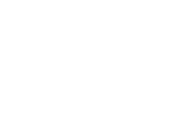
Subscribe to receive issue release notifications and newsletters from MDPI journals
- Search Close search
- Find a journal
- Search calls for papers
- Journal Suggester
- Open access publishing
We’re here to help
Find guidance on Author Services
Open access
Towards precision irrigation management: A review of GIS, remote sensing and emerging technologies
- Cite this article
- https://doi.org/10.1080/23311916.2022.2100573
1. Introduction
2. review methodology, 3. review findings and discussions, 4. opportunities and challenges of gis and rs technologies, 5. future prospects and emerging technologies for precision irrigation management, 6. conclusion, acknowledgements, disclosure statement, additional information.
- Full Article
- Figures & data
- Reprints & Permissions
- View PDF PDF View EPUB EPUB
Irrigation is the artificial application of water to crops to supply moisture. With rising drought indices and rapid population expansion, the need for irrigation water for food production is growing. Nonetheless, irrigated agriculture is battling several issues that have resulted in poor performance, inefficient water usage, and low crop water production. Improving water use efficiency in irrigated agriculture necessitates using technology that decreases water losses, matches available supplies to demand, and tracks performance. Geographical Information Systems (GIS) and Remote Sensing (RS) allow for effectively managing water and land resources for irrigation. Current GIS and RS uses in irrigation systems are covered in this study, covering land suitability for irrigation, crop water needs, irrigation scheduling, performance evaluation, and other related applications. The future potential of GIS and RS applications for sustainable irrigation water management are highlighted. This paper offers relevant information for researchers, irrigators, and policymakers on using GIS and RS in irrigation water management and how technological improvements will change irrigation water management to enhance water usage efficiency.
- data-driven
- precision irrigation
- machine learning
- water use efficiency
PUBLIC INTEREST STATEMENT
This research discusses the uses of geographic information systems and remote sensing in irrigation systems. We describe that these tools have been used to discover good agricultural locations, build maps for irrigated regions, determine crop water needs, evaluate irrigation system performance, and make judgments on the map. In addition, we show additional developing technologies, such as IoT, UAV, Big Data, and Machine Learning, which are being coupled with GIS and RS to increase precision irrigation management. With detailed research, we demonstrate that water use efficiency may be enhanced once these technologies are integrated into irrigation systems.
Climate change, particularly protracted drought, has resulted in poor agricultural production, particularly in arid and semi-arid countries. As a result, the use of irrigation systems to adapt to the impacts of climate change has increased. Irrigation contributes about 40 % of the world’s food production from 25 % of the worlds’ land area (FAO, Citation 2020 ). Irrigation ensures the continuous production of food, especially in arid and semi-arid lands of the world. Nevertheless, irrigation is a significant water user sector, as it accounts for 70 % of all freshwater withdraws, of which 60 % is lost in evaporation, seepage and deep percolation (Ungureanu et al., Citation 2020 ). Many countries are experiencing water scarcity, making it difficult to practice irrigation. Increasing drought indices in most parts of the world coupled with rapid population growth threatens the future availability of food and water resources (FAO, Citation 2018a ).
Irrigated agriculture faces tremendous challenges ranging from poor management, deteriorating infrastructure, lack of participation in decision-making, sedimentation of reservoirs (Iradukunda & Bwambale, Citation 2021 ) and poor irrigation scheduling (Kywe & Aye, Citation 2019 ). This has led to the poor performance of most surface irrigation schemes in Africa. On the other hand, pressurized irrigation systems like drip and sprinkler systems are associated with pipe leakages, component failures, high labour costs and variability of soil characteristics leading to spatial variation in irrigation (Belaud et al., Citation 2020 ). Therefore, sustainable management of irrigation systems using robust and holistic tools and software is necessary to improve water use efficiency and productivity (Bwambale et al., Citation 2019a ).
Geographical Information Systems (GIS) have evolved to address various spatial and temporal issues in irrigation and water resource management. GIS is capable of capturing, storing, manipulating, managing, and analyzing geographically referenced datasets and producing output. As a result, it is an essential tool in resource allocation, modelling, optimization, and decision-making processes. Gebeyehu ( Citation 2019 ) recognized GIS and RS as effective and powerful tools for detecting land cover and land use change. In combination with other tools, GIS has been used to understand plant health status, pest infestation, potential yield, soil conditions, crop identification, crop area estimation and agricultural water management (Gebeyehu, Citation 2019 ).
1.1. An overview of geographical information systems and remote sensing
Figure 1. GIS capabilities.

Furthermore, geographic information systems are utilized for the collecting, storage, and analysis of objects and phenomena when the geographical position is an essential property or vital to the research. GIS is a better information system due to its spatial searching and overlay capabilities. GIS is a sophisticated and effective planning tool for resource managers and decision-makers in calculating and analyzing large datasets in a short amount of time. Because of these characteristics, GIS has been used in a wide range of industries, including urban and rural planning, natural resource management, military, business, and agriculture.
Remote sensing is the art, science and technology of obtaining information about objects especially using aircraft or satellites (NOAA, Citation 2021 ). Remote sensors collected data by detecting energy from the earth. The energy can be light or any other form of electromagnetic radiation, force fields, or acoustic energy (Tempfli et al., Citation 2009 ). A remote sensor can be a conventional camera. Light reflected from an object passes through the lens and the light-sensitive film detects it, and a latent image is recorded. A photograph is then developed and generated in the photo lab for interpretation (Tempfli et al., Citation 2009 ). RS defined as above, is applied in many fields, including architecture ( Citation 2021 ), archaeology (Villa, Citation 2011 ), medicine (Suzuki et al., Citation 2012 ), industrial quality control (Barsi et al., Citation 2019 ), robotics (Guo & Wang, Citation 2012 ), extra-terrestrial mapping (Nina et al., Citation 2021 ), etc.
In agriculture, RS and GIS have enabled the spatio-temporal visualization of field information, allowing for monitoring crop and animal real-time conditions. GIS has been used in irrigation for a variety of purposes, including agricultural watershed management (Rudra et al., Citation 1993 ), hydrology (Y. Chen et al., Citation 2004 ), water productivity estimation (Tantawy, Citation 2019 ), irrigation delivery monitoring (Rowshon et al., Citation 2018 ), groundwater assessment (Megahed, Citation 2020 ; Bhunia et al., Citation 2018 ), calculating water demand (Santos et al., Citation 2008 ), spatiotemporal variations of water requirements (Kyagulanyi et al., Citation 2016 ).
(Rudra et al., Citation 1993 ), hydrology (Y. Chen et al., Citation 2004 ), water productivity estimation (Tantawy, Citation 2019 ), Related Research
One of the motivations for this systematic literature review is to develop a rich and comprehensive understanding of the existing application domains of GIS and RS in irrigation systems. Specifically, concerning the application of GIS and RS in irrigation, to the best of our knowledge, there are very few Systematic Literature Reviews (SLR) available.
The most recent review papers in the realm of geospatial technologies in irrigation are by Maina et al. ( Citation 2014 ). The authors review the literature on web GIS-based support services for irrigation management in the study. In our view, the study presents valuable information for researchers on the different tools and future developments of web GIS in irrigation water management. Similarly, Saroj et al. ( Citation 2014 ) reviewed the use of geographical information systems in irrigation water management. The authors suggested that future research should concentrate on building GIS tools that deal with the unpredictability of spatial and temporal data. However, information technology is fast evolving, and various irrigation tools have subsequently been created. A few reviews on remote sensing, particularly remote sensing applications in agriculture, have been published. (Karthikeyan et al., Citation 2020 ), application of remote sensing in precision agriculture (Sishodia et al., Citation 2020 ), and remote sensing for agricultural crops (Alvino & Marino, Citation 2017 ). Besides this study, very few reviews have been published regarding GIS and RS applications in irrigation systems. In the study, we discuss the different application domains of GIS and RS in irrigation and give future prospects of this field.
Our SLR is unique as it discusses the potential integration of other technologies to improve irrigation water management. Emerging technologies like cloud technology, real-time data, Internet of Things (IoT), 3D GIS, Mobile GIS and Unmanned Aerial Vehicles (UAVs), Big data and Machine Learning that are improving the capabilities and horizons of GIS are discussed in relation to agricultural water management.
The Systematic Literature Review (SLR) guidelines described by Xiao and Watson ( Citation 2019 ) were followed for this study. Keywords were chosen to obtain target results to address the study questions. The following search phrase was used for seeking the most relevant literature to our research:
(``GIS” OR `` Geographical Information System” OR `` Remote Sensing” OR ``Geomatics”)
(“Irrigation” OR “Agricultural water management”).
Table 1. Number of publications obtained from databases
Figure 2. Selection criteria.

Figure 3. Publications for the SLR: a) Before duplicates are removed b) After duplicates are removed, c) Publications according to application domain d) Number of publications per year.

3.1. GIS-based irrigation suitability analysis
Suitability analysis is a technique of selecting the optimal place to locate goods or regions to conserve. The site to be selected relies on the site-specific characteristics. Various GIS applications contain suitability tools/Modelers used for performing out suitability studies. A suitability Modeler is an interactive, exploratory platform for generating and assessing a suitability model (ESRI, Citation 2021 ). The Modeler delivers analytical input at each level of the model generation process and enables for smooth back and forth movement between stages. (ESRI, Citation 2021 ).
Figure 4. Flowchart for land suitability for irrigation. adapted from (AL-Taani et al., Citation 2021 ).

Table 2. Suitability analysis studies using GIS and RS
3.2. mapping in irrigation, table 3. mapping in irrigated agriculture.
Several researchers have used this procedure to map irrigated agricultural systems. Gumma et al. ( Citation 2011 ) mapped irrigated areas in Ghana using MODIS data at 30 m and 250 resolution, Ketchum et al. ( Citation 2020 ) used Irrimapper, using machine learning approaches to determine the trends and extent of irrigation coverage in the United States and noted a 15% increase of irrigated agriculture over 33 years. Vogels et al. ( Citation 2019 ) presented an object-based approach to map irrigated agriculture in the Central Rift Valley in Ethiopia using SPOT6 imagery. The authors came to the conclusion that SPOT6 imagery and GIS mapping are beneficial for assessing spatiotemporal dynamics of irrigated (smallholder) agriculture in complicated terrain and provide a foundation for agricultural land and water management. Mapping irrigated areas and rainfed areas under the same cropping conditions was done by Jin et al. ( Citation 2016 ) in China using Normalized Difference Vegetation Index (NDVI) at a 30 × 30 m spatial resolution derived from the Chinese HJ-1A/B.
The process of measuring soil salinity entails locating hotspots in an irrigated area. The following GIS tools are necessary for soil salinity mapping: Excel, ArcMap with the spatial analyst extension, Arc Toolbox, and Arc Catalog. To generate continuous surface maps from discrete sample points, the Spatial Analyst extension is necessary. Additionally, this expansion offers a wide range of analytic and spatial modelling capabilities, such as converting features (points, lines, and polygons) to rasters, carrying out zone and neighborhood studies, and modelling and analysing both raster and vector data. ArcPad and Geostatistical Analyst are further optional programs and extensions. Using a hand-held device equipped with a GPS, ArcPad is an application used to specify and georeference the boundaries of the surveyed area The Geostatistical Analyst extension can be used to define a spatial model that will be integrated into the kriging interpolation. New trends in mapping irrigated areas involve the use of advanced technologies, object-based image classification and non-parametric machine learning algorithms, and artificial intelligence evolved in a GIS environment (Basukala et al., Citation 2017 ;; Ketchum et al., Citation 2020 ).
3.3. Determination of crop water requirements
The amount of water a crop needs to grow in wide fields under unrestrictive soil conditions, such as soil water and fertility, and to reach its maximum output potential in the given growing environment is known as the crop’s water requirements (Pereira & Alves, Citation 2013 ). Crop evapotranspiration is a product of reference evapotranspiration and the crop coefficient. Detailed information on crop water requirements can be found in R. G. Allen et al. ( Citation 1998 ).
In a GIS environment, crop water requirements are estimated spatially using an inbuilt FAO Penman-Monteith Model that estimates the reference crop evapotranspiration. Monthly crop coefficients values are associated with each crop class and a reference evapotranspiration map derived from meteorological data (Casa et al., Citation 2009 ). Several researchers have used this approach to determine crop evapotranspiration (Elnashar et al., Citation 2021 ; Fares et al., Citation 2016 ; Ramírez-Cuesta et al., Citation 2018 ; Tessema Roba et al., Citation 2021 ; Garrido-Rubio et al., Citation 2018 ). Reyes-González et al. ( Citation 2018 ) estimated crop water use using a satellite-based vegetation index in northern Mexico for four growing seasons. The authors developed crop evapotranspiration maps which were used for irrigation scheduling. Similarly, Ramírez-Cuesta et al. ( Citation 2018 ) developed a novel ArcGIS toolbox which has been useful in estimating the amount of water required by a crop by integrating a dual crop coefficient method with multi-satellite imagery. The tool is appropriate for irrigation scheduling and other applications that account for water balance in agroecosystems.
For mapping and tracking ETa (actual evapotranspiration) at the field size, remote sensing methods using satellite and aircraft photography have become more popular in recent years. For example, potential differences in spatial and temporal resolutions have proved helpful for calculating ETa based on thermal infrared (TIR) data. Additionally, a number of sensors have been extensively used for mapping ETa in irrigated agricultural areas, including Landsat-TM/OLI (60–120 m), Moderate-resolution Imaging Spectrometer (MODIS) (1 km), ASTER (90 m), and GOES (4 km). The capabilities were recently improved with the start of the ESA’s Sentinel-2 and Sentinel-3 missions. The lack of fine spatial resolution in satellite TIR pictures is a key drawback despite extensive study on ETa estimates with satellites. Furthermore, ground validation is still insufficient.
Figure 5. Remote sensing determination of evapotranspiration.

3.4. Irrigation scheduling
Table 4. gis-enabled irrigation scheduling, 3.5. irrigation systems management.
The right allocation of water resources to distribute irrigation water with variable irrigation requirements is necessary to improve irrigation scheme management. Crop water requirements may vary in space and time and using the spatial data management and utilization tools of GIS, the Spatio-temporal variation in water allocation can be harmonized (Rowshon et al., Citation 2009 ) Citation 2009 . GIS provides an effective information system of the scheme that is interactive and representative of the hierarchy of irrigation system operation. Rowshon et al. ( Citation 2009 ) developed a Rice Irrigation Management System (RIMIS) in a GIS environment. The developed system was able to provide information on the uniformity of water distribution, with maps as output, irrigation managers were able to make informed decisions in regard to the operation and management of the scheme. Likewise, Garrido-Rubio et al. ( Citation 2020 ) Citation 2020 ; Maina et al. ( Citation 2014 ); Mohd et al. ( Citation 2014 ); ; Valencia et al. ( Citation 2020 ) Citation 2020 ; and Yang et al. ( Citation 2016 ) have applied GIS and RS techniques in designing irrigation canals, visualization of water management and demand, groundwater assessment, and evaluating irrigation approaches under various environmental conditions.
3.6. Decision support systems
Table 5. gis-based decision support systems for irrigation management, 3.7. irrigation performance assessments.
The systematic observation, documenting, and interpretation of activities connected to irrigated agriculture with the goal of continual improvement may be characterized as irrigation system performance assessment (Bwambale et al., Citation 2019b ). Evaluation of irrigation scheme performance is possible when water balance and information is combined with remotely sensed evapotranspiration. Recent developments in GIS and RS make it possible to assess irrigation performance on a pixel-by-pixel basis (Taghvaeian et al., Citation 2018 ). Performance Indicators like adequacy, equity, dependability, sustainability ratio, drainage ratio and drainage uniformity were estimated by Taghvaeian et al. ( Citation 2018 ) using GIS. Nikam et al. ( Citation 2020 ) used indicators of agricultural performance, water delivery performance and physical performance using remotely sensed data and GIS to evaluate the performance of a large scale irrigation project at a disaggregated level. The authors reported that the project positively impacted agriculture and economic growth of the region, despite excessive water logging in some parts of the command area and inadequacy at the tail end. Zafar et al. ( Citation 2021 ) demonstrated that using remote sensing-derived information to complement classic canal flow data with spatially discrete field-level water use information is essential to improve equity in canal water delivery in Punjab, Pakistan. Various organizations have developed models for monitoring land and water productivity. They make use of satellite data that is input and analyzed in a GIS environment to determine land and water productivity using inbuilt algorithms (Bastiaanssen & Steduto, Citation 2017 ). FAO’s WaPOR database helps monitor agricultural water productivity using satellite data analyzed in a GIS environment (FAO, Citation 2018a , Citation 2018b ). Researchers (Blatchford et al., Citation 2018 , Citation 2020 ; Sawadogo et al., Citation 2020 ; Tantawy, Citation 2019 ) have already used this database to monitor agricultural water productivity. The authors report that the GIS-enabled database is very accurate and important in estimating water productivity in production areas where field data is unavailable. This makes GIS a very important tool in estimating the productivity of land in both rainfed and irrigated agricultural systems.
GIS and remote sensing have shown to be excellent methods for monitoring irrigated areas in various locations throughout the globe under various environmental circumstances. They offer synoptic coverage of irrigated fields in numerous spectral areas and with temporal frequencies suitable to estimate agricultural water usage at various crop development stages. Furthermore, archival data that spans several years allows for picture comparison, demonstrating evolution. Because satellite data is digital, it is extremely simple to incorporate into a GIS for synthesis or comparison with other data sources. Remote sensing reduces the cost and time required to acquire datasets compared to conventional statistical surveys, which may entail aerial photography over huge regions. As a result, GIS and remote sensing technologies have become vital in underdeveloped nations where financing for data collecting for irrigation research is limited. Moreso, remote sensing offers meaningful geographical information on the specific locations of irrigated areas rather than just totals inside arbitrary administrative subdivisions. This is vital for optimizing water distribution, measuring irrigation performance, giving irrigation schedules, enumerating environmental effect, objectively assessing irrigation water consumption and understanding irrigation dynamics (Ozdogan et al., Citation 2010 ). Finally, remote sensing may give timing data, both in terms of the number of irrigation-related vegetation peaks and the amount of time irrigation is used over the course of a year.
Difficulty distinguishing between irrigated and non-irrigated lands. In humid places, separating irrigated fields from non-irrigated plots is challenging due to significant overlap in their spectral signatures. For example, during some development phases, the fingerprints of flooded irrigated fields may overlap with those of natural wetlands, reducing mapping accuracy. Researchers have addressed these constraints by combining time information on crop planting, maturity, and harvest with spectral data. Unfortunately, the gathering of remotely sensed data is limited by the orbit and return interval of a specific satellite, thus observations are not always caught at optimal periods. The availability of optical data is also a challenge in locations with regular cloud cover, such as humid tropical and subtropical settings.
The other difficulty faced with geospatial technology is real-time irrigation scheduling. Precision irrigation management attempts to provide the plant with the optimal moisture conditions in both time and space. These geospatial technologies, particularly satellite images, are unable to provide real-time data collecting to aid with irrigation scheduling choices. This complicates matching crop water demand with supply across wide regions in time and space.
Finally, detecting agricultural fields via remote sensing is problematic because irrigated landscapes are a subtype of croplands, which have historically proven difficult to map. Agrarian fields (particularly irrigated fields) are very dynamic since each field is at a distinct stage of development and hence may be mistaken with natural land cover classes. The categorical detail of land-cover maps is often negatively proportional to their accuracy. Because agriculture is difficult to identify and map in and of itself, recognizing irrigated regions as a subclass of farming becomes much more challenging. Perhaps here is where temporal data profiles will come in handy for distinguishing irrigation from rainfed crops.
GIS and RS technologies do not work in isolation; they must be integrated with other tools to acquire, store, visualize, analyze, and output geographical data effectively. The combination of geospatial technology with advances in agricultural Internet of Things, Artificial Intelligence, 3D GIS, Mobile GIS, Big data, and Machine Learning makes collecting and processing spatiotemporal agricultural data simpler.
Figure 6. Technological advancements that will shape future irrigation systems.

5.1. Cloud technology and GIS in irrigation systems
In precision irrigation technologies, cloud computing combined with geographical information systems will be critical to ensure variable rate application. In the cloud, revolutionary algorithms and software have the potential of thoroughly analyzing field conditions to estimate spatially distributed crop water requirements (Debauche et al., Citation 2018 ). The data can then be communicated to an irrigation control to perform for actuation. The farmer then can view soil moisture status on his smartphone or a web interface and with just a click, the farmer can control the system where he/she is. This has the potential of saving irrigation water and eliminating high operational costs.
Complex computations from ET algorithms can easily be handled and data sent to a GIS-enabled smartphone in the form of irrigation water to be applied. Forcén-Muñoz et al. ( Citation 2022 ) described the Irriman Platform, a system based on cloud computing techniques, which includes low-cost wireless data loggers, capable of acquiring data from a wide range of agronomic sensors, and novel software architecture for safely storing and processing such information, making crop monitoring and irrigation management easier. The proposed platform helps agronomists optimize irrigation procedures through a web-based tool that allows them to elaborate irrigation plans and evaluate their effectiveness over crops. Similarly, Younes and Salman ( Citation 2021 ) developed a cloud-based smart irrigation system leveraging satellite data. The system provides communication interfaces to trigger irrigation scheduling in the field. The application enables building a decision-support system that allows an inexperienced farmer to practice productive farming in a semi-automated manner. Other applications include, among others, the determination of actual evapotranspiration (Laipelt et al., Citation 2021 ), assessing irrigated area expansion (Naboureh et al., Citation 2021 ), and automated mapping of cropped areas (Xiong et al., Citation 2017 )
5.2. Internet of things for irrigation management
The advent of the Internet of Things (IoT) has resulted in a paradigm shift in agricultural water management. Traditionally, farmers use heuristic approaches to schedule irrigation which oftentimes results into over or under irrigation (Kashyap et al., Citation 2021 ). Closed-loop control strategies ensure that the amount of water to be applied is determined by the current soil moisture status and prevailing crop environment. IoT provides a platform for precision irrigation, wirelessly connecting several soil sensors and other different hardware on the farm. The coupling of Geographical Information Systems with the Internet of Things is essential in the automation of irrigation systems. The combination of Geographical Information systems and IoT will bring a dynamic change in the irrigation industry and other sectors as well. IoT technology is one of those techniques in which, by using the normal internet protocol, the desired technology may wirelessly transmit the data gained by digitizing the data of the object or domain of the adapter that it is adapting to the server. With a system architecture comprising of soil moisture sensors, weather sensors and solenoids placed on sprinklers, Lora modulation and 4 G connection, real-time irrigation requirements can be met (Rehman et al., Citation 2022 ).
Recently, Abioye, Abidin, et al. ( Citation 2021 ) implemented an IoT-based monitoring and data-driven modelling of drip irrigation system for mustard leaf cultivation experiment. The authors used plant sensors to monitor water stress and weather sensors to monitor the plant environment conditions and the data was sent over a wireless sensor network. The system was able to schedule irrigation in real-time. Other applications of IoT Integrated with GIS/RS include Real-time detection of irrigation water pollution (Lin et al., Citation 2020 ), estimation of leaching requirement (Bashir et al., Citation 2020 ), irrigation scheduling for water management (Roy et al., Citation 2021 ) and irrigation management (Liu, Citation 2021 ).
5.3. Augmented reality and virtual reality
Augmented reality is a field of research that combines the real environment and computer-generated data to increase the human perception of a scene in real-time, enhancing visible details and displaying invisible or inexistent objects (Mirauda et al., Citation 2017 ). This virtual-real merge is achieved via the appropriate Graphical User Interfaces (GUIs) on the user’s hand-held portable devices (smartphones, tablets, etc.). Future outdoor activities will be transformed by augmented reality. It gives mobile field personnel a handy tool for visualizing their surroundings, providing related information, and reducing the need to read maps and documentation while monitoring and managing operations. This will assist in bridging the gap between GIS data on a PC that is not helpful to field employees in real-time. An irrigator may use augmented reality to aim a phone camera at the ground and observe the position and direction of water pipelines and power wires buried under the earth. The AR software uses the GIS system and the irrigator’s position to visually represent the infrastructure underneath. This assists in detecting leaks in subsurface drip irrigation systems and subterranean conveyance pipelines.
3D GIS adds another dimension(z) to objects for greater details and visibility 3D GIS adds an elevation component to objects that 2D maps are unable to provide. 3D technologies in GIS maps gives explanatory illustrations to represent the scale of real-world objects (Jacob, Citation 2019 ). The models in three dimensions result in a great appearance, aiding surveying in different domains. Integrating 3D technologies can help irrigation engineers envision, evaluate and analyze how certain changes in irrigation layouts will look like, and how sustainable the changes are. A typical 3D model would consist of irrigation infrastructure information, satellite imagery and data to help irrigation designers decide on the most economical routes for irrigation conveyance systems. In ArcGIS, it is possible to integrate 2D GIS and 3D GIS for greater visualization and simulations of irrigation systems (Yang et al., Citation 2016 ). With this integration, it is possible to assess the impact of flooding on irrigated agriculture in 3D using GIS 3D spatial analysis tools.
By using 3D geographic information system (3D GIS) and remote sensing (RS) technologies, Cheng et al. ( Citation 2015 ) offered a simple, practical, and cost-effective technique. 3D terrain and ground feature models were built interactively using multi-source data such as Google Earth (GE) high-resolution remote sensing images, ASTER G-DEM, hydrological facility maps, etc. After that, both models were produced with texture data and linked into the ArcGIS platform. A vibrant, realistic 3D virtual picture of an irrigation field was created with a nice visual impact and basic GIS tools for data retrieval and analysis.
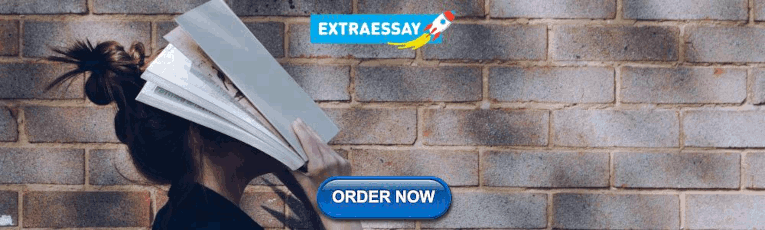
5.5 Mobile GIS
Mobile GIS makes it easier to conduct feasibility studies for irrigation development by allowing field personnel to gather, save, update, edit, analyze, and display geospatial data and information. This is made possible by integrating mobile devices, GIS software, GPS, and wireless connections that provide internet-based GIS access. With mobile GIS, it is possible to make changes and updates in real-time while collecting data, accelerating processing and increasing accuracy. Several mobile GIS applications have been developed for numerous purposes, and because to significant developments in mobile technology, these applications now go well beyond basic mapping (Mostafa, Citation 2019 ). Some of these applications were developed by private sector companies for commercial purposes. Weather forecasting, acquiring market prices, and detecting plant diseases are a few examples of mobile GIS applications purposefully designed for irrigated agricultural systems.
5.6 Unmanned aerial vehicles
An unmanned aerial vehicle (UAV) is an aircraft that carries no human pilot or passengers. UAVs, sometimes called “drones” can be fully or partially autonomous but are more often controlled remotely by a human pilot (RAND, Citation 2020 ). Unmanned aerial vehicles (UAVs) are evolving toward autonomy, choosing where to fly based on deep learning, a variety of navigational signals, and sensor data. In order to create accurate 3D models and orthophotos of a region of interest, on-demand detailed picture gathering with UAVs is a quick and affordable alternative (Giacomo & David, Citation 2018 ). UAV‐based remote sensing and GIS mapping of processed data can be used for irrigation scheduling, plant water status assessment and nutrient assessment applications. Recent studies have applied UAVs in energy balance of an irrigated field (Ortega-Farias et al., Citation 2021 ), surface moisture mapping (Zeyliger et al., Citation 2022 ) and determination of actual evapotranspiration (Mokhtari et al., Citation 2021 ). Remote sensing using unmanned aerial vehicles can provide precise information of irrigated crop areas through monitoring the phenological development of crops through multi-temporal images.
5.7 Big data and machine learning
To deliver sustainable irrigation, irrigation managers need to employ cutting edge technologies like big data, machine learning and blockchain. Data-driven- precision irrigation with these technologies is the most promising approach to solve existing and future problems (Alfred et al., Citation 2021 ; Bhat & Huang, Citation 2021 ; Kashyap et al., Citation 2021 ). Once vast data is obtained from an irrigated field, it can be used to drive irrigation and other farming decisions. Data-driven agriculture has been proven to improve crop yield, reduce cost, and ensure sustainability. The integration of GIS, RS with Big data and machine learning encourages the automation of farm data collection, analysis and decision making. GIS-based data management systems have been developed to help farmers with specific irrigation-related tasks like weather prediction, mapping, irrigation scheduling, automated field management, Big data, and Machine Learning tools and the increase in computational power has made it possible to develop algorithms from data collected from the field either by UAVs, satellites and sensors to make decisions.
Data-driven machine learning models for irrigation have gained attention (Abioye, Abidin, Aman et al., Citation 2021 ; Bwambale et al., Citation 2022 , Citation 2023 ; Lozoya et al., Citation 2014 ; McCarthy et al., Citation 2014 )) over physical/mechanistic models to predict soil moisture content because data-driven models require less data for calibration as compared to mechanistic model calibration. In addition, ML models process spatial and temporal data easily in less time and show good predictive performance over physical models. The ML model-based irrigation systems have a closed-loop controller that learns from calibration, in which the system itself employs feedback from the comparison between pre-processed data and measured real-time data (Abioye et al., Citation 2023 ).
Big data and machine learning techniques have been applied in paddy rice to ensure the optimum water level is maintained in the field. Climate data is obtained using satellites, drones ae used in monitoring water stress while a wireless sensor network is used to build a smart irrigation system for paddy. Significant increases in yields have been reported using this approach of maintaining a certain amount of water in the paddy field using a data-driven approach.
This study examines the most current uses of Geographical Information Systems in irrigation. Furthermore, developing technologies in irrigation management are highlighted. Geographical Information Systems have been used in land suitability analysis mapping, crop water consumption estimation, irrigation scheduling, productivity monitoring, and irrigation performance evaluations. Combining GIS with existing irrigation management systems is more powerful and effective when dealing with significant amounts of geographical and temporal data. It is used to organize, retrieve, and show geographical and geographic data for irrigation at the field or basin size. Despite the fact that a few tools and systems have been developed to simulate irrigation models, there is still a need for new tools to examine spatial irrigation water needs, which might be produced by altering current GIS software. Technological and application advancements in GIS will determine the future usage of these geospatial tools in irrigation systems. Integration of cloud computing, Internet of Things Big Data and Machine Learning, 3D GIS, Mobile GIS, Augmented and Virtual Reality, and Unmanned Aerial Vehicles with GIS and Remote Sensing technologies will transform agriculture and continue to play an important role in agriculture 5.0. In site-specific precision irrigation, smart irrigation systems like as drip and sprinkler irrigation are widely employed. With water supplies becoming scarce, sophisticated water management and irrigation techniques are required to conserve this valuable resource. To address under- or over-irrigation issues, as well as soil leaching or drying, requirement-based irrigation may be carried out utilizing a mix of geospatial technologies, information systems, and decision support systems.
The authors are grateful to the West African Centre for Water, Irrigation and Sustainable Agriculture (WACWISA), and the University for Development Studies.
No potential conflict of interest was reported by the author(s).
Notes on contributors

Erion Bwambale
Erion Bwambale is a PhD Candidate at the West African Center for Water, Irrigation and Sustainable Agriculture (WACWISA) hosted at the University for Development Studies in Ghana. Erion is an early-career researcher passionate about irrigation systems modelling for improved water use efficiency. He is also the Irrigation Engineer and co-founder at Irrisol Engineering Ltd, a company that provides farmers with custom-designed water and irrigation solutions in Uganda. He holds a masters degree in Civil Engineering with a specialization in Irrigation and Drainage from Jomo Kenyatta University of Agriculture and Technology, Kenya and a B.Sc in Agricultural Mechanization and Irrigation Engineering, Busitema University, Uganda. He is a Member of ASABE, ASCE, PASAE and ICID.
- Abdalla, F. (2012). Mapping of groundwater prospective zones using remote sensing and GIS techniques: A case study from the central eastern desert, Egypt. Journal of African Earth Sciences , 70 , 8–22. https://doi.org/10.1016/j.jafrearsci.2012.05.003 Web of Science ® Google Scholar
- Abioye, E. A., Abidin, M. S. Z., Mahmud, M. S. A., Buyamin, S., AbdRahman, M. K. I., Otuoze, A. O., Ramli, M. S. A., & Ijike, O. D. (2021). IoT-based monitoring and data-driven modelling of drip irrigation system for mustard leaf cultivation experiment. Information Processing in Agriculture , 8 ( 2 ), 270–283. https://doi.org/10.1016/j.inpa.2020.05.004 Google Scholar
- Abioye, E. A., Abidin, M. S. Z., Aman, M. N., Mahmud, M. S. A., & Buyamin, S. (2021). A model predictive controller for precision irrigation using discrete lagurre networks. Computers and Electronics in Agriculture , 181 ( July 2020 ), 105953. https://doi.org/10.1016/j.compag.2020.105953 Google Scholar
- Abioye, E. A., Shukri, M., Abidin, Z., Saiful, M., Mahmud, A., Buyamin, S., Denis, O., Okino, A., Abiodun, A., & Musbau, O. (2023). Smart agricultural technology a data-driven kalman filter-PID controller for fibrous capillary irrigation. Smart Agricultural Technology , 3 ( April 2022 ), 100085. https://doi.org/10.1016/j.atech.2022.100085 Google Scholar
- Abu-Hashim, M., Sayed, A., Zelenakova, M., Vranayova, Z., & Khalil, M. (2021). Soil water erosion vulnerability and suitability under different irrigation systems using parametric approach and. Sustainability (Switzerland) , 13 ( 3 ), 1057. https://doi.org/10.3390/su13031057 Web of Science ® Google Scholar
- Al-taani, A., Al-husban, Y., & Farhan, I. (2021). The Egyptian journal of remote sensing and space sciences land suitability evaluation for agricultural use using GIS and remote sensing techniques : The case study of Ma ’ an governorate, Jordan. The Egyptian Journal of Remote Sensing and Space Sciences , 24 ( 1 ), 109–117. https://doi.org/10.1016/j.ejrs.2020.01.001 Web of Science ® Google Scholar
- AL-Taani, A., Al-husban, Y., & Farhan, I. (2021). Land suitability evaluation for agricultural use using GIS and remote sensing techniques: The case study of Ma’an governorate, Jordan. Egyptian Journal of Remote Sensing and Space Science , 24 ( 1 ), 109–117. https://doi.org/10.1016/j.ejrs.2020.01.001 Web of Science ® Google Scholar
- Aldababseh, A., Temimi, M., Maghelal, P., Branch, O., & Wulfmeyer, V. (2018). Multi-criteria evaluation of irrigated agriculture suitability to achieve food security in an arid environment. Sustainability (Switzerland) , 10 ( 3 ), 803. https://doi.org/10.3390/su10030803 Web of Science ® Google Scholar
- Alfred, R., Obit, J. H., Chin, C. P. Y., Haviluddin, H., & Lim, Y. (2021). Towards paddy rice smart farming: A review on big data, machine learning, and rice production tasks. IEEE Access , 9 , 50358–50380. https://doi.org/10.1109/ACCESS.2021.3069449 Web of Science ® Google Scholar
- Allen, R. G., Pereira, L. S., Raes, D., & Smith, M. (1998). Crop evapotranspiration. guidelines for computing crop water requirements. FAO Irrigation and drainage paper 56 . Food and Agricultural Organization of the United Nations. Google Scholar
- Allen, R. G., Tasumi, M., Morse, A., Trezza, R., Wright, J. L., Bastiaanssen, W., Kramber, W., Lorite, I., & Robison, C. W. (2007). Satellite-based energy balance for mapping evapotranspiration with internalized calibration (METRIC)—applications. Journal of Irrigation and Drainage Engineering , 133 ( 4 ), 395–406. https://doi.org/10.1061/(asce)0733-9437(2007)133:4(395) Google Scholar
- Alvino, A., & Marino, S. (2017). Remote sensing for irrigation of horticultural crops. Horticulturae , 3 ( 2 ), 40. https://doi.org/10.3390/horticulturae3020040 Web of Science ® Google Scholar
- Andrade, M. A., O’Shaughnessy, S. A., & Evett, S. R. (2016). A GIS-based decision support tool for center pivot irrigation systems. 2016 American society of agricultural and biological engineers annual international meeting, ASABE 2016, December. https://doi.org/10.13031/aim.20162461449 Google Scholar
- Asfaw, E., Suryabhagavan, K. V., & Argaw, M. (2018). Soil salinity modeling and mapping using remote sensing and GIS: The case of Wonji sugar cane irrigation farm, Ethiopia. Journal of the Saudi Society of Agricultural Sciences , 17 ( 3 ), 250–258. https://doi.org/10.1016/j.jssas.2016.05.003 Google Scholar
- Barsi, Á., Kugler, Z., Juhász, A., Szabó, G., Batini, C., Abdulmuttalib, H., Huang, G., & Shen, H. (2019). Remote sensing data quality model: From data sources to lifecycle phases. International Journal of Image and Data Fusion , 10 ( 4 ), 280–299. https://doi.org/10.1080/19479832.2019.1625977 Web of Science ® Google Scholar
- Bashir, R. N., Bajwa, I. S., & Shahid, M. M. A. (2020). Internet of things and machine-learning-based leaching requirements estimation for saline soils. IEEE Internet of Things Journal , 7 ( 5 ), 4464–4472. https://doi.org/10.1109/JIOT.2019.2954738 Web of Science ® Google Scholar
- Bastiaanssen, W. G. M., Meneti, M., Feddes, R. A., & Holtslag, A. A. M. (1998, January). A remote sensing surface energy balance algorithm for land (SEBAL). Journal of Hydrology , 212-213 ( 213 ), 198–212. https://doi.org/10.1016/S0022-1694(98)00253-4 Google Scholar
- Bastiaanssen, W. G. M., & Steduto, P. (2017). The water productivity score (WPS) at global and regional level: Methodology and first results from remote sensing measurements of wheat, rice and maize. Science of the Total Environment , 575 , 595–611. https://doi.org/10.1016/j.scitotenv.2016.09.032 PubMed Web of Science ® Google Scholar
- Basukala, A. K., Oldenburg, C., Schellberg, J., Sultanov, M., & Dubovyk, O. (2017). Towards improved land use mapping of irrigated croplands: Performance assessment of different image classification algorithms and approaches. European Journal of Remote Sensing , 50 ( 1 ), 187–201. https://doi.org/10.1080/22797254.2017.1308235 Web of Science ® Google Scholar
- Bazzi, H., Baghdadi, N., Ienco, D., Hajj, M. E., Zribi, M., Belhouchette, H., Escorihuela, M. J., & Demarez, V. (2019). Mapping irrigated areas using sentinel-1 time series in Catalonia, Spain. Remote Sensing , 11 ( 15 ), 1–25. https://doi.org/10.3390/rs11151836 Web of Science ® Google Scholar
- Belaud, G., Mateos, L., Aliod, R., Buisson, M. C., Faci, E., Gendre, S., Ghinassi, G., Gonzales Perea, R., Lejars, C., Maruejols, F., & Zapata, N. (2020). Irrigation and energy: Issues and challenges. Irrigation and Drainage , 69 ( S1 ), 177–185. https://doi.org/10.1002/ird.2343 Google Scholar
- Bhat, S. A., & Huang, N. F. (2021). Big data and ai revolution in precision agriculture: Survey and challenges. IEEE Access , 9 , 110209–110222. https://doi.org/10.1109/ACCESS.2021.3102227 Web of Science ® Google Scholar
- Bhunia, G. S., Keshavarzi, A., Shit, P. K., Omran, E.-S. E., & Bagherzadeh, A. (2018). Evaluation of groundwater quality and its suitability for drinking and irrigation using GIS and geostatistics techniques in semiarid region of Neyshabur, Iran. Applied Water Science , 8 ( 6 ), 1–16. https://doi.org/10.1007/s13201-018-0795-6 Web of Science ® Google Scholar
- Blatchford, M., Stokvis, B., Galindo, A., Blatchford, M., & Hoekstra, A. Y. (2018). Database methodology : Level 3 data. The Science of the Total Environment , 653 :241–252. Issue August 2019. https://doi.org/10.1016/j.scitotenv.2018.10.311. PubMed Google Scholar
- Blatchford, M., Mannaerts, C. M., Zeng, Y., Nouri, H., & Karimi, P. Influence of spatial resolution on remote sensing-based irrigation performance assessment using WaPOR data. (2020). Remote Sensing , 12 ( 18 ), 2949. https://doi.org/10.3390/RS12182949 Web of Science ® Google Scholar
- Bwambale, E., Home, P., Raude, J., & Wanyama, J. (2019a). Development of a water allocation model for equitable water distribution at doho rice irrigation scheme, Uganda. Hydrology , 7 ( 4 ), 62. https://doi.org/10.11648/j.hyd.20190704.12 Google Scholar
- Bwambale, E., Home, P., Raude, J., & Wanyama, J. (2019b). Hydraulic performance evaluation of the water conveyance system of doho rice irrigation scheme in Uganda. Journal of Sustainable Research in Engineering , 5(December), 101–112. http://sri.jkuat.ac.ke/ojs/index.php/sri Google Scholar
- Bwambale, E., Abagale, F. K., & Anornu, G. K. (2022). Smart irrigation monitoring and control strategies for improving water use efficiency in precision agriculture : A review. Agricultural Water Management , 260 ( 107324 ), 1–12. https://doi.org/10.1016/j.agwat.2021.107324 Google Scholar
- Bwambale, E., Abagale, F. K., & Anornu, G. K. (2023). Data-driven model predictive control for precision irrigation management. Smart Agricultural Technology , 3 ( May 2022 ), 100074. https://doi.org/10.1016/j.atech.2022.100074 Google Scholar
- Campbell, J., & Shin, M. E. (2011). Essentials of G eographical I nformation Systems , 259 ( https://www.saylor.org/books/ ) ISSN:978-1-4533219-6-6 https://digitalcommons.liberty.edu/textbooks/2 . Google Scholar
- Casa, R., Rossi, M., Sappa, G., & Trotta, A. (2009). Assessing crop water demand by remote sensing and GIS for the pontina plain, central Italy. Water Resources Management , 23 ( 9 ), 1685–1712. https://doi.org/10.1007/s11269-008-9347-4 Web of Science ® Google Scholar
- Chen, Y., Takara, K., Cluckie, I., & De Smedt, F. H. (2004). GIS and remote sensing in hydrology, water resources and environment. In IAHS Series of Proceedings and Reports (Vol. 289, Issue January 2004). Google Scholar
- Chen, Z., Wang, J., Sun, J., Duan, A., Liu, Z., Song, N., & Liu, X. (2012). Water-saving irrigation management and decision support system based on WEBGIS. IFIP Advances in Information and Communication Technology , 368 AICT ( Part 1 ), 301–312. https://doi.org/10.1007/978-3-642-27281-3_36 Google Scholar
- Cheng, S., Dou, M., Wang, J., Zhang, S., & Chen, X. (2015). Approach to constructing 3d virtual scene of irrigation area using multi-source data. ISPRS Annals of the Photogrammetry, Remote Sensing and Spatial Information Sciences , 2 ( 2W2 ), 227–233. https://doi.org/10.5194/isprsannals-II-2-W2-227-2015 Google Scholar
- Chikabvumbwa, S. R., Sibale, D., & Chisale, S. W. (2021). Irrigation site selection using hybrid GIS-based approach. International Journal F Human Capital in Urban Management , 6 ( 2 ), 149–158. https://doi.org/10.22034/IJHCUM.2021.02.04 Google Scholar
- Corwin, D. L., & Scudiero, E. (2019). Review of soil salinity assessment for agriculture across multiple scales using proximal and/or remote sensors. In Advances in agronomy (Vol. 158 , pp. 1–130). . https://doi.org/10.1016/bs.agron.2019.07.001 Web of Science ® Google Scholar
- Debauche, O., El Moulat, M., Mahmoudi, S., Manneback, P., & Lebeau, F. (2018). Irrigation pivot-center connected at low cost for the reduction of crop water requirements. Proceedings - 2018 International conference on advanced communication technologies and networking, commnet 2018, 1–9. https://doi.org/10.1109/COMMNET.2018.8360259 Google Scholar
- Djuraev, A., Mirdjalalov, D., Nuratdinov, A., & Khushvaktov, T. (2021). Evaluation of soil salinity level through NDVI in Syrdarya province, Uzbekistan. E3S Web of Conferences, 03017. Google Scholar
- El Baroudy, A. A., Ali, A. M., Mohamed, E. S., Moghanm, F. S., Shokr, M. S., Savin, I., Poddubsky, A., Ding, Z., Kheir, A. M. S., Aldosari, A. A., Elfadaly, A., Dokukin, P., & Lasaponara, R. (2020). Modeling land suitability for rice crop using remote sensing and soil quality indicators: The case study of the Nile delta. Sustainability (Switzerland) , 12 ( 22 ), 1–25. https://doi.org/10.3390/su12229653 PubMed Web of Science ® Google Scholar
- Elnashar, A., Abbas, M., Sobhy, H., & Shahba, M. (2021). Crop water requirements and suitability assessment in arid environments: A new approach. Agronomy , 11 ( 2 ), 260. https://doi.org/10.3390/agronomy11020260 Google Scholar
- ESRI. (2021). The general suitability modeling workflow—ArcGIS Pro | Documentation . https://pro.arcgis.com/en/pro-app/latest/help/analysis/spatial-analyst/suitability-modeler/the-general-suitability-modeling-workflow.htm Google Scholar
- ESRI. (2022, February 26). What is GIS? | Geographic Information System Mapping Technology . https://www.esri.com/en-us/what-is-gis/overview Google Scholar
- FAO. (2018a). The future of food and agriculture – Alternative pathways to 2050 . http://www.fao.org/3/I8429EN/i8429en.pdf Google Scholar
- FAO. (2018b). WaPOR Database methodology: Level 1 data. In Remote Sensing for Water Productivity Technical Report: Methodology Series .September). http://www.fao.org/fileadmin/user_upload/faoweb/RS-WP/pdf_files/Web_WaPOR-beta_Methodology_document_Level1.pdf Google Scholar
- FAO. (2020). Irrigation Management . https://pdf4pro.com/download/irrigation-management-aims-at-increasing-food-23697e.html#google_vignette Google Scholar
- Fares, A., Awal, R., Fares, S., Johnson, A. B., & Valenzuela, H. (2016). Irrigation water requirements for seed corn and coffee under potential climate change scenarios. Journal of Water and Climate Change , 7 ( 1 ), 39–51. https://doi.org/10.2166/wcc.2015.025 Web of Science ® Google Scholar
- Forcén-Muñoz, M., Pavón-Pulido, N., López-Riquelme, J. A., Temnani-Rajjaf, A., Berríos, P., Morais, R., & Pérez-Pastor, A. (2022). Irriman platform: Enhancing farming sustainability through cloud computing techniques for irrigation management. Sensors , 22 ( 1 ), 228. https://doi.org/10.3390/s22010228 Google Scholar
- Fortes, P. S., Platonov, A. E., & Pereira, L. S. (2005). GISAREG - A GIS based irrigation scheduling simulation model to support improved water use. Agricultural Water Management , 77 ( 1–3 ), 159–179. https://doi.org/10.1016/j.agwat.2004.09.042 Web of Science ® Google Scholar
- Fue, K. G., & Sanga, C. (2015). Remote scheduling system for drip irrigation system using geographic information system. Journal of Geographic Information System , 7 ( 5 ), 551–563. https://doi.org/10.4236/jgis.2015.75044 Google Scholar
- Garrido-Rubio, J., Calera Belmonte, A., Fraile Enguita, L., Arellano Alcázar, I., Belmonte Mancebo, M., Campos Rodríguez, I., & Bravo Rubio, R. (2018). Remote sensing-based soil water balance for irrigation water accounting at the Spanish Iberian Peninsula. Proceedings of the international association of hydrological sciences, 380 , 29–35. https://doi.org/10.5194/piahs-380-29-2018 Google Scholar
- Garrido-Rubio J, Calera A, Arellano I, Belmonte M, Fraile L, Ortega T, Bravo R and González-Piqueras J. (2020). Evaluation of Remote Sensing-Based Irrigation Water Accounting at River Basin District Management Scale. Remote Sensing , 12 ( 19 ), 3187 10.3390/rs12193187 Web of Science ® Google Scholar
- Gebeyehu, M. N. (2019). Remote sensing and GIS application in agriculture and natural resource management. International Journal of Environmental Sciences & Natural Resources , 19 ( 2 ), 556009. https://doi.org/10.19080/IJESNR.2019.19.556009 Google Scholar
- Giacomo, R., & David, G. (2018). Gerard , Sylvester. In E-agriculture in action: Drones for agriculture . FAO and ITU 978-92-5-130246-0s https://www.fao.org/3/I8494EN/i8494en.pdf . Google Scholar
- Gorji, T., Tanik, A., & Sertel, E. (2015). Soil salinity prediction, monitoring and mapping using modern technologies. Procedia Earth and Planetary Science , 15 , 507–512. https://doi.org/10.1016/j.proeps.2015.08.062 Google Scholar
- Govind, A. (2021). Physics of remote sensing and sensor characteristics : A Primer . Remote Sensing Determination of Evapotranspiration. Google Scholar
- Gumma, M. K., Thenkabail, P. S., Hideto, F., Nelson, A., Dheeravath, V., Busia, D., & Rala, A. (2011). Mapping irrigated areas of Ghana using fusion of 30 m and 250 m resolution remote-sensing data. Remote Sensing , 3 ( 4 ), 816–835. https://doi.org/10.3390/rs3040816 Web of Science ® Google Scholar
- Guo, F., & Wang, Y. (2012). Study of remote sensing and remote sensing robot technology. Applied Mechanics and Materials , 214 , 914–918. https://doi.org/10.4028/www.scientific.net/AMM.214.914 Google Scholar
- Huisman, O. (2009). . In H. Otto & de B. Rolf A (Eds.), Principles of geographical information systems (Vol. 1 ITC Educational Textbook Series). H. Otto & de B. Rolf A (Eds.). The International Institute for Geo-Information Science and Earth Observation (ITC). Google Scholar
- Iradukunda, P., & Bwambale, E. (2021). Reservoir sedimentation and its effect on storage capacity–A case study of murera reservoir, Kenya. Cogent Engineering , 8 ( 1 ), 0–14. https://doi.org/10.1080/23311916.2021.1917329 Web of Science ® Google Scholar
- Jacob, A. (2019, June 26). What is 3D GIS and what are its uses? – Geospatial World . GeoSpatial World Blogs. https://www.geospatialworld.net/blogs/what-is-3d-gis-and-what-are-its-uses/ Google Scholar
- Jasmin, I., & Mallikarjuna, P. (2015). Evaluation of groundwater suitability for irrigation in the araniar river basin, south India — a case study using gis approach †. Irrigation and Drainage , 64 ( 5 ), 600–608. https://doi.org/10.1002/ird.1930 Web of Science ® Google Scholar
- Jin, N., Tao, B., Ren, W., Feng, M., Sun, R., He, L., Zhuang, W., & Yu, Q. (2016). Mapping irrigated and rainfed wheat areas using multi-temporal satellite data. Remote Sensing , 8 ( 207 ), 1–19. https://doi.org/10.3390/rs8030207 Google Scholar
- Karthikeyan, L., Chawla, I., & Mishra, A. K. (2020). A review of remote sensing applications in agriculture for food security: Crop growth and yield, irrigation, and crop losses. Journal of Hydrology , 586 ( March ), 124905. https://doi.org/10.1016/j.jhydrol.2020.124905 Google Scholar
- Kashyap, P. K., Kumar, S., Jaiswal, A., Prasad, M., & Gandomi, A. H. (2021). Towards precision agriculture : IoT-Enabled. IEEE Sensors Journal , 21 ( 16 ), 17479–17491. https://doi.org/10.1109/JSEN.2021.3069266 Web of Science ® Google Scholar
- Ketchum D, Jencso K, Maneta M P, Melton F, Jones M O and Huntington J. (2020). IrrMapper: A Machine Learning Approach for High Resolution Mapping of Irrigated Agriculture Across the Western U.S. Remote Sensing , 12 ( 14 ), 2328. https://doi.org/10.3390/rs12142328. Web of Science ® Google Scholar
- Kyagulanyi, J., Kabenge, I., Banadda, N., Muyonga, J., Mulamba, P., & Kiggundu, N. (2016). Estimation of spatial and temporal water requirements of grain amaranth using satellite, local and virtual weather stations datasets in Uganda. International Journal of Agricultural and Biological Engineering , 9 ( 2 ), 85–97. https://doi.org/10.3965/j.ijabe.20160902.1676 Web of Science ® Google Scholar
- Kywe, K., & Aye, N. (2019). Possible solutions to the challenges of irrigation water pricing for saedawgyi irrigated area. American Scientific Research Journal for Engineering, Technology, and Sciences ASRJETS , 52(1) , 176–188 https://core.ac.uk/display/235050773?utm_source=pdf&utm_medium=banner&utm_campaign=pdf-decoration-v1 . Google Scholar
- Laipelt, L., Henrique Bloedow Kayser, R., Santos Fleischmann, A., Ruhoff, A., Bastiaanssen, W., Erickson, T. A., & Melton, F. (2021). Long-term monitoring of evapotranspiration using the SEBAL algorithm and google earth engine cloud computing. ISPRS Journal of Photogrammetry and Remote Sensing , 178 , 81–96. https://doi.org/10.1016/j.isprsjprs.2021.05.018 Web of Science ® Google Scholar
- Li, X., Zhang, J., Liu, J., Liu, J., Zhu, A., Lv, F., & Zhang, C. (2011). A modified checkbook irrigation method based on GIS-coupled model for regional irrigation scheduling. Irrigation Science , 29 ( 2 ), 115–126. https://doi.org/10.1007/s00271-010-0221-9 Web of Science ® Google Scholar
- Lin, Y. P., Mukhtar, H., Huang, K. T., Petway, J. R., Lin, C. M., Chou, C. F., & Liao, S. W. (2020). Real-time identification of irrigation water pollution sources and pathways with a wireless sensor network and blockchain framework. Sensors (Switzerland) , 20 ( 13 ), 1–22. https://doi.org/10.3390/s20133634 Web of Science ® Google Scholar
- Liu, H. (2021). Agricultural water management based on the internet of things and data analysis. Acta Agriculturae Scandinavica Section B: Soil and Plant Science , 1–12. https://doi.org/10.1080/09064710.2021.1966496 Google Scholar
- Lozoya, C., Mendoza, C., Mejía, L., Quintana, J., Mendoza, G., Bustillos, M., Arras, O., & Solís, L. (2014). Model predictive control for closed-loop irrigation. IFAC Proceedings , 19 ( January ), 4429–4434. https://doi.org/10.3182/20140824-6-za-1003.02067 Volumes (IFAC-PapersOnline) Google Scholar
- Maina, M. M., Amin, M. S. M., & Yazid, M. A. (2014). Web geographic information system decision support system for irrigation water management: A review. Acta Agriculturae Scandinavica Section B: Soil and Plant Science , 64 ( 4 ), 283–293. https://doi.org/10.1080/09064710.2014.896935 Google Scholar
- Mandal, B., Dolui, G., & Satpathy, S. (2018). Land suitability assessment for potential surface irrigation of river catchment for irrigation development in Kansai watershed, Purulia, West Bengal, India. Sustainable Water Resources Management , 4 ( 4 ), 699–714. https://doi.org/10.1007/s40899-017-0155-y Web of Science ® Google Scholar
- McCarthy, A. C., Hancock, N. H., & Raine, S. R. (2014). Simulation of irrigation control strategies for cotton using Model Predictive Control within the VARIwise simulation framework. Computers and Electronics in Agriculture , 101 , 135–147. https://doi.org/10.1016/j.compag.2013.12.004 Web of Science ® Google Scholar
- Megahed, H. A. (2020). GIS-based assessment of groundwater quality and suitability for drinking and irrigation purposes in the outlet and central parts of Wadi El-Assiuti, Assiut governorate, Egypt. Bulletin of the National Research Centre , 44 ( 187 ), 1–31. https://doi.org/10.1186/s42269-020-00428-3 Google Scholar
- Mirauda, D., Erra, U., Agatiello, R., & Cerverizzo, M. (2017). Applications of mobile augmented reality to water resources management. Water (Switzerland) , 9 ( 9 ), 699. https://doi.org/10.3390/w9090699 Google Scholar
- Mohammed, H. R., Alkanaani, H. A., & Alowaid, H. K. (2021). Using GIS technology for land suitability analysis to select drainage project location : Nasiriya city south of Iraq as a case study using GIS technology for land suitability analysis to select drainage project location : Nasiriya city south of Iraq as a. Journal of Physics: Conference Series, 01 ( 20). https://doi.org/10.1088/1742-6596/1895/1/012001 Google Scholar
- Mohd, M. M., Soom, M., Amin, M., Kamal, R., & Wayayok, A. (2014). Application of web geospatial decision support system for tanjung karang rice precision irrigation water management. International Conference on Agriculture, Food and Environmental Engineering (ICAFEE’2014) Jan. 15-16, 2014, 24–28. Google Scholar
- Mokhtari, A., Ahmadi, A., Daccache, A., & Drechsler, K. (2021). Actual evapotranspiration from UAV images: A multi-sensor data fusion approach. Remote Sensing , 13 ( 12 ), 2315. https://doi.org/10.3390/rs13122315 Web of Science ® Google Scholar
- Mostafa, G. (2019 Developing a Mobile GIS Application for Facilitating Information Communications in Agri-Environmental Programs. (The University of GuelphIn)Accessed 12 February 2022 https://atrium.lib.uoguelph.ca/xmlui/handle/10214/17664 ). . Google Scholar
- Naboureh, A., Li, A., Ebrahimy, H., Bian, J., Azadbakht, M., Amani, M., Lei, G., & Nan, X. (2021). Assessing the effects of irrigated agricultural expansions on Lake Urmia using multi-decadal landsat imagery and a sample migration technique within google earth engine. International Journal of Applied Earth Observation and Geoinformation , 105 25 December 2021 , 102607. https://doi.org/10.1016/j.jag.2021.102607 Google Scholar
- Narany, T. S., Ramli, M. F., Fakharian, K., & Aris, A. Z. (2016). A GIS-index integration approach to groundwater suitability zoning for irrigation purposes. Arabian Journal of Geosciences , 9 ( 7 ), 502. https://doi.org/10.1007/s12517-016-2520-9 Google Scholar
- Narziev, J., Nikam, B., & Gapparov, F. (2021). Infrastructure mapping and performance assessment of irrigation system using GIS and remote sensing. E3S Web of Conferences, 03005. Google Scholar
- Ng Cheong, L. R., & Teeluck, M. (2018). Development of an irrigation scheduling software for sugarcane. Sugar Tech , 20 ( 1 ), 36–39. https://doi.org/10.1007/s12355-017-0517-7 Web of Science ® Google Scholar
- Nigussie, W., Hailu, B. T., & Azagegn, T. (2019). Mapping of groundwater potential zones using sentinel satellites (−1 SAR and −2A MSI) images and analytical hierarchy process in ketar watershed, main Ethiopian rift. Journal of African Earth Sciences 160 , 103632. https://doi.org/10.1016/j.jafrearsci.2019.103632 Web of Science ® Google Scholar
- Nikam, B. R., Garg, V., Thakur, P. K., & Aggarwal, S. P. (2020). Application of remote sensing and GIS in performance evaluation of irrigation project at disaggregated level. Journal of the Indian Society of Remote Sensing , 48 ( 7 ), 979–997. https://doi.org/10.1007/s12524-020-01128-1 Web of Science ® Google Scholar
- Nina, A., Radovanović, M., & Popović, L. (2021). Extraterrestrial influences on remote sensing in the earth’s atmosphere. Remote Sensing , 13 ( 5 ), 1–4. https://doi.org/10.3390/rs13050890 Web of Science ® Google Scholar
- NOAA. (2021, February 26). What is remote sensing? https://oceanservice.noaa.gov/facts/remotesensing.html Google Scholar
- Ortega-Farias, S., Esteban-Condori, W., Riveros-Burgos, C., Fuentes-Peñailillo, F., & Bardeen, M. (2021). Evaluation of a two-source patch model to estimate vineyard energy balance using high-resolution thermal images acquired by an unmanned aerial vehicle (UAV). Agricultural and Forest Meteorology 304-305 , January 108433. https://doi.org/10.1016/j.agrformet.2021.108433 Web of Science ® Google Scholar
- Owusu, S., Mul, M. L., Ghansah, B., Kofi, P., Owusu, O., Awotwe, V., & Davie, P. (2017). Assessing land suitability for aquifer storage and recharge in northern Ghana using remote sensing and GIS multi-criteria decision analysis technique. Modeling Earth Systems and Environment , 3 ( 4 ), 1383–1393. https://doi.org/10.1007/s40808-017-0360-6 Web of Science ® Google Scholar
- Ozdogan, M., Yang, Y., Allez, G., & Cervantes, C. (2010). Remote sensing of irrigated agriculture: Opportunities and challenges. Remote Sensing , 2 ( 9 ), 2274–2304. https://doi.org/10.3390/rs2092274 Web of Science ® Google Scholar
- Patra, S., Mishra, P., & Mahapatra, S. C. (2018). Delineation of groundwater potential zone for sustainable development: A case study from ganga alluvial plain covering hooghly district of India using remote sensing, geographic information system and analytic hierarchy process. Journal of Cleaner Production , 172 , 2485–2502. https://doi.org/10.1016/j.jclepro.2017.11.161 Web of Science ® Google Scholar
- Paul, M., Negahban-Azar, M., Shirmohammadi, A., & Montas, H. (2020). Assessment of agricultural land suitability for irrigation with reclaimed water using geospatial multi-criteria decision analysis. Agricultural Water Management , 231 (April 2019):105987. https://doi.org/10.1016/j.agwat.2019.105987. Web of Science ® Google Scholar
- Peragón, J. M., Pérez-Latorre, F. J., Delgado, A., & Tóth, T. (2018). Best management irrigation practices assessed by a GIS-based decision tool for reducing salinization risks in olive orchards. Agricultural Water Management , 202 ( July 2017 ), 33–41. https://doi.org/10.1016/j.agwat.2018.02.010 Google Scholar
- Pereira, L. S., & Alves, I. (2013). Crop water requirements. In Reference module in earth systems and environmental sciences (issue may) , 322-334. Elsevier Inc. ISBN: 978-0-12-409548-9. https://doi.org/10.1016/b978-0-12-409548-9.05129-0 Google Scholar
- Ramírez-Cuesta, J. M., Mirás-Avalos, J. M., Rubio-Asensio, J. S., & Intrigliolo, D. S. (2018). A novel ArcGIS toolbox for estimating crop water demands by integrating the dual crop coefficient approach with multi-satellite imagery. Water (Switzerland) , 11 ( 1 ), 38. https://doi.org/10.3390/w11010038 Google Scholar
- RAND. (2020, May 12). Unmanned Aerial Vehicles . https://www.rand.org/topics/unmanned-aerial-vehicles.html Google Scholar
- Rehman, A., Saba, T., Kashif, M., Fati, S. M., Bahaj, S. A., & Chaudhry, H. (2022). A revisit of internet of things technologies for monitoring and control strategies in smart agriculture. Agronomy , 12 ( 1 ), 127. https://doi.org/10.3390/agronomy12010127 Google Scholar
- Reyes-González, A., Kjaersgaard, J., Trooien, T., Hay, C., & Ahiablame, L. (2018). Estimation of crop evapotranspiration using satellite remote sensing-based vegetation index. Advances in Meteorology , 2018 ( 1 ), 1–12. https://doi.org/10.1155/2018/4525021 Web of Science ® Google Scholar
- Rowshon M, Kwok C and Lee T. (2003). GIS-based scheduling and monitoring of irrigation delivery for rice irrigation system. Agricultural Water Management , 62 ( 2 ), 105–116. https://doi.org/10.1016/S0378-3774(03)00092-1 Google Scholar
- Rowshon M K, Amin M S, Lee T S and Shariff A R. (2009). GIS-Integrated Rice Irrigation Management Information System for a River-Fed Scheme. Water Resour Manage , 23 ( 14 ), 2841–2866. https://doi.org/10.1007/s11269-009-9412-7 Web of Science ® Google Scholar
- Rowshon, M. K., Iqbal, M., Mojid, M. A., Amin, M. S. M., & Lai, S. H. (2018). Optimization of equitable irrigation water delivery for a large-scale rice irrigation scheme. International Journal of Agricultural and Biological Engineering , 11 ( 5 ), 160–166. https://doi.org/10.25165/j.ijabe.20181105.3536 Web of Science ® Google Scholar
- Roy, S. K., Misra, S., Raghuwanshi, N. S., & Das, S. K. (2021). AgriSens: IoT-Based dynamic irrigation scheduling system for water management of irrigated crops. IEEE Internet of Things Journal , 8 ( 6 ), 5023–5030. https://doi.org/10.1109/JIOT.2020.3036126 Web of Science ® Google Scholar
- Rudra, R. P., Dickinson, W. T., & Sharma, D. N. (1993). Application of GIS in watershed management. New Techniques for Modelling of Stormwater Quality Impacts , January 2016 , 469–482. https://doi.org/10.14796/jwmm.r175-21 Google Scholar
- Sankar, G., Ali, B., Pravat, K., Shit, K., Sayed, E., Omran, E., & Bagherzadeh, A. (2018). Evaluation of groundwater quality and its suitability for drinking and irrigation using GIS and geostatistics techniques in semiarid region of Neyshabur, Iran. Applied Water Science , 8 ( 6 ), 1–16. https://doi.org/10.1007/s13201-018-0795-6 Web of Science ® Google Scholar
- Santos, C., Lorite, I. J., Tasumi, M., Allen, R. G., & Fereres, E. (2008). Integrating satellite-based evapotranspiration with simulation models for irrigation management at the scheme level. Irrigation Science , 26 ( 3 ), 277–288. https://doi.org/10.1007/s00271-007-0093-9 Web of Science ® Google Scholar
- Santos B, Gonçalves J, Martins A M, Pérez-Cano M T, Mosquera-Adell E, Dimelli D, Lagarias A and Almeida P G. (2021). GIS in Architectural Teaching and Research: Planning and Heritage. Education Sciences , 11 ( 6 ), 307 10.3390/educsci11060307 Web of Science ® Google Scholar
- Saroj, A., Ashish, P., & C, U. C. (2014). Use of geographical information systems in irrigation management: A review. Journal of Indian Water Resources Society , 34(2), 32–39. https://docplayer.net/14420348-Use-of-geographic-information-systems-in-irrigation-management-a-review.html . Google Scholar
- Sawadogo, A., Kouadio, L., Traoré, F., Zwart, S. J., Hessels, T., & Gündoğdu, K. S. (2020). Spatiotemporal assessment of irrigation performance of the kou valley irrigation scheme in Burkina Faso using satellite remote sensing-derived indicators. ISPRS International Journal of Geo-Information , 9 ( 8 ), 484. https://doi.org/10.3390/ijgi9080484 Web of Science ® Google Scholar
- Sebnie, W., Adgo, E., & Kendie, H. (2020). Irrigation suitability evaluation of zamra irrigation scheme, abergelle district of wag ‑ himra zone, Amhara region Ethiopia. Environmental Systems Research , 9 ( 1 ), 1–9. https://doi.org/10.1186/s40068-020-00200-6 Google Scholar
- Seif-Ennasr, M., Bouchaou, L., El Morjani, Z. E. A., Hirich, A., Beraaouz, E. H., & Choukr-Allah, R. (2020). GIS-based land suitability and crop vulnerability assessment under climate change in chtouka Ait Baha, Morocco. Atmosphere , 11 ( 11 ), 1167. https://doi.org/10.3390/atmos11111167 Web of Science ® Google Scholar
- Seyedmohammadi, J., Sarmadian, F., Asghar, A., & Mcdowell, R. W. (2019). Geoderma development of a model using matter element, AHP and GIS techniques to assess the suitability of land for agriculture. Geoderma , 352 ( Feb 2018 ): 80–95. https://doi.org/10.1016/j.geoderma.2019.05.046 Google Scholar
- Singh, A. K., Dubey, O. P., & Ghosh, S. K. (2016). Irrigation scheduling using intervention of geomatics tools—A case study of Khedli minor. Agricultural Water Management , 177 , 454–460. https://doi.org/10.1016/j.agwat.2016.05.024 Web of Science ® Google Scholar
- Sishodia, R. P., Ray, R. L., & Singh, S. K. (2020). Applications of remote sensing in precision agriculture: A review. Remote Sensing , 12 ( 19 ), 1–31. https://doi.org/10.3390/rs12193136 Web of Science ® Google Scholar
- Sultan, D. (2013). Assessment of irrigation land suitability and development of map for the fogera catchment using gis, south gonder. Asian Journal of Agriculture and Rural Development , 3 ( 1 ), 7–17. https://doi.org/10.22004/ag.econ.198027 Google Scholar
- Suzuki, S., & Takemi, M. (2012). Remote sensing for medical and health care applications. In ItechOpen. Ed., Remote Sensing Applications , (vol. 32 (ItechOpen), pp. 137–144). http://www.intechopen.com/books/trends-in-telecommunications-technologies/gps-total-electron-content-tec-prediction-at-ionosphere-layer-over-the-equatorial-region%0AInTec Google Scholar
- Taghvaeian, S., Neale, C. M. U., Osterberg, J. C., Sritharan, S. I., & Watts, D. R. (2018). Remote sensing and GIS techniques for assessing irrigation performance: Case study in southern California. Journal of Irrigation and Drainage Engineering , 144 ( 6 ), 05018002. https://doi.org/10.1061/(ASCE)IR.1943-4774.0001306 Web of Science ® Google Scholar
- Tantawy, I. (2019). The application of the FAO WaPOR data portal to monitor efficient water use in agriculture: A case study on the eastern Nile river basin . TU DELFT. Google Scholar
- Tempfli, K., Kerle, N., Huurneman, G. C., Janssen, L. L. F., Bakker, W. H., Feringa, W., Gieske, A. S. M., Gorte, B. G. H., Grabmaier, K. A., Hecker, C. A., Horn, J. A., Huurneman, G. C., Janssen, L. L. F., Kerle, N., Meer, F. D., Van Der, P., Pohl, G. N., Reeves, C., Ruitenbeek, C. V., … Woldai, T. (2009). Principles of remote sensing : An introductory textbook . The International Institute for Geo-Information Science and Earth Observation (ITC). Google Scholar
- Tessema Roba, N., Kebede Kassa, A., & Yadeta Geleta, D. (2021). Modeling climate change impacts on crop water demand, middle Awash River basin, case study of Berehet woreda. Water Practice and Technology , 1–22. https://doi.org/10.2166/wpt.2021.033 Google Scholar
- Thapliyal, A., Panwar, A., Space, U., Centre, A., Sum, W., & Classification, Q. (2019). Agriculture potential zone identification using gis technique in the hilly terrain block of uttarkashi district. The International Archives of the Photogrammetry, Remote Sensing and Spatial Information Sciences , XLII ( February ), 18–20. https://doi.org/10.5194/isprs-archives-XLII-3-W6-439-2019 Google Scholar
- Ungureanu, N., Vlăduț, V., & Voicu, G. (2020). Water scarcity and wastewater reuse in crop irrigation. Sustainability (Switzerland) , 12 ( 21 ), 1–19. https://doi.org/10.3390/su12219055 PubMed Web of Science ® Google Scholar
- USC. (2021, February 23). The Future of GIS - USC GIS Online . //Gis.Usc.Edu/Blog. https://gis.usc.edu/blog/the-future-of-gis/ Google Scholar
- Valencia J, Monserrate F, Casteleyn S, Bax V, Francesconi W and Quintero M. (2020). A GIS-based methodological framework to identify superficial water sources and their corresponding conduction paths for gravity-driven irrigation systems in developing countries. Agricultural Water Management , 232 , 106048. https://doi.org/10.1016/j.agwat.2020.106048 Web of Science ® Google Scholar
- Villa, R Orlando, P. (2011). Remote sensing applications in archaeology. Archeologia E Calcolatori , 22, 147–168 https://core.ac.uk/display/33151603?utm_source=pdf&utm_medium=banner&utm_campaign=pdf-decoration-v1 . Google Scholar
- Vogels, M. F. A., de Jong, S. M., Sterk, G., & Addink, E. A. (2019). Mapping irrigated agriculture in complex landscapes using SPOT6 imagery and object-based image analysis – A case study in the central rift valley, Ethiopia –. International Journal of Applied Earth Observation and Geoinformation , 75 ( May 2018 ):118–129. https://doi.org/10.1016/j.jag.2018.07.019. Google Scholar
- Xiao Y and Watson M. (2019). Guidance on Conducting a Systematic Literature Review. Journal of Planning Education and Research , 39 ( 1 ), 93–112. 10.1177/0739456X17723971 Web of Science ® Google Scholar
- Xiong, J., Thenkabail, P. S., Gumma, M. K., Teluguntla, P., Poehnelt, J., Congalton, R. G., Yadav, K., & Thau, D. (2017). Automated cropland mapping of continental Africa using Google earth engine cloud computing. ISPRS Journal of Photogrammetry and Remote Sensing , 126 , 225–244. https://doi.org/10.1016/j.isprsjprs.2017.01.019 Web of Science ® Google Scholar
- Yang, B., Chen, D., Ren, B., & Wu, Y. (2016). Application of GIS and 3D visualization simulation in irrigation management system-an effective solution of visualization and dynamic for water resourses management International Conference on Applied Mathematics, Simulation and Modelling. , 219–222. https://doi.org/10.2991/amsm-16.2016.50 Google Scholar
- Younes, M., & Salman, A. (2021). A cloud-based application for smart irrigation management system. 2021 8th International Conference on Electrical and Electronics Engineering, ICEEE 2021, 85–92. https://doi.org/10.1109/ICEEE52452.2021.9415913 Google Scholar
- Zafar, A., Prathapar, S., & Bastiaanssen, W. (2021). Optimization of canal management based on irrigation performance analysis using satellite measurements. (165). http://dx.doi.org/10.22617/BRF210022-2 Google Scholar
- Zeyliger, A. M., Muzalevskiy, K. V., Zinchenko, E. V., & Ermolaeva, O. S. (2022). Field test of the surface soil moisture mapping using Sentinel-1 radar data. Science of the Total Environment , 807 (2) , 151121. https://doi.org/10.1016/j.scitotenv.2021.151121 PubMed Web of Science ® Google Scholar
- Ziadat, F. M., & Sultan, K. A. (2011). Combining current land use and farmers ’ knowledge to design land-use requirements and improve land suitability evaluation. Renewable Agriculture and Food Systems , 26 ( 4 ), 287–296. https://doi.org/10.1017/S1742170511000093 Web of Science ® Google Scholar
- Back to Top
Related research
People also read lists articles that other readers of this article have read.
Recommended articles lists articles that we recommend and is powered by our AI driven recommendation engine.
Cited by lists all citing articles based on Crossref citations. Articles with the Crossref icon will open in a new tab.
- People also read
- Recommended articles
To cite this article:
Download citation, your download is now in progress and you may close this window.
- Choose new content alerts to be informed about new research of interest to you
- Easy remote access to your institution's subscriptions on any device, from any location
- Save your searches and schedule alerts to send you new results
- Export your search results into a .csv file to support your research
Login or register to access this feature
Register now or learn more
- Bibliography
- More Referencing guides Blog Automated transliteration Relevant bibliographies by topics
- Automated transliteration
- Relevant bibliographies by topics
- Referencing guides
Undergraduate Studies
- Level 8 Degrees
- Open Days | Events
- Guidance Counsellors
- Visit Maynooth University
- How to Apply | CAO
- A Maynooth Education
- Prospectus & Booklets
- Scholarships
- Certificates and Short Courses
- Postgraduate Studies
- Taught Master's, Diplomas and Certificates
- Research Programmes
- Micro-credentials
- Springboard Courses
- Fees, Funding & Scholarships
- How to Apply for a Postgraduate Programme
International
- The Maynooth Student Experience
- Exchange Incoming (ERASMUS and Study Abroad)
- Study Abroad Incoming
- Summer and Tailored Programmes
- Prospective Full Degree Students
- Go Abroad with Maynooth
- Pre-Arrival & Orientation
Search form
Msc geograp.information systems & remote sensing.
- Study at Maynooth /
Qualification : MASTER OF SCIENCE DEGREE
Award Type and NFQ level : TAUGHT MASTERS (9)
CAO/PAC code : MHN58
CAO Points :
Closing Date : 31 July 2025
View FETAC details
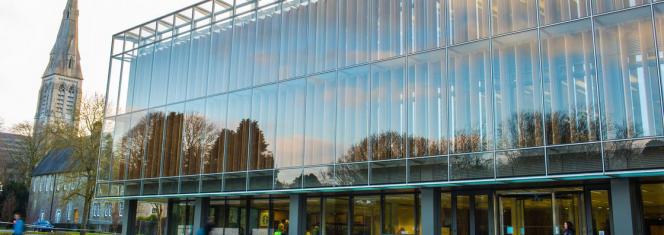
- Entry Requirements
- Department People
- Course Structure
- Career Options
- How to Apply
Given the wider development of Citizen GIS and an increased public awareness and knowledge of the power and value of spatial data, vastly increased amounts of such data from different sources are now available to researchers. However, in order to turn these data into useful information, they must be efficiently managed, processed and analysed before being displayed in a comprehensible format. Geographical Information Systems and the associated field of Remote Sensing greatly aid us in such tasks. The course is equally split between both parts - GIS and Remote Sensing - with four core modules introducing the theory and practice of both subject at an introductory and advanced level. Geographical Information Systems or GIS as they are better known, are widely used in a wide variety of subject fields across the physical and social sciences and even in the humanities, with applicability in everything from archaeology and astronomy to geomorphology and globalisation to soil science and social planning. Remote Sensing the analysis and interpretation of aerial and satellite imagery has transformed the manner in which we view the Earth. The synoptic view of the Earth that it has given us has greatly improved our understanding of atmospheric and oceanic processes, sustained environmental management and the interaction of humans with the natural world. It is now a standard research tool in many fields such as geology, geography, pollution control, agriculture and climatology. Additional optional modules in Programming, Spatial Databases and Marine Remote Sensing are also available to students who want to develop the technical side more fully, though the course has a strong applied flavour throughout. In addition, all students complete a work placement in the summer months which allows them to gain valuable practical experience to test and develop the skills learnt across the course.
The MSc in GIS and Remote Sensing is accredited by both the Society of Chartered Surveyors Ireland (SCSI) and the Royal Institution of Chartered Surveyors (RICS) in the UK. Graduates of this MSc are eligible to proceed along the pathway to become Chartered Geomatics Surveyors.
Aims of the Course
- To provide highly qualified, motivated graduates who have been trained in Geographical Information Systems, Remote Sensing and Digital Image Processing and who can apply the information technology skills they obtain.
- To produce marketable graduates who will make significant contributions to GIS and RS application areas including; industry, government, academia, the community and voluntary sector and other public and private bodies.
- To provide an understanding of Geographical Information Systems and Remote Sensing, the technology involved and its applications for specific investigations.
Commences September (*optional courses may start ahead of the formal term date).
The basic entry requirement is a degree with a minimum of Second Class Honours (2:1) or equivalent in any of the following subjects: Geography, Planning, Physics, Computer Science, Environmental Science, Geology, Mathematics, Engineering, Geophysics, Public Administration, Public Health or a cognate discipline. Applicants must have a recognised primary degree which is considered equivalent to Irish university primary degree level.
Minimum English language requirements:
Applicants for whom English is not their first language are required to demonstrate their proficiency in English in order to benefit fully from their course of study. For information about English language tests accepted and required scores, please see here . The requirements specified are applicable for both EU and International applicants.
Maynooth University's TOEFL code is 8850
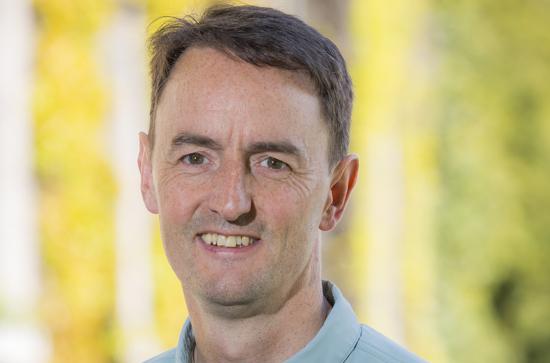
Dr Stephen McCarron
Head of Department
Department of Geography
Department of ICARUS
Read Full Biography
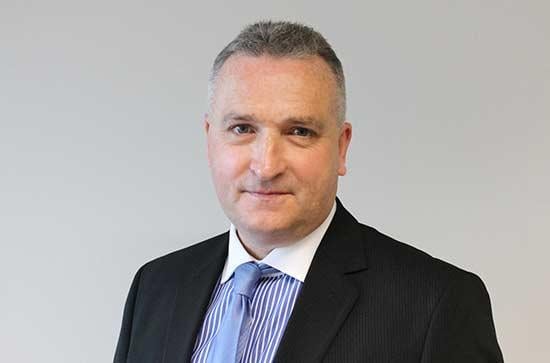
Prof Mark Boyle
Department of Social Sciences Institute (MUSSI)
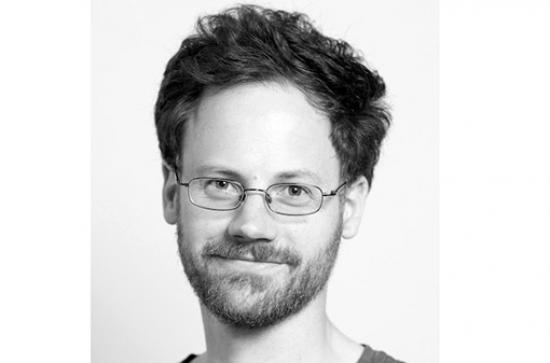
Dr Patrick Bresnihan
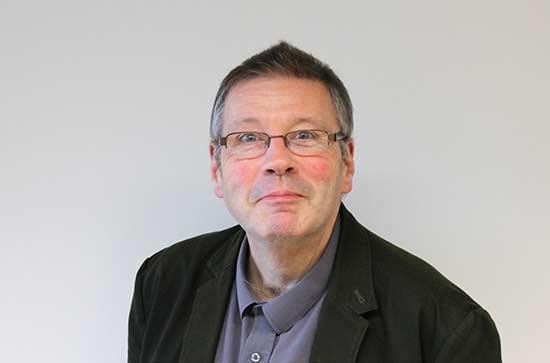
Prof Chris Brunsdon
Department of National Centre for Geocomputation (NCG)
Department of Hamilton Institute
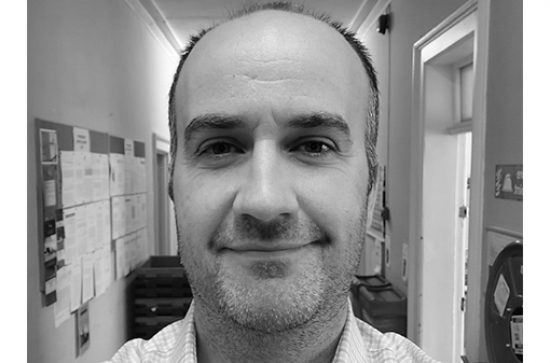
Dr Conor Cahalane
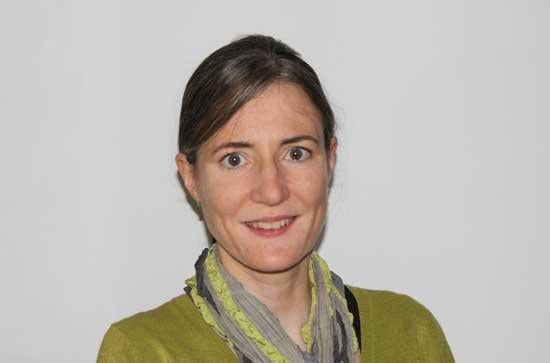
Dr Ro Charlton
Dr kevin credit.
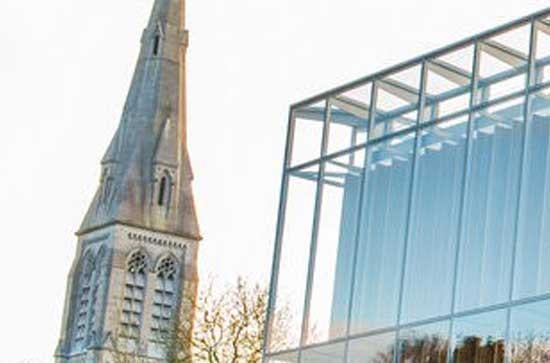
Laure De Tymowski
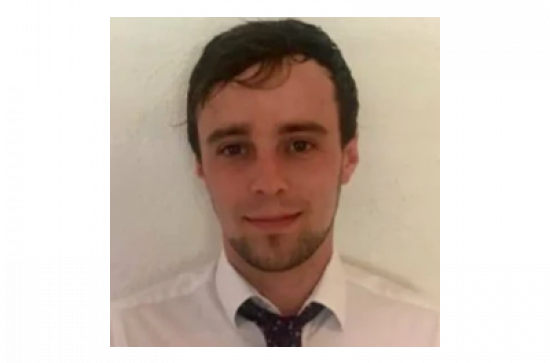
Dr William Durkan
Prof rowan fealy.
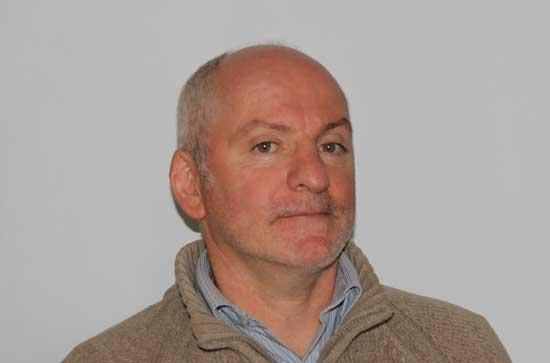
Dr Ronan Foley
Department of ALL Institute
Dr Alistair Fraser
Prof mary gilmartin.
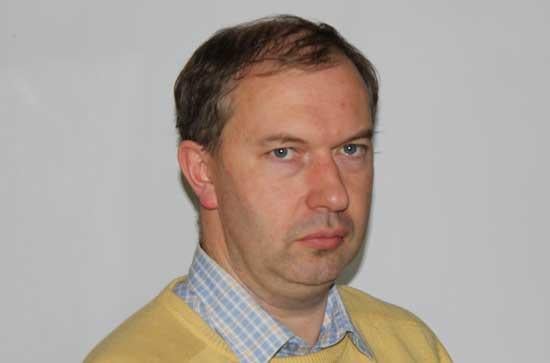
Dr Adrian Kavanagh
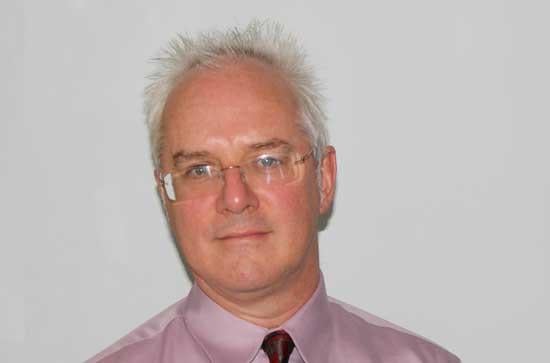
Prof Gerry Kearns
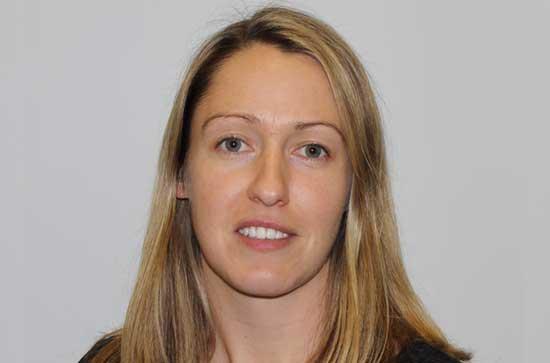
Dr Sinéad Kelly
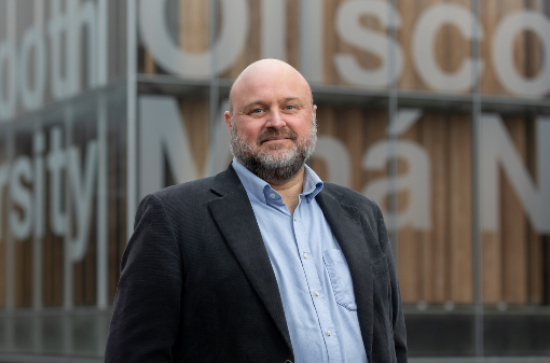
Prof Rob Kitchin
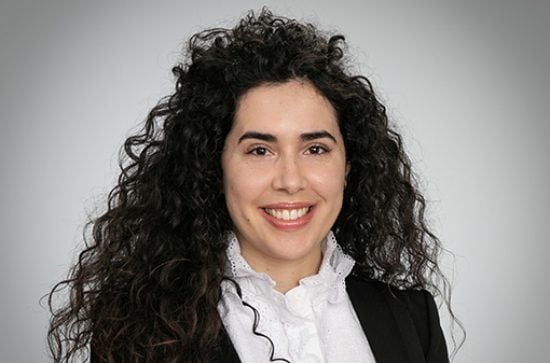
Dr Carla Mateus
Dr gerard mccarthy.
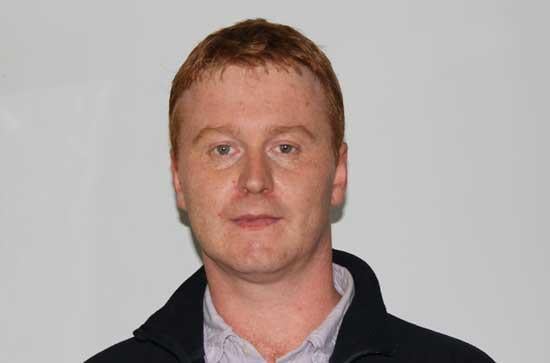
Prof Conor Murphy
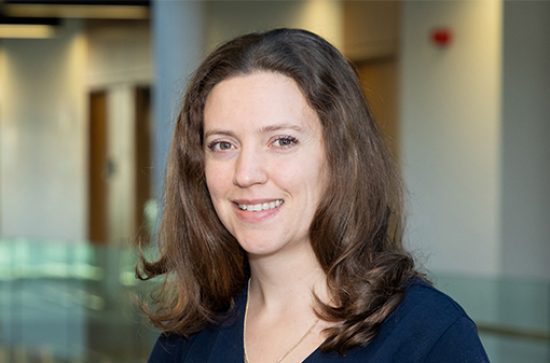
Dr Lisa Orme
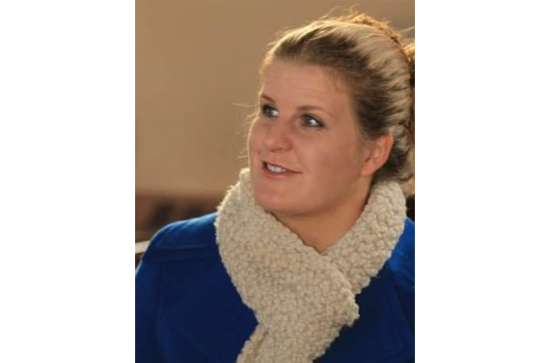
Dr Martina Roche
Dr carla sa ferreira.
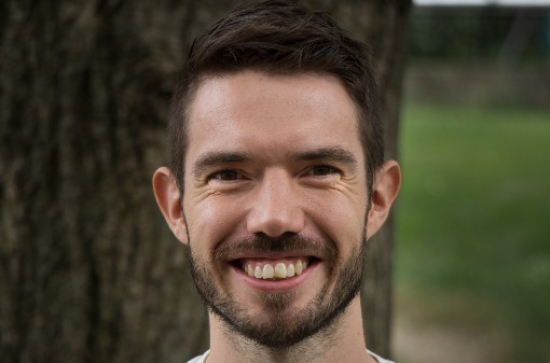
Dr Nick Scroxton
Dr Helen Shaw
Department of Faculty of Social Sciences
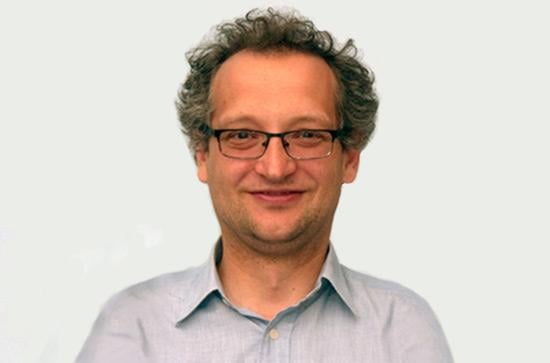
Prof Peter Thorne
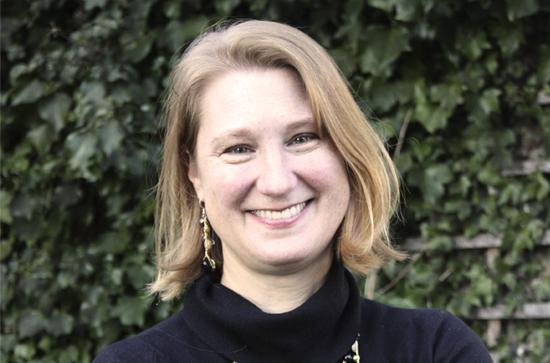
Prof Karen Till
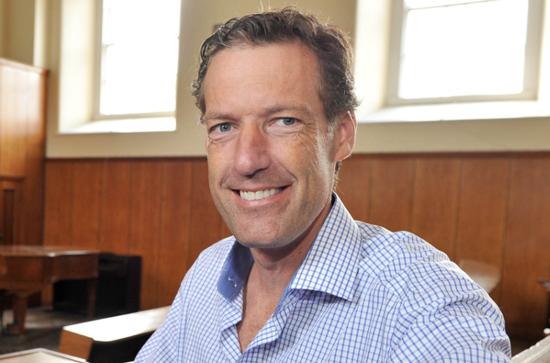
Dr Chris Van Egeraat
The course consists of six modules, five of which are compulsory. Two of these cover the theoretical concepts underpinning GIS and Remote Sensing. Two other modules involve gaining the theoretical and technical skills necessary to become proficient in the management and analysis of spatial data. A fifth module involves an assessed work placement during the summer months.