Code | Title | Credits |
Required Courses | |
| GIS Programming and Software Development | 3 |
| Cartography and Visualization | 3 |
| Geographical Information Analysis | 3 |
Electives | |
Select 6 credits from the following: | 6 |
| GEOVISUAL ANALYTICS | |
| Spatial Data Science Ethics | |
| Geospatial System Analysis and Design | |
| Open Web Mapping | |
| Analytical Approaches in Spatial Data Science | |
| Emerging Trends in Remote Sensing | |
Total Credits | 15 |
Graduate courses carry numbers from 500 to 699 and 800 to 899. Advanced undergraduate courses numbered between 400 and 499 may be used to meet some graduate degree requirements when taken by graduate students. Courses below the 400 level may not. A graduate student may register for or audit these courses in order to make up deficiencies or to fill in gaps in previous education but not to meet requirements for an advanced degree.
Learning Outcomes
- KNOW: Graduates will be able to characterize the state of the art in spatial data science methodologies and technologies.
- APPLY/CREATE: Graduates will be able to create new analytical and visualization solutions to complex spatial data science problems that intersect people and the environment.
- COMMUNICATE: Graduates will demonstrate effective scientific writing, presentation, and cartographic communication skills.
- THINK: Graduates will be able to explain and contextualize the distinguishing characteristics of spatial data sources, spatial analysis methods, and geographic visualizations.
- PROFESSIONAL PRACTICE: Graduates will be able to evaluate spatial data science problems to address ethical and professional challenges.
World Campus Program and Contact Information Campus | World Campus |
Graduate Program Head | Anthony C Robinson |
Director of Graduate Studies (DGS) or Professor-in-Charge (PIC) | Anthony C Robinson |
Program Contact | |
Program Website | |
Print Options
Print this page.
The PDF will include all information unique to this page.
The PDF will include content on the Overview tab only.
The PDF will include content on the Admission Requirements tab only.
The PDF will include content on the Certificate Requirements tab only.
The PDF will include content on the Courses tab only.
The PDF will include content on the Learning Outcomes tab only.
The PDF will include content on the Contact tab only.
Download Complete Bulletin PDFs
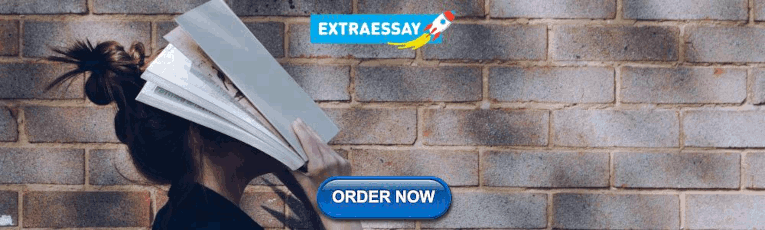
COMMENTS
An NRT-sponsored program in Data Science Overview Overview Advances in computational speed and data availability, and the development of novel data analysis methods, have birthed a new field: data science. This new field requires a new type of researcher and actor: the rigorously trained, cross-disciplinary, and ethically responsible data scientist. Launched in Fall 2017, the …
Ph.D. / Full-time / On Campus. 23,071 EUR / year. 4 years. University of Tulsa Tulsa, Oklahoma, United States. Ranked top 5%. Top 5% of Universities worldwide according to the Studyportals Meta Ranking.
Based in San Diego, California, National University (NU) offers a variety of online programs, including a Ph.D. in data science. NU's program requires 60 credits and takes an estimated 40 months ...
The Department requires that students in the Statistics and Data Science PhD program: Meet the department minimum residency requirement of 2 years. Complete the following courses: STAT 344-0 Statistical Computing. STAT 350-0 Regression Analysis. STAT 353-0 Advanced Regression. STAT 415-0 I ntroduction to Machine Learning.
A PhD in data analytics prepares professionals to work in data-driven fields, including research, business, healthcare, and government. The most common reason people pursue a PhD in a data-related field is that they are passionate about data and would like to have a career that involves research and making discoveries, usually within a sub ...
The PhD in statistics and data science at Yale University offers broad training in the areas of statistical theory, probability theory, stochastic processes, asymptotics, information theory, machine learning, data analysis, statistical computing, and graphical methods. Students need to complete 12 courses in the first year on these topics.
To enhance career prospects, students can pursue Graduate Certificate in Data Science, and possibly use the certificate courses to fulfill the PhD degree elective requirements. NSM Career Success Center is available to support professional development and experiential learning of students. GRE test score is not required for admission.
A PhD in Data Analysis opens up a wide range of career opportunities in both academia and industry. Many graduates go on to become data scientists, working in sectors such as finance, healthcare, marketing, and technology. As a data scientist, you will be responsible for analyzing large datasets, developing predictive models, and providing ...
The PhD program in data science, analytics and engineering engages students in fundamental and applied research. The program's educational objective is to develop each student's ability to perform original research in the development and execution of data-driven methods for solving major societal problems. This includes the ability to identify ...
The PhD in Big Data Analytics is an interdisciplinary STEM PhD program focusing on systems and technologies for processing data and information. Unlike pure data science programs, this program includes the human and social implications of information and technology, bringing in critical components of cognition, ethics, biases and storytelling ...
PhD in Data Science (on-campus) Stevens Institute of Technology is accredited by the Middle States Commission on Higher Education. 10. University of Central Florida. Located along Orlando's Space Coast, the University of Central Florida is a public research university with a student enrollment of approximately 69,525.
Data analysis involves analyzing large data sets and interpreting them to make strategic choices. When pursuing PhD, you will learn about the techniques and tools involved in data analysis. You will also have to research the same and develop new suggestions. Whereas data science uses the analyzed data to create solutions for business problems.
PhD requirements include coursework as well as a research component. Together they total a minimum of 60 credit hours beyond the Data Science master's degree requirement.Students entering the Ph.D. Program with a bachelor's degree first complete the M.S. in data science at WPI using the M.S. Thesis option as first step towards their Ph.D. degree.
The Ph.D. in Big Data Analytics requires 72 hours beyond an earned Bachelor's degree. Required coursework includes 30 credit hours of courses, 21 credit hours of restricted elective coursework, and 21 credit hours of dissertation research. All Ph.D. students must have an approved Plan of Study (POS) developed by the student and advisor that ...
A Data Analytics program teaches skills in data analysis tools, programming languages, data collection and cleaning, statistical methods, data visualisation, and applying analytics to solve business problems. Skills required for a degree in Data Analytics include mathematical proficiency, programming skills, database understanding, and ...
Overview. The core mission of the Mendoza PhD in Analytics is to develop thought-leaders in the analytics space that are engaged in impactful, cutting-edge scholarly research that considers the ethical dimension of data and its usage. Graduates of the PhD program are well-positioned to attain academic jobs at top business schools, where they ...
National University. National University offers a Doctor of Philosophy in Data Science. The program is 100% online and designed to allow students to study on their own time. New classes start every week, so students can enroll whenever it is most convenient. The program requires the completion of 20 courses.
Understand conceptual and practical aspects of data collection, management and analysis. Prepare to present scholarly work and to thoughtfully critique the work of others. This degree is offered online; however, learners are expected to participate in two five-day residencies on or near GCU's campus in Phoenix.
A Ph.D. degree is the highest academic qualification anyone can earn. And with Harvard Business Review declaring data scientist to be the sexiest job of the 21 st century, a Ph.D. in Data Science should probably be called the sexiest university degree of the century.. Universities in Canada, like the ones in other highly advanced countries, are offering such degrees in abundance.
Organized Data Management: PhD researchers benefit from Dedoose's organizational features, streamlining the coding and retrieval of data for a more efficient analysis process. Tool 7: HyperRESEARCH HyperRESEARCH caters to various qualitative research methods, including content analysis and grounded theory, offering a flexible platform for PhD ...
IISc Bangalore : 4. IIT Delhi : 5. BITS Pilani : Here are some institutes that currently have their applications open for a PhD in India. 1.Indian Statistical Institute (ISI): The PhD program at ISI provides the students with an exceptional academic environment through a variety of educational and research opportunities.
And place questionnaires, copies of focus groups and interviews, and data sheets in the appendix. On the other hand, one must put the statistical analysis and sayings quoted by interviewees within the dissertation. 8. Thoroughness of Data. It is a common misconception that the data presented is self-explanatory.
A PhD in statistics gives you a very strong foundation in the future labour market, where there is more demand for competency in data analysis. The PhD programme in statistics at Uppsala University provide both a broadening and deepening in statistics, providing skills in modern statistics methods and research areas. ...
The best data analysis software for your PhD research is the one that aligns with your research style and empowers you to derive meaningful insights from your data. Before making your final choice, consider conducting trials or exploring free versions of software to get a feel for their interface and capabilities. Additionally, consult with ...
Karolina Naranjo-Velasco, who presented her work on facilitating data-drive approaches to legal text analysis of documents from the Colombian Constitutional Court; Jason Wang, who presented his work assessing computer vision-based worker poster analysis methods; Leadership and Service Award. Beau LeBlond, a third-year Ph.D. student
WEST LAFAYETTE, Ind. — Data scientists who can make sense of today's epic floods of data to generate actionable insights and communicate them to a variety of audiences are in demand in almost any field, from retail business and industry to health care, government, education, and more. The U.S ...
data analysis employment expectations for new graduates. Furthermore, this work seeks to highlight issues relevant to curriculum management in university degree programs. 786 job postings were ... doctorate/PhD, 144 required a master's and 60 required a minimum of a high school diploma.
Lauren Bennett, PhD is product engineering director of Esri's Spatial Analysis and Data Science team. In her role, she oversees research and development of the ArcGIS analytical framework, which includes spatial and spatiotemporal statistics, raster and multidimensional analysis, and machine learning and big data analytics.
PhD Programme in Data Science; Undergraduate Programmes. School of Data Science (BDDS) Bachelor of Science in Data Science (BSc DS) ... Bayesian Data Analysis: 3: SDSC6004: Data Analytics for Smart Cities: 3: SDSC6006: Dissertation: 6: SDSC6007: Dynamic Programming and Reinforcement Learning: 3: SDSC6008:
The Certificate in Spatial Data Science prepares students who have prior experience with the application of Geographic Information Systems (GIS) to research, design, and create novel analytical and visualization solutions to complex problems that intersect people and the environment. The program engages key theories, emerging research, and contemporary spatial analysis techniques so that ...