
An official website of the United States government
The .gov means it’s official. Federal government websites often end in .gov or .mil. Before sharing sensitive information, make sure you’re on a federal government site.
The site is secure. The https:// ensures that you are connecting to the official website and that any information you provide is encrypted and transmitted securely.
- Publications
- Account settings
- My Bibliography
- Collections
- Citation manager
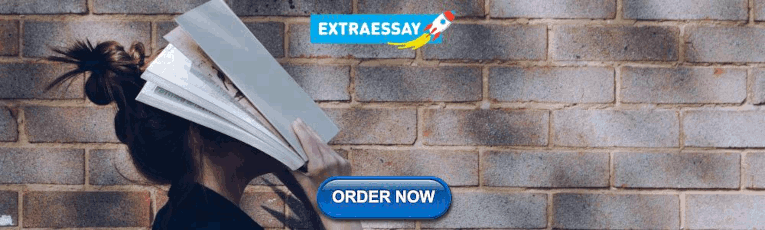
Save citation to file
Email citation, add to collections.
- Create a new collection
- Add to an existing collection
Add to My Bibliography
Your saved search, create a file for external citation management software, your rss feed.
- Search in PubMed
- Search in NLM Catalog
- Add to Search
Multiple Intelligences in Teaching and Education: Lessons Learned from Neuroscience
Affiliation.
- 1 Multiple Intelligences Research and Consulting, Inc., Kent, OH 44240, USA. [email protected].
- PMID: 31162465
- PMCID: PMC6480719
- DOI: 10.3390/jintelligence6030038
This brief paper summarizes a mixed method review of over 500 neuroscientific reports investigating the proposition that general intelligence ( g or IQ) and multiple intelligences (MI) can be integrated based on common and unique neural systems. Extrapolated from this interpretation are five principles that inform teaching and curriculum so that education can be strengths-based and personalized to promote academic achievement. This framework is proposed as a comprehensive model for a system of educational cognitive neuroscience that will serve the fields of neuroscience as well as educators. Five key principles identified are culture matters, every brain is unique-activate strengths, know thyself, embodied cognition/emotional rudder, and make it mean something.
Keywords: and make it mean something; culture matters; embodied cognition/emotional rudder; every brain is unique—activate strengths; know thyself; multiple intelligences.
PubMed Disclaimer
Conflict of interest statement
The author is the creator and publisher of the Multiple Intelligences Developmental Assessment Scales (MIDAS) mentioned in this article. No other potential conflicts of interest are declared.
Personalized educational cognitive neuroscience: a…
Personalized educational cognitive neuroscience: a framework. Note : The bridge between existing psychological…
Similar articles
- Why multiple intelligences theory is a neuromyth. Waterhouse L. Waterhouse L. Front Psychol. 2023 Aug 28;14:1217288. doi: 10.3389/fpsyg.2023.1217288. eCollection 2023. Front Psychol. 2023. PMID: 37701872 Free PMC article. Review.
- Building a functional multiple intelligences theory to advance educational neuroscience. Cerruti C. Cerruti C. Front Psychol. 2013 Dec 19;4:950. doi: 10.3389/fpsyg.2013.00950. eCollection 2013. Front Psychol. 2013. PMID: 24391613 Free PMC article.
- Student and educator experiences of maternal-child simulation-based learning: a systematic review of qualitative evidence protocol. MacKinnon K, Marcellus L, Rivers J, Gordon C, Ryan M, Butcher D. MacKinnon K, et al. JBI Database System Rev Implement Rep. 2015 Jan;13(1):14-26. doi: 10.11124/jbisrir-2015-1694. JBI Database System Rev Implement Rep. 2015. PMID: 26447004
- Discussion of Teaching With Multiple Intelligences to Corporate Employees' Learning Achievement and Learning Motivation. Lei DY, Cheng JH, Chen CM, Huang KP, James Chou C. Lei DY, et al. Front Psychol. 2021 Oct 18;12:770473. doi: 10.3389/fpsyg.2021.770473. eCollection 2021. Front Psychol. 2021. PMID: 34733224 Free PMC article.
- Matching purpose with practice: revolutionising nurse education with mita. Denny M, Weber EF, Wells J, Stokes OR, Lane P, Denieffe S. Denny M, et al. Nurse Educ Today. 2008 Jan;28(1):100-7. doi: 10.1016/j.nedt.2007.03.004. Epub 2007 Apr 24. Nurse Educ Today. 2008. PMID: 17459538 Review.
- Transcend socioeconomic status constraints to mathematics and science achievement by collaborative problem-solving: The female people-smartness hypothesis. Chiu MS. Chiu MS. Front Psychol. 2022 Aug 26;13:944329. doi: 10.3389/fpsyg.2022.944329. eCollection 2022. Front Psychol. 2022. PMID: 36092087 Free PMC article.
- Understanding the Emotional Intelligence Discourse on Social Media: Insights from the Analysis of Twitter. Shankar S, Tewari V. Shankar S, et al. J Intell. 2021 Nov 24;9(4):56. doi: 10.3390/jintelligence9040056. J Intell. 2021. PMID: 34842754 Free PMC article.
- Confronting the Challenges of Anatomy Education in a Competency-Based Medical Curriculum During Normal and Unprecedented Times (COVID-19 Pandemic): Pedagogical Framework Development and Implementation. Naidoo N, Akhras A, Banerjee Y. Naidoo N, et al. JMIR Med Educ. 2020 Oct 7;6(2):e21701. doi: 10.2196/21701. JMIR Med Educ. 2020. PMID: 32873536 Free PMC article.
- Assessment of multiple intelligences in elementary school students in Mexico: An exploratory study. González-Treviño IM, Núñez-Rocha GM, Valencia-Hernández JM, Arrona-Palacios A. González-Treviño IM, et al. Heliyon. 2020 Apr 14;6(4):e03777. doi: 10.1016/j.heliyon.2020.e03777. eCollection 2020 Apr. Heliyon. 2020. PMID: 32322737 Free PMC article.
- Shearer C.B., Karanian J.M. The neuroscience of intelligence: Empirical support for the theory of multiple intelligences? Trends Neurosci. Educ. 2017;6:211–223. doi: 10.1016/j.tine.2017.02.002. - DOI
- Gardner H. Frames of Mind: The Theory of Multiple Intelligences. Basic Books; New York, NY, USA: 1993.
- Chen J.Q., Moran S., Gardner H. Multiple Intelligences around the World. John Wiley & Sons; Hoboken, NJ, USA: 2009.
- Koretz D. The Testing Charade: Pretending to Make Schools Better. University of Chicago Press; Chicago, IL, USA: 2017.
- Johnson D., Johnson B. High Stakes: Poverty, Testing, and Failure in American Schools. Rowan & Littlefield Publishers; Lanham, MD, USA: 2006.
Related information
Linkout - more resources, full text sources.
- Europe PubMed Central
- PubMed Central
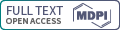
- Citation Manager
NCBI Literature Resources
MeSH PMC Bookshelf Disclaimer
The PubMed wordmark and PubMed logo are registered trademarks of the U.S. Department of Health and Human Services (HHS). Unauthorized use of these marks is strictly prohibited.
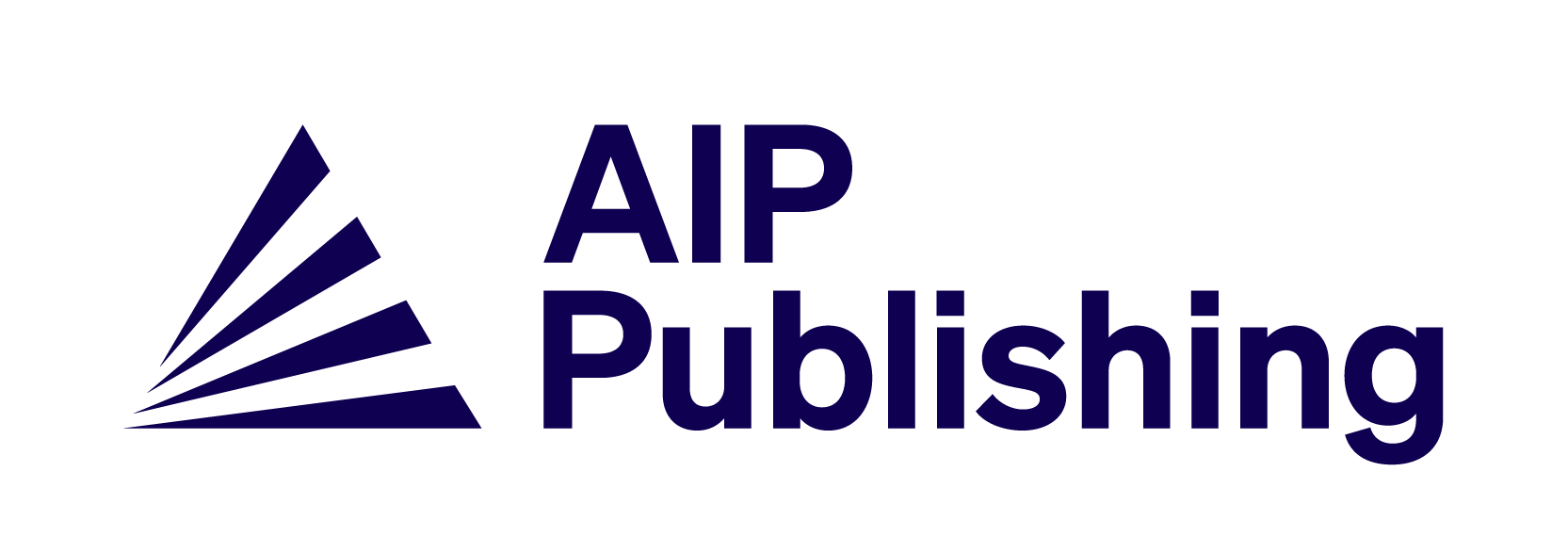
Gardner’s multiple intelligences in science learning: A literature review
- Article contents
- Figures & tables
- Supplementary Data
- Peer Review
- Reprints and Permissions
- Cite Icon Cite
- Search Site
Fibriyana Safitri , Dadi Rusdiana , Wawan Setiawan; Gardner’s multiple intelligences in science learning: A literature review. AIP Conf. Proc. 28 April 2023; 2619 (1): 100014. https://doi.org/10.1063/5.0122560
Download citation file:
- Ris (Zotero)
- Reference Manager
The purpose of this article is to review articles related to multiple intelligences in learning and figure out the multiple intelligences approach in learning activities and several bits of intelligence that play an important role in science learning. This paper is the result of a review of 40 articles related to learning activities that use multiple intelligence-based approaches, media, and learning models, published from 2011 to 2021. The multiple intelligences theory was put forward by Howard Gardner, an expert in education and psychology. There are nine types of intelligence based on Gardner’s theory, namely: verbal-linguistic intelligence, visual-spatial intelligence, musical intelligence, logical-mathematics intelligence, interpersonal intelligence, intrapersonal intelligence, bodily-kinesthetic intelligence, naturalist intelligence, and existential intelligence, which have different characteristics. The method used in this study is a systematic literature review with the following stages: determining research questions; determining criteria; generating a framework for articles; searching, filtering, and selecting; analyzing and interpreting the content of each reviewed article; article writing, and publishing. This study discusses Gardner’s multiple intelligence theory, the multiple intelligences approach in learning activities, and the most influential intelligence in science learning. The results show that several bits of intelligence play an important role in science learning.
Citing articles via
Publish with us - request a quote.
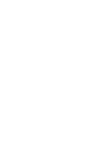
Sign up for alerts
Related content.
- Online ISSN 1551-7616
- Print ISSN 0094-243X
- For Researchers
- For Librarians
- For Advertisers
- Our Publishing Partners
- Physics Today
- Conference Proceedings
- Special Topics
pubs.aip.org
- Privacy Policy
- Terms of Use
Connect with AIP Publishing
This feature is available to subscribers only.
Sign In or Create an Account
Multiple Intelligences: What Does the Research Say?
Proposed by Howard Gardner in 1983, the theory of multiple intelligences has revolutionized how we understand intelligence. Learn more about the research behind his theory.
Your content has been saved!
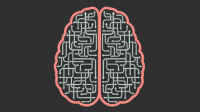
Many educators have had the experience of not being able to reach some students until presenting the information in a completely different way or providing new options for student expression. Perhaps it was a student who struggled with writing until the teacher provided the option to create a graphic story, which blossomed into a beautiful and complex narrative. Or maybe it was a student who just couldn't seem to grasp fractions, until he created them by separating oranges into slices.
Because of these kinds of experiences, the theory of multiple intelligences resonates with many educators. It supports what we all know to be true: A one-size-fits-all approach to education will invariably leave some students behind. However, the theory is also often misunderstood, which can lead to it being used interchangeably with learning styles or applying it in ways that can limit student potential. While the theory of multiple intelligences is a powerful way to think about learning, it’s also important to understand the research that supports it.
Howard Gardner's Eight Intelligences
The theory of multiple intelligences challenges the idea of a single IQ, where human beings have one central "computer" where intelligence is housed. Howard Gardner, the Harvard professor who originally proposed the theory, says that there are multiple types of human intelligence, each representing different ways of processing information:
- Verbal-linguistic intelligence refers to an individual's ability to analyze information and produce work that involves oral and written language, such as speeches, books, and emails.
- Logical-mathematical intelligence describes the ability to develop equations and proofs, make calculations, and solve abstract problems.
- Visual-spatial intelligence allows people to comprehend maps and other types of graphical information.
- Musical intelligence enables individuals to produce and make meaning of different types of sound.
- Naturalistic intelligence refers to the ability to identify and distinguish among different types of plants, animals, and weather formations found in the natural world.
- Bodily-kinesthetic intelligence entails using one's own body to create products or solve problems.
- Interpersonal intelligence reflects an ability to recognize and understand other people's moods, desires, motivations, and intentions.
- Intrapersonal intelligence refers to people's ability to recognize and assess those same characteristics within themselves.
The Difference Between Multiple Intelligences and Learning Styles
One common misconception about multiple intelligences is that it means the same thing as learning styles. Instead, multiple intelligences represents different intellectual abilities. Learning styles, according to Howard Gardner, are the ways in which an individual approaches a range of tasks. They have been categorized in a number of different ways -- visual, auditory, and kinesthetic, impulsive and reflective, right brain and left brain, etc. Gardner argues that the idea of learning styles does not contain clear criteria for how one would define a learning style, where the style comes, and how it can be recognized and assessed. He phrases the idea of learning styles as "a hypothesis of how an individual approaches a range of materials."
Everyone has all eight types of the intelligences listed above at varying levels of aptitude -- perhaps even more that are still undiscovered -- and all learning experiences do not have to relate to a person's strongest area of intelligence. For example, if someone is skilled at learning new languages, it doesn’t necessarily mean that they prefer to learn through lectures. Someone with high visual-spatial intelligence, such as a skilled painter, may still benefit from using rhymes to remember information. Learning is fluid and complex, and it’s important to avoid labeling students as one type of learner. As Gardner states, "When one has a thorough understanding of a topic, one can typically think of it in several ways."
What Multiple Intelligences Theory Can Teach Us
While additional research is still needed to determine the best measures for assessing and supporting a range of intelligences in schools, the theory has provided opportunities to broaden definitions of intelligence. As an educator, it is useful to think about the different ways that information can be presented. However, it is critical to not classify students as being specific types of learners nor as having an innate or fixed type of intelligence.
Practices Supported by Research
Having an understanding of different teaching approaches from which we all can learn, as well as a toolbox with a variety of ways to present content to students, is valuable for increasing the accessibility of learning experiences for all students. To develop this toolbox, it is especially important to gather ongoing information about student strengths and challenges as well as their developing interests and activities they dislike. Providing different contexts for students and engaging a variety of their senses -- for example, learning about fractions through musical notes, flower petals, and poetic meter -- is supported by research. Specifically:
- Providing students with multiple ways to access content improves learning (Hattie, 2011).
- Providing students with multiple ways to demonstrate knowledge and skills increases engagement and learning, and provides teachers with more accurate understanding of students' knowledge and skills (Darling-Hammond, 2010).
- Instruction should be informed as much as possible by detailed knowledge about students' specific strengths, needs, and areas for growth (Tomlinson, 2014).
As our insatiable curiosity about the learning process persists and studies continue to evolve, scientific research may emerge that further elaborates on multiple intelligences, learning styles, or perhaps another theory. To learn more about the scientific research on student learning, visit our Brain-Based Learning topic page .
Darling-Hammond, L. (2010). Performance Counts: Assessment Systems that Support High-Quality Learning . Washington, DC: Council of Chief State School Officers.
Hattie, J. (2011). Visible Learning for Teachers: Maximizing Impact on Learning . New York, NY: Routledge.
Tomlinson, C. A. (2014). The Differentiated Classroom: Responding to the Needs of All Learners . Alexandria, VA: ASCD.
Resources From Edutopia
- Are Learning Styles Real - and Useful? , by Todd Finley (2015)
- Assistive Technology: Resource Roundup , by Edutopia Staff (2014)
- How Learning Profiles Can Strengthen Your Teaching , by John McCarthy (2014)
- An Interview with the Father of Multiple Intelligences , by Owen Edwards (2009)
Additional Resources on the Web
- Howard Gardner’s website
- Howard Gardner: ‘Multiple intelligences’ are not ‘learning styles’ (The Washington Post, 2013)
- Books published by Howard Gardner
- Multiple Intelligences Resources (ASCD)
- Project Zero (Harvard Graduate School of Education)
- Multiple Intelligences Research Study (MIRS)
- Multiple Intelligences Lesson Plan (Discovery Education)
- Multiple Intelligences Resources (New Horizons for Learning [NHFL], John Hopkins University)
CONCEPTUAL ANALYSIS article
Why multiple intelligences theory is a neuromyth.
- The College of New Jersey, Ewing Township, NJ, United States
A neuromyth is a commonly accepted but unscientific claim about brain function. Many researchers have claimed Howard Gardner’s multiple intelligences (MI) theory is a neuromyth because they have seen no evidence supporting his proposal for independent brain-based intelligences for different types of cognitive abilities. Although Gardner has made claims that there are dedicated neural networks or modules for each of the intelligences, nonetheless Gardner has stated his theory could not be a neuromyth because he never claimed it was a neurological theory. This paper explains the lack of evidence to support MI theory. Most important, no researcher has directly looked for a brain basis for the intelligences. Moreover, factor studies have not shown the intelligences to be independent, and studies of MI teaching effects have not explored alternate causes for positive effects and have not been conducted by standard scientific methods. Gardner’s MI theory was not a neuromyth initially because it was based on theories of the 1980s of brain modularity for cognition, and few researchers then were concerned by the lack of validating brain studies. However, in the past 40 years neuroscience research has shown that the brain is not organized in separate modules dedicated to specific forms of cognition. Despite the lack of empirical support for Gardner’s theory, MI teaching strategies are widely used in classrooms all over the world. Crucially, belief in MI and use of MI in the classroom limit the effort to find evidence-based teaching methods. Studies of possible interventions to try to change student and teacher belief in neuromyths are currently being undertaken. Intervention results are variable: One research group found that teachers who knew more about the brain still believed education neuromyths. Teachers need to learn to detect and reject neuromyths. Widespread belief in a neuromyth does not make a theory legitimate. Theories must be based on sound empirical evidence. It is now time for MI theory to be rejected, once and for all, and for educators to turn to evidence-based teaching strategies.
Introduction
Howard Gardner’s theory ( Gardner, 1983 , 1993 , 2011 ) of multiple intelligences is widely regarded as a neuromyth ( Geake, 2008 ; Dekker et al., 2012 ; Howard-Jones, 2014 ; Ruhaak and Cook, 2018 ; Blanchette Sarrasin et al., 2019 ; Rogers and Cheung, 2020 ; Craig et al., 2021 ; Menz et al., 2021 ). A neuromyth is a commonly accepted but unscientific claim about brain function that may be based on a misunderstanding of brain research findings ( Dekker et al., 2012 ). The goal of this paper is to explain why Howard Gardner’s Multiple Intelligences (MI) theory is a neuromyth.
Specifically, critics have claimed that Gardner’s neuromyth is his proposal that humans have independent brain-based intelligences for different types of cognitive abilities: linguistic; logical-mathematical; visual-spatial; bodily-kinesthetic; musical; interpersonal; intrapersonal; and naturalistic.
Although critics have labeled MI theory as a neuromyth, little evidence has been published to support this claim. Geake (2008) noted that one neuroscience study found that the frontal lobe governed different forms of cognition. From this Geake argued the intelligences could not have separate brain networks. Howard-Jones (2014) asserted that the “general processing complexity of the brain makes it unlikely that anything resembling Multiple Intelligences theory can ever be used to describe it” (p. 818), but he did not provide evidence of the brain’s complexity. Craig et al. (2021) , Dekker et al. (2012) , Ruhaak and Cook (2018) , and Blanchette Sarrasin et al. (2019) simply labeled MI theory as a neuromyth.
Rousseau (2021b) argued that MI theory itself was not a neuromyth. He stated that it was a “legitimate scientific theory of intelligence” (p. 4) that could be validated by finding the brain bases for the intelligences. However, Rousseau (2021b) claimed that it was a myth that matching students’ multiple intelligences to teaching methods could enhance learning.
The current criticisms of Gardner’s theory are not new ( Shaler, 2006 ; Waterhouse, 2006 ), and over time Gardner has taken four distinct unsatisfactory positions to defend against criticisms of his theory. One position was to ignore criticisms. Gardner asserted “For over a decade, I was content to let MI theory take on a life of its own. I had issued an ensemble of ideas (or “memes”) to the world, and I was inclined to let those memes fend for themselves” ( Gardner, 2011 , p. 79). A second position was to argue that empirical support for his theory was provided by his personal reading of research: “Gardner cast a wide net that included neuroscience, cognitive science, anthropology, and evolutionary sciences. This broader view allowed Gardner to reconceptualize intelligences” ( Gardner and Moran, 2006 , p. 229). But personal reading of research is how theories are created, not how they are validated. Empirical support for a theory requires testing the theory. Gardner’s third position was to tell researchers to read his previous writings to find an argument for the validity of his theory ( Gardner, 2020b ). He stated “there is an entire 400-page book Howard Gardner Under Fire in which I respond to these and other critiques ( Shaler, 2006 ). I would ask that both researchers and educators review these writings and exchanges before connecting the theory that I developed with the provocative, and contentious phrase “neuromyth” ( Gardner, 2020b , p. 3).
A fourth position has been Gardner’s continuing assertion ( Gardner, 2011 ) that MI theory could not be a neuromyth because he had never claimed there were brain bases for the intelligences. He stated “while brain evidence was cited in my original work, I have never claimed that “MI” is a neurological theory” ( Gardner, 2011 , p. 3). However, Gardner did make claims for the neural basis of the intelligences. Gardner argued “that the mind/brain consists of many modules/organs/intelligences, each of which operates according to its own rules in relative autonomy from the others” ( Gardner, 2011 , p. xxiii). Gardner also argued that patients with brain damage demonstrated that “various abilities can be destroyed, or spared, in isolation from other abilities” ( Gardner, 1993 , p. 7). Gardner defined each intelligence as a separate pattern of thinking, “where each operates according to its own procedures and has its own biological bases” ( Gardner, 2011 , p. 72). Importantly, Gardner asserted that each “intelligence …clearly involves processes that are carried out by dedicated neural networks. No doubt each of the intelligences has its characteristic neural processes” ( Gardner, 2020a , p. 94). Gardner specifically argued that “MI theory demands that linguistic processing, for example, occur via a different set of neural mechanisms than does spatial or interpersonal processing” ( Gardner, 2020a , p. 99), and Gardner and his colleagues ( Davis et al., 2011 ) proposed that there should be “an atlas of the neural correlates of each of the intelligences” (p. 495). Davis et al. also predicted that “The biological basis of the theory—its neural and genetic correlates—should be clarified in the coming years” ( Davis et al., 2011 , p. 498).
But, to date, no neural correlates of the intelligences have been found ( Waterhouse, 2006 ; Geake, 2008 ; Dekker et al., 2012 ; Howard-Jones, 2014 ; Ruhaak and Cook, 2018 ; Blanchette Sarrasin et al., 2019 ; Craig et al., 2021 ; Rousseau, 2021b ). Consequently, until researchers find evidence that “each of the intelligences has its characteristic neural processes” ( Gardner, 2020b , p. 94), MI theory is and will remain a neuromyth. And until each intelligence is shown to have its own unique brain processing, there is no basis for the superiority of MI teaching strategies. Despite the lack of evidence for multiple intelligences, Ruhaak and Cook (2018) reported that 90% of teacher trainees surveyed in the United States planned to use MI teaching strategies, and Blanchette Sarrasin et al. (2019) reported that 94% of teachers surveyed in Quebec stated they used MI theory in the classroom. Others, including Abenti (2020) , Hanafin (2014) , Shearer (2020a) , and Yavich and Rotnitsky (2020) all have argued that use of MI theory in the classroom was effective and important. Shearer noted that many educators “continue to alter lesson plans, re-envision curriculum and design whole schools inspired by the multiple intelligences” ( Shearer, 2020a , p. 49). But because MI theory has not been validated, significant efforts should be made to dispel the widespread belief in MI theory and the belief in the effectiveness of MI-inspired teaching strategies.
Three lines of evidence undermine MI theory
Three lines of evidence presented here explain why MI theory is a neuromyth. Each line of evidence counters a specific belief about multiple intelligences. One, although there is a pervasive public belief that there are distinct multiple intelligences, studies have shown that the intelligences do not function separately from one another. Two, although many educators believe in the effectiveness of MI teaching strategies, these strategies have not been studied appropriately and cannot prove the intelligences exist. Three, although many educators and researchers believe there is a brain basis for each intelligence, this is improbable because neuroscience research has shown that the brain is organized in complex multifunction networks, and multifunction networks, as understood now, preclude the possibility of separate neural networks for the individual intelligences.
The paper begins with a brief review of the lack of standard measures for the intelligences, and then discusses each of the three lines of evidence that makes MI theory a neuromyth. Following this, the paper explains the brain’s multifunction networks. The paper concludes with a discussion of possible ways to dispel the belief that MI theory is a good basis for teaching.
There are no standard measures of the intelligences
Having a standard measure for each of the intelligences is a requirement for exploring the validity of MI theory, and Gardner et al. (1998) did create measures to assess the intelligences, but these measures did not become standard measures. Davis et al. (2011) argued that standard measures of the multiple intelligences were needed, and encouraged the development of standard measures. Gardner, however, argued that it was up to others to create measures of the intelligences, and said he “would prefer to spend more resources helping learners understand and develop their individual intelligence profiles…. He has made the personal decision not to become directly involved in testing” ( Gardner and Moran, 2006 , p. 230). Gardner and Moran (2006) claimed it was hard to test the intelligences by psychometric means, i.e., “paper-and-pencil assessments” (p. 230). Nonetheless, Gardner (2011) stated that “as psychologists have increased their tools for measuring intelligence, psychometric evidence in favor of multiple intelligences has accrued” (p. 40).
Teele (1992) published the Teele Inventory of Multiple Intelligences (TIMI). This psychometric test of the intelligences was used in 1,000 schools. However, McMahon et al. (2004) evaluated the TIMI and discovered that “Reliability analyses for each of the subscales of the TIMI suggested that the instrument does not provide consistent measurement” (p. 48). They concluded that the poor reliability of the TIMI meant that it should not be used by educators.
At present there are no standard measures of the intelligences, thus individual researchers have to create their own measures for the intelligences. Unfortunately, without standard measures, MI study findings cannot be compared to one another. Also, the lack of standard measures means that no synthesis of MI research findings can be built.
Evidence for the independence of the intelligences is lacking
Gardner (1983 , 1993 , 2011 , 2017 , 2020a) claimed that the intelligences were independent abilities, and Gardner stated that evidence for “the independence of an intelligence comes from studies that identify individuals who either excel at, or lack, a certain capacity, as well as “dedicated” neural regions that appear to subserve these capacities” ( Gardner, 2011 , p. 50). Gardner and Walters stated that the independence of the intelligences was a crucial component of MI theory, and they worried that if there were “a significant correlation among these faculties, as measured by appropriate assessments, the supposed independence of the faculties would be invalidated” ( Gardner and Walters, 1993 , p. 38).
Importantly, the independence of the intelligences is crucial for MI theory, because if the intelligences are intercorrelated they do not exist as theorized. But only a few studies have explored the independence of the intelligences, and these studies were not based on shared standard measures of the intelligences. A factor analysis by Plucker et al. (1996) did report evidence for independent linguistic, logical–mathematical and spatial subscales. However, Visser et al. (2006a) analyzed the score correlations of 200 individuals on their measures of each of the eight intelligences, and they found that test scores representing most of the intelligences were intercorrelated “despite representing different domains of Gardner’s framework” (p. 500). Visser et al. concluded that their findings “contradict Gardner’s assertion that there are at least eight independent intelligence domains” ( Visser et al., 2006a , p. 501).
Gardner (2006) claimed that Visser et al. (2006a) only found intercorrelations for the intelligences because they used measures that relied on two of the multiple intelligences—linguistic and logical-mathematical. Visser et al. (2006b) , however, responded that intercorrelations of the intelligences depended on the association of individual differences in task performance, and not on whether a test item used language or reasoning. Visser et al. (2006b) , also noted that Gardner had not established measures for his eight intelligences, and the researchers requested that Gardner should do so. However, as noted above, no standard measures of the intelligences have yet been developed.
Almeida et al. (2010) conducted a confirmatory factor analysis on scores from a set of Gardner’s multiple intelligence assessment tasks, including linguistic, logical, visual/spatial, bodily-kinesthetic, naturalistic and musical intelligences, and scores from the Battery of General and Differential Aptitudes (BADyG), including reasoning, memory, verbal aptitude, numerical aptitude and spatial aptitude, in a sample of 294 children. Almeida et al. (2010) found that there was no general common factor across the two test batteries. Instead, the researchers found that there were two factors, one for the BADyG measures, and another for the multiple intelligence assessment scores. The single multiple intelligence factor suggests that the individual intelligences are not independent of one another.
Castejon et al. (2010) explored the construct validity of the multiple intelligences through confirmatory factor analysis of measures of the intelligences that they had developed. They reported that no model exhibited a totally satisfactory fit to the empirical data, but the best fitting factor structure was a model that was based in the intercorrelation of the intelligences, along with some individuation. The researchers ( Castejon et al., 2010 ) concluded that their analyses demonstrated that the intelligences are not truly independent of one another. Consequently, to date, no clear division of cognition into separate intelligences has been proven.
Belief in MI teaching strategies is strong but is not supported by valid evidence
Educators have continued to use MI teaching methods because they believe that there are separate brain-based intelligences that vary in strength for each individual ( Attwood, 2020 ; Mavrelos and Daradoumis, 2020 ). These beliefs support the idea that teaching to a student’s stronger intelligences will be more effective. For example, Shearer stated that “multiple intelligences serve as ‘levers’ to personalize important cognitive processes underlying learning” ( Shearer, 2020a , p. 57), and Gardner argued that the “intelligences can function…as the preferred means for inculcating diverse subject matter” ( Gardner, 2011 , p. 409). He asserted that computer programming would be better taught to an individual’s strongest intelligence. He stated that “an individual with a strong musical bent might best be introduced to programming by attempting to program a simple musical piece” and that “An individual with strong spatial abilities might be initiated through … a flow chart or some other spatial diagram” ( Gardner, 2011 , p. 409). A ‘strong musical bent’ and ‘strong spatial abilities’ mean better musical and spatial intelligence skills which stem from more effective neural networks dedicated to musical intelligence and visual-spatial intelligence.
Even though Gardner and colleagues stated that “Individualized education does not depend on the existence of MI theory” ( Davis et al., 2011 , p. 499), teacher training continues to promote MI theory as an effective basis for individualized lessons ( Sheahan et al., 2015 ; Armstrong, 2018 ; Abenti, 2020 ; Attwood, 2020 ). Myriad educators and researchers believe that “teachers should be encouraged to learn about student engagement strategies which MI theory provides” ( Attwood, 2020 , p. 6), and they have argued that individualized and non-individualized MI teaching strategies are more effective than standard instruction. For example, Yavich and Rotnitsky (2020) asserted that MI teaching strategies can significantly contribute to “the development of logical, critical and creative thinking abilities, as well as the development of high levels of thinking” (p. 111), and Abenti (2020) reviewed studies of MI teaching strategies and concluded that MI teaching methods are the best way to “educate the most people in any education environment” (p. 32).
Problems for studies of MI teaching strategies
There are many published papers addressing the use of MI theory in the classroom. A search of databases on January 14, 2023 using the query ‘multiple intelligences classroom’ for the time period 1983 to 2022 found 407 papers on Education Full Text, and 92 papers on PubMed. Most of the published classroom studies found improved learning through use of MI teaching methods. Bas (2016) , Batdi (2017) , and Ferrero et al. (2021) conducted meta-analyses of studies of the effectiveness of using MI in the classroom, and all three meta-analyses reported many studies with positive results for MI teaching. Some MI teaching strategies have focused on teaching students the different intelligences. For example, Winarti et al. (2019) compared student improvement in the eight intelligences when taught by MI strategies with student improvement when not taught using MI strategies. Students taught by MI strategies showed significant improvement in measures of six intelligences—intrapersonal, interpersonal, kinesthetic, visual spatial, musical intelligence, and linguistic intelligence. By contrast, there was no improvement in the control group for any of the eight intelligences.
Most MI teaching strategies, however, follow Gardner’s (2011) argument that the intelligences be used “for inculcating diverse subject matter” (p. 409). For example, Sheahan et al. (2015) conducted a study of first year nursing students who were learning clinical skills. The researchers reported that 46 first year nursing students taught by multiple intelligence strategies received significantly higher scores on three structured clinical examination tests than a control group of 44 first year nursing students taught by conventional methods.
However, the apparent success of matching a student’s stronger intelligence to specific task can cannot prove the existence of multiple intelligences because there is no evidence that the intelligences are independent ( Visser et al., 2006a ; Almeida et al., 2010 ; Castejon et al., 2010 ), and no evidence that there is a brain basis for each intelligence ( Waterhouse, 2006 ; Geake, 2008 ; Dekker et al., 2012 ; Howard-Jones, 2014 ; Ruhaak and Cook, 2018 ; Blanchette Sarrasin et al., 2019 ; Craig et al., 2021 ; Rousseau, 2021b ). Davis et al. argued “because any educational intervention is multifaceted, it is not possible to attribute school success or failure strictly to MI interventions” ( Davis et al., 2011 , p. 498). In fact, it is unlikely that any enhanced learning is due to the existence of multiple intelligences. Where MI intervention studies have reported evidence of enhanced learning, it is likely that learning is actually enhanced by other well-known methods that have been tested and found effective. These include, but are not limited to, the extended repetition of information, greater student attention to novel teaching strategies, increased personal attention from the instructor, and the greater enthusiasm of teachers using new teaching methods. Importantly, cognitive neuroscience research has established that repetition enhances learning ( Zhan et al., 2018 ; Adams and Delany, 2023 ), and that there is a novelty ‘switch’ in the brain that enhances the processing of something new in the environment ( Gómez-Ocádiz et al., 2022 ). Learning is also enhanced when there is individual attention to a student by a teacher ( Schacter, 2000 ), and when excitement occurs at the time the material is presented ( Perugini et al., 2012 ; Leventon et al., 2018 ).
Studies of MI teaching strategies ( Ferrero et al., 2021 ) have not controlled for any of these many likely alternate causes for enhanced learning. Also problematic, Ferrero et al. (2021) found that most of the MI teaching studies they reviewed in their meta-analysis could not prove the effectiveness of MI for enhancing learning because these studies did not have large enough sample sizes, appropriate control groups, or reliable and valid outcome measures. The researchers warned that sound studies of MI in the classroom were needed before “its use in the classroom can be recommended or promoted” ( Ferrero et al., 2021 , p. 12).
There are no empirical studies of the brain basis of MI theory
As stated above, Gardner’s claims for the neural basis of the intelligences led educators and researchers to believe that each of the eight intelligences had a distinct brain basis. Armstrong (2018) stated the intelligences were “eight relatively autonomous brain systems” (p. 14), where each intelligence has “its roots deeply embedded in the evolution of human beings” (p. 22) and Shearer (2018) claimed that “each of the eight intelligences have unique neural architectures” (p. 3). However, Rousseau (2021b) and Waterhouse (2006) argued that empirical research was needed to test the belief that there is a dedicated brain network for each intelligence. Ideally, a brain validity test of MI theory should measure brain processing while individuals are engaged in behaviors that characterize a particular intelligence ( Waterhouse, 2006 ).
Although Davis et al. (2011) had declared that the brain basis of MI theory “should be clarified in the coming years” (p. 498), and although Rousseau (2021b) argued that studies should test for brain bases for the multiple intelligences, Gardner (2020b) made two claims that, if accepted, would effectively block future studies to test MI theory.
One, he claimed MI theory cannot be evaluated by research findings from cognitive psychology and neuroscience. Even though Gardner and Moran (2006) declared that MI theory “encompasses cognitive and developmental psychology, differential psychology, (and) neuroscience” (p. 227), nonetheless, Gardner (2020b) argued that MI theory could not be evaluated by research findings from cognitive psychology and neuroscience. He claimed this research used study samples that were too risky. He asserted that “Much neural evidence comes from studies of animals…. But even when the population that has been studied consists of Homo sapiens , generalizations are risky” ( Gardner, 2020b , p. 3). However, Gardner’s concern for the riskiness of generalizations from these study samples is unfounded. Research using samples of animals and humans have been the source of most of our current valid knowledge of the brain basis of cognition ( Gazzaniga et al., 2018 ). Thus, MI theory can be evaluated by findings from cognitive psychology and cognitive neuroscience research. If his theory cannot be evaluated by scientific findings it remains a personal philosophy and an untested speculation.
Two, Gardner claimed that because MI theory is a scientific theory with educational implications, it cannot be tested for validity by standard research methods. Gardner (2020b) argued that a scientific theory with educational implications, such as MI theory, was different from a purely scientific theory. Gardner asserted that purely scientific theories could be supported or countered by research, but theories about the brain with educational implications could not be similarly tested because an “educational claim turns out to be either circular or vacuous. Indeed, once one moves from “science” to “education” one has indubitably entered the realm of cultural values” ( Gardner, 2020b , p. 2).
However, a scientific claim about the brain with educational implications can be supported or countered by research without entering the realm of cultural values. For example, one important scientific theory about the brain that has educational implications argues that student repetition of information enhances learning through brain activity changes. For example, a study by Zhan et al. (2018) found that repetition of information did improve long term learning, and repetition was correlated with significantly increasing activation in the hippocampus, as well as increased connectivity between the hippocampus and other brain regions. Another important scientific theory about brain activity with educational implications is that learning is enhanced by brain activity that occurs while we sleep—even during naps. Klinzing et al. (2019) reviewed a wide range of studies of the effect of sleep on learning, and concluded that what we learn while awake is repeated during slow wave sleep (SWS) and this neuronal replay while we sleep strengthens the information flow to brain networks, thus enhancing learning. Importantly, studies like those of Zhan et al. (2018) and Klinzing et al. (2019) can be supported or countered by future research.
Although future studies to explore the brain basis of the intelligences could be conducted, they will not stem from past or ongoing research, because, as noted above, there is no evidence that empirical brain validity studies have been done ( Waterhouse, 2006 ; Rousseau, 2021b ). While it is possible there may be MI neural validity studies, none have been cited by Gardner (1993 , 2011 , 2020a , b) . Moreover, evidence that validity studies have not been done can be seen in the lack of published studies. On January 10, 2023, using the query “validity Gardner multiple intelligences,” for the time period 1983–2022, the database PubMed yielded only two published papers, neither of which were validity studies. And for the same query on the same date, the database Education Full Text yielded just nine papers published between 1983 and 2022, and among these nine papers, only one, Shearer (2020b) , provided an assessment of MI neural validity. However, Shearer’s paper ( Shearer, 2020b ) does cite another Shearer study— Shearer and Karanian (2017) .
Shearer and Karanian (2017) and Shearer (2020b) reported that characteristics of the intelligences were aligned with the functions of cognitive brain networks. However, because alignment studies do not measure brain processing while individuals are engaged in activities that characterize a particular intelligence, these studies are not true validity studies, and thus cannot prove that the intelligences have dedicated neural networks.
Shearer and Karanian (2017) reviewed 318 studies of brain regions and reported that brain region functions were aligned with the proposed functions of each of the eight intelligences. They concluded that their analysis of the 318 studies provided “robust evidence that each of the eight intelligences possesses its own unique neural architecture” ( Shearer and Karanian, 2017 , p. 221). However, there are problems with Shearer and Karanian’s methods and conclusions. First, the researchers did not adequately describe how they selected the 318 neuroscience studies. They simply stated “Several extensive meta-analysis and topic reviews served as guides for finding pertinent studies in the target area” ( Shearer and Karanian, 2017 , p. 212). Second, the researchers claimed the unique neural architecture for linguistic intelligence included eight brain regions—temporal cortex, frontal cortex, parietal cortex, occipital cortex, the subcortical region, the cerebellum, the cingulate cortex, and the insular cortex. These eight regions comprise nearly the entire brain. Although language processing involves many links between multifunction networks across different regions of the brain ( Popham et al., 2021 ; Rolls et al., 2022 ; Wang et al., 2022 ), the entire brain is not a unique neural architecture dedicated to language processing.
A similar alignment study was conducted by Shearer (2020b) . He reviewed 48 studies of brain connectivity, and argued that “neural regions cited by Gardner’s original research in 1983 are among the same brain structures identified by modern neuroimaging technologies for each intelligence” (p. 142). He stated that “7–15 neural networks were found to be well aligned with seven of the eight multiple intelligences” ( Shearer, 2020b , p. 127). Shearer (2020b) claimed that these seven alignments were: (1) visual–spatial intelligence with visual networks and subnetworks; (2) kinesthetic intelligence with primary motor, sensorimotor, cerebellum, and basal ganglia networks; (3) logical-mathematical intelligence with fronto-parietal and executive control networks; (4) musical intelligence with the auditory network; (5 and 6) intrapersonal and interpersonal intelligences with a network for internal self-awareness and social perceptions; and (7) linguistic intelligence with the language network.
The key problem with both Shearer and Karanian’s study ( Shearer and Karanian, 2017 ) and Shearer’s study ( Shearer, 2020b ) is that the brain networks they have described are significantly oversimplified. Gardner’s linguistic intelligence cannot be aligned with “the language network,” because there is no isolated single “language network” in the brain ( Popham et al., 2021 ; Rolls et al., 2022 ; Wang et al., 2022 ). Networks for language and other forms of cognition are shared, and language processing occurs in the same networks that also process many other cognitive tasks such as mathematics, music, and logic, and varied perceptual and motor skills as well. For example, syntax, or word order, is generated by brain network activity in the inferior frontal gyrus region and the left orbitofrontal region. And syntax processing networks are driven by two word-level networks: the network that governs visual object meaning; and the network that governs visual motion, and auditory, and somatosensory semantic processes ( Rolls et al., 2022 ). Adding to the complexity of these processes, the brain networks governing syntax also serve as hubs for social perception and cognition, including the perception of faces and human motion, as well as the understanding others’ actions, and mental states.
Another example of the complexity of language processing in the brain is that the network for understanding the meaning of verbs also provides control for our motor actions. Specifically, Wang et al. (2022) reported that the inferior parietal lobe supports verb generation and verb retrieval, and the inferior parietal lobe also processes abstract information of object-directed actions, and it governs action organization and understanding the intention of others’ actions.
Because the varied neural networks that govern language processing also govern many other forms of cognition, a unitary dedicated language network cannot be isolated.
Multifunction brain networks
There are complex multifunction networks for all aspects of cognition. However, this complexity was not evident 40 years ago. Neuroscientists in the 1980s and 1990s theorized that there were modular cognitive mechanisms in the brain. Fodor (1983) defined cognitive modules as “domain-specific, innately specified, hardwired, autonomous” (p. 36). Fodor (1983) also defined cognitive modules as “informationally encapsulated” (p. 64) containing specific information within a “fixed neural architecture” (p. 98), and Sperber (1994) similarly stated that “The function of a module is to process a specific range of information in a specific manner” (p. 52).
Thus, Gardner’s MI theory was not a neuromyth in 1983 or 1993 because it was based on then current theories of brain modularity for cognition, and at that time few researchers were concerned by the absence of neural validity studies ( Waterhouse, 2006 ). However, since then evidence has demonstrated that the brain is not organized in cognitive modules uniquely dedicated to different forms of content ( Anderson, 2014 ; Sporns and Betzel, 2016 ; Zerilli, 2017 ; Elimari and Lafargue, 2020 ; Holyoak and Monti, 2021 ; Wang et al., 2021 ; Roy et al., 2022 ). Therefore, the lack of brain validity for the multiple intelligences has become a serious concern, and has led to the claims that MI theory is a neuromyth.
The word module is still used in cognitive neuroscience research. However, its current use does not mean a cognitive module as proposed by Sperber (1994) or Fodor (1983) . Instead, the word module now is applied to many varied groups of biological elements within the brain, including groups of interacting proteins, or sets of genes that work together in cellular networks, or neuron assemblies that are “building blocks in the organization of brain networks” ( Sporns and Betzel, 2016 ).
The idea of complex networks for cognition was proposed by Lieberman (2002) . He suggested that assemblies of subcortical neurons in basal ganglia and cerebellum, and in many separate cortical regions shared control of many different complex behaviors including walking, talking, gesturing, reasoning, speaking, tool-making, and comprehending the meaning of sentences. As discussed above, there are now many lines of evidence demonstrating the shared complex brain networks for the processing of information. Wang et al. (2021) reported evidence for the current model of switching between brain integration and segregation. This model argues that individual forms of cognition occur in a process of constant shifts between large networks and small networks, and not in separate content-dedicated static modules. Roy et al. (2022) found evidence that a single memory is stored in multiple coded information units that are widely distributed across the brain. Holyoak and Monti (2021) reported evidence that mental representations of ideas, regardless of specific content—i.e., language, logic, and mathematics—involve neural activity that is distributed across the parietal and frontal lobes of the brain. Anderson et al. (2013) conducted a meta-analysis of more than 2,000 functional imaging studies of the brain and discovered functional diversity at every location in the brain. Specifically, the researchers reported that language, vision, emotion, and mathematics did not have discrete local specialization. Instead, they found that each region of the brain supported a varied array of tasks, where multiple regions and networks supported different components of cognitive, perceptual, and motor tasks or skills.
A likely cause for the functional diversity of many brain networks is the evolution of the brain through exaptation. Exaptation is the reuse of neurons in existing brain networks as the basis for new networks to support the additional processing activity needed for new skills and new knowledge ( Zerilli, 2017 ). Exaptation is adaptive because it reduces the amount of glucose energy needed to build new brain networks. It is likely that exaptation is the cause of the layering of varied perceptual, cognitive and motor functions in multi-use brain networks that govern many varied forms of thinking and action. And Elimari and Lafargue (2020) claimed that the most recently evolved cognitive skills, such as language and mathematics, rely on a “greater number and diversity of neural structures” (p. 11).
What will convince educators that MI theory is a neuromyth?
Even though Gardner has consistently argued that the intelligences are real ( Gardner, 1983 , 1993 , 2011 ), surprisingly Gardner (2011) also asserted the “intelligences are fictions—at most, useful fictions—for discussing processes and abilities that (like all of life) are continuous with one another” (p. 74).
As outlined here, studies have not confirmed that the intelligences are real. Factor studies have not shown the intelligences to be independent. Studies of MI teaching effects have not explored alternate causes for positive effects and have not been conducted by standard scientific methods. No research has directly looked for a brain basis for the intelligences. Most important, neuroscience research has shown that the brain is not organized in separate modules dedicated to specific forms of cognition. Consequently, MI theory is a neuromyth that should not be taught to teachers, and MI teaching strategies should not be used in the classroom.
Because so many educators use and value MI teaching strategies, it is likely that many teachers and researchers do not know the evidence that has made MI theory a neuromyth. Craig et al. (2021) reported that only 25% of teachers surveyed in Canada, the United States, and the United Kingdom believed MI theory was a neuromyth. Dekker et al. (2012) found that teachers who knew more about the brain still believed education neuromyths. The researchers also reported that “teachers who are enthusiastic about the possible application of neuroscience findings in the classroom, often find it challenging to distinguish pseudoscience from scientific facts” ( Dekker et al., 2012 , p. 6). And Ruhaak and Cook (2018) found that teachers’ “knowledge of neuromyths—not general knowledge of the brain—was associated with greater likelihood of implementing more effective instructional practices than ineffective, neuromyth-based approaches” (p. 160).
Many interventions have attempted to change teachers’ belief in neuromyths. Rousseau (2021a) conducted a comprehensive review of interventions for fostering conceptual change in teachers that would improve their ability to recognize and refute neuromyths. Rousseau (2021a) reported that “The high prevalence of beliefs in neuromyths among educators did not decline over the past decade” (p. 9), and his review of intervention research led him to conclude that much more data was needed to understand what teaching processes would be most effective in changing teachers’ minds. Rousseau’s (2021a) review of interventions revealed that neither the direct refutation of neuromyths nor training in neuroscience had been shown to be consistently effective in reducing students’ belief in neuromyths. Rousseau reported, however, that although an undergraduate course in neuroscience was “insufficient to reduce beliefs in neuromyths” ( Rousseau, 2021a , p. 8), “training in the context of teacher professional development (workshops, seminars) looks promising” ( Rousseau, 2021a , p. 8). Dubinsky et al. (2022) argued that teachers needed to learn current concepts of neuroscience, and posited that “where teachers are given the opportunity to discuss what the physiological neuroscience concepts might mean for their own classrooms, strong connections between neuroscience principles and effective pedagogical practices have been documented” (p. 272). Rousseau (2021a) stated that “Given that intuitive/anecdotal evidence might contribute to consolidate false beliefs through a variety of cognitive biases (availability, familiarity, confirmation biases), some intervention approaches focus upon making educators more aware of the propensity of the human mind to rely on intuitive thinking at the expense of rational thinking” (p. 6). Rousseau (2021a) argued that it is important to break through the intuitive beliefs many students have for neuromyths, and he recommended that teacher training programs address anecdotal evidence and focus on cognitive biases.
Craig et al. (2021) , Dekker et al. (2012) , Goswami (2006) , Grospietsch and Mayer (2018) , Howard-Jones (2014) , Ruhaak and Cook (2018) , and Blanchette Sarrasin et al. (2019) have also outlined various possible strategies to undo or reduce belief in neuromyths. Howard-Jones (2014) argued that that neuroscientists and educators should work together to establish a new field of educational neuroscience which could address neuromyths and “encourage scientific insight regarding the relationship of neural processes to the complex behaviors that are observed in the classroom” (p. 822). Dekker et al. (2012) recommended that research should discover what sources, such as books, colleagues, and commercial education companies, lead teachers to know and believe neuromyths. Dekker et al. (2012) also recommended that there be interdisciplinary connections between neuroscientists and educators. Goswami argued that learning current neuroscience findings was very important for educators ( Goswami, 2019 ), but had earlier cautioned that neuroscientists were not appropriate instructors for dispelling neuromyths because they provide “too much data” ( Goswami, 2006 , p. 412), and “are not necessarily gifted at communicating with society at large” (p. 6). However, Goswami (2006) recommended that educators should establish a group of “ex-scientists with an interest in education, perhaps attached to universities or to national education departments. They could fulfill a dual role: interpreting neuroscience from the perspective of and in the language of educators, and feeding back research questions and ideas from educators to neuroscientists” (p. 413).
Goswami (2006) cautioned that “Most teachers prefer broad brush messages with a ‘big picture,’ and being ‘told what works’” (p. 412). Blanchette Sarrasin et al. (2019) recommended that teachers should be trained in critical thinking, and that education textbooks should include current knowledge about the neuroscience of learning, and clear refutations of neuromyths. Similarly, Craig et al. (2021) argued that teacher training should include information that helps teachers be critical thinkers about educational neuroscience. And Craig et al. (2021) asserted that because school psychologists are trained in evaluating research and determining the evidence base for interventions, therefore “school psychologists are obligated to take action to de-implement harmful or wasteful practices in schools, drawing on their diverse and multi-faceted training across the domains of education, psychology, and neuroscience” (p. 136).
Dekker et al. (2012) proposed that it was important to develop and test interventions that explain neuromyths and teach educators evidence-based teaching methods, and this is happening with varying degrees of success ( Rousseau, 2021a ). Educators need to learn to detect and reject neuromyths, and neuroscientists and educators should work together to establish a new field of educational neuroscience. Ideally, Gardner or an MI theory adherent should publish a paper or book in which the lack of evidence for multiple intelligences is accepted, reviewed and addressed. However, this is unlikely to happen. Nonetheless, the widespread belief in multiple intelligences inhibits the development of effective, evidence-based teaching strategies, and must be dispelled.
Conflict of interest
The author declares that the research was conducted in the absence of any commercial or financial relationships that could be construed as a potential conflict of interest.
Publisher’s note
All claims expressed in this article are solely those of the authors and do not necessarily represent those of their affiliated organizations, or those of the publisher, the editors and the reviewers. Any product that may be evaluated in this article, or claim that may be made by its manufacturer, is not guaranteed or endorsed by the publisher.
Author contributions
The author confirms being the sole contributor of this work and has approved it for publication.
Abenti, H. F. (2020). How do I teach you? An examination of multiple intelligences and the impact on communication in the classroom. Lang. Commun. 73, 29–33. doi: 10.1016/j.langcom.2020.04.001
CrossRef Full Text | Google Scholar
Adams, R. L., and Delany, P. F. (2023). Do we remember when to better recall what? Repetition benefits are probably not due to explicit temporal context memory. J. Mem. Lang. 131:104415. doi: 10.1016/j.jml.2023.104415
Almeida, L. S., Prieto, M. D., Ferreira, A. I., Bermejo, M. R., Ferrando, M., and Ferrándiz, C. (2010). Intelligence assessment: Gardner multiple intelligence theory as an alternative. Learn. Individ. Differ. 20, 225–230. doi: 10.1016/j.lindif.2009.12.010
Anderson, M. L. (2014). After phrenology: Neural reuse and the interactive brain . Cambridge, MA: MIT Press.
Google Scholar
Anderson, M. L., Kinnison, J., and Pessoa, L. (2013). Describing functional diversity of brain regions and brain networks. NeuroImage 73, 50–58. doi: 10.1016/j.neuroimage.2013.01.071
PubMed Abstract | CrossRef Full Text | Google Scholar
Armstrong, T. (2018). Multiple intelligences in the classroom 4. Alexandria Virginia: ASCD.
Attwood, A. I. (2020). A conceptual analysis of the semantic use of multiple intelligences theory and implications for teacher education. Front. Psychol. 13:920851. doi: 10.3389/fpsyg.2022.920851
Bas, G. (2016). The effect of multiple intelligences theory–based education on academic achievement: a meta–analytic review. Educ. Sci. Theory Pract. 16, 1833–1864. doi: 10.12738/estp.2016.6.0015
Batdi, V. (2017). The effect of multiple intelligences on academic achievement: a meta–analytic thematic study. Educ. Sci. Theory Pract. 17, 2057–2092. doi: 10.12738/estp.2017.6.0104
Blanchette Sarrasin, J., Riopel, M., and Masson, S. (2019). Neuromyths and their origin among teachers in Quebec. Mind Brain Educ. 13, 100–109. https://doi:10.1111/mbe.12193. doi: 10.1111/mbe.12193
Castejon, J. L., Perez, A. M., and Gilar, R. (2010). Confirmatory factor analysis of project spectrum activities. A second-order g factor or multiple intelligences? Intelligence 38, 481–496. doi: 10.1016/j.intell.2010.07.002
Craig, H. L., Wilcox, G., Makarenko, E. M., and Mac Master, F. P. (2021). Continued educational neuromyth belief in pre-and in-service teachers: a call for de-implementation action for school psychologists. Can. J. Sch. Psychol. 36, 127–141. doi: 10.1177/0829573520979605
Davis, K., Christodoulou, J., Seider, S., and Gardner, H. (2011). “The theory of multiple intelligences” in The Cambridge handbook of intelligence . eds. R. J. Sternberg and S. B. Kaufman (New York: Cambridge University Press), 485–503.
Dekker, S., Lee, N. C., Howard-Jones, P., and Jolles, J. (2012). Neuromyths in education: prevalence and predictors of misconceptions among teachers. Front. Psychol. 3:29. doi: 10.3389/fpsyg.2012.00429
Dubinsky, J. M., Roehrig, G., and Varma, S. (2022). Neuroscience in teacher knowledge and education. Mind Brain Educ. 16, 267–276. doi: 10.1111/mbe.12334
Elimari, N., and Lafargue, G. (2020). Network neuroscience and the adapted mind: rethinking the role of network theories in evolutionary psychology. Front. Psychol. 11:545632. doi: 10.3389/fpsyg.2020.545632
Ferrero, M., Vadillo, M. A., and León, S. P. (2021). A valid evaluation of the theory of multiple intelligences is not yet possible: problems of methodological quality for intervention studies. Intelligence 88:101566. doi: 10.1016/j.intell.2021.101566
Fodor, J. A. (1983). The modularity of mind: An essay on faculty psychology . Cambridge, MA: MIT Press.
Gardner, H. (1983). Frames of mind: A theory of multiple intelligences . New York: Basic Books.
Gardner, H. (1993). Multiple intelligences: The theory in practice . New York: Basic Books.
Gardner, H. (2006). On failing to grasp the core of MI theory: a response to Visser et al. Intelligence 34, 503–505. doi: 10.1016/j.intell.2006.04.002
Gardner, H. (2011). Frames of mind: A theory of multiple intelligences . New York: Basic Books.
Gardner, H. (2017). Taking a multiple intelligences (MI) perspective. Behav. Brain Sci. 40:e203. doi: 10.1017/S0140525X16001631
Gardner, H. (2020a). Intelligence reframed: Multiple intelligences for the 21st century . New York: Basic Books.
Gardner, H. (2020b). “Neuromyths”: a critical consideration. Mind Brain Educ. 14, 2–4. doi: 10.1111/mbe.12229
Gardner, H., Feldman, D. H., and Krechevsky, M. (1998). Project zero frameworks for early childhood education : Volume 3, Project Spectrum preschool assessment handbook. Teachers College Press.
Gardner, H., and Moran, S. (2006). The science of multiple intelligences theory: a response to Lynn Waterhouse. Educ. Psychol. 41, 227–232. doi: 10.1207/s15326985ep4104_2
Gardner, H., and Walters, J. (1993). “Questions and answers about multiple intelligences theory” in Multiple intelligences: The theory in practice . ed. H. Gardner (New York: Basic Books), 35–48.
Gazzaniga, M., Ivry, R. B., and Mangun, G. R. (2018). Cognitive neuroscience: The biology of the mind . 5th Edn. New York: W. W Norton and Company.
Geake, J. (2008). Neuromythologies in education. Education Research 50, 123–133. doi: 10.1080/00131880802082518
Gómez-Ocádiz, R., Trippa, M., Zhang, C. L., Posani, L., Coco, S., Monasson, R., et al. (2022). A synaptic signal for novelty processing in the hippocampus. Nat. Commun. 13:4122. doi: 10.1038/s41467-022-31775-6
Goswami, U. (2006). Neuroscience and education: from research to practice? Nat. Rev. Neurosci. 7, 406–413. doi: 10.1038/nrn1907
Goswami, U. (2019). Cognitive development and cognitive neuroscience: The learning brain . Abingdon, UK: Routledge.
Grospietsch, F., and Mayer, J. (2018). Professionalizing pre-service biology teachers’ misconceptions about learning and the brain through conceptual change. Educ. Sci. 8:120. doi: 10.3390/educsci8030120
Hanafin, J. (2014). Multiple intelligences theory, action research, and teacher professional development: the Irish MI project. Aust. J. Teach. Educ. 39, 126–142. doi: 10.14221/ajte.2014v39n4.8
Holyoak, K. J., and Monti, M. M. (2021). Relational integration in the human brain: a review and synthesis. J. Cogn. Neurosci. 33, 341–356. doi: 10.1162/jocn_a_01619
Howard-Jones, P. A. (2014). Neuroscience and education: myths and messages. Nat. Rev. Neurosci. 15, 817–824. doi: 10.1038/nrn3817
Klinzing, J. G., Niethard, N., and Born, J. (2019). Mechanisms of systems memory consolidation during sleep. Nat. Neurosci. 22, 1598–1610. doi: 10.1038/s41593-019-0467-3
Leventon, J. S., Camacho, G. L., Ramos Rojas, M. D., and Ruedas, A. (2018). Emotional arousal and memory after deep encoding. Acta. Psycholol. 188, 1–8. doi: 10.1016/j.actpsy.2018.05.006
Lieberman, P. (2002). On the nature and evolution of the neural bases of human language. Supplement Yearbook Phys. Anthropol. 119, 36–62. doi: 10.1002/ajpa.10171
Mavrelos, M., and Daradoumis, T. (2020). Exploring multiple intelligence theory prospects as a vehicle for discovering the relationship of neuroeducation with imaginative/Waldorf pedagogy: a systematic literature review. Educ. Sci. 10, 1–26. doi: 10.3390/educsci10110334
McMahon, S. D., Rose, D. S., and Parks, M. (2004). Multiple intelligences and reading achievement: an examination of the Teele inventory of multiple intelligences. J. Exp. Educ. 73, 41–52. doi: 10.3200/JEXE.71.1.41-52
Menz, C., Spinath, B., and Seifried, E. (2021). Misconceptions die hard: prevalence and reduction of wrong beliefs in topics from educational psychology among preservice teachers. Eur. J. Psychol. Educ. 36, 477–494. doi: 10.1007/s10212-020-00474-5
Perugini, A., Laing, M., Berretta, N., Aicardi, G., and Bashir, Z. I. (2012). Synaptic plasticity from amygdala to perirhinal cortex: a possible mechanism for emotional enhancement of visual recognition memory? Eur. J. Neurosci. 36, 2421–2427. doi: 10.1111/j.1460-9568.2012.08146.x
Plucker, J. A., Callahan, C. M., and Tomchin, E. M. (1996). Wherefore art thou, multiple intelligences? Alternative assessment for identifying talent in ethnically diverse and low income students. Gifted Child Q. 40, 81–91. doi: 10.1177/001698629604000205
Popham, S. F., Huth, A. G., Bilenko, N. Y., Deniz, F., Gao, J. S., Nunez-Elizalde, A. O., et al. (2021). Visual and linguistic semantic representations are aligned at the border of human visual cortex. Nat. Neurosci. 24, 1628–1636. doi: 10.1038/s41593-021-00921-6
Rogers, J., and Cheung, A. (2020). Pre-service teacher education may perpetuate myths about teaching and learning. J. Educ. Teach. 46, 417–420. doi: 10.1080/02607476.2020.1766835
Rolls, E. T., Deco, G., Huang, C.-C., and Feng, J. (2022). The human language effective connectome. NeuroImage 258:119352. doi: 10.1016/j.neuroimage.2022.119352
Rousseau, L. (2021a). Interventions to dispel neuromyths in educational settings – a review. Front. Psychol. 12:719692. doi: 10.3389/fpsyg.2021.719692
Rousseau, L. (2021b). “Neuromyths” and multiple intelligences (MI) theory: a comment on Gardner (2020). Front. Psychol. 12:720706. doi: 10.3389/fpsyg.2021.720706
Roy, D. S., Park, Y.-G., Kim, M. E., Zhang, Y., Ogawa, S. K., DiNapoli, N., et al. (2022). Brain-wide mapping reveals that engrams for a single memory are distributed across multiple brain regions. Nat. Commun. 13:1799. doi: 10.1038/s41467-022-29384-4
Ruhaak, A. E., and Cook, B. G. (2018). The prevalence of educational neuromyths among pre-service special education teachers. Mind Brain Educ. 12, 155–161. doi: 10.1111/mbe.12181
Schacter, J. (2000). Does individual tutoring produce optimal learning? Am. Educ. Res. J. 37, 801–829. doi: 10.3102/00028312037003801
Shaler, J. A. (2006). Howard Gardner under fire: The rebel psychologist faces his critics . Chicago: Open Court.
Sheahan, L., While, A., and Bloomfield, J. (2015). An exploratory trial exploring the use of a multiple intelligences teaching approach (MITA) for teaching clinical skills to first year undergraduate nursing students. Nurse Educ. Today 35, 1148–1154. doi: 10.1016/j.nedt.2015.05.002
Shearer, C. B. (2018). Multiple intelligences in teaching and education: lessons learned from neuroscience. J. Intelligence 6:38. doi: 10.3390/jintelligence6030038
Shearer, C. B. (2020a). Multiple intelligences in gifted and talented education: lessons learned from neuroscience after 35 years. Roeper Rev. 42, 49–63. doi: 10.1080/02783193.2019.1690079
Shearer, C. B. (2020b). A resting state functional connectivity analysis of human intelligence: broad theoretical and practical implications for multiple intelligences theory. Psychol. Neurosci. 13, 127–148. doi: 10.1037/pne0000200
Shearer, C. B., and Karanian, J. M. (2017). The neuroscience of intelligence: empirical support for the theory of multiple intelligences? Trends Neurosci. Educ. 6, 211–223. doi: 10.1016/j.tine.2017.02.002
Sperber, D. (1994). “The modularity of thought and the epidemiology of representations” in Mapping the mind: Domain specificity in cognition and culture . eds. L. A. Hirschfeld and S. A. Gelman (New York: Cambridge University Press), 39–67.
Sporns, O., and Betzel, R. F. (2016). Modular brain networks. Annu. Rev. Psychol. 67, 613–640. doi: 10.1146/annurev-psych-122414-033634
Teele, S. (1992). Teele inventory of multiple intelligences . Redlands, CA: Sue Teele and Associates
Visser, B. A., Ashton, M. C., and Vernon, P. A. (2006a). Beyond g: putting multiple intelligences theory to the test. Intelligence 34, 487–502. doi: 10.1016/j.intell.2006.02.004
Visser, B. A., Ashton, M. C., and Vernon, P. A. (2006b). G and the measurement of multiple intelligences: a response to Gardner. Intelligence 34, 507–510. doi: 10.1016/j.intell.2006.04.006
Wang, R., Liu, M., Cheng, X., Wu, Y., Hildebrandt, A., and Zhou, C. (2021). Segregation, integration, and balance of large-scale resting brain networks configure different cognitive abilities. PNAS 118:e202288118. doi: 10.1073/pnas.202288118
Wang, Z., Xi, Q., Zhang, H., Song, Y., and Cao, S. (2022). Different neural activities for actions and language within the shared brain regions: from action and verb generation. Behav. Sci. 12:243. doi: 10.3390/bs12070243
Waterhouse, L. (2006). Multiple intelligences, the Mozart effect and emotional intelligence: a critical review. Educ. Psychol. 41, 207–225. doi: 10.1207/s15326985ep4104_1
Winarti, A., Yuanita, L., and Nur, M. (2019). The effectiveness of multiple intelligences based teaching strategy in enhancing the multiple intelligences and science process skills of junior high school students. J. Technol. Sci. Educ. 9, 122–135. doi: 10.3926/jotse.404
Yavich, R., and Rotnitsky, I. (2020). Multiple intelligences and success in school studies. Int. J. High. Educ. 9:107. doi: 10.5430/ijhe.v9n6p107
Zerilli, J. (2017). Against the ‘system’ module. Philos. Psychol. 30, 235–250. doi: 10.1080/09515089.2017.1280145
Zhan, L., Guo, D., Chen, G., and Yang, J. (2018). Effects of repetition learning on associative recognition over time: roles of the hippocampus and prefrontal cortex. Front. Hum. Neurosci. 12:277. doi: 10.3389/fnhum.2018.00277
Keywords: multiple intelligences, brain, neuromyth, teaching methods, cognition, neuroscience
Citation: Waterhouse L (2023) Why multiple intelligences theory is a neuromyth. Front. Psychol . 14:1217288. doi: 10.3389/fpsyg.2023.1217288
Received: 05 May 2023; Accepted: 14 August 2023; Published: 28 August 2023.
Reviewed by:
Copyright © 2023 Waterhouse. This is an open-access article distributed under the terms of the Creative Commons Attribution License (CC BY) . The use, distribution or reproduction in other forums is permitted, provided the original author(s) and the copyright owner(s) are credited and that the original publication in this journal is cited, in accordance with accepted academic practice. No use, distribution or reproduction is permitted which does not comply with these terms.
*Correspondence: Lynn Waterhouse, [email protected]
Disclaimer: All claims expressed in this article are solely those of the authors and do not necessarily represent those of their affiliated organizations, or those of the publisher, the editors and the reviewers. Any product that may be evaluated in this article or claim that may be made by its manufacturer is not guaranteed or endorsed by the publisher.

An official website of the United States government
The .gov means it’s official. Federal government websites often end in .gov or .mil. Before sharing sensitive information, make sure you’re on a federal government site.
The site is secure. The https:// ensures that you are connecting to the official website and that any information you provide is encrypted and transmitted securely.
- Publications
- Account settings
The PMC website is updating on October 15, 2024. Learn More or Try it out now .
- Advanced Search
- Journal List

Types of Intelligence and Academic Performance: A Systematic Review and Meta-Analysis
Raquel lozano-blasco.
1 Department of Psychology and Sociology, Faculty of Humanities and Science Education, University of Zaragoza, 50001 Zaragoza, Spain
Alberto Quílez-Robres
2 Department of Educational Sciences, Faculty of Human Sciences and Education, University of Zaragoza, 22003 Huesca, Spain
Pablo Usán
3 Department of Psychology and Sociology, Faculty of Education, University of Zaragoza, 50009 Zaragoza, Spain
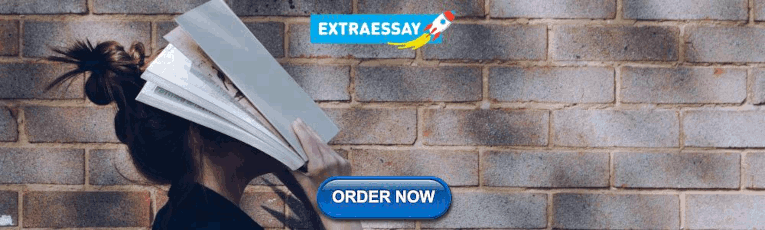
Carlos Salavera
4 Department of Educational Sciences, Faculty of Humanities and Science Education, University of Zaragoza, 50001 Zaragoza, Spain
Raquel Casanovas-López
5 Instituto de Estudios Interdisciplinarios de la Niñez y la Adolescencia (INEINA), Universidad Nacional, Heredia 40101, Costa Rica
The concept of intelligence has been extensively studied, undergoing an evolution from a unitary concept to a more elaborate and complex multidimensional one. In addition, several research studies have focused their efforts for decades on the study of intelligence as a predictor of academic performance of students at different educational stages, being a stable and highly relevant predictor along with other variables such as executive functions, social context, culture or parental guardianship. Thus, the present study, based on a systematic review and meta-analysis, includes 27 studies with a total sample of 42,061 individuals. The main objective was to analyse the relationship between intelligence and academic performance using different predictive models that include moderating variables such as country of origin, type of intelligence, gender and age. The findings of this research highlight the significant, positive and moderate relationship between intelligence and academic performance (r = 0.367; p < 0.001), highlighting the predictive capacity on school performance when the type of intelligence (general and implicit; 35%) or the country of origin (45%) is taken as a moderating variable, with the explanatory models on age or sex not being significant. Therefore, it can be concluded that intelligence, in addition to being a good predictor of academic performance, is influenced depending on the type of intelligence or theoretical model taken as a reference, and also depending on the country or culture of origin.
1. Introduction
The educational community has traditionally and extensively studied academic performance. This concept is closely related to the teaching–learning process focused on a specific goal: achievement in school ( Von Stumm and Ackerman 2013 ). Therefore, issues such as school success or failure, discouragement and dropout have produced a great deal of research ( Balkis 2018 ). Proof of this would be the study by Nieto Martín ( 2008 ), who reviewed 654 studies conducted between 1970 and 1990. The author stresses that the variables under study and related to academic success have changed over time; for example, intelligence was traditionally studied from a single-factor point of view, but later this approach was expanded and, at present, other variables such as executive functions, motivation or self-esteem and self-efficacy are at the forefront of the study. In addition, the new century has seen the emergence of new methodological variables such as group collaboration, collaborative work, project-based learning and the length of the school day. The literature has traditionally categorised these variables as contextual or personal: socio-environmental variables (family, friends, colleagues), institutional variables (school, school organisation, teachers) and instructional variables (content, methods, tasks). In addition, another group included are cognitive (intelligence, learning styles) and motivational (self-image, goals, values) variables ( Quílez-Robres et al. 2021b ). Therefore, academic performance can be understood as a construct that includes quantitative and qualitative values (quantitative if we talk about numerical measurement, test results and qualitative if we talk about the development of skills, values and competences), related to knowledge, attitudes and values developed by the student in the teaching–learning process” ( Navarro 2003, pp. 15–16 ).
However, this study focuses on the relationship and explanation of academic performance through intelligence, understood as different types of intelligence. There is extensive literature on the relationship, prediction and explanation of intelligence with academic performance. However, studies concerning intelligence have expanded conventional psychometric notions by introducing modalities such as crystallised intelligence, fluid intelligence, emotional intelligence, multiple intelligences, etc., in an attempt to provide greater predictive validity in reference to academic achievement ( Sternberg 2019 ).
The conceptual definitions of intelligence are diverse. For ( Quílez-Robres et al. 2021a ) it consists of the ability to understand and adapt to solve everyday problems. On the other hand, for Plomin and Deary ( 2015 ), intelligence has a biological substrate that varies according to individuals and cultures, being the potential that facilitates learning, planning, reasoning, adaptation and decision-making.
Catell ( 1963 ) differentiated between two kinds of intelligence: fluid intelligence and crystallised intelligence and suggested that intelligence is composed of different capacities that form general intelligence and that are complementary. Crystallised intelligence is the result of education and culture and therefore depends directly on the individual’s prior knowledge and ability to learn ( Nisbett et al. 2012 ) and fluid intelligence with a genetic component is the ability to solve problems through non-verbal abstract reasoning and adaptation to different contexts ( Nisbett et al. 2012 ). In addition, it is linked to individual learning and memory ( Amin et al. 2015 ). The latter is considered as one of the main predictors of individual academic achievement according to several studies in different settings ( Deary et al. 2007 ; Geary 2011 ; Laidra et al. 2007 ; Monir et al. 2016 ; Verbitskaya et al. 2020 ). Rabbitt and Lowe ( 2000 ) suggest that fluid intelligence is altered in the ageing process, while crystallised intelligence remains stable.
The study of intelligence expanded, and Gardner ( 1985 ) proposed an alternative and a critique of the general intelligence approach by elaborating the theory of multiple intelligences. He proposed the existence of several independent intelligences that interact and mutually enhance each other, such as linguistic, logical–mathematical, spatial, kinaesthetic bodily and others. Thus, most students possess more than one. However, Singh et al. ( 2017 ) report that only logical–mathematical, spatial and musical intelligence are related to IQ. These results are consistent with the research of Castejon et al. ( 2010 ) and Visser et al. ( 2006 ), who reported a strong relationship between cognitive component intelligences and general intelligence.
Sternberg ( 1985 ) elaborated the Triarchic Theory of intelligence, establishing three categories within it: competency, experiential and contextual. Thus, the acquisition and storage of information, the ability to encode, combine and compare that information and finally the adaptation of information to context were involved. He expanded on this theory and called it successful intelligence, which combined ability, exploitation, adaptation, creativity, etc. It is about being able to solve problems, and depending on the way it is done, analytical intelligence (both familiar and abstract problems), creative intelligence (formulating ideas, problems of a novel nature) and practical intelligence (applying ideas and analysis effectively) will appear ( Sternberg et al. 2010 ).
The theories of Gardner ( 1985 ) and Sternberg ( 1985 ) were fundamental for the emergence of the theory of emotional intelligence since these ideas underlay the new concept that would germinate in the theories of Salovey and Mayer ( 1990 ), but it would be Goleman ( 1996 ) who popularised it by stating that emotional intelligence consists of a series of skills such as discovering, recognising and managing emotions and feelings ( Goleman 1999 ). The relevant role of emotional aspects in academic results is evident in previous studies such as the meta-analysis conducted by Molero-Puertas et al. ( 2020 ), which concludes with a significant effect size between emotional intelligence and academic performance. Other research suggests that this variable is a good predictor of academic achievement at different educational stages and even indicates that it is second only to general intelligence ( MacCann et al. 2020 ; Perera and DiGiacomo 2013 ; Sanchez-Ruiz et al. 2013 ).
Finally, implicit intelligence, regarded as the self-perception of intelligence grounded in everyday experience, is a key variable for understanding academic performance ( Enea-Drapeau et al. 2017 ). Since this includes a component of expectation as cognitive self-representation, some authors point out that the relationship is especially direct in the early years and concerning specific performance areas rather than global performance ( Dinger et al. 2013 ; Geary 2011 ; Priess-Groben and Hyde 2017 ; Wigfield et al. 2016 ); other authors argue that implicit intelligence is a good predictor for academic performance in maths ( Kriegbaum et al. 2015 ; Steinmayr and Spinath 2009 ); for Steinmayr et al. ( 2019a ) and Lotz et al. ( 2018 ), this predictive value extends over all areas, as confidence in one’s own abilities can be a more important variable than cognitive abilities in the analysis of academic performance. In this sense, implicit theories are presented as definitions, or theories that scientists have about some phenomena ( Sternberg 1985 ). Precisely in these beliefs lies the importance of understanding people’s implicit theories. This is important because these beliefs guide people’s attitudes and behaviours and, as discussed in various theories of the development of talent and intelligence, intelligence is not composed of a single factor but is multidimensional, with contextual, creative and motivational aspects related to people’s behaviours intervening in its conception. The theory of social cognition indicates that beliefs determine attitudes and willingness to engage in certain behaviours ( Pintrich 2002 ). Undoubtedly, these aspects mean that implicit intelligence must be taken into account in relation to academic performance and learning.
With regard to academic performance, its prediction has been a relevant topic for a long time and different variables have been analysed to help explain the academic results of schoolchildren. Different research has related it to individual characteristics of basic cognitive processes such as processing speed, working memory, fluid intelligence, etc. ( El Jaziz et al. 2020 ; Kiuru et al. 2012 ; Kuncel et al. 2004 ; Richardson et al. 2012 ; Sternberg et al. 2001 ). However, academic performance as a product of learning serves as an indicator of the level of learning ( Alquichire R and Arrieta R 2018 ). For Ariza et al. ( 2018 ), it is nothing more than a measure of what students have learned as a result of an educational process. He defines it as the ability to respond to a series of educational stimuli, which in turn is interpreted on the basis of the established objectives.
In view of previous research, it is not new that measures of general intelligence predict academic performance ( Deary et al. 2007 ; Quílez-Robres et al. 2021b ; Sternberg 2019 ). Systematic study has resulted in the predictive value of intelligence in the educational world and has pointed to significant correlations with different variables, but there is also some variation depending on the educational stage analysed ( Sternberg et al. 2001 ). While agreeing that intelligence is one of the most important variables in academic performance as it has a direct impact on learning ( González et al. 2008 ), it should be noted that it does not behave uniformly, as the correlation between intelligence and academic performance decreases when the student reaches the university stage ( Ren et al. 2015 ).
If the aim is to increase the predictive value of the different measures of intelligence, one possibility is to broaden the concept of intelligence itself. A review of the scientific literature shows that there are no studies that integrate the different types of intelligence theorised in reference to academic achievement. This meta-analysis aims to analyse the relationship between different types of intelligence and academic performance from a meta-analytical perspective by reviewing the scientific literature with a broad conception of the concept of intelligence, taking into account the studies that indicate that there is no single way of understanding and defining this construct.
A research registry protocol ( Figure 1 ) was established following the Cochrane systematic review manual in Higgins and Green ( 2011 ) and PRISMA ( 2015 ). Inclusion criteria were determined using the specifications set out by Ausina and Sánchez-Meca ( 2015 ) and Moreau and Gamble ( 2020 ): (a) Research methodology: quantitative, correlational, longitudinal, cross-sectional and clinical. (b) Time frame: 2000–2020. (c) Methodological rigour: studies indexed in prestigious rankings (Scimago Journal and Country Rank). (d) Measuring instruments: psychometric tests rated in academic publications and in accordance with the culture of the sample. (e) Language: English.

Flowchart of search methodology.
The exclusion criteria were established according to the manuals of Ausina and Sánchez-Meca ( 2015 ) and Moreau and Gamble ( 2020 ): (a) Adult population with previous disorders or pathologies, including, however, research in which there were control groups without pathologies. (b) The appearance of imprecise, poorly defined data, unclear methodology or with indications of non-compliance with ethical principles, as well as statistical or psychometric errors in the measurement of the tests, following the indications of Hunter and Schmidt ( 2004 ) and Friese and Frankenbach ( 2020 ).
The search strategy was carried out using the criteria of Botella and Gambara ( 2002 ), Ausina and Sánchez-Meca ( 2015 ) and PRISMA ( 2015 ). Three databases were used: Psycoinfo, Pubmed and Science Direct, and research was performed in February 2021. The Boleean action was “academic achievement” and “intelligence” in the range 2000–2020.
Eligibility criteria for sample selection were defined according to the Cochrane systematic review manual in Higgins and Green ( 2011 ) and PRISMA ( 2015 ). It should be noted that manual coding was carried out by reviewing each article returned by Boolean actions according to the inclusion and exclusion criteria. Firstly, the abstract was screened so that only those that dealt with the subject of the study were selected. On the other hand, the criteria of methodological rigour and measurement instruments led to the exclusion of a significant percentage of the research. This was due to the absence of standardised instruments or the incorrect measurement of the study parameters according to the pre-established psychometric test.
The transformation of all means to Fisher Z ( Martín-Andrés and Luna del Castillo 2004 ), the execution of the relevant analyses (model comparison and meta-regression), the study of heterogeneity, the performance of the Eggers test for publication bias and the obtaining of figures were carried out using the CMA statistical software.
3.1. Demographic Description
In recent years (2000–2020), the relationship between types of intelligence and academic performance at different educational stages has been studied in depth. In total, the meta-analysis ( Table 1 ) consists of 27 studies with k = 47 samples from Europe, Asia, Africa, America and Oceania. According to Bonett ’s ( 2006 ) criteria, the sample k = 47 exceeds the minimum required to avoid distortion of the upper confidence limit. On the other hand, heterogeneity is evident in the sample sizes, with the smallest sample size being 81 participants and the largest 4036 participants.
Sociodemographic data.
Authors | Number of Samples | Size of Samples | Age | Female | Male | Type of Intelligence | Type of Achievement | Country | Geographical Region |
---|---|---|---|---|---|---|---|---|---|
( ) | 1 | 123 | 18.67 | 99 | 24 | general | general | Indonesia | Asia |
( ) | 2 | 198 | 4.84 | 106 | 92 | fluid | mathematics | USA | North America |
( ) | 3 | 81 | 16.02 | 41 | 40 | general | general | Australia | Oceania |
( ) | 1 | 506 | 11 | 247 | 259 | emotional | general | USA | North America |
( ) | 1 | 312 | 19.88 | 187 | 125 | general | general | China | Asia |
( ) | 1 | 96 | 21.46 | 71 | 11 | emotional | general | USA | North America |
( ) | 1 | 163 | 21.8 | 112 | 51 | emotional | general | Malaysia | Asia |
( ) | 1 | 524 | 17.43 | 278 | 246 | implicit | general | Germany | Central Europe |
( ) | 1 | 2062 | 12 | 1031 | 1031 | emotional | general | Norway | Northern Europe |
( ) | 1 | 282 | 10.4 | 154 | 126 | general | general | USA | North America |
( ) | 1 | 151 | 22.8 | 88 | 63 | emotional | general | Barbados | America |
( ) | 1 | 167 | 16.34 | 95 | 72 | kinesthetic | general | Morocco | North Africa |
( ) | 1 | 521 | 20.56 | 374 | 147 | emotional | general | Russia | Eastern Europe |
( ) | 1 | 476 | 13 | 290 | 186 | general | general | Finlandia | Northern Europe |
( ) | 4 | 300 | 19.48 | 221 | 79 | general | general | Russia | Eastern Europe |
( ) | 3 | 4036 | 15.41 | 2066 | 1970 | emotional | general | China | Asia |
( ) | 2 | 407 | 9.5 | 203 | 204 | general | lenguage | Egypt | North Africa |
( ) | 1 | 320 | 14.14 | No data | No data | general | general | UK | Central Europe |
( ) | 1 | 165 | 17.35 | 77 | 88 | implicit | general | USA | North America |
( ) | 1 | 3826 | 71.19 | 2066 | 1760 | general | general | USA | North America |
( ) | 1 | 115 | 12.70 | 67 | 48 | emotional | general | USA | North America |
( ) | 1 | 323 | 23 | 113 | 210 | emotional | general | UK | Central Europe |
( ) | 4 | 325 | 10.67 | 146 | 179 | general | musical | USA | North America |
( ) | 1 | 354 | 17.48 | 200 | 145 | verbal | general | Germany | Central Europe |
( ) | 1 | 476 | 16.43 | 244 | 232 | general | general | Germany | Central Europe |
( ) | 9 | 1560 | 6.8 | 718 | 842 | fluid | language | Russia | Eastern Europe |
( ) | 1 | 1120 | 4 | No data | No data | general | general | USA | North America |
The total sample is made up of 42,061 participants, 47.16% of whom are male and 48.99% female. In this sense, it is necessary to clarify that the two studies do not provide data on the sex of their participants. The average age of the participants is 16.45 years, although some studies did not report a specific average age, but rather a range of years or school years, making it necessary to take the arithmetic mean to be able to manage the data quantitatively.
In terms of culture, social anthropology points to the need to attend to cultural diversity ( Molano 2007 ). In this study, 30.13% are Asian (China, Indonesia and Malaysia), 4.73% are Central European (Germany, UK), 37.37% are Eastern European (Russia), 6.01% are Northern European (Norway and Finland), 2.32% are North African (Morocco and Egypt), 18.83% are American (USA and Barbados) and 0.57% are from Oceania (Australia).
3.2. Statistical Analysis
The aim of this meta-analysis is to study the relationship between type of intelligence and student achievement, but encompassing different educational stages and different contexts. To this end, 108 effect sizes were coded, taking as a reference the data based on Pearson’s r and their subsequent treatment using the CMA statistical programme.
Figure 2 (forest plot) shows the effect size with a 99% confidence interval (0.302–0.428, p = 0.001) for the different studies, the effect size being r = 0.367, p = 0.001. In other words, a moderate level of correlation is obtained according to Cohen between the intelligence presented by the students and academic performance. The ethical criteria set out by Moreau and Gamble ( 2020 ) are followed when exposing all the conversions, opting for a policy of “open materials”.

Forest Plot.
On the other hand, it is crucial to study the heterogeneity of the sample according to Cochrane in Higgins and Green ( 2011 ). The Q statistic of Dersimonian and Laird ( 1986 ) (Q = 2478.71, df = 46, p < 0.0001) describes a high variability, i.e., the homogeneity hypothesis is rejected. The statistic I2 = 98.144% explains the percentage of variability resulting from heterogeneity and not from chance. In other words, the sample is highly heterogeneous in its statistical nature ( Higgins et al. 2013 ). Consistently, the Random model or random effects model is applied ( Bonett 2006 ; Martín-Andrés and Luna del Castillo 2004 ). Although the inclusion and exclusion criteria contemplate the reliability and methodological and psychometric quality of the research, the Egg’s test with 99% reliability was carried out to study the effect of bias ( Botella and Gambara 2002 ; Ausina and Sánchez-Meca 2015 ). The results of the test show the inexistence of publication bias with a 99% confidence interval ( p -value 1 tailed = 0.07; p -value 2 tailed = 0.15) ( Egger et al. 1997 ). The standard error value (SE = 2.04) reaffirms the absence of bias, as it is very close to the regression line ( Martín-Andrés and Luna del Castillo 2004 ).
The diversity shown in the Q and I2 statistics could be a sign of extreme data; however, the tight confidence interval (0.302–0.428, p = 0.001) limits this heterogeneity. These results are consistent with the Funnel Plot graph ( Figure 3 ) where the variability and heterogeneity of the sample are reaffirmed. This situation reiterates the diversity of studies, as concluded by the Egger test, without any bias effect. However, it should be noted that the apparent variability could be affected by the transformation to Fisher Z -values since x-values >0.5 tend to be more distorted on the T-Student curve than, in comparison, on the normal curve, although this transformation is accepted by the scientific community for meta-analysis methodology ( Martín-Andrés and Luna del Castillo 2004 ).

Funnel plot and standard error.
3.3. Moderating Variables and Meta-Regression Analysis
The state-of-the-art research shows the existence of moderating factors, which is why it is considered necessary to establish the study of seven moderating variables: type of intelligence, type of performance, age, country, male sex, female sex and geographical distribution. The objective pursued through the use of both techniques is to statistically determine the reason for such heterogeneity ( Ausina and Sánchez-Meca 2015 ; Jak and Cheung 2020 ). In this way, a comparison of models is established (see Table 2 ) by generating seven models: (1) type of intelligence; (2) type of performance; (3) age; (4) country; (5) male gender; (6) female gender; (7) geographical distribution.
Comparison of models: Random effects (MM), Z distribution, Fisher’s Z.
Models | TauSq | R² | Q | df | -Value |
---|---|---|---|---|---|
Model 1 Intelligence | 0.03 | 0.35 | 30.49 | 9 | 0.0004 |
Model 2 Performance | 0.06 | 0.00 | 6.33 | 5 | 0.2758 |
Model 3 Age | 0.05 | 0.05 | 0,00 | 1 | 0.9754 |
Model 4 Country | 0.03 | 0.45 | 54.65 | 12 | 0.0000 |
Model 5 Female | 0.06 | 0.00 | 2.81 | 1 | 0.09 |
Model 6 Male | 0.05 | 0.03 | 2.92 | 1 | 0.08 |
Model 7 Geography | 0.03 | 0.37 | 15.73 | 1 | 0.15 |
The first model, which specifies the type of intelligence, explains 35% of academic performance, with an efficiency level of over 99%, although, as model 2 shows, the type of performance has no effect. In other words, it is intelligence that determines student academic performance and success, but doing well in these subjects does not seem to affect intelligence overall. On the other hand, the models of age, gender and geographical distribution do not explain the relationship between the two factors to any extent. However, there are important differences between countries, which may be explained by diversity in the education system. Association with a given nation accounts for 45% of the variability in the sample ( Table 2 ).
It is therefore necessary to study in greater depth the type of intelligence that seems to determine academic performance. For this reason, a meta-regression ( Table 3 ) is carried out in which it is evident that general intelligence (Z = 2.00, p = 0.04) and implicit intelligence (Z = 3.69, p = 0.00) are the ones that stand out, showing a clear difference.
Meta-regression of model 1: Intelligence.
Meta-Regression M.1 | |||||||||
---|---|---|---|---|---|---|---|---|---|
Covariate | Coefficient | Standard Error | 95% Lower | 95% Upper | 2-Sided -Value | Q | df | ||
Intercept | 0.10 | 0.20 | −0.29 | 0.49 | 0.50 | 0.61 | 30.49 | 9 | 0.0004 |
Crystallised | 0.34 | 0.29 | −0.22 | 0.91 | 1.19 | 0.23 | |||
Emotional | 0.13 | 0.21 | −0.27 | 0.55 | 0.64 | 0.52 | |||
Spatial | 0.01 | 0.28 | −0.55 | 0.57 | 0.04 | 0.97 | |||
Fluid | 0.24 | 0.20 | −0.17 | 0.65 | 1.15 | 0.25 | |||
General | 0.41 | 0.20 | 0.08 | 0.82 | 2.00 | 0.04 | |||
Implicit | 1.05 | 0.28 | 0.49 | 1.61 | 3.69 | 0.00 | |||
Mathematical | 0.14 | 0.28 | −0.42 | 0.71 | 0.50 | 0.61 | |||
Synaesthetic | 0.35 | 0.29 | −0.22 | 0.92 | 1.20 | 0.23 | |||
Verbal | 0.19 | 0.23 | −0.26 | 0.65 | 0.82 | 0.41 |
As far as the different countries are concerned, significant differences are found in the comparison models. Therefore, it is necessary to perform a meta-regression (see Table 4 ) that points out the differences between education systems. In this case, the countries that differ from the sample are China, Indonesia and the UK (United Kingdom).
Meta-regression of model 2: countries.
Meta-Regression M.2 | |||||||||
---|---|---|---|---|---|---|---|---|---|
Covariate | Coefficient | Standard Error | 95% Lower | 95% Upper | -Value | 2-Sided -Value | Q | df | |
Intercept | 0.48 | 0.10 | 0.26 | 0.69 | 4.42 | 0.00 | 54.65 | 12 | 0.0000 |
Australia | 0.12 | 0.16 | −0.20 | 0.44 | 0.74 | 0.45 | |||
Barbados | 0.17 | 0.22 | −0.27 | 0.62 | 0.78 | 0.43 | |||
China | −0.31 | 0.14 | −0.60 | −0.03 | −2.22 | 0.02 | |||
Egypt | −0.23 | 0.17 | −0.57 | 0.10 | −1.36 | 0.17 | |||
Finland | −0.13 | 0.21 | −0.55 | 0.29 | −0.59 | 0.55 | |||
Indonesia | 0.69 | 0.23 | 0.23 | 1.14 | 2.97 | 0.00 | |||
Malaysia | −0.41 | 0.22 | −0.86 | 0.03 | −1.82 | 0.06 | |||
Morocco | −0.32 | 0.22 | −0.47 | 0.41 | −0.14 | 0.88 | |||
Norway | −0.07 | 0.21 | −0.49 | 0.34 | −0.34 | 0.73 | |||
Russia | −0.19 | 0.12 | −0.43 | 0.03 | −1.65 | 0.09 | |||
UK | −0.52 | 0.17 | −0.86 | −0.17 | −2.98 | 0.00 | |||
USA | 0.09 | 0.12 | −0.14 | 0.33 | 0.76 | 0.44 |
4. Discussion
Given that the review of the scientific literature indicates that there is no single way of understanding, defining and analysing the construct of intelligence, this meta-analysis analyses the relationship between intelligence and academic performance in terms of the different types of intelligence studied in previous research, as well as the existence of models of moderating variables that clarify their predictive nature. Therefore, effect size, type of intelligence (general, crystallised, fluid, implicit, emotional, etc.), age, gender, country of residence or geographical area are of interest for this study. Of all these variables, effect size, general intelligence, implicit intelligence (R 2 = 0.35; p < 0.001) and country of residence (R 2 = 0.45; p < 0.001) are those that appear to be relevant and significant.
From the results obtained, a number of factors stand out, such as the relationship between academic performance and intelligence with a moderate effect size (0.367; significance < 0.001). Previous research addressing the interrelations between intelligence and academic performance indicates that it is the most stable and powerful predictor of school performance (r = 0.5) ( Geary 2011 ; Laidra et al. 2007 ; Luo et al. 2006 ; Rodic et al. 2015 ). These results are corroborated in the meta-analysis of Cortés Pascual et al. ( 2019 ) who equate it with that obtained for executive functions. They point out that intelligence is decisive for new learning and, on the contrary, executive functions are primordial for repetitive and competence-focused learning and also show their relationship in different educational disciplines.
Another noteworthy element of the research is that when analysing moderating variables and comparing models, it is found that intelligence determines that the relationship with academic performance is unidirectional. That is, intelligence is a good predictor of academic achievement, but not the other way around, so the predictive model of intelligence type explains 35% of the variance. Consistent with this result, Buckle et al. ( 2005 ) assigned it a predictive power of 26%. This is in line with previous research findings that intelligence is the best predictor of academic success ( Blankson et al. 2019 ; Erath et al. 2015 ; Li et al. 2017 ; Quílez-Robres et al. 2021a ; Ren et al. 2015 ; Rhodes et al. 2017 ; Tikhomirova et al. 2020 ). However, most studies have related it to the cognitive dimension ( Castejon et al. 2010 ; Visser et al. 2006 ), marginalising the behavioural and emotional aspects ( Gioia et al. 2017 ). Therefore, it is necessary to consider other facets of intelligence, as they are nothing more than different capacities that complement each other ( Catell 1963 ).
From the meta-regression of the intelligence model, general and implicit intelligence emerge with significance ( p < 0.05 and p < 0.01). Implicit intelligence is decisive in school outcomes, as the beliefs that are elaborated about one’s own intelligence and the nature of intelligence guide student behaviours towards achieving success or failure at school ( Chen and Tutwiler 2017 ; Lotz et al. 2018 ; Steinmayr et al. 2019b ). Thus, it is considered relevant for its efficacy in considering that cognitive ability is not a fixed trait but has an adaptive quality that gives it incremental strength. This malleability performs a protective function against school failure, as there is confidence in one’s own abilities. However, it seems that this incremental capacity decreases with age ( Chen and Tutwiler 2017 ). In the same line of research, Kornilova et al. ( 2009 ) found that implicit intelligence predicts general intelligence, as by adopting learning goals and increasing their competence, students overcome setbacks and seek new challenges. It should also be noted that both general and implicit intelligence show an indirect effect with academic success through other variables such as motivation or executive functions ( Aditomo 2015 ). Furthermore, it should be noted that general intelligence has traditionally been broken down into fluid and crystallised intelligence. “Crystallised” intelligence has been considered one of the most significant predictors of individual achievement in different contexts, age ranges and educational conditions ( Deary et al. 2007 ; Nisbett et al. 2012 ; Verbitskaya et al. 2020 ), and “fluid” intelligence has been found to be a better predictor of processing speed tests ( Luo et al. 2006 ) and mathematics performance ( Blankson et al. 2019 ; Sarver et al. 2012 ).
Neither emotional intelligence nor the different types of multiple intelligences show remarkable values in this research. In this regard, the scientific literature is not conclusive. Some studies find that emotional intelligence occupies a pre-eminent position behind general or global intelligence ( MacCann et al. 2020 ; Perera and DiGiacomo 2013 ) and explain that this type of intelligence is related to academic performance due to its importance in promoting adaptive behaviours ( Chew et al. 2013 ; Fayombo 2012 ; Usán Supervía and Quílez Robres 2021 ). The perception of positive interpersonal and intrapersonal emotional intelligence substantially explains academic success, as it comprises learner ability to control, regulate and manage the demands of the academic context ( Cheshire et al. 2015 ; Chew et al. 2013 ; Kornilova et al. 2018 ; Okwuduba et al. 2021 ; Romero et al. 2014 ). However, the research that studies this claim presents mixed results since some authors such as Engin ( 2017 ) or Zhoc et al. ( 2018 ) did not observe associations between academic performance and emotional intelligence. On the other hand, and within the multiple intelligences, musical intelligence has been related to academic performance, especially through cross-sectional data, from which it is difficult to infer a generalisation of cause and effect with respect to school achievement ( Müllensiefen et al. 2015 ; Schellenberg 2011 ). This is despite the fact that Castejon et al. ( 2010 ) and Visser et al. ( 2006 ) point to the existence of a relationship between some of the multiple intelligences and general intelligence due to their cognitive component.
Concerning the type of “perfomance”, comparison analysis model showed its non-significance. However, it is necessary to point out that the performance types are not homogeneous in the meta-sample. There is a large amount of general performance, but there are hardly any cases of music or mathematics. Despite its non-significance, investigation of this aspect in further research is considered necessary, and nevertheless, there are studies that advocate the importance of this variable.
As for gender and age, they are not moderating variables, perhaps influenced by the type of assessment of these variables and the different theoretical concepts of greater or lesser importance assigned to the relationship between them. On the other hand, there are difficulties in predicting the role of gender and age in implicit intelligence ( Diseth et al. 2014 ), but Robins and Trzesniewski ( 2005 ) point out that there is a strong relationship in favour of girls and at an older age. These results can be related to emotional intelligence and higher perceived self-efficacy ( King et al. 2012 ).
As noted above, the country of residence model is the moderating variable that explains 45% of the variance, increasing the predictive power of the intelligence type model. These results are consistent with previous research pointing to the importance of adaptation to different contexts ( Deary et al. 2007 ; Verbitskaya et al. 2020 ) or those indicating that the relationship between intelligence and academic performance was the result of education and the culture in which one was immersed ( Nisbett et al. 2012 ; Plomin and Deary 2015 ; Rodic et al. 2015 ). In his theories, Sternberg, for example, pointed out the importance of adaptation to the context of different skills and abilities, as well as of the differences originating in the beliefs of one’s own abilities in their contribution to academic achievement as a function of the cultural environment that generate individual profiles with different strengths and weaknesses ( Sternberg 2019 ; Sternberg et al. 2001 ). Ultimately, intelligence is related to social competence ( Sternberg 1985 ).
When analysing the meta-regression across countries, three countries are significant: Indonesia, the United Kingdom (UK) and China. Indonesia is considered a very deterministic culture (if you are not very smart, you do not pass) ( Aditomo 2015 ). On the other hand, the United Kingdom (UK) as a model of the Anglo-Saxon education system associates intelligence with linguistic ability and problem-solving skills ( Sternberg 1985 ). Finally, in China, authoritarian filial piety beliefs are associated with an entity view of intelligence, which impairs the students’ academic performance ( Chen and Wong 2014 ). Cultural views of motivational processes can shed light on the ways in which motivational beliefs develop as a product of cultural or socialisation processes, which, in turn, contribute to or determine the students’ academic success ( Chen and Wong 2014 ; Li et al. 2017 ). These differences by country of origin are likely to point to the meanings attributed to intelligence by different cultural groups. There are indications that individuals from Western countries attach a much broader meaning to the concept of intelligence (skills, context, etc.) and, therefore, when studying subjects from non-Western countries, consideration should be given to using specific domains that provide greater certainty to the results, always bearing in mind that the mindset about intelligence and academic ability is very different ( Aditomo 2015 ). As Carroll ( 1992 ) points out, intelligence is a concept within the mind of a society and personal references are those of each culture where individuals are immersed. Some cultures such as the Asian ones continue to use teaching–learning methods based on cognitive aspects such as memory and one’s own intelligence, while the European and Anglo-Saxon models are based on the development of competence through social interaction ( Quílez-Robres et al. 2021a ).
Other reason may be due to different factors such as, for example, the statistical weight of the samples, or others related to cultural elements such as different understandings of academic performance and different assessments of different types of intelligence.
Furthermore, following Serpell ( 2000 ), culture can be approached from three perspectives: culture as a language, culture as a womb, and culture as a forum. According to the language perspective, culture would constitute a distinct system of meanings in the mind within which the concept of intelligence would be embedded. According to the womb perspective, human cultures create environments that nurture personal growth and stimulate the development of human intelligence. Finally, the forum view, which is based on the interaction of members of a community organising aspects of education and constructing new meanings about intelligence, proposes research on cognitive development as a function of culture.
On the other hand, Sternberg and Grigorenko ( 2004 ) indicate that intelligence cannot be understood completely outside of cultural control or influence. There are behaviours that are considered intelligent in some cultures, and those same behaviours are considered unintelligent in other cultures. Furthermore, each culture has implicit (folk) theories of intelligence, and therefore the aspects that fall under this concept vary from culture to culture. In this sense, the three influential cultures in this study belong to two different cultural approaches: individualistic (UK) versus collectivistic (China and Indonesia). Moreover, these countries have different ways of understanding academic performance and attach different degrees of importance to intelligence in academic, social and occupational performance ( Quílez-Robres et al. 2021b ).
5. Conclusions
This research was conducted to identify the ways in which different aspects of student intelligence contribute to differences in academic performance. Of the seven models studied, the country of residence model was found to be the most important predictor of academic performance, explaining 45% of the variance, followed by the type of intelligence model, which explains 35% of the variance. The latter model highlights the importance of general intelligence and implicit intelligence for student grades in academic subjects. The results therefore extend knowledge about the role of intelligence for academic achievement. Implicit intelligence scores better in relation to academic achievement than global intelligence, highlighting the importance of one’s beliefs in one’s own abilities. Students with similar intelligence scores, with identical values and the same prior attainment will see improved academic outcomes by believing in their own competencies and abilities ( Steinmayr et al. 2019a ). If one concludes that academic performance is determined by a multitude of variables including psychological factors that influence student response to overcome setbacks, the evidence points to intelligence as a predictor of success, but also, as this research shows, to a positive mindset in relation to one’s own intelligence and academic abilities. This positive mindset will also be established by the context in which their academic life takes place, i.e., society, beliefs, values, education system, etc. ( Aditomo 2015 ; Hong et al. 1999 ). Therefore, the results of this study point the way to implement interventions aimed at improving the students’ own beliefs about their subject-specific mastery skills.
Finally, we conclude with the need to expand the study in order to limit the term intelligence. What would its general structure be, and how do the different types of intelligence add significance to the general and traditional concept? What conceptual divergences exist between the different theories? Do all these concepts have the same impact on new or repeated learning, on general and specific?
Acknowledgments
The authors would like to thank the University of Zaragoza for their support in this research.
Funding Statement
This research did not receive any specific grant from funding agencies in the public, commercial, or not-for-profit sectors.
Author Contributions
Conceptualization, A.Q.-R. and R.L.-B.; methodology, A.Q.-R.; software, R.L.-B.; validation, R.C.-L., P.U. and C.S.; formal analysis, R.L.-B.; investigation, R.L.-B.; resources, A.Q.-R.; data curation, A.Q.-R.; writing—original draft preparation, R.L.-B.; writing—review and editing, A.Q.-R.; visualization, R.C.-L.; supervision, C.S.; project administration, P.U. All authors have read and agreed to the published version of the manuscript.
Conflicts of Interest
The authors declare no conflict of interest.
Publisher’s Note: MDPI stays neutral with regard to jurisdictional claims in published maps and institutional affiliations.
- Aditomo Anindito. Students’ response to academic setback: “Growth mindset” as a buffer against demotivation. International Journal of Educational Psychology. 2015; 4 :198–222. doi: 10.17583/ijep.2015.1482. [ CrossRef ] [ Google Scholar ]
- Alquichire R Shirley Lorena, Arrieta R Juan Carlos. Relación entre habilidades de pensamiento crítico y rendimiento académico. Voces y Silencios. Revista Latinoamericana de Educación. 2018; 9 :28–52. doi: 10.18175/vys9.1.2018.03. [ CrossRef ] [ Google Scholar ]
- Amin Hafeez Ullah, Malik Aamir Saeed, Kamel Nidal, Chooi Weng-Tink, Hussain Muhammad. P300 correlates with learning & memory abilities and fluid intelligence. Journal of Neuroengineering and Rehabilitation. 2015; 12 :87. doi: 10.1186/s12984-015-0077-6. [ PMC free article ] [ PubMed ] [ CrossRef ] [ Google Scholar ]
- Ariza Carla Patricia, Toncel Luis Ángel Rueda, Blanchar Jainer Sardoth. El rendimiento académico: Una problemática compleja. Revista Boletín Redipe. 2018; 7 :137–41. [ Google Scholar ]
- Ausina Juan Botella, Sánchez-Meca Julio. Meta-Análisis en Ciencias Sociales y de la Salud. Síntesis; Madrid: 2015. [ Google Scholar ]
- Balkis Murat. Desmotivación académica e intención de abandono escolar: El papel mediador del logro académico y el absentismo. Asia Pacific Journal of Education. 2018; 38 :257–70. doi: 10.1080/02188791.2018.1460258. [ CrossRef ] [ Google Scholar ]
- Blankson A. Nayena, Gudmundson Jessica A., Kondeh Memuna. Cognitive predictors of kindergarten achievement in African American children. Journal of Educational Psychology. 2019; 111 :1273–83. doi: 10.1037/edu0000346. [ PMC free article ] [ PubMed ] [ CrossRef ] [ Google Scholar ]
- Bonett Douglas G. Robust Confidence Interval for a Ratio of Standard Deviations. Applied Psychological Measurements. 2006; 30 :432–39. doi: 10.1177/0146621605279551. [ CrossRef ] [ Google Scholar ]
- Botella Juan, Gambara Hilda. Qué es el Meta-Análisis. Biblioteca Nueva; Madrid: 2002. [ Google Scholar ]
- Buckle Sarah K., Lancaster Sandra, Powell Martine B., Higgins Daryl J. The relationship between child sexual abuse and academic achievement in a sample of adolescent psychiatric inpatients. Child Abuse & Neglect. 2005; 29 :1031–47. doi: 10.1016/j.chiabu.2004.12.013. [ PubMed ] [ CrossRef ] [ Google Scholar ]
- Carroll John. ¿Qué es la inteligencia? In: Sternberg Robert J., Detterman Douglas K., editors. ¿Qué es la Inteligencia? Enfoque Actual de su Naturaleza y Definición. Pirámide; Madrid: 1992. pp. 69–72. [ Google Scholar ]
- Castejon Juan L., Perez Antonio M., Gilar Raquel. Confirmatory factor analysis of Project Spectrum activities. A second-order g factor or multiple intelligences? Intelligence. 2010; 38 :481–96. doi: 10.1016/j.intell.2010.07.002. [ CrossRef ] [ Google Scholar ]
- Cattell Raymond B. Theory of fluid and crystallized intelligence: A critical experiment. Journal of Educational Psychology. 1963; 54 :1. doi: 10.1037/h0046743. [ PubMed ] [ CrossRef ] [ Google Scholar ]
- Chen Jason A., Tutwiler M. Shane. Implicit theories of ability and self-efficacy. Zeitschrift für Psychologie. 2017; 225 :127–36. doi: 10.1027/2151-2604/a000289. [ CrossRef ] [ Google Scholar ]
- Chen Wei-Wen, Wong Yi-Lee. What my parents make me believe in learning: The role of filial piety in Hong Kong students’ motivation and academic achievement. International Journal of Psychology. 2014; 49 :249–56. doi: 10.1002/ijop.12014. [ PubMed ] [ CrossRef ] [ Google Scholar ]
- Cheshire Michelle H., Strickland Haley P., Carter Melondie R. Comparing traditional measures of academic success with emotional intelligence scores in nursing students. Asia-Pacific Journal of Oncology Nursing. 2015; 2 :99–106. doi: 10.4103/2347-5625.154090. [ PMC free article ] [ PubMed ] [ CrossRef ] [ Google Scholar ]
- Chew Boon How, Zain Azhar Md, Hassan Faezah. Emotional intelligence and academic performance in first and final year medical students: A cross-sectional study. BMC Medical Education. 2013; 13 :44. doi: 10.1186/1472-6920-13-44. [ PMC free article ] [ PubMed ] [ CrossRef ] [ Google Scholar ]
- Cortés Pascual Alejandra, Muñoz Nieves Moyano, Robres Alberto Quílez. Relación entre funciones ejecutivas y rendimiento académico en educación primaria: Revisión y metanálisis. Frente. Psicol. 2019; 10 :1582. doi: 10.3389/fpsyg.2019.01582. [ CrossRef ] [ Google Scholar ]
- Deary Ian J., Strand Steve, Smith Pauline, Fernandes Cres. Intelligence and educational achievement. Intelligence. 2007; 35 :13–21. doi: 10.1016/j.intell.2006.02.001. [ CrossRef ] [ Google Scholar ]
- Dersimonian Rebecca, Laird Nan. Meta-analysis in clinical trials. Controlled Clinical Trials. 1986; 7 :177–88. doi: 10.1016/0197-2456(86)90046-2. [ PubMed ] [ CrossRef ] [ Google Scholar ]
- Dinger Felix C., Dickhäuser Oliver, Spinath Birgit, Steinmayr Ricarda. Antecedents and consequences of students’ achievement goals: A mediation analysis. Learning and Individual Differences. 2013; 28 :90–101. doi: 10.1016/j.lindif.2013.09.005. [ CrossRef ] [ Google Scholar ]
- Diseth Åge, Meland Eivind, Breidablik Hans Johan. Self-beliefs among students: Grade level and gender differences in self-esteem, self-efficacy and implicit theories of intelligence. Learning and Individual Differences. 2014; 35 :1–8. doi: 10.1016/j.lindif.2014.06.003. [ CrossRef ] [ Google Scholar ]
- Egger Matthias, Smith George Davey, Phillips Andrew N. Meta-analysis: Principles and procedures. Britihs Medical Journal BMJ. 1997; 315 :1533–37. doi: 10.1136/bmj.315.7121.1533. [ PMC free article ] [ PubMed ] [ CrossRef ] [ Google Scholar ]
- El Jaziz Anas, Lotfi Said, Ahami Ahmad O. T. Interrelationship of physical exercise, perceptual discrimination and academic achievement variables in high school students. Ann Ig. 2020; 32 :528–40. doi: 10.7416/ai.2020.2373. [ PubMed ] [ CrossRef ] [ Google Scholar ]
- Enea-Drapeau Claire, Carlier Michèle, Huguet Pascal. Implicit theories concerning the intelligence of individuals with Down syndrome. PLoS ONE. 2017; 12 :e0188513. doi: 10.1371/journal.pone.0188513. [ PMC free article ] [ PubMed ] [ CrossRef ] [ Google Scholar ]
- Engin Melih. Analysis of Students’ Online Learning Readiness Based on Their Emotional Intelligence Level. Universal Journal of Educational Research. 2017; 5 :32–40. doi: 10.13189/ujer.2017.051306. [ CrossRef ] [ Google Scholar ]
- Erath Stephen A., Tu Kelly M., Buckhalt Joseph A., El-Sheikh Mona. Associations between children’s intelligence and academic achievement: The role of sleep. Journal of Sleep Research. 2015; 24 :510–13. doi: 10.1111/jsr.12281. [ PMC free article ] [ PubMed ] [ CrossRef ] [ Google Scholar ]
- Fayombo Grace A. Relating emotional intelligence to academic achievement among university students in Barbados. [(accessed on 5 January 2020)]; The International Journal of Emotional Education. 2012 4 :43–54. Available online: https://www.um.edu.mt/library/oar/handle/123456789/6141 [ Google Scholar ]
- Friese Malte, Frankenbach Julius. p-Hacking and publication bias interact to distort meta-analytic effect size estimates. Psychological Methods. 2020; 25 :456–71. doi: 10.1037/met0000246. [ PubMed ] [ CrossRef ] [ Google Scholar ]
- Gardner Howard. The Mind’s New Science: A History of the Cognitive Revolutión. Basic Books; New York: 1985. [ Google Scholar ]
- Geary David C. Cognitive predictors of achievement growth in mathematics: A 5-year longitudinal study. Developmental Psychology. 2011; 47 :1539–52. doi: 10.1037/a0025510. [ PMC free article ] [ PubMed ] [ CrossRef ] [ Google Scholar ]
- Gioia Gerard A., Isquith Peter, Guy Steven C., Kenworthy Lauren. In: Santamaria (Adapters), BRIEF-2. Evaluación Conductual de la Función Ejecutiva. Maldonado María Jesús, Fournier María de la Concepción, Martínez-Arias Rosario, González-Marqués Javier, Espejo-Saavedra Juan Manuel, Santamaría Pablo., editors. TEA Ediciones; Madrid: 2017. [ Google Scholar ]
- Goleman Daniel. Inteligencia Emocional. Kairós; Barcelona: 1996. [ Google Scholar ]
- Goleman Daniel. La Práctica de la Inteligencia Emocional. Kairós; Barcelona: 1999. [ Google Scholar ]
- González Gustavo, Solano Alejandro Castro, González Federico. Perfiles aptitudinales, estilos de pensamiento y rendimiento académico. [(accessed on 4 January 2020)]; Anuario de Investigaciones. 2008 15 :33–41. Available online: https://www.redalyc.org/pdf/3691/369139944035.pdf [ Google Scholar ]
- Higgins Julian P. T., Green Sally. Cochrane Handbook for Systematic Reviews of Interventions Version 5.1.0. The Cochrane Collaboration; London: 2011. [(accessed on 4 January 2020)]. Available online: www.cochrane-handbook.org [ Google Scholar ]
- Higgins Julian P. T., Ramsay Craig, Reeves Barnaby C., Deeks Jonathan J., Shea Beverley, Valentine Jeffrey C., Tugwell Peter, Wells George. Issues relating to study design and risk of bias when including non-randomized studies in systematic reviews on the effects of interventions. Research Synthesis Methods. 2013; 4 :12–25. doi: 10.1002/jrsm.1056. [ PubMed ] [ CrossRef ] [ Google Scholar ]
- Hong Ying-yi, Chiu Chi-yue, Dweck Carol S., Lin Derrick M-S., Wan Wendy. Implicit theories, attributions, and coping: A meaning system approach. Journal of Personality and Social Psychology. 1999; 77 :588–99. doi: 10.1037/0022-3514.77.3.588. [ CrossRef ] [ Google Scholar ]
- Hunter John E., Schmidt Frank L. Methods of Meta-Analysis: Correcting Error and Bias in Research Findings. Sage; New York: 2004. [ Google Scholar ]
- Jak Suzanne, Cheung Mike W-L. Meta-analytic structural equation modeling with moderating effects on SEM parameters. Psychological Methods. 2020; 25 :430–55. doi: 10.1037/met0000245. [ PubMed ] [ CrossRef ] [ Google Scholar ]
- King Ronnel B., McInerney Dennis M., Watkins David A. How you think about your intelligence determines how you feel in school: The role of theories of intelligence on academic emotions. Learning and Individual Differences. 2012; 22 :814–19. doi: 10.1016/j.lindif.2012.04.005. [ CrossRef ] [ Google Scholar ]
- Kiuru Noona, Salmela-Aro Katariina, Nurmi Jari-Erik, Zettergren Peter, Andersson Håkan, Bergman Lars. Best friends in adolescence show similar educational careers in early adulthood. Journal of Applied Developmental Psychology. 2012; 33 :102–11. doi: 10.1016/j.appdev.2011.12.001. [ CrossRef ] [ Google Scholar ]
- Kornilova Tatiana V., Chumakova Maria A., Krasavtseva Yulia V. Emotional intelligence, patterns for coping with decisional conflict, and academic achievement in cross-cultural perspective (evidence from selective Russian and Azerbaijani student populations) [(accessed on 4 January 2020)]; Psychology in Russia: State of the Art. 2018 11 :114–33. doi: 10.11621/pir.2018.0209. Available online: http://psychologyinrussia.com/volumes/index.php?article=7230 [ CrossRef ] [ Google Scholar ]
- Kornilova Tatiana V., Kornilov Sergey A., Chumakova Maria A. Subjective evaluations of intelligence and academic self-concept predict academic achievement: Evidence from a selective student population. Learning and Individual Differences. 2009; 19 :596–608. doi: 10.1016/j.lindif.2009.08.001. [ CrossRef ] [ Google Scholar ]
- Kriegbaum Katharina, Jansen Malte, Spinath Birgit. Motivation: A predictor of PISA’s mathematical competence beyond intelligence and prior test achievement. Learning and Individual Differences. 2015; 43 :140–48. doi: 10.1016/j.lindif.2015.08.026. [ CrossRef ] [ Google Scholar ]
- Kuncel Nathan R., Hezlett Sarah A., Ones Deniz S. Academic performance, career potential, creativity, and job performance: Can one construct predict them all? Journal of Personality and Social Psychology. 2004; 86 :148–61. doi: 10.1037/0022-3514.86.1.148. [ PubMed ] [ CrossRef ] [ Google Scholar ]
- Laidra Kaia, Pullmann Helle, Allik Jüri. Personality and intelligence as predictors of academic achievement: A cross-sectional study from elementary to secondary school. Personality and Individual Differences. 2007; 42 :441–51. doi: 10.1016/j.paid.2006.08.001. [ CrossRef ] [ Google Scholar ]
- Li Ping, Zhou Nan, Zhang Yuchi, Xiong Qing, Nie Ruihong, Fang Xiaoyi. Incremental theory of intelligence moderated the relationship between prior achievement and school engagement in Chinese high school students. Frontiers in Psychology. 2017; 8 :1703. doi: 10.3389/fpsyg.2017.01703. [ PMC free article ] [ PubMed ] [ CrossRef ] [ Google Scholar ]
- Lotz Christin, Schneider Rebecca, Sparfeldt Jörn R. Differential relevance of intelligence and motivation for grades and competence tests in mathematics. Learning and Individual Differences. 2018; 65 :30–40. doi: 10.1016/j.lindif.2018.03.005. [ CrossRef ] [ Google Scholar ]
- Luo Dasen, Thompson Lee A., Detterman Douglas K. The criterion validity of tasks of basic cognitive processes. Intelligence. 2006; 34 :79–120. doi: 10.1016/j.intell.2004.12.003. [ CrossRef ] [ Google Scholar ]
- MacCann Carolyn, Jiang Yixin, Brown Luke E. R., Double Kit S, Bucich Micaela, Minbashian Amirali. Emotional intelligence predicts academic performance: A meta-analysis. Psychological Bulletin. 2020; 146 :150. doi: 10.1037/bul0000219. [ PubMed ] [ CrossRef ] [ Google Scholar ]
- Martín Andrés A., Luna del Castillo J. D. D. Bioestadística para las Ciencias de la Salud. Capitel Ediciones, SL; Madrid: 2004. [ Google Scholar ]
- Molano Olga Lucía. Identidad cultural un concepto que evoluciona. [(accessed on 5 January 2020)]; Revista Opera. 2007 7 :69–84. Available online: https://dialnet.unirioja.es/servlet/articulo?codigo=4020258 [ Google Scholar ]
- Molero-Puertas Pilar, Zurita-Ortega Félix, Chacón-Cuberos Ramón, Castro-Sánchez Manuel, Ramírez-Granizo Irwin, Valero-González Gabriel. La inteligencia emocional en el ámbito educativo: Un metaanálisis. Anales de Psicología/Annals of Psychology. 2020; 36 :84–91. doi: 10.6018/analesps.345901. [ CrossRef ] [ Google Scholar ]
- Monir Zeinab M., El-Din Ebtissam M. Salah, El-Alameey Inas R., Yamamah Gamal A., Megahed Hala S., Salem Samar M., Ibrahim Tarek S. Academic Achievement and Psychosocial Profile of Egyptian Primary School Children in South Sinai. Open Access Macedonian Journal of Medical Sciences. 2016; 4 :624–29. doi: 10.3889/oamjms.2016.111. [ PMC free article ] [ PubMed ] [ CrossRef ] [ Google Scholar ]
- Moreau David, Gamble Beau. Conducting a meta-analysis in the age of open science: Tools, tips, and practical recommendations. Advance online publication. Psychological Methods. 2020; 27 :426–32. doi: 10.1037/met0000351. [ PubMed ] [ CrossRef ] [ Google Scholar ]
- Müllensiefen Daniel, Harrison Peter, Caprini Francesco, Fancourt Amy. Investigating the importance of self-theories of intelligence and musicality for students’ academic and musical achievement. Frontiers in Psychology. 2015; 6 :1702. doi: 10.3389/fpsyg.2015.01702. [ PMC free article ] [ PubMed ] [ CrossRef ] [ Google Scholar ]
- Navarro Rubén Edel. El rendimiento académico: Concepto, investigación y desarrollo. [(accessed on 5 January 2020)]; REICE. Revista Iberoamericana sobre Calidad, Eficacia y Cambio en Educación. 2003 1 :1–16. Available online: https://revistas.uam.es/index.php/reice/article/view/5354/5793 [ Google Scholar ]
- Nieto Martín Santiago. Hacia una teoría sobre el rendimiento académico en enseñanza primaria a partir de la investigación empírica: Datos preliminares. Teoría de la Educación. Revista Interuniversitaria. 2008; 20 :249–74. doi: 10.14201/ted.992. [ CrossRef ] [ Google Scholar ]
- Nisbett Richard E., Aronson Joshua, Blair Clancy, Dickens William, Flynn James, Halpern Diane F., Turkheimer Eric. Intelligence: New findings and theoretical developments. American Psychologist. 2012; 67 :130–59. doi: 10.1037/a0026699. [ PubMed ] [ CrossRef ] [ Google Scholar ]
- Okwuduba Emmanuel Nkemakolam, Nwosu Kingsley Chinaza, Okigbo Ebele Chinelo, Samuel Naomi Nkiru, Achugbu Chinwe. Impact of intrapersonal and interpersonal emotional intelligence and self-directed learning on academic performance among pre-university science students. Heliyon. 2021; 7 :e06611. doi: 10.1016/j.heliyon.2021.e06611. [ PMC free article ] [ PubMed ] [ CrossRef ] [ Google Scholar ]
- Perera Harsha N., DiGiacomo Michelle. The relationship of trait emotional intelligence with academic performance: A meta-analytic review. Learning and Individual Differences. 2013; 28 :20–33. doi: 10.1016/j.lindif.2013.08.002. [ CrossRef ] [ Google Scholar ]
- Pintrich Paul R. The role of metacognitive knowledge in learning, teaching, and assessing. Theory into Practice. 2002; 41 :219–25. doi: 10.1207/s15430421tip4104_3. [ CrossRef ] [ Google Scholar ]
- Plomin Robert, Deary Ian J. Genetics and intelligence differences: Five special findings. Molecular Psychiatry. 2015; 20 :98–108. doi: 10.1038/mp.2014.105. [ PMC free article ] [ PubMed ] [ CrossRef ] [ Google Scholar ]
- Priess-Groben Heather A., Hyde Janet Shibley. Implicit theories, expectancies, and values predict mathematics motivation and behavior across high school and college. Journal of Youth and Adolescence. 2017; 46 :1318–32. doi: 10.1007/s10964-016-0579-y. [ PubMed ] [ CrossRef ] [ Google Scholar ]
- PRISMA Grupo PRISMA-P. Preferred Reporting Items for Systematic Review and Meta-Analysis Protocols (PRISMA-P) 2015: Elaboration and explanation. British Medical Journal BMJ. 2015; 349 :g7647. doi: 10.1136/bmj.g7647. [ PubMed ] [ CrossRef ] [ Google Scholar ]
- Quílez-Robres Alberto, González-Andrade Alejandro, Ortega Zaira, Santiago-Ramajo Sandra. Intelligence quotient, short-term memory and study habits as academic achievement predictors of elementary school: A follow-up study. Studies in Educational Evaluation. 2021a; 70 :101020. doi: 10.1016/j.stueduc.2021.101020. [ CrossRef ] [ Google Scholar ]
- Quílez-Robres Alberto, Moyano Nieves, Cortés-Pascual Alejandra. Motivational, Emotional, and Social Factors Explain Academic Achievement in Children Aged 6–12 Years: A Meta-Analysis. Education Sciences. 2021b; 11 :513. doi: 10.3390/educsci11090513. [ CrossRef ] [ Google Scholar ]
- Rabbitt Patrick, Lowe Christine. Patterns of cognitive ageing. Psychological Research. 2000; 63 :308–16. doi: 10.1007/s004269900009. [ PubMed ] [ CrossRef ] [ Google Scholar ]
- Ren Xuezhu, Schweizer Karl, Wang Tengfei, Xu Fen. The prediction of students’ academic performance with fluid intelligence in giving special consideration to the contribution of learning. Advances in Cognitive Psychology. 2015; 11 :97–105. doi: 10.5709/acp-0175-z. [ PMC free article ] [ PubMed ] [ CrossRef ] [ Google Scholar ]
- Rhodes Emma, Devlin Kathryn N., Steinberg Laurence, Giovannetti Tania. Grit in adolescence is protective of late-life cognition: Non-cognitive factors and cognitive reserve. Aging, Neuropsychology, and Cognition. 2017; 24 :321–32. doi: 10.1080/13825585.2016.1210079. [ PMC free article ] [ PubMed ] [ CrossRef ] [ Google Scholar ]
- Richardson Michelle, Abraham Charles, Bond Rod. Psychological Correlates of University Students’ Academic Performance: A Systematic Review and Meta-Analysis. Psychological Bulletin. 2012; 138 :353–87. doi: 10.1037/a0026838. [ PubMed ] [ CrossRef ] [ Google Scholar ]
- Robins Richard W., Trzesniewski Kali H. Self-esteem development across the lifespan. Current Directions in Psychological Science. 2005; 14 :158–62. doi: 10.1111/j.0963-7214.2005.00353.x. [ CrossRef ] [ Google Scholar ]
- Rodic Maja, Zhou Xinlin, Tikhomirova Tatiana, Wei Wei, Malykh Sergei, Ismatulina Victoria, Sabirova Elena, Davidova Yulia, Tosto Maria Grazia, Lemelin Jean-Pascal, et al. Cross-cultural investigation into cognitive underpinnings of individual differences in early arithmetic. Developmental Science. 2015; 18 :165–74. doi: 10.1111/desc.12204. [ PubMed ] [ CrossRef ] [ Google Scholar ]
- Romero Carissa, Master Allison, Paunesku Dave, Dweck Carol S., Gross James J. Academic and emotional functioning in middle school: The role of implicit theories. Emotion. 2014; 14 :227–34. doi: 10.1037/a0035490. [ PubMed ] [ CrossRef ] [ Google Scholar ]
- Salovey Peter, Mayer John D. Emotional Intelligence. Imagination, Cognition and Personality. 1990; 9 :185–211. doi: 10.2190/DUGG-P24E-52WK-6CDG. [ CrossRef ] [ Google Scholar ]
- Sanchez-Ruiz Maria-Jose, Mavroveli Stella, Poullis Joseph. Trait emotional intelligence and its links to university performance: An examination. Personality and Individual Differences. 2013; 54 :658–62. doi: 10.1016/j.paid.2012.11.013. [ CrossRef ] [ Google Scholar ]
- Sarver Dustin E., Rapport Mark D., Kofler Michael J., Scanlan Sean W., Raiker Joseph S., Altro Thomas A., Bolden Jennifer. Attention problems, phonological short-term memory, and visuospatial short-term memory: Differential effects on near-and long-term scholastic achievement. Learning and Individual Differences. 2012; 22 :8–19. doi: 10.1016/j.lindif.2011.09.010. [ CrossRef ] [ Google Scholar ]
- Schellenberg E. Glenn. Examining the association between music lessons and intelligence. British Journal Psychology. 2011; 102 :283–302. doi: 10.1111/j.2044-8295.2010.02000.x. [ PubMed ] [ CrossRef ] [ Google Scholar ]
- Serpell Roberto. Inteligencia y cultura. In: Sternberg Roberto J., editor. Manual de Inteligencia. Cambridge University Press; Cambridge: 2000. pp. 549–77. [ CrossRef ] [ Google Scholar ]
- Singh Yashpal, Makharia Archita, Sharma Abhilasha, Agrawal Kruti, Varma Gowtham, Yadav Tarun. A study on different forms of intelligence in Indian school-going children. Industrial Psychiatry Journal. 2017; 26 :71–76. doi: 10.4103/ipj.ipj_61_16. [ PMC free article ] [ PubMed ] [ CrossRef ] [ Google Scholar ]
- Steinmayr Ricarda, Spinath Birgit. The importance of motivation as a predictor of school achievement. Learning and Individual Differences. 2009; 19 :80–90. doi: 10.1016/j.lindif.2008.05.004. [ CrossRef ] [ Google Scholar ]
- Steinmayr Ricarda, Weidinger Anne F., Schwinger Malte, Spinath Birgit. The importance of students’ motivation for their academic achievement–replicating and extending previous findings. Frontiers in Psychology. 2019a; 10 :1730. doi: 10.3389/fpsyg.2019.01730. [ PMC free article ] [ PubMed ] [ CrossRef ] [ Google Scholar ]
- Steinmayr Ricarda, Wirthwein Linda, Modler Laura, Barry Margaret M. Development of subjective well-being in adolescence. International Journal of Environmental Research and Public Health. 2019b; 16 :3690. doi: 10.3390/ijerph16193690. [ PMC free article ] [ PubMed ] [ CrossRef ] [ Google Scholar ]
- Sternberg Robert J. Beyond IQ: A Triarchic Theory of Human Intelligence. Cambridge University Press; Cambridge: 1985. [ Google Scholar ]
- Sternberg Robert J. A theory of adaptive intelligence and its relation to general intelligence. Journal of Intelligence. 2019; 7 :23. doi: 10.3390/jintelligence7040023. [ PMC free article ] [ PubMed ] [ CrossRef ] [ Google Scholar ]
- Sternberg Robert J., Grigorenko Elena L. Intelligence and culture: How culture shapes what intelligence means, and the implications for a science of well–being. Philosophical Transactions of the Royal Society of London. Series B: Biological Sciences. 2004; 359 :1427–34. doi: 10.1098/rstb.2004.1514. [ PMC free article ] [ PubMed ] [ CrossRef ] [ Google Scholar ]
- Sternberg Robert J., Grigorenko Elena L., Bundy Donald A. The predictive value of IQ. [(accessed on 5 January 2020)]; Merrill-Palmer Quarterly. 2001 47 :1–41. doi: 10.1353/mpq.2001.0005. Available online: https://www.jstor.org/stable/23093686 [ CrossRef ] [ Google Scholar ]
- Sternberg Robert, Grigorenko Elena, Ferrando Mercedes, Hernández Daniel, Ferrándiz Carmen, Bermejo Rosario. Enseñanza de la inteligencia exitosa para alumnos superdotados y talentos. [(accessed on 5 January 2020)]; Revista Electrónica Interuniversitaria de Formación del Profesorado. 2010 13 :111–18. Available online: https://www.redalyc.org/pdf/2170/217014922011.pdf [ Google Scholar ]
- Tikhomirova Tatiana, Malykh Artem, Malykh Sergey. Predicting academic achievement with cognitive abilities: Cross-sectional study across school education. Behavioral Sciences. 2020; 10 :158. doi: 10.3390/bs10100158. [ PMC free article ] [ PubMed ] [ CrossRef ] [ Google Scholar ]
- Usán Supervía Pablo, Quílez Robres Alberto. Emotional Regulation and Academic Performance in the Academic Context: The Mediating Role of Self-Efficacy in Secondary Education Students. International Journal of Environmental Research and Public Health. 2021; 18 :5715. doi: 10.3390/ijerph18115715. [ PMC free article ] [ PubMed ] [ CrossRef ] [ Google Scholar ]
- Verbitskaya Ludmila A., Zinchenko Yury P., Malykh Sergey B., Gaidamashko Igor V., Kalmyk Olga A., Tikhomirova Tatiana N. Cognitive Predictors of Success in Learning Russian Among Native Speakers of High School Age in Different Educational Systems. [(accessed on 4 January 2020)]; Psychology in Russia. State of the Art. 2020 13 :2–15. doi: 10.11621/pir.2020.0201. Available online: http://psychologyinrussia.com/volumes/pdf/2020_2/Psychology_2_2020_2-15_Verbitskaya.pdf [ CrossRef ] [ Google Scholar ]
- Visser Beth A., Ashton Michael C., Vernon Philip A. Beyond g: Putting multiple intelligences theory to the test. Intelligence. 2006; 34 :487–502. doi: 10.1016/j.intell.2006.02.004. [ CrossRef ] [ Google Scholar ]
- Von Stumm Sopfhie, Ackerman Phillip L. Inversión e intelecto: Una revisión y meta-análisis. Psychological Bulletin. 2013; 139 :841–69. doi: 10.1037/a0030746. [ PubMed ] [ CrossRef ] [ Google Scholar ]
- Wigfield Allan, Tonks Stephen, Klauda Susan Lutz. Expectancy-value theory. In: Wentzel Kathryn R., Miele David B., editors. Handbook of Motivation in School. 2nd ed. Routledge; London: 2016. pp. 55–74. [ Google Scholar ]
- Willoughby Michael T., Magnus Brooke, Vernon-Feagans Lynne, Blair Clancy B., Family Life Project Investigators Developmental delays in executive function from 3 to 5 years of age predict kindergarten academic readiness. Journal of Learning Disabilities. 2017; 50 :359–72. doi: 10.1177/0022219415619754. [ PMC free article ] [ PubMed ] [ CrossRef ] [ Google Scholar ]
- Zhoc Karen C. H., Chung Tony S. H., King Ronnel B. Emotional intelligence (EI) and self-directed learning: Examining their relation and contribution to better student learning outcomes in higher education. British Educational Research Journal. 2018; 44 :982–1004. doi: 10.1002/berj.3472. [ CrossRef ] [ Google Scholar ]
Academia.edu no longer supports Internet Explorer.
To browse Academia.edu and the wider internet faster and more securely, please take a few seconds to upgrade your browser .
Enter the email address you signed up with and we'll email you a reset link.
- We're Hiring!
- Help Center
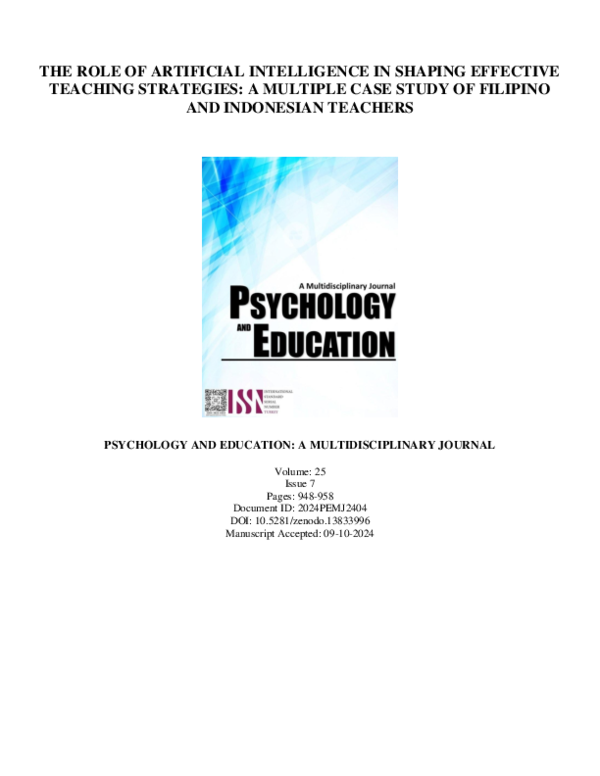
Download Free PDF
The Role of Artificial Intelligence in Shaping Effective Teaching Strategies: A Multiple Case Study of Filipino and Indonesian Teachers

2024, Psychology and Education: A Multidisciplinary Journal
Artificial Intelligence is considered a technological platform that impacts the teaching and learning process, particularly on its effect on assessment and grading and influence on graduates' future careers. However, the use of AI in the field of education, specifically on effective teaching strategies and classroom management poses issues experienced by teachers in the field. The increased use of AI in the classroom faces significant challenges because of insufficient resources for complete implementation. Nevertheless, researchers ground the argument in the gap and observed the need to find out more in the context of the experiences, coping mechanisms, and insights of Filipino and Indonesian teachers regarding the role of AI in shaping effective teaching strategies to improve student learning outcomes within the Philippine and Indonesian education system. Through this study initiative, an international university collaboration is established to conduct a multiple case study using qualitative interviews to gather essential information. Face-to-face interviews and online interviews were conducted with the participants with the guide of a given research questionnaire. Thus, semi-structured interviews are conducted with three Filipino and two Indonesian teachers as participants from private schools to gather their experiences in implementing and using AI-driven teaching strategies in their classrooms, forming the basis of this multiple case study. The researchers then proceeded to analyze the gathered information using descriptive-case analysis, within-case analysis, and cross-case analysis. In the concluding part of this study, from technical obstacles to systemic issues of digital infrastructure and technological inequality, these complexities underscore the need for concerted efforts to address barriers to AI-driven education. Despite these challenges, teachers demonstrate resilience and resourcefulness in navigating technological hurdles and promoting student engagement. By investing in professional development and fostering collaboration, educators unlock the full potential of AI in education and create inclusive learning environments that empower all students to succeed in the digital age.
Related papers
Psychology and Education: A Multidisciplinary Journal, 2024
This qualitative-descriptive study aimed to identify the different verbal interactions and most frequent and least observed interactions in the College of Teacher Education classrooms of a private non-sectarian institution in Davao City. Flanders' Interaction Analysis Category System (FIACS) was used to analyze the gathered data. In order to utilize the FIACS, the study employed observation, where three teachers were observed for two consecutive hours, and unstructured in-depth interviews, where five teachers were interviewed. The results revealed that the different verbal interactions used by the teachers involved accepting feelings, praising or encouraging, accepting or using pupils' ideas, asking questions, lecturing, giving directions, criticizing or justifying authority, pupil-talk response, pupil-talk initiation, and silence. Among the ten categories, the most frequently observed verbal interaction was pupil talkresponse, and the least observed was silence or confusion. The findings revealed how the teachers' verbal interactions promote active engagement in the teaching-learning process and create a thriving learning environment. From this, the teachers should provide a variety of learning opportunities to accommodate different learning needs and create interactive activities in the classroom to improve the quality of the teaching-learning process.
As automation becomes increasingly integrated into educational settings, understanding its role in shaping students' writing experiences is crucial. The goal of this qualitative phenomenological research was to explore the lived experiences, motivations, role of AI in students' writing, influence of AI on the learning process, and insights in using AI writing tools of the senior high school students of Monkayo National High School. There were 10 participants in this study who were selected through snowball sampling. An in-depth interview was used to gather the information needed. The findings revealed the potentials and pitfalls of AI in developing the students' writing skills. Most of the participants have seen AI's advantages, like completing the writing tasks easily, which meets the deadline, making good-quality outputs adhering to the technicalities of grammar, improving academic achievements, and gaining knowledge about writing. However, there are also some drawbacks, like students becoming indolent and AI dependent; AI diminishes one's critical thinking skills; AI gives inaccurate information; there is a limitation on usage; it is inaccessible when offline; and it is bad for one's health. Nevertheless, the role of AI in students' writing processes is significant. Its effectiveness and efficiency cannot be denied, but it should be used in moderation. Hence, incorporating AI in learning should be guided by strategies that balance its benefits with the need to maintain creative and critical thinking in students' writing. Educators should use AI as a tool to enhance, not replace, human creativity. This approach ensures that while students benefit from AI's strengths, they continue to develop their originality and critical thinking skills in writing.
This descriptive qualitative research aimed to describe the teacher and students' interaction using the Foreign Language Interaction (FLINT) system. This study also utilized Initiation-Response-Follow-up Acts (IRF) to identify the categories of initiation, response, and follow-up acts used in CTE classrooms at the University of Mindanao. The study utilized purposive sampling to select the participants. Then, data collection was done through an interview and observation conducted among eight (8) participants: five (5) CTE teachers for the interview and three (3) CTE classrooms for a 2-hour observation each. The results show that in terms of initiation, students occasionally initiate conversation, and then teachers often follow up with a response. Thus, it does not imply that follow-up happens after every contact in the classroom. Moreover, the data gathered revealed that the teacher and students take equal turns in the learning process. Teachers encouraged students to reflect on their thinking and learning processes and promote student autonomy. This includes accepting students' feelings, praising or encouraging them, using their ideas, asking questions, and giving time to support silent and confused students during class. On the other hand, students occasionally respond to teachers' initiation and even initiate queries and share ideas. Regarding turn-taking, it was found that one or two moves are needed, such as a follow-up move and an initiating move or a responding move and an initiating move. Hence, it cannot be argued that the order of initiation-response-follow always governs classroom engagement.
This study examined the stress levels and coping mechanisms of high-achieving and low-achieving students in a hyblend learning modality, with the goal of establishing a foundation for the development of a psychosocial support program. Data were gathered from a varied group of 300 college students from National University (NU) using a mixed-methods technique that involved questionnaires and semi-structured interviews. The findings indicate that student who perform well academically face increased levels of stress, mostly as a result of feelings of powerlessness and a lack of belief in their own abilities. In contrast, high-performing students utilize a greater number of problem-focused and emotion-focused coping techniques, whereas low-performing individuals tend to rely more heavily on avoidant coping mechanisms. The study revealed no statistically significant disparity in stress levels or coping mechanisms between the two groups. This implies that variables other than academic achievement, such as individual characteristics and contextual influences, may have a substantial impact on students' experiences of stress and their reactions to it. These findings emphasize the necessity of implementing comprehensive psychosocial therapies specifically designed to address the unique difficulties encountered by kids who perform at both high and low levels. A need to develop more specific support measures and further investigation on the root causes of stress and coping mechanisms in academic environments. This study enhances comprehension of the intricate correlation among academic achievement, stress, and coping strategies, calling for a comprehensive approach to student support services.
This study aimed to investigate the challenges faced and the strategies employed by Filipino educators in teaching English expository writing based on their lived experiences. Participants who were enrolled in the program Doctor of Philosophy in Education, major in English during the School Year 2022-2023 in a private university in the Philippines were selected through convenience sampling method. Labov’s thematic analysis approach was utilized as the framework for narrative analysis. Based on the findings of this research, the participants normally experience the challenges of cultural diversity, writing proficiency, time constraints, resource limitations, diverse learning styles, and student engagement. In order to navigate such challenges, however, the participants employ various strategies. For cultural diversity, the participants resort to the enhancement of a culturally inclusive content, respectful feedback, and peer collaboration. In terms of writing proficiency, the participants utilize the strategies of differentiation, skill building, and individualized support. To address time constraints, the courses of action of educators are prioritizing key concepts, chunking and sequencing, and structured lesson plans. In coping with resource limitations, the teachers of English expository writing utilize adaptable materials, minimalist approaches, and collaborative learning in their teaching methods. As regards diversity in students’ learning styles, the teachers adapt multimodal instruction, student choice, and universal design. Lastly, the lack of student engagement is being addressed through interactive activities, real-world relevance, and gamification. A researcher-designed comprehensive training and development plan was recommended to further improve the educators’ skills in teaching expository writing. This training and development model is envisioned to provide opportunities for teachers to upskill their pedagogies and better improve their teaching effectiveness. To verify the validity of the results, it is strongly recommended that a larger-scale variant of this study be conducted.
There are different factors and reasons why a subject is treated as the students’ weakness or a very challenging one especially when its nature is boring or not in line with the students’ interest. This study described the experiences of the learners and teachers experiences in the subject “The Works and Life of Jose Rizal” at Cebu Technological University – Moalboal Campus, Cebu Province for the school year 2023 – 2024. This study used the Husserl Phenomenology. The sampling design utilized in this study was purposive sampling following established inclusion criteria to identify the participants of the study. 13 participants volunteered to be part of the study. This study used Braun and Clarke’s Thematic Analysis to analyze the experiences of the participants. There are three emerging themes that this research generated. These are the (1) Symbol of Filipino Nationalism, (2) Struggle of Bridging Past and Present in Education, and (3) Strategic Instruction for Effective Learning. These themes are pertinent in understanding what is happening inside a classroom where teachers and students share their experiences in learning and appreciating the Life and Works of Rizal. It is highly recommended to encourage teachers to explore different strategies and methods in teaching the subject to make it more engaging and absorbing.
This study assessed the reading comprehension skills of the Grade 6 Pupils during the School Year 2022-2023. It sought to answer the following questions which involved the profile of the student-respondents in terms of age, sex, economic status, educational attainment of parents and their relationship to the parents and the teachers. Also, the reading problems encountered by the Grade 6 learners and their level of reading comprehension skills of the students based on phonetic awareness, phonics, decoding, word identification, fluency, vocabulary and comprehension. Likewise, a significant relationship between the profile of the pupils and their reading comprehension skills was also determined. The program of activities can be adopted by the teachers to improve the reading comprehension skills of Grade 6 pupils served as output of the study. Findings showed that the Grade 6 learners are younger female-dominated learners with middle socioeconomic status backgrounds whose parents neither finished secondary level nor degree holder and undergraduate units with either one or both parents are working. Further, learners in the division had supervision with their parents and had regular study habit. The reading problems encountered by the Grade 6 learners are still developing with a focus on basic understanding and making meaning on fluency, vocabulary, background knowledge, comprehension strategies, lack of self-motivation, lack of background knowledge and inability to comprehend the texts were the foremost problem. Likewise, the reading comprehension ability of learners along vocabulary knowledge is developing. Further, the supervision of parents and good study habits influence the reading comprehension of learners. Additionally, these strategies could strengthen the comprehension ability of learners in school and at home. It is recommended in the study that the teachers should provide learners with specific resources or strategies aligned with their demographic background, e.g., culturally relevant texts for students from a specific background. Thus, an intervention of interactive materials shall be developed to address the concerns of Grade 6 learners in reading.
The purpose of this study was to evaluate the level of technology utilization and its impact on learner engagement in the classrooms of Kinder-2nd Grade teachers for the school year 2023-2024. The study examined respondents' demographic profiles and the usage levels of various technologies in the classroom, including Educational Online Videos, Independent Online Programs, Gamified Online Programs, and Interactive Technology. Additionally, learner engagement was assessed in relation to these applied technologies. The study employed a descriptive-comparativedevelopmental design, integrating both quantitative and qualitative approaches. A purposive sampling method was used to select respondents, and data were collected through survey questionnaires and focus group discussions. Statistical analysis was conducted using frequency, percentage, mean, standard deviation, t-tests, and one-way Analysis of Variance, supported by PostHoc Analysis using Scheffe's method. Results revealed that educational online videos were the most frequently utilized technology, while interactive technology was the least utilized. Conversely, learners showed the highest engagement with interactive technology and the least engagement with independent online learning. The study found that age, sex, and years of teaching experience significantly impacted the level of technology utilization, whereas educational attainment did not. Additionally, the findings indicated that learners were more engaged when interactive technology was employed in the classroom, despite teachers' lower utilization levels of such technology. The study also highlighted challenges faced by teachers, including learners' proficiency in digital literacy and the need for an assessment tool to evaluate learner engagement. In conclusion, the study demonstrates that the level of technology utilization by teachers has a significant impact on learner engagement in the classroom.
This study was conducted to determine the extent of work-related stressors of secondary teachers and its relationship to their job performance in secondary schools of Pasig City during the school year 2023-2024 to serve as a basis for a proposed training program. The design used in this study was descriptive correlational with the survey questionnaire as the main data gathering instrument. The weighted mean, t-test and Pearson r were the statistical tools used to treat the data. The respondents were composed of 236 teachers and five school heads in public secondary schools in District II, Division of Pasig City. Based on the findings, the teacher's extent of experience with work-related stressors based on the three key indicators (i.e. workload, students' behavior, and responsiveness to stakeholders), was moderate with a grand weighted mean of 1.58. in addition, Both the teachers and the school heads strongly agree with the indicators that describe the level of teacher's performance in terms of classroom management, lesson planning, lesson delivery and assessment of learning. Likewise, there was no significant relationship between the work-related stressors experienced by the teachers and their level of performance. Furthermore, stress prevention and management were deemed necessary, thus, was proposed in this study.
The educational system of the Philippines has adopted a bilingual policy of using both Filipino and English as languages of instruction at all academic levels. This bilingual policy has led to the development and nurturing of English and Filipino languages in the school domain, where students receive teachers' guidance and motivation to enhance their learning performance. In the home or family domain, the parents and other family members likewise provide support to ensure continuing learning of the dual languages. Previous researches affirmed that parents are highly reliable assessors of the children's language learning and development and that parental attitudes and involvement are crucial to the bilingual learning of children. This paper explored the parental attitudes on bilingualism from the vantage point of the family domain. Fifteen employees of the General Services Department of a leading autonomous tertiary institution with children presently enrolled in Grade School and Junior High School participated in this qualitative study. Results confirmed that all participants expressed a positive attitude toward the bilingual policy of the school. They also identified the benefits and drawbacks of the bilingual policy. Finally, to support the bilingual education of their children, different strategies and resources, such as improved language practices, use of educational technology, and parent-teacher collaboration, may be employed.
Loading Preview
Sorry, preview is currently unavailable. You can download the paper by clicking the button above.
Psychology and Education: A Multidisciplinary Journal , 2023
Related topics
- We're Hiring!
- Help Center
- Find new research papers in:
- Health Sciences
- Earth Sciences
- Cognitive Science
- Mathematics
- Computer Science
- Academia ©2024
Climate change and artificial intelligence: assessing the global research landscape
- Perspective
- Open access
- Published: 28 September 2024
- Volume 4 , article number 64 , ( 2024 )
Cite this article
You have full access to this open access article
- Joanna I. Lewis ORCID: orcid.org/0000-0002-5362-1308 1 ,
- Autumn Toney 2 , 3 &
- Xinglan Shi 4
Artificial Intelligence (AI) could revolutionize our ability to understand and address climate change. Studies to date have focused on specific AI applications to climate science, technologies, and policy. Yet despite the vast demonstrated potential for AI to change the way in which climate research is conducted, no study has presented a systematic and comprehensive understanding of the way in which AI is intersecting with climate research around the world. Using a novel merged corpus of scholarly literature which contains millions of unique scholarly documents in multiple languages, we review the community of knowledge at the intersection of climate change and AI to understand how AI methods are being applied to climate-related research and which countries are leading in this area. We find that Chinese research institutions lead the world in publishing and funding research at the intersection of climate and AI, followed by the United States. In mapping the specific AI tasks or methods being applied to specific climate research fields, we highlight gaps and identify opportunities to expand the use of AI in climate research. This paper can therefore greatly improve our understanding of both the current use and the potential use of AI for climate research.
Explore related subjects
- Artificial Intelligence
Avoid common mistakes on your manuscript.
1 Background
Artificial Intelligence (AI) could revolutionize our ability to understand and address climate change. AI tasks and methods can increase the speed of problem solving with applications for better understanding the causes of climate change, responding to its impacts, and formulating solutions [ 1 , 6 , 11 ].
Today, scholars have begun to analyze the potential role that AI could play in addressing global climate change, both through improving our scientific understanding of the causes and impacts of climate change and by helping to develop solutions [ 22 , 57 ]. We are increasingly seeing examples of how AI and machine learning can be used to improve the accuracy of climate system modeling [ 5 ], fill time series data gaps [ 26 ], estimate emissions inventories [ 20 ], refine climate scenario projections [ 44 ] and climate impact assessments [ 12 ], as well as develop applications for low carbon technology deployment through power, transportation and building system optimization [ 7 , 8 ].
Multiple studies have shown that that AI simulations and machine learning are being integrated into weather and climate modeling, including emulating and forecasting weather patterns and climate processes with greater consistency, data efficiency, and improved generalization [ 28 , 32 , 36 , 54 , 55 ]. AI is used in in flood risk modeling frameworks to increase the performance and accuracy of prediction methods [ 43 , 46 , 61 ]. Using neural networks for weather and climate modeling has improved agriculture and crop yield predictions under a range of climate scenarios, and machine learning algorithms have been applied in areas such as monitoring soil quality, managing crops, and modeling evapotranspiration, rainfall, drought, and pest outbreaks [ 15 , 50 , 60 ].
AI algorithms are increasingly being used for improving the efficient management of natural resources. For example, combining deep learning with statistical techniques could create more useful assessments of the impact of deforestation on rising carbon emissions in metropolitan areas [ 34 ]. In addition, machine learning approaches are being applied in developing low carbon materials [ 19 ]; for example the application of machine learning in optimizing concrete and steel production have demonstrated how AI can be integrated into supply chain modeling for heavy industries [ 24 , 39 , 51 ]. AI frameworks have been applied to minimize water consumption and emissions from oil and gas reservoirs, while other research has demonstrated methods using machine learning in assessing the carbon footprint of buildings [ 13 , 16 , 29 , 47 ].
Many studies have used AI methods in renewable energy research and demonstrated the broadening number of use cases for integrating AI into renewable energy systems. AI techniques are becoming a key tool in deploying data-integrated renewable energy networks [ 2 , 4 , 23 , 37 ]; estimating and forecasting solar radiation resources [ 17 , 30 , 31 , 38 ] and wind energy resources; [ 18 , 25 , 63 ] as well as in micro-grid management [ 27 , 42 , 58 ].
Additionally, AI has been shown to be a powerful tool to assess and develop carbon markets and generate more accurate carbon price models, including dynamic carbon pricing mechanisms [ 3 ], and more robust comparison models for carbon price forecasting [ 56 ]. Such methods have been applied to studies of emissions trading schemes including in China [ 35 ] and the UK [ 45 ].
While we have a sense of the general scope of climate change research being undertaken [ 21 , 49 , 52 , 62 ], and studies have previously laid out the potential for AI to improve climate research and enable the achievement of global sustainable development goals [ 48 , 53 ], no studies to date have taken a systematic and comprehensive approach to characterizing the way in which AI is intersecting with climate change research at a large scale, despite the vast demonstrated potential for AI to change the way in which climate research is conducted.
In this paper we map the community of knowledge at the intersection of climate change and AI to review how AI methods are being applied to climate related research, and which countries are leading in the application of AI to climate research. In mapping the specific AI tasks or methods being applied to specific climate research fields, we highlight gaps and identify opportunities to expand the use of AI in climate-related research.
Our analysis is based on a novel merged corpus of scholarly literature which contains millions of unique scholarly documents in multiple languages, and associated research clusters which are organized into a Map of Science. This is the first such study of the application of AI tasks and methods to climate change research using such a comprehensive data set. This paper can therefore greatly improve our understanding of both the current use and the potential use of AI for climate research.
In order to map the community of knowledge at the intersection of climate change and AI, we use a novel merged corpus of global scholarly literature, including Digital Science’s Dimensions, Clarivate’s Web of Science, Microsoft Academic Graph, China National Knowledge Infrastructure, arXiv, and Papers with Code, with CSET’s Map of Science. Footnote 1 This dataset allows for a far more comprehensive review than most traditional bibliometric analyses. In addition, it includes more than 120,000 research clusters derived from citation relationships within the merged corpus. Research clusters are groupings of scholarly documents based on citation links, not on topic similarity or author networks; thus, research clusters are groupings of scientific publications that address similar research questions. Each research cluster includes a set of research publications and aggregated metadata generated from the member publications, such as, key areas of research (fields and topics), key researchers in the field, and key funders. Footnote 2
We perform our analysis by identifying climate change related research papers via a keyword search, linking the publications to their research clusters, and then analyzing research clusters of interest. Figure 1 illustrates our data collection pipeline, starting with a set of keyword publications and ending with a set of research clusters and their member publications. Each dot in the map of science represents a research cluster and is colored by its broad area of research.
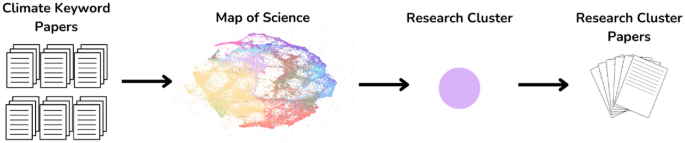
Data Collection Pipeline using CSET’s Merged Corpus and Map of Science
This scientific research data pipeline enables us to find research clusters of interest by locating research publications in the Map of Science. We can then look at a subset of research clusters and analyze aggregate statistics from their member papers. This approach to identifying scientific research of interest requires a seed set of publications. We generated a scientific research corpus of climate change literature ( \({R}_{climate}\) ) using a regular expression search. We generated a scientific research corpus of climate change literature using a regular expression search in English and Chinese, including terms for climate change, global warming, carbon emissions and low carbon (Table 1 ). Footnote 3 If a publication contains one of the terms in its title or abstract it is included in our climate change publication set.
We ran a search through the CSET merged corpus using the terms generated above; publications were selected as being related to climate change research if their title or abstract contained at least one keyword. We based these keywords on other studies that have conducted bibliometric analysis [ 21 ]. This search resulted in 947,616 climate change-related publications, which we refer to as \({R}_{climate}\) . We select RCs that contain at least one of these climate change publications, which results in 46,703 research clusters.
For each research cluster selected in this initial cluster search, we computed the percentage of papers that are contained in \({R}_{climate}\) out of the total number of papers in the RC. This allows us to sort and filter these resulting RCs based on the concentration of climate change-related papers. Our research cluster analysis for climate research includes 413,303 publications pulled from the 95th percentile of climate focused literature in our dataset which linked to 2,351 research clusters that have five percent or more \({R}_{climate}\) publications [ 33 ].
Our final filtering was through an identification of clusters with high percentages of AI-related publications. We use the AI percentage from the Map of Science, which identifies the concentration of AI-related publications in a given cluster. AI relatedness in English language publications were classified using a model trained on arXiv publications [ 14 ], and Chinese-language publications were classified using a regular expression query [ 10 ]. Thus, similarly to how we filter for climate change-related RCs, we can filter for AI-related RCs.
This allowed us to sort our dataset both by climate and AI relevance. We did this by looking at the clusters in both the 95th percentile of climate research and the 95th percentile of AI research. By selecting research clusters that have both 95% or more concentrations of climate change related publications and AI-related publications we identify 111 research clusters to analyze from the starting set of 2,351 climate change clusters.
Figure 2 displays the full Map of Science and the two subsets (climate change and climate change and AI) of research clusters we identify highlighted within it.
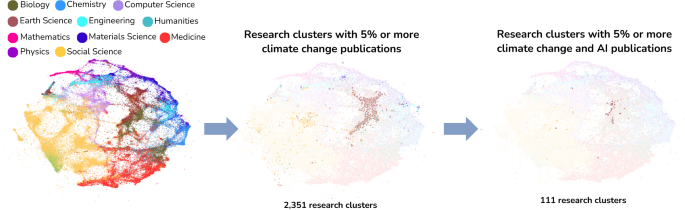
Climate Change and Climate Change AI Research Clusters Highlighted in the Map of Science
In the synthesis section we discuss further methods that were used to analyze and synthesize the dataset described above. This includes extracting 67 clusters that have either China, or the U.S. listed as the top country and have on average more than 2 citations per paper to filter for clusters with community engagement, and an examination of the leading AI and climate change tasks and methods by cluster at the individual publication level as described in Sects. 3.2 and 3.3 .
3 Synthesis
3.1 characterizing the climate change and ai research landscape.
In order to contextualize the landscape of climate change and AI research, we compare the general research fields and countries of publication for each research cluster set. Each research cluster is assigned a broad discipline from the following list: Biology, Chemistry, Computer Science, Earth Science, Engineering, Humanities, Materials Science, Mathematics, Medicine, Physics, and Social Science. This discipline assignment represents the majority of member papers in a given research cluster and does not indicate that every member paper falls unambiguously under this area. Figure 3 displays the percentages of climate change related research clusters by their general discipline (displaying discipline areas that have at least a 1% share of publications).
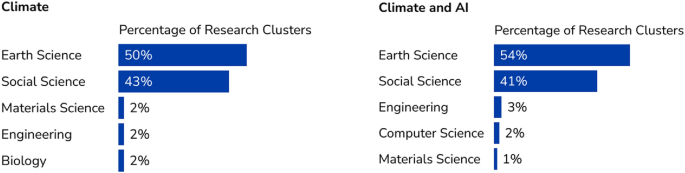
Comparison of Climate and Climate + AI Research Clusters by Discipline
The climate research cluster set is comprised of 50% earth science publications and 43% social science publications, and includes materials science, engineering and biology publications. In contrast, the climate and AI dataset is comprised of 54% earth science and 41% social science publications, along with some engineering, computer science and materials science publications. While there is not a huge difference in fields between climate research and climate and AI research, biology drops off and is replaced by computer science in the second category as a leading field.
Articles at the intersection of climate and AI research include multiple disciplines from both the natural and social sciences. While the earth sciences dominate the research clusters identified, this is very closely followed by the social sciences. It is somewhat surprising that engineering and computer science do not show up in greater percentages in this area, likely because most climate related research is in fact not being done in these fields, but rather the models and techniques are being applied by climate researchers in their respective fields. A potential limitation of these categorizations however is that much of this work is interdisciplinary and may in fact span the natural and social sciences.
3.2 Leading countries, institutions and funders
Each research publication is assigned country data using the location of the organization that an author is affiliated with. This means that if there are multiple authors from different countries, a given publication will have multiple countries assigned. For all member publications in a given research cluster, a “top country” categorization is assigned based on the country being listed on the most publications in that research cluster. We treat all EU-27 countries as one entity due to their high rates of collaboration and research funding allocation. Figure 4 displays the top five leading countries by research cluster count.
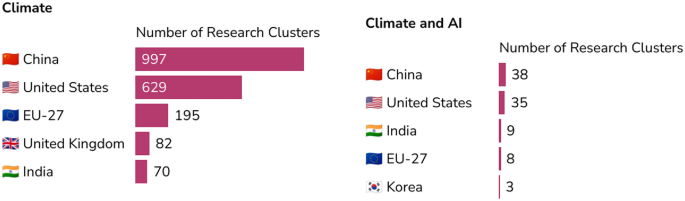
Comparison of Climate and Climate and AI Research Clusters by Top Country
We find that China produced more research in our climate research clusters and climate and AI research clusters, with U.S. authors producing the second highest number of research in both sets. It is perhaps not surprising given China’s role in climate change research shown here, and its strong role in AI research [ 41 ]. Yet China has a more sizable publication output lead in climate research generally than in climate and AI research. The other countries that produce significant climate and AI research outputs differ from those that produce more climate research generally. The EU-27, UK, and India follow China and the United States in climate research generally, while India, the EU-27, and South Korea follow China and the United States in research on climate and AI. It is worth noting that if results were adjusted by factors such as population size or other measures of capacity, the analysis would yield different results.
Due to the publication output lead that China and the U.S. hold, we further refine our set of 111 climate change and AI research cluster to the 67 clusters that have either China or the U.S. listed as the top country and have on average more than 2 citations per paper to filter for clusters with community engagement [ 33 ]. This allows us to examine a variety of relevant variables including: (1) leading countries of author affiliation; (2) leading research fields; (3) leading author affiliations; (4) leading funding organizations; (5) leading industry affiliations; and (6) AI-related tasks and methods; thereby facilitating a more granular analysis of the research landscape at the intersection of climate and AI.
In order to identify research institutes with the highest global publication output at the intersection of climate and AI, we examine the research institutes that the study authors are associated with. Footnote 4 The top 10 institutes are listed in Table 2 .
As China is the leading country by author affiliation as presented above, we see that many research institutes publishing at the intersection of climate and AI research are based in China. The Chinese Academy of Sciences, the largest research institute in China, is by far the dominant research institute where research at the intersection of climate and AI is being conducted. Within the Chinese Academy of Sciences (CAS), the leading research institute associated with climate change and AI publications in our database is University of the Chinese Academy of Sciences (438 publications), followed by the Institute of Geographic Sciences and Natural Resources (277 publications), and the Institute of Remote Sensing and Digital Earth (246 publications). Other leading Chinese research institutes include Beijing Normal University, Wuhan University, and Tsinghua University.
Within the United States, the University of Maryland, College Park has the largest number of publications in our climate and AI dataset, followed by the United States Geological Survey, University of Wisconsin-Madison, and the United States Forest Service. The two other countries with research institutes that show up in the top ten are the Netherlands and Australia.
We examine the observable leading funding organizations associated with climate and AI publications and find that China-based funding organizations have supported research that contributed to the largest number of publications, including the National Natural Science Foundation of China (4,391 publications) and China’s Ministry of Science and Technology (1,938 publications) in the first and second position. In third place is the United States National Science Foundation (1,527 publications), followed by the European Commission (998 publications) and the Chinese Academy of Sciences (710) which not only conducts but also funds research. The top ten funders are listed in Table 3 .
While no private companies appear as leading research institutes or funders, we took a closer look to determine which companies are the most associated with climate and AI publications in our database. The top five companies that appear in our database in either a funding capacity or research affiliation are Google based in the United States (62 publications), Science Systems and Applications based in the United States (30 publications), State Grid Corporation based in China (30 publications), Footnote 5 IBM based in the United States (22 publications), and Volkswagen Group based in Germany (15 publications).
The Chinese Academy of Sciences (CAS) is listed in Table 2 as being associated with the largest number of publications at the intersection of climate and AI by far. However, CAS is a large organization comprised of multiple research institutes distributed throughout the country. As a result, we took a closer look at the specific research institutes within CAS to better understand their contributions to research in this area. We found that the University of Chinese Academy of Sciences is the source of the highest number of publications, followed by the Institute of Geographic Sciences and Natural Resources Research, and the Institute of Remote Sensing and Digital Earth as listed in Table 4 .
The names of the CAS institutes give some indication of the type of research where AI is being applied to climate research, including in the areas of geographic sciences and remote sensing. More detail is available at the websites provided in Table 4 .
3.3 AI tasks and methods used in climate research fields
To better understand exactly how AI is being utilized within climate research, we examined the AI-related tasks and methods that are automatically assigned to individual research publications in our database using a named entity recognition model trained on tasks and methods as developed in [ 59 ]. Each task and method label falls under several broad areas, such as “natural language processing” or “causal inference.” For our analysis, we aggregated the tasks and methods that appeared in member publications of our 67 research clusters of interest. For each RC, we looked at the top five most frequent tasks and methods from the research clusters’ member publications and represented them in nine distinct categorizations from the “Papers with Code” taxonomy: causal inference, computer vision, graphs, methodology, natural language processing, neural networks, reinforcement learning, robots, and time series [ 40 ].
Next, we manually verified nine climate-related categorization labels based on the occurrence of keywords in the research cluster metadata: climate impacts, climate modeling, emission trends, energy efficiency, energy technology, energy trends, land use change, public perception, and transportation, based in part on the categories used in [ 48 ]. We then identified all distinct pairings between the nine AI-related tasks and methods and the nine climate-related categories. For example, if a research cluster had both climate modeling and neural networks labels, that would be represented in Table 5 by a checkmark. Footnote 6
In Table 5 we see a wide range of AI tasks and methods being applied to the 9 climate research areas that we extract from our climate and AI RC dataset. For example, we identify six AI tasks and methods being used in studies of climate impacts, including causal interference, computer vision, natural language processing, neural networks, robots and time series. Studies involving climate modeling are using at least five AI tasks and methods including computer vision, graphs, neural networks, robots and time series.
This analysis also reveals some areas of climate research that are using fewer AI tasks and methods. While energy technologies research is using multiple methods (examples include computer vision, AI methodology, natural language processing, and reinforcement learning), we see other areas of energy research such as energy trends studies and public perception studies using fewer methods. As a result, there appear to be gaps in certain climate research areas where AI tasks and methods are not being used as widely and where there may be useful applications. Exploring these gaps identified in Table 5 is an area for future research.
4 Discussion and conclusions
Given the vast potential of AI tasks and methods to revolutionize all aspects of research and analysis, it is not surprising that they are being applied to one of today’s most pressing global challenges, addressing climate change. Our study contributes to the understanding of how AI is being used in climate related research with three key findings.
First, we find that articles at the intersection of climate and AI research include multiple disciplines from both the natural and social sciences. While the earth sciences dominate the research clusters identified, this is very closely followed by the social sciences. It is somewhat surprising that engineering and computer science do not show up in greater percentages in this area, likely because most climate related research is in fact not being done in these fields. A potential limitation of these categorizations however is that much of this work is interdisciplinary and may in fact span the natural and social sciences.
Second, we find that Chinese research institutions lead the world in publishing and funding research at the intersection of climate and AI, followed by the United States. In examining the research institutes that the study authors are associated with, we find that just as China is the leading country by author affiliation as presented above, many of leading research institutes at the intersection of climate and AI research are based in China. The Chinese Academy of Sciences, the largest research institute in China, is by far the dominant research institute where research at the intersection of climate and AI is being conducted. We also find that the leading funders associated with climate and AI publications are also based in China: The National Natural Science Foundation of China and China’s Ministry of Science and Technology. China’s dominance in AI applications has been well documented, and we show that China also leads the world in climate released research, as well as at the climate-AI interface. This is also reflected in Chinese government policy; for example, the Chinese government has issued explicit guidance on the use of AI in climate research in the “Meteorological Science and Technology Development Plan (2021–2035)” issued by the Ministry of Science and Technology and Chinese Academy of Sciences in March 2022 [ 9 ].
Third, by mapping the specific AI tasks or methods being applied to specific climate research fields, we find gaps and identify opportunities to expand the use of AI in climate research. While we believe this is the first study to examine this in a systematic way, we acknowledge some deficiencies in our methods, namely that we manually identified subfields in climate research using some keyword analysis as well as some subjective judgement, and that our pairing of AI-related tasks and methods to climate-related research areas represents the occurrence but not the frequency of these pairings. However, our findings raise multipole questions that present opportunities for future research and inquiry, including why certain tasks and methods are being used in specific fields, and what other fields might learn from applications to date.
Of course, any effort to make broad generalizations about fields as vast and complex as the fields of climate change and AI comes with some limitations. There are likely applications of AI to climate research that are not included here due to limitations in our original search terms or in the way in which we develop climate subfields in order to map them against AI tasks and methods. These are rapidly involving fields of research in which new methods and applications are being developed all the time. Furthermore, the field of research at the intersection of AI and climate change is growing very rapidly, so any attempt to assess the state of the field could be quickly outdated.
Yet given the tremendous opportunity that emerging AI tools provide in addressing a challenge so vast and multifaceted as climate change, the study of their application is no doubt of tremendous academic and practical importance. This paper allows for a more globally comprehensive and nuanced analysis of this relationship than past studies and consequently provides a tangible contribution to our broader understanding of the use of AI tasks and methods in climate change research.
This study also examines the role of specific countries and specific funding organizations in shaping the direction of climate and AI research which will be increasingly important to understand. Furthermore, tensions between China and the West are already shaping national decisions about investments in AI research and could influence future research directions.
Given the very limited time remaining to avoid even more dangerous impacts of climate change globally, the expanded use of AI tasks and methods presents the opportunity to transform our ability to understand and address climate change. This paper helps to identify opportunities to expand the use of AI tasks and methods in climate related research, and the predominance of China and the United States in this area raises important questions about national leadership and competitiveness.
Data availability
The data used in this analysis is available on Mendeley Data [Joanna I. Lewis and Autumn Toney, “AI Applications in Climate Research Dataset” (Mendeley Data, 2024), https://doi.org/10.17632/wjwbwrn28p.1 .
Code availability
Not applicable.
China National Knowledge Infrastructure is furnished for use in the United States by East View Information Services, Minneapolis, MN, USA. Dimensions is provided by Digital Science, Web of Science is provided by Clarivate Analytics, and China National Knowledge Infrastructure is furnished for use in the United States by East View Information Services, Minneapolis, MN, USA.
The data for this study was extracted on April 21, 2022. The latest version of the full database is available at https://sciencemap.eto.tech/?mode=map .
Most non-English language publications translate the abstract into English so this search will include a range of non-English language publications. The most frequent exception to this is Chinese-language publications which is why we also include Chinese-language search terms.
Here, we shift our analysis here to the member publications of the research clusters, thus Tables 2 – 4 are counts of publications as opposed to research clusters.
The State Grid Corporation of China is technically a state-owned as opposed to a purely privately held company.
In this way, Table 5 denotes the AI-related tasks and methods that have been applied to climate-related areas but does not represent the frequency of these pairings.
Abbot J, Marohasy J. The application of machine learning for evaluating anthropogenic versus natural climate change. GeoResJ. 2017;14(December):36–46. https://doi.org/10.1016/j.grj.2017.08.001 .
Article Google Scholar
Ahmad T, Zhang D, Huang C, Zhang H, Dai N, Song Y, Chen H. Artificial intelligence in sustainable energy industry: status quo, challenges and opportunities. J Clean Prod. 2021;289(March): 125834. https://doi.org/10.1016/j.jclepro.2021.125834 .
Aiyegbusi O, Yalamova R, Usher J. Carbon pricing in dynamic regulation and changing economic environment—agent based model. Reg Bus Stud. 2011;3(1 Suppl.):497–509.
Google Scholar
Al-Othman A, Tawalbeh M, Martis R, Dhou S, Orhan M, Qasim M, Olabi AG. Artificial intelligence and numerical models in hybrid renewable energy systems with fuel cells: advances and prospects. Energy Convers Manage. 2022;253(February): 115154. https://doi.org/10.1016/j.enconman.2021.115154 .
Anderson GJ, Lucas DD. Machine learning predictions of a multiresolution climate model ensemble. Geophys Res Lett. 2018;45(9):4273–80. https://doi.org/10.1029/2018GL077049 .
Carozza DA, Boudreault M. A global flood risk modeling framework built with climate models and machine learning. J Adv Model Earth Syst. 2021. https://doi.org/10.1029/2020MS002221 .
Chen C, Yuhan Hu, Karuppiah M, Kumar PM. Artificial intelligence on economic evaluation of energy efficiency and renewable energy technologies. Sustain Energy Technol Assess. 2021;47(October): 101358. https://doi.org/10.1016/j.seta.2021.101358 .
Chen L, Chen Z, Zhang Y, Liu Y, Osman AI, Farghali M, Hua J, et al. Artificial intelligence-based solutions for climate change: a review. Environ Chem Lett. 2023;21(5):2525–57. https://doi.org/10.1007/s10311-023-01617-y .
China Meteorological Administration, and Chinese Academy of Sciences. 2022. China meteorological science and technology development plan (2021–2035) 中国气象科技发展规划 (2021—2035年). 2022. https://www.gov.cn/xinwen/2022-03/03/content_5676714.htm .
Daniel C. 2022. Counting AI Research: exploring ai research output in english- and chinese-language sources. https://cset.georgetown.edu/publication/counting-ai-research/ .
Cowls J, Tsamados A, Taddeo M, Floridi L. The AI gambit: leveraging artificial intelligence to combat climate change—opportunities, challenges, and recommendations. AI Soc. 2023;38(1):283–307. https://doi.org/10.1007/s00146-021-01294-x .
Crane-Droesch A. Machine learning methods for crop yield prediction and climate change impact assessment in agriculture. Environ Res Lett. 2018;13(11):114003. https://doi.org/10.1088/1748-9326/aae159 .
Ding C, Ke J, Levine M, Zhou N. Potential of artificial intelligence in reducing energy and carbon emissions of commercial buildings at scale. Nat Commun. 2024;15(1):5916. https://doi.org/10.1038/s41467-024-50088-4 .
Dunham J, Melot J, Murdick D. 2020. Identifying the development and application of artificial intelligence in scientific text. arXiv. https://doi.org/10.48550/arXiv.2002.07143 .
Kumar EPG, Lydia M. 2021. machine learning algorithms for modelling agro-climatic indices: a review. In smart computing techniques and applications, edited by Suresh Chandra Satapathy, Vikrant Bhateja, Margarita N. Favorskaya, and T. Adilakshmi, 15–23. Smart Innovation, Systems and Technologies. Singapore: Springer. https://doi.org/10.1007/978-981-16-1502-3_3 .
Soheil F, Srinivasan R. 2019. Climate change impacts on campus buildings energy use: an ai-based scenario analysis. In Proceedings of the 1st ACM International Workshop on Urban Building Energy Sensing, Controls, Big Data Analysis, and Visualization, 112–19. UrbSys’19. New York, NY, USA: Association for Computing Machinery. https://doi.org/10.1145/3363459.3363540 .
Feng Y, Cui N, Zhang Q, Zhao L, Gong D. Comparison of artificial intelligence and empirical models for estimation of daily diffuse solar radiation in north China plain. Int J Hydr Energy. 2017;42(21):14418–28. https://doi.org/10.1016/j.ijhydene.2017.04.084 .
Fu T, Wang C. A hybrid wind speed forecasting method and wind energy resource analysis based on a swarm intelligence optimization algorithm and an artificial intelligence model. Sustainability. 2018;10(11):3913. https://doi.org/10.3390/su10113913 .
Gu GH, Noh J, Kim I, Jung Y. Machine learning for renewable energy materials. J Mater Chem A. 2019;7(29):17096–117. https://doi.org/10.1039/C9TA02356A .
You H, Gopal A, Ouyang L, Key A. 2021. Estimation of corporate greenhouse gas emissions via machine learning. arXiv:2109.04318 [Cs, Stat], September. http://arxiv.org/abs/2109.04318 .
Haunschild R, Bornmann L, Marx W. Climate change research in view of bibliometrics. PLoS ONE. 2016;11(7): e0160393. https://doi.org/10.1371/journal.pone.0160393 .
Huntingford C, Jeffers ES, Bonsall MB, Christensen HM, Lees T, Yang H. Machine learning and artificial intelligence to aid climate change research and preparedness. Environ Res Lett. 2019;14(12): 124007. https://doi.org/10.1088/1748-9326/ab4e55 .
Jha SK, Bilalovic J, Jha A, Patel N, Zhang H. Renewable energy: present research and future scope of artificial intelligence. Renew Sustain Energy Rev. 2017;77(September):297–317. https://doi.org/10.1016/j.rser.2017.04.018 .
John N, Wesseling JH, Worrell E, Hekkert M. How key-enabling technologies’ regimes influence sociotechnical transitions: the impact of artificial intelligence on decarbonization in the steel industry. J Clean Prod. 2022;370(October): 133624. https://doi.org/10.1016/j.jclepro.2022.133624 .
Jursa R, Rohrig K. Short-term wind power forecasting using evolutionary algorithms for the automated specification of artificial intelligence models. Int J Forecast Energy Forecast. 2008;24(4):694–709. https://doi.org/10.1016/j.ijforecast.2008.08.007 .
Kadow C, Hall DM, Ulbrich U. Artificial intelligence reconstructs missing climate information. Nat Geosci. 2020;13(6):408–13. https://doi.org/10.1038/s41561-020-0582-5 .
Karim MA, Currie J, Lie T-T. A distributed machine learning approach for the secondary voltage control of an islanded micro-grid. 2016 IEEE Innov Smart Grid Technol Asia. 2016. https://doi.org/10.1109/ISGT-Asia.2016.7796454 .
Kashinath K, Mustafa M, Albert A, Wu J-L, Jiang C, Esmaeilzadeh S, Azizzadenesheli K, et al. Physics-informed machine learning: case studies for weather and climate modelling. Philos Trans Royal Soc A. 2021;379(2194):20200093. https://doi.org/10.1098/rsta.2020.0093 .
Article MathSciNet Google Scholar
Katterbauer K, Sofi AA, Marsala A, Yousif A. An innovative artificial intelligence framework for reducing carbon footprint in reservoir management. OnePetro. 2021. https://doi.org/10.2118/205856-MS .
Khosravi A, Nunes RO, Assad MEH, Machado L. Comparison of artificial intelligence methods in estimation of daily global solar radiation. J Clean Prod. 2018;194(September):342–58. https://doi.org/10.1016/j.jclepro.2018.05.147 .
Kuo P-H, Huang C-J. A green energy application in energy management systems by an artificial intelligence-based solar radiation forecasting model. Energies. 2018;11(4):819. https://doi.org/10.3390/en11040819 .
Levy O, Shahar S. Artificial intelligence for climate change biology: from data collection to predictions. Integr Compe Biol. 2024. https://doi.org/10.1093/icb/icae127 .
Lewis JI, Toney A. AI applications in climate research dataset. Mendeley Data. 2024. https://doi.org/10.17632/wjwbwrn28p.1 .
Lobo S, Amin I, Agarwal M, Gurnani R, Priya RL. Analyzing the impact of deforestation and population on carbon footprint in indian cities using statistical and deep learning techniques. Advances in intelligent systems and computing. In: Sivakumar Reddy V, Kamakshi Prasad V, Wang J, Reddy KTV, editors. Soft computing and signal processing. Singapore: Springer; 2021. p. 89–99. https://doi.org/10.1007/978-981-33-6912-2_9 .
Chapter Google Scholar
Lu H, Ma X, Huang K, Azimi M. Carbon trading volume and price forecasting in China using multiple machine learning models. J Clean Prod. 2020;249(March): 119386. https://doi.org/10.1016/j.jclepro.2019.119386 .
Martin TCM, Rocha HR, Perez GMP. Fine scale surface climate in complex terrain using machine learning. Int J Climatol. 2021;41(1):233–50. https://doi.org/10.1002/joc.6617 .
Mazzeo D, Herdem MS, Matera N, Bonini M, Wen JZ, Nathwani J, Oliveti G. Artificial intelligence application for the performance prediction of a clean energy community. Energy. 2021;232(October): 120999. https://doi.org/10.1016/j.energy.2021.120999 .
Mehdizadeh S, Behmanesh J, Khalili K. Comparison of artificial intelligence methods and empirical equations to estimate daily solar radiation. J Atmos Solar Terr Phys. 2016;146(August):215–27. https://doi.org/10.1016/j.jastp.2016.06.006 .
Meng H, Wang W. Definition method for carbon footprint of iron and steel energy supply Chain based on relational dispersed degree. J Intell Fuzzy Syst. 2020;38(6):7407–16. https://doi.org/10.3233/JIFS-179814 .
Meta AI Research. 2023. Papers with code—the methods corpus. 2023. https://paperswithcode.com/methods .
Min C, Zhao Y, Yi B, Ding Y, Wagner CS. Has China caught up to the US in AI research? An exploration of mimetic isomorphism as a model for late industrializers. arXiv. 2023. https://doi.org/10.48550/arXiv.2307.10198 .
Mishra M, Panigrahi RR, Rout PK. A combined mathematical morphology and extreme learning machine techniques based approach to micro-grid protection. Ain Shams Eng J. 2019;10(2):307–18. https://doi.org/10.1016/j.asej.2019.03.011 .
Mosavi A, Ozturk P, Chau K-W. Flood prediction using machine learning models: literature review. Water. 2018;10(11):1536. https://doi.org/10.3390/w10111536 .
Nichol JJ, Peterson MG, Peterson KJ, Matthew Fricke G, Moses ME. Machine learning feature analysis illuminates disparity between E3SM climate models and observed climate change. J Comput Appl Math. 2021;395:113451. https://doi.org/10.1016/j.cam.2021.113451 .
Ojo M. The future of UK Carbon pricing: artificial intelligence and the emissions trading system. MPRA paper. University Library of Munich, Germany. 2019. https://econpapers.repec.org/paper/pramprapa/94887.htm .
Park SJ, Lee DK. Prediction of coastal flooding risk under climate change impacts in South Korea using machine learning algorithms. Enviro Res Lett. 2020;15(9):094052. https://doi.org/10.1088/1748-9326/aba5b3 .
Ploszaj-Mazurek M, Rynska E, Grochulska-Salak M. Methods to optimize carbon footprint of buildings in regenerative architectural design with the use of machine learning, convolutional neural network, and parametric design. Energies. 2020;13(20):5289. https://doi.org/10.3390/en13205289 .
Rolnick D, Donti PL, Kaack LH, Kochanski K, Lacoste A, Sankaran K, Ross AS, et al. 2019. Tackling climate change with machine learning. arXiv:1906.05433 [Cs, Stat], November. http://arxiv.org/abs/1906.05433 .
Shaamala A, Yigitcanlar T, Nili A, Nyandega D. Algorithmic green infrastructure optimization: review of artificial intelligence driven approaches for tackling climate change. Sustain Cities Soc. 2024;101(February): 105182. https://doi.org/10.1016/j.scs.2024.105182 .
Shin J-Y, Kim KR, Ha J-C. Seasonal forecasting of daily mean air temperatures using a coupled global climate model and machine learning algorithm for field-scale agricultural management. Agric For Meteorol. 2020;281:107858. https://doi.org/10.1016/j.agrformet.2019.107858 .
Thilakarathna PSM, Seo S, Kristombu Baduge KS, Lee H, Mendis P, Foliente G. Embodied carbon analysis and benchmarking emissions of high and ultra-high strength concrete using machine learning algorithms. J Clean Prod. 2020;262(July): 121281. https://doi.org/10.1016/j.jclepro.2020.121281 .
Verendel V. Tracking artificial intelligence in climate inventions with patent data. Nat Clim Chang. 2023;13(1):40–7. https://doi.org/10.1038/s41558-022-01536-w .
Vinuesa R, Azizpour H, Leite I, Balaam M, Dignum V, Domisch S, Felländer A, Langhans SD, Tegmark M, Nerini FF. The role of artificial intelligence in achieving the sustainable development goals. Nat Commun. 2020;11(1):233. https://doi.org/10.1038/s41467-019-14108-y .
Watson-Parris D. Machine learning for weather and climate are worlds apart. Philos Trans Royal Soc A. 2021;379(2194):20200098. https://doi.org/10.1098/rsta.2020.0098 .
Watt-Meyer O, Brenowitz ND, Clark SK, Henn B, Kwa A, Jeremy McGibbon W, Perkins A, Bretherton CS. Correcting weather and climate models by machine learning nudged historical simulations. Geophys Res Lett. 2021. https://doi.org/10.1029/2021GL092555 .
Wei S, Chongchong Z, Cuiping S. Carbon pricing prediction based on wavelet transform and K-ELM optimized by bat optimization algorithm in China ETS: the case of Shanghai and Hubei carbon markets. Carbon Manage. 2018;9(6):605–17. https://doi.org/10.1080/17583004.2018.1522095 .
World Economic Forum. 2018. Harnessing artificial intelligence for the earth. fourth industrial revolution for the earth series. http://www3.weforum.org/docs/Harnessing_Artificial_Intelligence_for_the_Earth_report_2018.pdf .
Yao W. Analysis on the Application of the Artificial Intelligence Neural Network on the New Energy Micro Grid. In: Yao W, editor. Proceedings of the 2017 4th international conference on machinery, materials and computer (MACMC 2017). Xi’an: Atlantis Press; 2018. https://doi.org/10.2991/macmc-17.2018.144 .
Yousuf RB, Biswas S, Kaushal KK, Dunham J, Gelles R, Muthiah S, Self N, Butler P, Ramakrishnan N. Lessons from deep learning applied to scholarly information extraction: what works, what doesn’t, and future directions. arXiv. 2022. https://doi.org/10.48550/arXiv.2207.04029 .
Yu H, Wen X, Li Bo, Yang Z, Min Wu, Ma Y. Uncertainty analysis of artificial intelligence modeling daily reference evapotranspiration in the Northwest end of China. Comput Electron Agric. 2020;176(September): 105653. https://doi.org/10.1016/j.compag.2020.105653 .
Zabihi O, Siamaki M, Gheibi M, Akrami M, Hajiaghaei-Keshteli M. A smart sustainable system for flood damage management with the application of artificial intelligence and multi-criteria decision-making computations. Int J Dis Risk ReducT. 2023;84(January): 103470. https://doi.org/10.1016/j.ijdrr.2022.103470 .
Zennaro F, Furlan E, Simeoni C, Torresan S, Aslan S, Critto A, Marcomini A. Exploring machine learning potential for climate change risk assessment. Earth Sci Rev. 2021;220(September): 103752. https://doi.org/10.1016/j.earscirev.2021.103752 .
Zhao X, Wang C, Jinxia Su, Wang J. Research and application based on the swarm intelligence algorithm and artificial intelligence for wind farm decision system. Renew Energy. 2019;134(April):681–97. https://doi.org/10.1016/j.renene.2018.11.061 .
Download references
Acknowledgements
We would like to thank Igor Mikolic-Torreira, Dewey Murdick, Melissa Flagg and Catherine Aiken for feedback on earlier versions of this paper. For research assistance we would like to thank Laura Edwards.
Partial financial support was received from the Center for Security and Emerging Technology (CSET) at Georgetown University.
Author information
Authors and affiliations.
Science, Technology and International Affairs Program, Edmund A. Walsh School of Foreign Service, Georgetown University, Washington, DC, USA
Joanna I. Lewis
Center for Security and Emerging Technology, Edmund A. Walsh School of Foreign Service, Georgetown University, Washington, DC, USA
Autumn Toney
Department of Computer Science, Georgetown University, Washington, DC, USA
Communications, Culture and Technology Program, Georgetown University, Washington, DC, USA
Xinglan Shi
You can also search for this author in PubMed Google Scholar
Contributions
All authors contributed to the study conception and design. All authors contributed to material preparation, data collection and analysis. The first draft of the manuscript was written by Joanna Lewis and all authors commented on previous versions of the manuscript. All authors read and approved the final manuscript.
Corresponding author
Correspondence to Joanna I. Lewis .
Ethics declarations
Competing interests.
The authors have no competing interests to declare that are relevant to the content of this article.
Additional information
Publisher's note.
Springer Nature remains neutral with regard to jurisdictional claims in published maps and institutional affiliations.
Rights and permissions
Open Access This article is licensed under a Creative Commons Attribution-NonCommercial-NoDerivatives 4.0 International License, which permits any non-commercial use, sharing, distribution and reproduction in any medium or format, as long as you give appropriate credit to the original author(s) and the source, provide a link to the Creative Commons licence, and indicate if you modified the licensed material. You do not have permission under this licence to share adapted material derived from this article or parts of it. The images or other third party material in this article are included in the article’s Creative Commons licence, unless indicated otherwise in a credit line to the material. If material is not included in the article’s Creative Commons licence and your intended use is not permitted by statutory regulation or exceeds the permitted use, you will need to obtain permission directly from the copyright holder. To view a copy of this licence, visit http://creativecommons.org/licenses/by-nc-nd/4.0/ .
Reprints and permissions
About this article
Lewis, J.I., Toney, A. & Shi, X. Climate change and artificial intelligence: assessing the global research landscape. Discov Artif Intell 4 , 64 (2024). https://doi.org/10.1007/s44163-024-00170-z
Download citation
Received : 30 April 2024
Accepted : 12 September 2024
Published : 28 September 2024
DOI : https://doi.org/10.1007/s44163-024-00170-z
Share this article
Anyone you share the following link with will be able to read this content:
Sorry, a shareable link is not currently available for this article.
Provided by the Springer Nature SharedIt content-sharing initiative
- Climate change
- AI tasks and methods
- Publication analysis
Advertisement
- Find a journal
- Publish with us
- Track your research
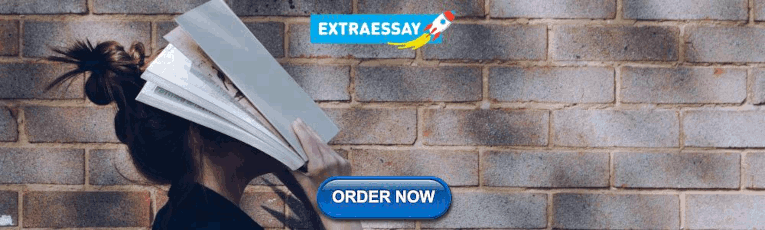
IMAGES
VIDEO
COMMENTS
This brief paper summarizes a mixed method review of over 500 neuroscientific reports investigating the proposition that general intelligence (g or IQ) and multiple intelligences (MI) can be integrated based on common and unique neural systems. Extrapolated from this interpretation are five principles that inform teaching and curriculum so that education can be strengths-based and personalized ...
The multiple intelligence theory posits that one can develop abilities within each intelligence over time, altering one's unique combination of strengths and weaknesses (Gardner, 1983). According ...
This research aimed to determine if there is neuroscience evidence that provides support for the neural validity of the eight identified intelligences. Methods: This investigation reviewed 318 neuroscience reports and extracted neural regions cited for the core cognitive components of each intelligence.
Since Gardner suggested that human beings hold multiple intelligences, numerous teachers have adapted and incorporated the multiple intelligence theory (MIT) into their daily routine in the classroom. However, to date, the efficacy of MIT-inspired methodologies remains unclear. The focus of the present study was to perform a systematic review and a meta-analysis to assess the impact of ...
Multiple Intelligences In addition to his previous interest in and work on cognitive abilities, Gard- ner's participation in a research project funded by the Bernard van Leer Foun- dation contributed to the writing of Frames of Mind. This project focused on conducting research on human potential.
This paper will examine the theory of Multiple Intelligences (MI), view the applications in the classroom, and observe the arguments by the advocates for and the dissenters against the theory of ...
Abstract The applications of multiple intelligence theory in education are wide. Students apply the learning in the classroom according to their own dominant intelligence and learning style, which is most effective for them. Combining learning styles with dominant intelligences enhances the students' learning processes .
Abstract This brief paper summarizes a mixed method review of over 500 neuroscientific reports investigating the proposition that general intelligence (g or IQ) and multiple intelligences (MI) can be integrated based on common and unique neural systems. Extrapolated from this interpretation are five principles that inform teaching and curriculum so that education can be strengths-based and ...
Abstract The present mixed-methods classroom-based study investigated whether the provision of multiple intelligences teaching approach to physically disabled learners could contribute to activating multiple intelligences and have a positive impact on their classroom engagement. To address this issue, three intact classes of 10 Iranian physically disabled learners participated in this study ...
Abstract and Figures This brief paper summarizes a mixed method review of over 500 neuroscientific reports investigating the proposition that general intelligence (g or IQ) and multiple ...
The theory of multiple intelligences, developed by psychologist Howard Gardner in the. late 1970's and early 1980's, posits that individuals possess eight or more relatively autonomous. intelligences. Individuals draw on these intelligences, individually and corporately, to create. products and solve problems that are relevant to the ...
This paper is the result of a review of 40 articles related to learning activities that use multiple intelligence-based approaches, media, and learning models, published from 2011 to 2021. The multiple intelligences theory was put forward by Howard Gardner, an expert in education and psychology.
Abstract. This article, to be submitted to the national journal, Gifted Child Today, explores how Howard Gardner's Multiple Intelligences Theory (MI) specifically affects the development and delivery of gifted education. Included are discussions of the definitions on MI and giftedness programming and how each approach identification, curriculum ...
Abstract: This paper presents findings from an action research project that investigated the application of Multiple Intelligences (MI) theory in classrooms and schools.
Adapting teaching methods to the "multiple intelligences" of students leads to better learning. The opening survey statement from Blanchette Sarrasin et al. (2019) caught Howard Gardner's attention, because it clearly draws from his Multiple Intelligences (henceforth MI) theory (Gardner, 1983). In a recent paper, Gardner (2020) says he was disturbed by this so-called "neuromyth," both ...
PDF | On Jan 1, 2022, Yuxi Wu and others published A Review of Multiple Intelligence Theory | Find, read and cite all the research you need on ResearchGate
Proposed by Howard Gardner in 1983, the theory of multiple intelligences has revolutionized how we understand intelligence. Learn more about the research behind his theory.
A neuromyth is a commonly accepted but unscientific claim about brain function. Many researchers have claimed Howard Gardner's multiple intelligences (MI) theory is a neuromyth because they have seen no evidence supporting his proposal for independent brain-based intelligences for different types of cognitive abilities.
The present study attempts to investigate the relationship between the multiple intelligences and the academic performance achievement levels of high school students based on Gardner's multiple intelligences theory. This was a descriptive correlation study. To accomplish this purpose, 270 students of high school of Bandar Abbas selected by clustering random sampling, and all of them filled ...
The concept of intelligence has been extensively studied, undergoing an evolution from a unitary concept to a more elaborate and complex multidimensional one. In addition, several research studies have focused their efforts for decades on the study of ...
PDF | The multiple intelligence theory (MIT) was proposed by Gardner in 1983, and since then it has been a major concern for many researchers. The... | Find, read and cite all the research you ...
This paper examines the theory of Multiple Intelligences (MI) as the most viable and effective platform for 21st century educational and instructional methodologies based on the understanding of the value of diversity in today's... more. Download. by Donovan McFarlane. 5.
As Felix (2020) suggests, further research is necessary to explore how different AI systems influence learner-instructor interaction in online education and to uncover the underlying reasons behind these effects. Methodology Research Design This study used a qualitative multiple-case study as a research design.
Artificial Intelligence (AI) could revolutionize our ability to understand and address climate change. Studies to date have focused on specific AI applications to climate science, technologies, and policy. Yet despite the vast demonstrated potential for AI to change the way in which climate research is conducted, no study has presented a systematic and comprehensive understanding of the way in ...
Research on multiple intelligences theory and its enlig htenment to. higher education. Haijun Wang*. Shandong University of Political Science and Law, Jinan, Shandong, China. ABSTRACT: The theory ...