How to think like a programmer — lessons in problem solving

By Richard Reis
If you’re interested in programming, you may well have seen this quote before:
“Everyone in this country should learn to program a computer, because it teaches you to think.” — Steve Jobs
You probably also wondered what does it mean, exactly, to think like a programmer? And how do you do it??
Essentially, it’s all about a more effective way for problem solving .
In this post, my goal is to teach you that way.
By the end of it, you’ll know exactly what steps to take to be a better problem-solver.
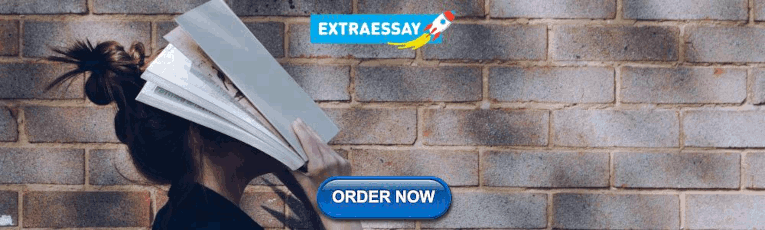
Why is this important?
Problem solving is the meta-skill.
We all have problems. Big and small. How we deal with them is sometimes, well…pretty random.
Unless you have a system, this is probably how you “solve” problems (which is what I did when I started coding):
- Try a solution.
- If that doesn’t work, try another one.
- If that doesn’t work, repeat step 2 until you luck out.
Look, sometimes you luck out. But that is the worst way to solve problems! And it’s a huge, huge waste of time.
The best way involves a) having a framework and b) practicing it.
“Almost all employers prioritize problem-solving skills first. Problem-solving skills are almost unanimously the most important qualification that employers look for….more than programming languages proficiency, debugging, and system design. Demonstrating computational thinking or the ability to break down large, complex problems is just as valuable (if not more so) than the baseline technical skills required for a job.” — Hacker Rank ( 2018 Developer Skills Report )
Have a framework
To find the right framework, I followed the advice in Tim Ferriss’ book on learning, “ The 4-Hour Chef ”.
It led me to interview two really impressive people: C. Jordan Ball (ranked 1st or 2nd out of 65,000+ users on Coderbyte ), and V. Anton Spraul (author of the book “ Think Like a Programmer: An Introduction to Creative Problem Solving ”).
I asked them the same questions, and guess what? Their answers were pretty similar!
Soon, you too will know them.
Sidenote: this doesn’t mean they did everything the same way. Everyone is different. You’ll be different. But if you start with principles we all agree are good, you’ll get a lot further a lot quicker.
“The biggest mistake I see new programmers make is focusing on learning syntax instead of learning how to solve problems.” — V. Anton Spraul
So, what should you do when you encounter a new problem?
Here are the steps:
1. Understand
Know exactly what is being asked. Most hard problems are hard because you don’t understand them (hence why this is the first step).
How to know when you understand a problem? When you can explain it in plain English.
Do you remember being stuck on a problem, you start explaining it, and you instantly see holes in the logic you didn’t see before?
Most programmers know this feeling.
This is why you should write down your problem, doodle a diagram, or tell someone else about it (or thing… some people use a rubber duck ).
“If you can’t explain something in simple terms, you don’t understand it.” — Richard Feynman
Don’t dive right into solving without a plan (and somehow hope you can muddle your way through). Plan your solution!
Nothing can help you if you can’t write down the exact steps.
In programming, this means don’t start hacking straight away. Give your brain time to analyze the problem and process the information.
To get a good plan, answer this question:
“Given input X, what are the steps necessary to return output Y?”
Sidenote: Programmers have a great tool to help them with this… Comments!
Pay attention. This is the most important step of all.
Do not try to solve one big problem. You will cry.
Instead, break it into sub-problems. These sub-problems are much easier to solve.
Then, solve each sub-problem one by one. Begin with the simplest. Simplest means you know the answer (or are closer to that answer).
After that, simplest means this sub-problem being solved doesn’t depend on others being solved.
Once you solved every sub-problem, connect the dots.
Connecting all your “sub-solutions” will give you the solution to the original problem. Congratulations!
This technique is a cornerstone of problem-solving. Remember it (read this step again, if you must).
“If I could teach every beginning programmer one problem-solving skill, it would be the ‘reduce the problem technique.’ For example, suppose you’re a new programmer and you’re asked to write a program that reads ten numbers and figures out which number is the third highest. For a brand-new programmer, that can be a tough assignment, even though it only requires basic programming syntax. If you’re stuck, you should reduce the problem to something simpler. Instead of the third-highest number, what about finding the highest overall? Still too tough? What about finding the largest of just three numbers? Or the larger of two? Reduce the problem to the point where you know how to solve it and write the solution. Then expand the problem slightly and rewrite the solution to match, and keep going until you are back where you started.” — V. Anton Spraul
By now, you’re probably sitting there thinking “Hey Richard... That’s cool and all, but what if I’m stuck and can’t even solve a sub-problem??”
First off, take a deep breath. Second, that’s fair.
Don’t worry though, friend. This happens to everyone!
The difference is the best programmers/problem-solvers are more curious about bugs/errors than irritated.
In fact, here are three things to try when facing a whammy:
- Debug: Go step by step through your solution trying to find where you went wrong. Programmers call this debugging (in fact, this is all a debugger does).
“The art of debugging is figuring out what you really told your program to do rather than what you thought you told it to do.”” — Andrew Singer
- Reassess: Take a step back. Look at the problem from another perspective. Is there anything that can be abstracted to a more general approach?
“Sometimes we get so lost in the details of a problem that we overlook general principles that would solve the problem at a more general level. […] The classic example of this, of course, is the summation of a long list of consecutive integers, 1 + 2 + 3 + … + n, which a very young Gauss quickly recognized was simply n(n+1)/2, thus avoiding the effort of having to do the addition.” — C. Jordan Ball
Sidenote: Another way of reassessing is starting anew. Delete everything and begin again with fresh eyes. I’m serious. You’ll be dumbfounded at how effective this is.
- Research: Ahh, good ol’ Google. You read that right. No matter what problem you have, someone has probably solved it. Find that person/ solution. In fact, do this even if you solved the problem! (You can learn a lot from other people’s solutions).
Caveat: Don’t look for a solution to the big problem. Only look for solutions to sub-problems. Why? Because unless you struggle (even a little bit), you won’t learn anything. If you don’t learn anything, you wasted your time.
Don’t expect to be great after just one week. If you want to be a good problem-solver, solve a lot of problems!
Practice. Practice. Practice. It’ll only be a matter of time before you recognize that “this problem could easily be solved with .”
How to practice? There are options out the wazoo!
Chess puzzles, math problems, Sudoku, Go, Monopoly, video-games, cryptokitties, bla… bla… bla….
In fact, a common pattern amongst successful people is their habit of practicing “micro problem-solving.” For example, Peter Thiel plays chess, and Elon Musk plays video-games.
“Byron Reeves said ‘If you want to see what business leadership may look like in three to five years, look at what’s happening in online games.’ Fast-forward to today. Elon [Musk], Reid [Hoffman], Mark Zuckerberg and many others say that games have been foundational to their success in building their companies.” — Mary Meeker ( 2017 internet trends report )
Does this mean you should just play video-games? Not at all.
But what are video-games all about? That’s right, problem-solving!
So, what you should do is find an outlet to practice. Something that allows you to solve many micro-problems (ideally, something you enjoy).
For example, I enjoy coding challenges. Every day, I try to solve at least one challenge (usually on Coderbyte ).
Like I said, all problems share similar patterns.
That’s all folks!
Now, you know better what it means to “think like a programmer.”
You also know that problem-solving is an incredible skill to cultivate (the meta-skill).
As if that wasn’t enough, notice how you also know what to do to practice your problem-solving skills!
Phew… Pretty cool right?
Finally, I wish you encounter many problems.
You read that right. At least now you know how to solve them! (also, you’ll learn that with every solution, you improve).
“Just when you think you’ve successfully navigated one obstacle, another emerges. But that’s what keeps life interesting.[…] Life is a process of breaking through these impediments — a series of fortified lines that we must break through. Each time, you’ll learn something. Each time, you’ll develop strength, wisdom, and perspective. Each time, a little more of the competition falls away. Until all that is left is you: the best version of you.” — Ryan Holiday ( The Obstacle is the Way )
Now, go solve some problems!
And best of luck ?
Special thanks to C. Jordan Ball and V. Anton Spraul . All the good advice here came from them.
Thanks for reading! If you enjoyed it, test how many times can you hit in 5 seconds. It’s great cardio for your fingers AND will help other people see the story.
Learn to code. Build projects. Earn certifications—All for free.
If you read this far, thank the author to show them you care. Say Thanks
Learn to code for free. freeCodeCamp's open source curriculum has helped more than 40,000 people get jobs as developers. Get started
What Is Problem Solving? How Software Engineers Approach Complex Challenges
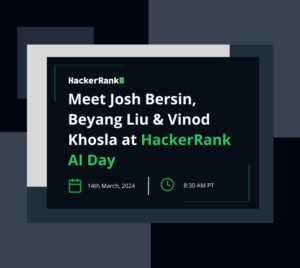
From debugging an existing system to designing an entirely new software application, a day in the life of a software engineer is filled with various challenges and complexities. The one skill that glues these disparate tasks together and makes them manageable? Problem solving .
Throughout this blog post, we’ll explore why problem-solving skills are so critical for software engineers, delve into the techniques they use to address complex challenges, and discuss how hiring managers can identify these skills during the hiring process.
What Is Problem Solving?
But what exactly is problem solving in the context of software engineering? How does it work, and why is it so important?
Problem solving, in the simplest terms, is the process of identifying a problem, analyzing it, and finding the most effective solution to overcome it. For software engineers, this process is deeply embedded in their daily workflow. It could be something as simple as figuring out why a piece of code isn’t working as expected, or something as complex as designing the architecture for a new software system.
In a world where technology is evolving at a blistering pace, the complexity and volume of problems that software engineers face are also growing. As such, the ability to tackle these issues head-on and find innovative solutions is not only a handy skill — it’s a necessity.
The Importance of Problem-Solving Skills for Software Engineers
Problem-solving isn’t just another ability that software engineers pull out of their toolkits when they encounter a bug or a system failure. It’s a constant, ongoing process that’s intrinsic to every aspect of their work. Let’s break down why this skill is so critical.
Driving Development Forward
Without problem solving, software development would hit a standstill. Every new feature, every optimization, and every bug fix is a problem that needs solving. Whether it’s a performance issue that needs diagnosing or a user interface that needs improving, the capacity to tackle and solve these problems is what keeps the wheels of development turning.
It’s estimated that 60% of software development lifecycle costs are related to maintenance tasks, including debugging and problem solving. This highlights how pivotal this skill is to the everyday functioning and advancement of software systems.
Innovation and Optimization
The importance of problem solving isn’t confined to reactive scenarios; it also plays a major role in proactive, innovative initiatives . Software engineers often need to think outside the box to come up with creative solutions, whether it’s optimizing an algorithm to run faster or designing a new feature to meet customer needs. These are all forms of problem solving.
Consider the development of the modern smartphone. It wasn’t born out of a pre-existing issue but was a solution to a problem people didn’t realize they had — a device that combined communication, entertainment, and productivity into one handheld tool.
Increasing Efficiency and Productivity
Good problem-solving skills can save a lot of time and resources. Effective problem-solvers are adept at dissecting an issue to understand its root cause, thus reducing the time spent on trial and error. This efficiency means projects move faster, releases happen sooner, and businesses stay ahead of their competition.
Improving Software Quality
Problem solving also plays a significant role in enhancing the quality of the end product. By tackling the root causes of bugs and system failures, software engineers can deliver reliable, high-performing software. This is critical because, according to the Consortium for Information and Software Quality, poor quality software in the U.S. in 2022 cost at least $2.41 trillion in operational issues, wasted developer time, and other related problems.
Problem-Solving Techniques in Software Engineering
So how do software engineers go about tackling these complex challenges? Let’s explore some of the key problem-solving techniques, theories, and processes they commonly use.
Decomposition
Breaking down a problem into smaller, manageable parts is one of the first steps in the problem-solving process. It’s like dealing with a complicated puzzle. You don’t try to solve it all at once. Instead, you separate the pieces, group them based on similarities, and then start working on the smaller sets. This method allows software engineers to handle complex issues without being overwhelmed and makes it easier to identify where things might be going wrong.
Abstraction
In the realm of software engineering, abstraction means focusing on the necessary information only and ignoring irrelevant details. It is a way of simplifying complex systems to make them easier to understand and manage. For instance, a software engineer might ignore the details of how a database works to focus on the information it holds and how to retrieve or modify that information.
Algorithmic Thinking
At its core, software engineering is about creating algorithms — step-by-step procedures to solve a problem or accomplish a goal. Algorithmic thinking involves conceiving and expressing these procedures clearly and accurately and viewing every problem through an algorithmic lens. A well-designed algorithm not only solves the problem at hand but also does so efficiently, saving computational resources.
Parallel Thinking
Parallel thinking is a structured process where team members think in the same direction at the same time, allowing for more organized discussion and collaboration. It’s an approach popularized by Edward de Bono with the “ Six Thinking Hats ” technique, where each “hat” represents a different style of thinking.
In the context of software engineering, parallel thinking can be highly effective for problem solving. For instance, when dealing with a complex issue, the team can use the “White Hat” to focus solely on the data and facts about the problem, then the “Black Hat” to consider potential problems with a proposed solution, and so on. This structured approach can lead to more comprehensive analysis and more effective solutions, and it ensures that everyone’s perspectives are considered.
This is the process of identifying and fixing errors in code . Debugging involves carefully reviewing the code, reproducing and analyzing the error, and then making necessary modifications to rectify the problem. It’s a key part of maintaining and improving software quality.
Testing and Validation
Testing is an essential part of problem solving in software engineering. Engineers use a variety of tests to verify that their code works as expected and to uncover any potential issues. These range from unit tests that check individual components of the code to integration tests that ensure the pieces work well together. Validation, on the other hand, ensures that the solution not only works but also fulfills the intended requirements and objectives.
Explore verified tech roles & skills.
The definitive directory of tech roles, backed by machine learning and skills intelligence.
Explore all roles
Evaluating Problem-Solving Skills
We’ve examined the importance of problem-solving in the work of a software engineer and explored various techniques software engineers employ to approach complex challenges. Now, let’s delve into how hiring teams can identify and evaluate problem-solving skills during the hiring process.
Recognizing Problem-Solving Skills in Candidates
How can you tell if a candidate is a good problem solver? Look for these indicators:
- Previous Experience: A history of dealing with complex, challenging projects is often a good sign. Ask the candidate to discuss a difficult problem they faced in a previous role and how they solved it.
- Problem-Solving Questions: During interviews, pose hypothetical scenarios or present real problems your company has faced. Ask candidates to explain how they would tackle these issues. You’re not just looking for a correct solution but the thought process that led them there.
- Technical Tests: Coding challenges and other technical tests can provide insight into a candidate’s problem-solving abilities. Consider leveraging a platform for assessing these skills in a realistic, job-related context.
Assessing Problem-Solving Skills
Once you’ve identified potential problem solvers, here are a few ways you can assess their skills:
- Solution Effectiveness: Did the candidate solve the problem? How efficient and effective is their solution?
- Approach and Process: Go beyond whether or not they solved the problem and examine how they arrived at their solution. Did they break the problem down into manageable parts? Did they consider different perspectives and possibilities?
- Communication: A good problem solver can explain their thought process clearly. Can the candidate effectively communicate how they arrived at their solution and why they chose it?
- Adaptability: Problem-solving often involves a degree of trial and error. How does the candidate handle roadblocks? Do they adapt their approach based on new information or feedback?
Hiring managers play a crucial role in identifying and fostering problem-solving skills within their teams. By focusing on these abilities during the hiring process, companies can build teams that are more capable, innovative, and resilient.
Key Takeaways
As you can see, problem solving plays a pivotal role in software engineering. Far from being an occasional requirement, it is the lifeblood that drives development forward, catalyzes innovation, and delivers of quality software.
By leveraging problem-solving techniques, software engineers employ a powerful suite of strategies to overcome complex challenges. But mastering these techniques isn’t simple feat. It requires a learning mindset, regular practice, collaboration, reflective thinking, resilience, and a commitment to staying updated with industry trends.
For hiring managers and team leads, recognizing these skills and fostering a culture that values and nurtures problem solving is key. It’s this emphasis on problem solving that can differentiate an average team from a high-performing one and an ordinary product from an industry-leading one.
At the end of the day, software engineering is fundamentally about solving problems — problems that matter to businesses, to users, and to the wider society. And it’s the proficient problem solvers who stand at the forefront of this dynamic field, turning challenges into opportunities, and ideas into reality.
This article was written with the help of AI. Can you tell which parts?
Get started with HackerRank
Over 2,500 companies and 40% of developers worldwide use HackerRank to hire tech talent and sharpen their skills.
- For Individuals
- For Businesses
- For Universities
- For Governments
- Online Degrees
- Find your New Career
- Join for Free

Problem Solving Using Computational Thinking
Financial aid available
86,140 already enrolled

Gain insight into a topic and learn the fundamentals

Instructor: Chris Quintana
(1,235 reviews)
What you'll learn
Recognize Computational Thinking concepts in practice through a series of real-world case examples.
Develop solutions through the application of Computational Thinking concepts to real world problems.
Skills you'll gain
- Computer Programming
- Computational Thinking
Details to know

Add to your LinkedIn profile
See how employees at top companies are mastering in-demand skills
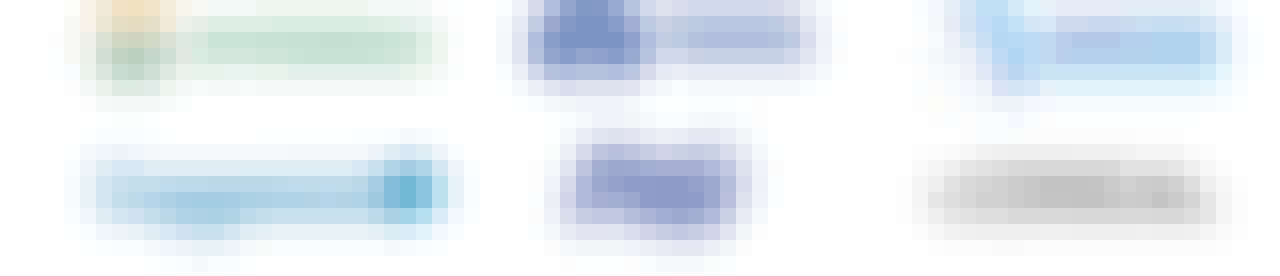
Earn a career certificate
Add this credential to your LinkedIn profile, resume, or CV
Share it on social media and in your performance review
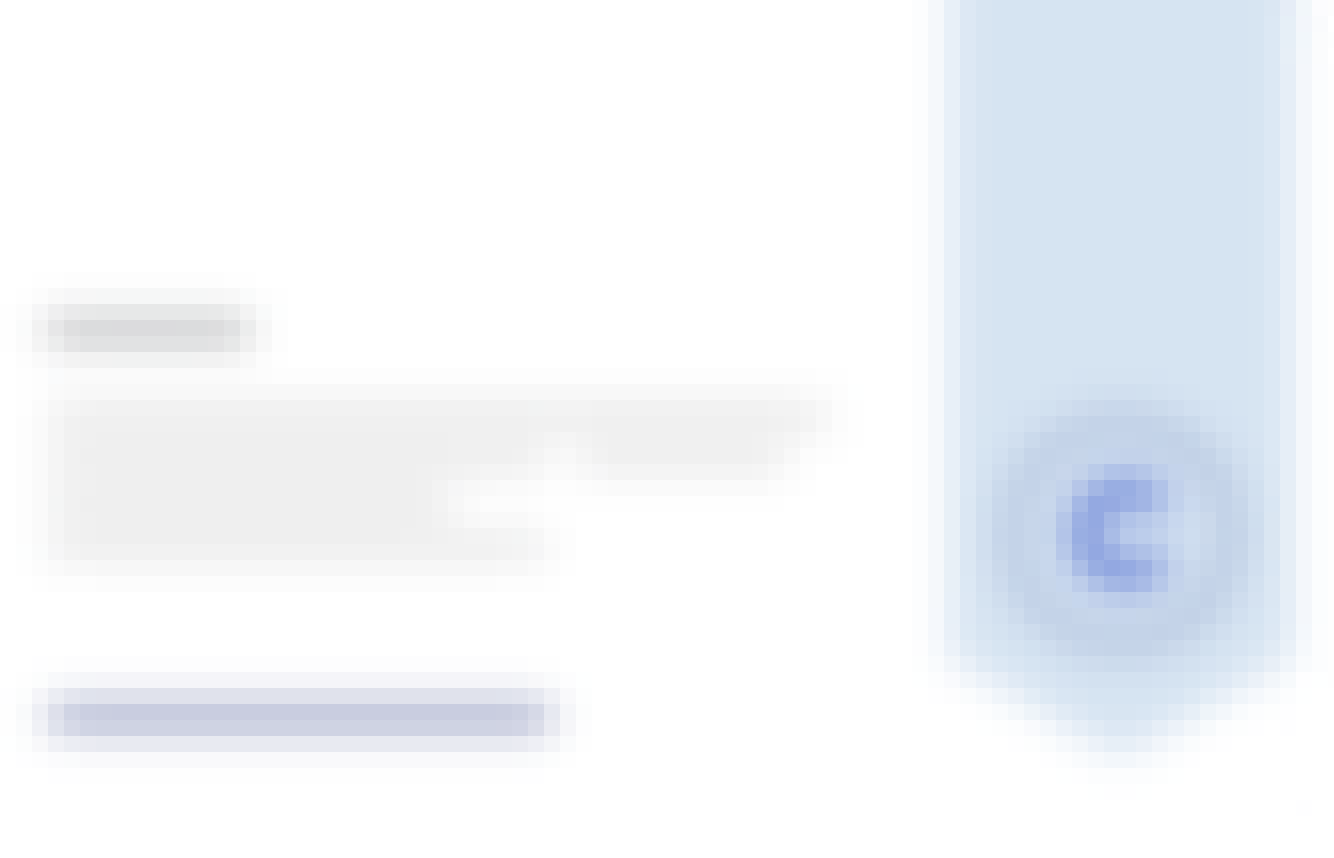
There are 5 modules in this course
Have you ever heard that computers "think"? Believe it or not, computers really do not think. Instead, they do exactly what we tell them to do. Programming is, "telling the computer what to do and how to do it."
Before you can think about programming a computer, you need to work out exactly what it is you want to tell the computer to do. Thinking through problems this way is Computational Thinking. Computational Thinking allows us to take complex problems, understand what the problem is, and develop solutions. We can present these solutions in a way that both computers and people can understand. The course includes an introduction to computational thinking and a broad definition of each concept, a series of real-world cases that illustrate how computational thinking can be used to solve complex problems, and a student project that asks you to apply what they are learning about Computational Thinking in a real-world situation. This project will be completed in stages (and milestones) and will also include a final disaster response plan you'll share with other learners like you. This course is designed for anyone who is just beginning programming, is thinking about programming or simply wants to understand a new way of thinking about problems critically. No prior programming is needed. The examples in this course may feel particularly relevant to a High School audience and were designed to be understandable by anyone. You will learn: -To define Computational Thinking components including abstraction, problem identification, decomposition, pattern recognition, algorithms, and evaluating solutions -To recognize Computational Thinking concepts in practice through a series of real-world case examples -To develop solutions through the application of Computational Thinking concepts to real world problems
Foundations of Computational Thinking
What's included.
3 videos 5 readings 2 quizzes 1 discussion prompt
3 videos • Total 43 minutes
- Welcome to Computational Thinking • 15 minutes • Preview module
- Example: Making a Cake • 16 minutes
- Introduction to the Graphic Organizer • 11 minutes
5 readings • Total 50 minutes
- Welcome and Syllabus • 10 minutes
- Help Us Learn More about You! • 10 minutes
- Contributor Acknowledgements • 10 minutes
- Introduction to the Graphic Organizer • 10 minutes
- Would you like to plan your learning journey with Michigan Online? • 10 minutes
2 quizzes • Total 35 minutes
- Foundations of Computational Thinking Quiz • 25 minutes
- Foundations of Computational Thinking Practice Questions • 10 minutes
1 discussion prompt • Total 10 minutes
- Real-World Applications of Computational Thinking • 10 minutes
Case Study: Airport Surveillance and Image Analysis
6 videos 3 readings 3 quizzes 2 discussion prompts
6 videos • Total 29 minutes
- Image Analysis: Importance of Computational Thinking - Part 1 • 2 minutes • Preview module
- Image Analysis: Importance of Computational Thinking - Part 2 • 1 minute
- Image Analysis: Abstraction and Algorithms • 10 minutes
- Image Analysis: Algorithms, Optional Advanced Video • 9 minutes
- Image Analysis: Evaluating Solutions • 4 minutes
- Image Analysis: Problem Identification and Decomposition • 0 minutes
3 readings • Total 30 minutes
- Introduction to Airport Surveillance Case-Study • 10 minutes
- Airport Surveillance Case-Study Check-In 1 • 10 minutes
- Airport Surveillance Check-In 2 • 10 minutes
3 quizzes • Total 55 minutes
- Airport Surveillance Case-Study Quiz • 20 minutes
- Airport Surveillance Practice Questions Set 1 • 15 minutes
- Airport Surveillance Practice Questions Set 2 • 20 minutes
2 discussion prompts • Total 20 minutes
- Image Analysis: What Would You Do? • 10 minutes
- Other Applications • 10 minutes
Case Study: Epidemiology
6 videos 5 readings 2 quizzes 2 discussion prompts
6 videos • Total 52 minutes
- Epidemiology: Introduction and Problem Identification • 1 minute • Preview module
- Epidemiology: Problem Identification Part 2 • 8 minutes
- Epidemiology: Abstraction and Decomposition • 14 minutes
- Epidemiology: Algorithms and Evaluating Solutions - Part 1 • 13 minutes
- Epidemiology: Algorithms and Evaluating Solutions - Part 2 • 8 minutes
- Epidemiology: Conclusion • 6 minutes
- Introduction to Epidemiology Case-Study • 10 minutes
- Epidemiology Case-Study Check-In 1 • 10 minutes
- Up Next: Rafael's Algorithm • 10 minutes
- Epidemiology Case-Study Check-In 2 • 10 minutes
- Stay in touch on University of Michigan online courses • 10 minutes
2 quizzes • Total 36 minutes
- Epidemiology Case-Study Quiz • 20 minutes
- Epidemiology Practice Questions • 16 minutes
- Using Computational Thinking in Public Health • 10 minutes
- Understanding the Problem • 10 minutes
Case Study: Human Trafficking
3 videos 2 readings 2 quizzes
3 videos • Total 34 minutes
- Human Trafficking: Importance of Computational Thinking • 10 minutes • Preview module
- Human Trafficking: How Computational Thinking May Apply - Part 1 • 11 minutes
- Human Trafficking: How Computational Thinking May Apply - Part 2 • 12 minutes
2 readings • Total 20 minutes
- Introduction to Human Trafficking Case-Study • 10 minutes
- Human Trafficking Case-Study Check-In • 10 minutes
2 quizzes • Total 50 minutes
- Next Case: Potential Applications of Computational Thinking to Human Trafficking • 30 minutes
- Human Trafficking Practice Questions • 20 minutes
Final Project
8 readings 1 peer review
8 readings • Total 80 minutes
- Introduction to the Final Project • 10 minutes
- Final Project Part 1. Background and Context • 10 minutes
- Final Project Part 2: Graphic Organizer and Project Justification • 10 minutes
- Final Project Part 3: Project Justification • 10 minutes
- Final Project Part 4: Algorithm depiction • 10 minutes
- Course Feedback • 10 minutes
- Create innovative learning environments for students with Introduction to Learning Experience Design • 10 minutes
- Keep Learning with Michigan Online • 10 minutes
1 peer review • Total 60 minutes
- Final Project • 60 minutes
Instructor ratings
We asked all learners to give feedback on our instructors based on the quality of their teaching style.

The mission of the University of Michigan is to serve the people of Michigan and the world through preeminence in creating, communicating, preserving and applying knowledge, art, and academic values, and in developing leaders and citizens who will challenge the present and enrich the future.
Recommended if you're interested in Algorithms
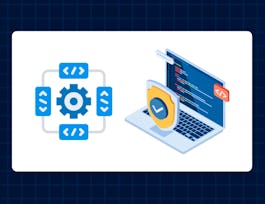
Board Infinity
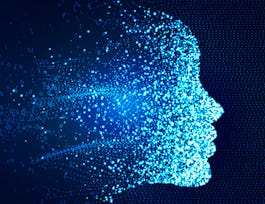
University of Pennsylvania
Computational Thinking for Problem Solving
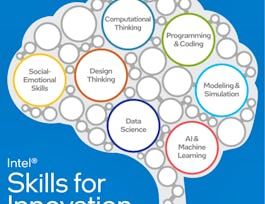
Establishing Effective Educator-Machine Partnerships
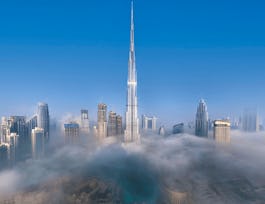
The University of Edinburgh
Climate Solutions: UAE
Why people choose coursera for their career.

Learner reviews
Showing 3 of 1235
1,235 reviews
Reviewed on May 27, 2021
The course helped me develop problem thinking skills and I appreciate the real life examples used in teaching the course. They made understanding the concepts much easier.
Reviewed on Jul 27, 2021
This course is what I really need to understand what is Computational Thinking. I learned about all aspect of it. To who want to begin your road to Computer Science, this course is my recommend
Reviewed on May 30, 2021
The course is highly enlightening. It has helped me see that a lot of problems can be solved using computational thinking. I will recommend to anyone willing to gain knowledge in this area.
New to Algorithms? Start here.

Open new doors with Coursera Plus
Unlimited access to 7,000+ world-class courses, hands-on projects, and job-ready certificate programs - all included in your subscription
Advance your career with an online degree
Earn a degree from world-class universities - 100% online
Join over 3,400 global companies that choose Coursera for Business
Upskill your employees to excel in the digital economy
Frequently asked questions
When will i have access to the lectures and assignments.
Access to lectures and assignments depends on your type of enrollment. If you take a course in audit mode, you will be able to see most course materials for free. To access graded assignments and to earn a Certificate, you will need to purchase the Certificate experience, during or after your audit. If you don't see the audit option:
The course may not offer an audit option. You can try a Free Trial instead, or apply for Financial Aid.
The course may offer 'Full Course, No Certificate' instead. This option lets you see all course materials, submit required assessments, and get a final grade. This also means that you will not be able to purchase a Certificate experience.
What will I get if I purchase the Certificate?
When you purchase a Certificate you get access to all course materials, including graded assignments. Upon completing the course, your electronic Certificate will be added to your Accomplishments page - from there, you can print your Certificate or add it to your LinkedIn profile. If you only want to read and view the course content, you can audit the course for free.
What is the refund policy?
You will be eligible for a full refund until two weeks after your payment date, or (for courses that have just launched) until two weeks after the first session of the course begins, whichever is later. You cannot receive a refund once you’ve earned a Course Certificate, even if you complete the course within the two-week refund period. See our full refund policy Opens in a new tab .
Is financial aid available?
Yes. In select learning programs, you can apply for financial aid or a scholarship if you can’t afford the enrollment fee. If fin aid or scholarship is available for your learning program selection, you’ll find a link to apply on the description page.
More questions
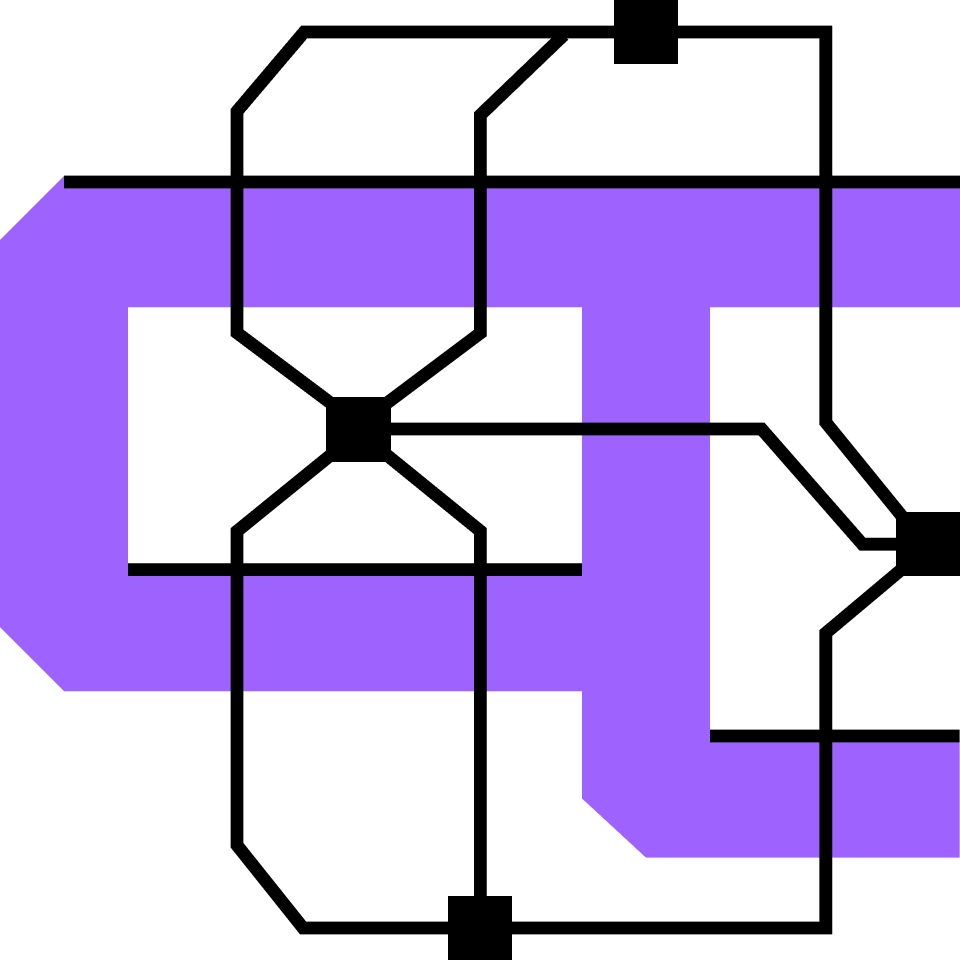
3.2 Computer Science Fundamentals
Wrap your mind around computational thinking, from everyday tasks to algorithms.
Making Decisions
Computers use decision trees to turn many simple decisions into one big decision.
Searching for Solutions
Sometimes, the right way to solve a computational problem is by “brute force.”
- Parallelism
When Pierre the baker wants to get lots of things done, it helps to do many things at once.
End of Unit 1
Complete all lessons above to reach this milestone.
0 of 3 lessons complete
Resource Tradeoffs
Computer scientists deal with tradeoffs all the time. So does Farhad when he does his chores.
Order and Search
Information needs to be organized for use by humans or computers, as Tiye the librarian knows well.
Computer systems and people need to be able to reliably find and access people and resources.
Abstraction
Mayor Jing uses abstraction—a critical tool in computer science—to help her run City Hall.
Abstractions have interfaces that explain what they can and cannot do.
End of Unit 2
0 of 5 lessons complete
Algorithms and Implementations
Algorithms are step-by-step processes for achieving an outcome. They can be very specific or quite general.
Divide and Conquer
Problems often get easier when you split them in half, as the 20 Questions guessing game shows.
- Binary Search
Binary search is a more algorithm-friendly version of the 20 Questions game.
Thinking with Graphs
Graphs are a powerful tool for understanding problems and solving them in clever ways.
Representing Games and Puzzles
Graphs can help us plan solutions to complex problems, like this classic river-crossing puzzle.
Graph Search
Some of the most fundamental algorithms on graphs are designed to get you from point A to point B.
End of Unit 3
0 of 6 lessons complete
Course description
Learn the key ideas of computer science with this interactive course – no coding required! This course is ideal for a high school or college student who wants to learn the fundamentals, or an early professional who wants to strengthen their knowledge of core computer science concepts. Whether you're exploring computer science for the first time or looking to deepen your understanding, this course will allow you to develop the problem-solving techniques you need to think like a computer scientist. Follow librarians, cooks, and mayors to see how computer science problem solving techniques affect their daily lives. Get hands-on with a few specific algorithms, and learn the general principles demonstrated by these algorithms.
Topics covered
- Brute-Force Search
- Concurrency
- Decision Trees
- Graph Abstractions
- Greedy Algorithms
- Programming
Prerequisites and next steps
You don’t need any previous computer science experience to take this course! This course is for anyone excited to actively learn more about how computer scientists think and understand our world.
4.1 Applied Python
Expand your Python skills by leveraging (or using) strings and dictionaries to analyze and generate text.
Browse Course Material
Course info.
- Prof. John Guttag
Departments
- Electrical Engineering and Computer Science
As Taught In
- Computer Science
Introduction to Computer Science and Programming
Lecture 3: problem solving.
- Download video
- Download transcript

You are leaving MIT OpenCourseWare

Member-only story
Hands-on Tutorial: How To Improve Your Problem-Solving Skills As A Programmer
The technical part is the easiest.

Kurtis Pykes
Towards Data Science
Programming is ultimately problem-solving. We only apply the programming language to express how we’ve thought about a problem and the approach we’re using to solve it.
The worst thing you could do is to start chipping away at the problem once it’s presented. This is where most newbie programmers get stuck and give up. Think back to school days when you had to do written assessments. Usually, the paper [or invigilator] would tell you how much time you have for planning and how much should be set aside for writing. It’s the same thing with programming.
Every problem is a coat for a series of smaller problems that have to be tackled. The technical part is always the easiest once you’ve clearly devised a clear plan of attack for how to solve the problem.
“ If I only had an hour to chop down a tree, I would spend the first 45 minutes sharpening my axe. ” — Abraham Lincoln.
To demonstrate this we’re going to work through a coding problem together. Coding problems are a good way to develop your ability to think through programs. The more exposure you get to…
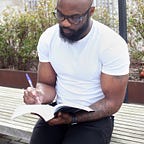
Written by Kurtis Pykes
SaaS Content Writer | Sharing everything I learn as I build my solo business to $10M in revenue
Text to speech
Problem Solving
Solving problems is the core of computer science. Programmers must first understand how a human solves a problem, then understand how to translate this "algorithm" into something a computer can do, and finally how to "write" the specific syntax (required by a computer) to get the job done. It is sometimes the case that a machine will solve a problem in a completely different way than a human.
Computer Programmers are problem solvers. In order to solve a problem on a computer you must:
Know how to represent the information (data) describing the problem.
Determine the steps to transform the information from one representation into another.
Information Representation
A computer, at heart, is really dumb. It can only really know about a few things... numbers, characters, booleans, and lists (called arrays) of these items. (See Data Types). Everything else must be "approximated" by combinations of these data types.
A good programmer will "encode" all the "facts" necessary to represent a problem in variables (See Variables). Further, there are "good ways" and "bad ways" to encode information. Good ways allow the computer to easily "compute" new information.
An algorithm (see Algorithm) is a set of specific steps to solve a problem. Think of it this way: if you were to tell your 3 year old neice to play your favorite song on the piano (assuming the neice has never played a piano), you would have to tell her where the piano was, and how to sit on the bench, and how to open the cover, and which keys to press, and which order to press them in, etc, etc, etc.
The core of what good programmers do is being able to define the steps necessary to accomplish a goal. Unfortunately, a computer, only knows a very restricted and limited set of possible steps. For example a computer can add two numbers. But if you want to find the average of two numbers, this is beyond the basic capabilities of a computer. To find the average, you must:
- First: Add the two numbers and save this result in a variable
- Then: Divide this new number the number two, and save this result in a variable.
- Finally: provide this number to the rest of the program (or print it for the user).
We "compute" all the time. Computing is the act of solving problems (or coming up with a plan to solve problems) in an organized manner. We don't need computers to "compute". We can use our own brain.
Encapsulation and Abstraction and Complexity Hiding
Computer scientists like to use the fancy word "Encapsulation" to show how smart we are. This is just a term for things we do as humans every day. It is combined with another fancy term: "Abstraction".
Abstraction is the idea of "ignoring the details". For example, a forest is really a vastly complex ecosystem containing trees, animals, water paths, etc, etc, etc. But to a computer scientist (and to a normal person), its just "a forest".
For example, if your professor needs a cup of coffee, and asks you the single item: "Get me a cup of coffee", he has used both encapsulation and abstraction. The number of steps required to actually get the coffee are enumerable. Including, getting up, walking down the hall, getting in your car, driving to a coffee stand, paying for the coffee, etc, etc, etc. Further, the idea of what a cup of coffee is, is abstract. Do you bring a mug of coffee, or a Styrofoam cup? Is it caffeinated or not? Is it freshly brewed or from concentrate? Does it come from Africa or America?
All of this information is TOO MUCH and we would quickly be unable to funciton if we had to remember all of these details. Thus we "abstract away" the details and only remember the few important items.
This brings us to the idea of "Complexity Hiding". Complexity hiding is the idea that most of the times details don't matter. In a computer program, as simple an idea as drawing a square on the screen involves hundreds (if not thousands) of (low level) computer instructions. Again, a person couldn't possible create interesting programs if every time they wanted to do something, they had to re-write (correctly) every one of those instructions. By "ecapsulating" what is meant by "draw square" and "reusing" this operation over and over again, we make programming tractable.
Encapsulation
The idea behind encapsulation is to store the information necessary to a particular idea in a set of variables associated with a single "object". We then create functions to manipulate this object, regardless of what the actual data is. From that point on, we treat the idea from a "high level" rather than worry about all the parts (data) and actions (functions) necessary to represent the object in a computer.
Brute Force
Brute force is a technique for solving problems that relies on a computers speed (how fast it can repeat steps) to solve a problem. For example, if you wanted to know how many times the number 8 goes into the number 100, you could do the following:
Of course this is a silly way for a computer (or a human) to solve this problem. The real way we would do it is:
When in doubt, you can often use "brute force" to solve a problem, but it often saves time (at least computer time) to think about the problem and solve it in an elegant manner.
Oberlin College Computer Science
- Course Websites
- CS Lab Helper Schedule
Problem Solving Tips
- Course Catalog
- Major/Minor in CS
- Electives Schedule
- Intro Course Placement
- Academic Calendar
- Department Honors
- Bob Geitz (chair)
- Stephen Checkoway
- Roberto Hoyle
- Dylan McKay
- Sam Taggart
- Cynthia Taylor
- Blair Rossetti
- Molly Feldman
- 2013-Wearable-Electronics
- 2011 ACM Programming Contest
- 2013 Alexa's Tenure Celebration
- 2013 Pledge Ceremony
- 2012 Games Showcase
- 2011 CSMC Unix Night
- MCURCSM 2009
- 2009 Games Showcase
- OCCS 25th Anniversary
- 2010 Spring Social
- 2009 Spring Picnic
- 2009 Math/CS Ice Cream Social
- 2008 Spring Picnic
- 2010 Denison Programming Contest
- 2008 Math/CS Sundae Friday
- 2009 ACM Programming Contest
- 2009 Denison Programming Contest
- 2008 ACM Programming Contest
- 2008 Denison Programming Contest
- 2007 ACM Programming Contest
- 2006 ACM Programming Contest
- Oberlin College
One of the most important skills you learn in your computer science courses is how to problem solve. Although we cover some general problem solving paradigms in class, the best way to improve these skills is to get practice, practice, and more practice. Different people have different techniques that work best for them; below are some general tips that work for most people.
Please read these suggestions carefully.
Questions the Helpers May Ask You
When you ask a lab helper for their assistance, they will assume you have tried to solve the problem yourself. They will (reasonably) expect that you have tried out the steps outlined in this document; you should therefore be prepared to answer the following questions:
- Did you re-read the prelab and lab?
- Do you understand the problem?
- Have you tried solving some examples by hand?
- (For problems designing a solution) What have you tried? What topic from class does this most ressemble?
- If you can’t solve the problem whole-hog, what small case can you solve?
- (For syntax errors) What line of your code is causing the error? What do you think the compile error means, and what usually causes this kind of problem?
- (For logical errors) On what example does your program consistently break? Have you traced through the program? Which line of your program is not doing what it should?
Four Main Problem Solving Steps:
1. understand the problem..
Solving the right problem is the most important part of problem solving. Be sure, absolutely 100% positively sure, that you understand the problem before attempting a solution. This involves:
- Reading the prelab and lab very carefully (including all bold text, italicized text, and everything else);
- Reviewing class notes on related topics;
- Trying some small examples to make sure you understand what is being asked; if examples are given to you, make sure you understand them before continuing, as they are usually there to help clarify some common misconceptions; and
- Asking someone to help clarify anything that is still confusing.
2. Design a Solution.
Formulate an algorithm to solve your problem. This involves:
- Understanding what is being asked of you. See step 1.
- Draw out some examples. Use paper . How would you solve these small cases, by hand? Is there a method to what you are doing? Try to formalize the steps you are taking, and try to think about whether they would work more generally, in bigger cases. Then try some bigger cases and convince yourself.
- Reread the prelab . Did you already run some examples by hand? Did you have trouble with it then?
- Write down the stuff you know about the problem and the examples you’ve tried, so that you can more easily find patterns .
- Might a recent topic from class help? Usually at least some, if not most, of the lab will make use of recently covered material . Go over that topic, make sure you understand it, then try to make connections to lab.
- Split the problem into smaller (more manageable) chunks, and try to solve the simpler problems. Go as small as you need in order to find some solution. Once you have the smaller problem solved, worry about how to generalize it to a slightly larger problem.
- Just try something , anything, even if it is completely random and obviously wrong. When/if your attempt doesn’t work, it may still give you insight into what may work. It is not as crazy as it initially sounds!
- Use a friend, lab helper, puppet, etc. as a sounding board ; sometimes, just voicing your problem will lead you to the “aha!” moment you need.
- If you are still stuck, step away from the keyboard . Take a walk, go eat dinner or have a coffee. Sleep on it. Not literally. Taking a break is sometimes the most productive thing you can do, trust me.
- Finally, stay positive . Even when things don’t work, you can still gain a better understanding of the problem. Don’t give up, just go with the flow and see where it takes you. Struggling is part of the process!
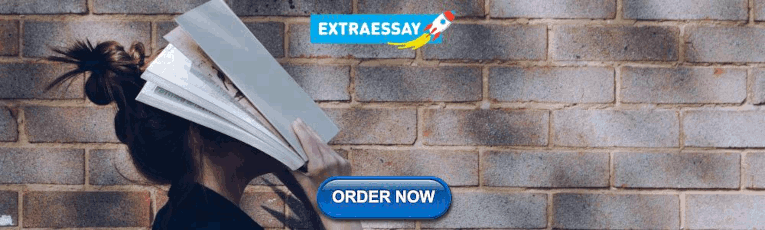
3. Implement your Solution.
Write the code to solve your problem. This involves
- Understanding the problem, and designing a solution on paper. See steps 1 and 2.
- Translating your design into actual code. Rather than doing this linearly, implement small chunks at a time. Break your code into subroutines, and make sure that each subroutine works before proceeding to the next. Compile and save often .
- If you run into syntax errors, determine which line of your code is causing the problem. You can do this by systematically commenting out blocks of code until you find the block that causes the problem.
- If you run into logical errors (as in, the program compiles but does not do what it is supposed to), find some examples on which your problem consistently fails. Trace through the program line by line, with one of these examples, to figure out exactly which line is not doing what you intend it to.
- If the output doesn’t match what you expect, use print statements to trace through what your program is doing, and compare that to what your program should be doing. Even better, if you know how to use a debugger (in eclipse, for example, use it!)
4. Check your Solution.
This step is often overlooked, but is absolutely crucial. Your program does not necessarily work because it works on the given test cases on the lab. You have to think critically about what you code. This involves
- Certainly check your program on all test cases given to you on the lab and prelab. The prelab often specifically contains hand-solved test cases precisely for this purpose!
- Thinking about the “ boundary cases ,” such as, when would this array go out of bounds? For what indices will this for loop start and end?
- Think: how would this program break ? Then, that failing: how would I convince my skeptical friend it can’t be broken?
Remember: problem solving is a creative process, which cannot be forced. Don’t get angry if you don’t see the answer right away, or you don’t see it as fast as your friend. You will have different strengths, and you can always improve. You will learn from your mistakes, so that’s always a plus!
Last updated July 3rd, 2012 by asharp
Recent Posts
- Congratulations Professor Stephen Checkoway; recipient of this prestigious award!
- Class of 2021 Celebration
- Undergraduate Research Symposium
- Grad School Information meeting
- Tech Resume Workshop Thursday April 1 4:45pm
- Information
- Jobs/Internships
- Uncategorized
- Association for Computing Machinery (ACM)
- National Center for Women & Information Technology (NCWIT)
- Computer Research Association (CRA)
OCCS Social Media
- Entries feed
- Comments feed
- WordPress.org
Oberlin College Computer Science Department -- 10 N. Professor St., Oberlin, OH 44074 -- Phone: (440) 775-8043
Copyright © 1985-2024 Oberlin College Computer Science. Design by Benjamin A. Kuperman . Powered by WordPress .
Problem-Solving Strategies
- First Online: 06 August 2020
Cite this chapter
- Orit Hazzan ORCID: orcid.org/0000-0002-8627-0997 4 ,
- Noa Ragonis ORCID: orcid.org/0000-0002-8163-0199 5 &
- Tami Lapidot 4
1494 Accesses
Problem-solving is generally considered as one of the most important and challenging cognitive activities in everyday as well as in any professional contexts. Specifically, it is one of the central activities performed by computer scientists as well as by computer science learners. However, it is not a uniform or linear process that can be taught as an algorithm to be followed, and the understanding of this individual process is not always clear. Computer science learners often face difficulties in performing two of the main stages of a problem-solving process: problem analysis and solution construction. Therefore, it is important that computer science educators be aware of these difficulties and acquire appropriate pedagogical tools to guide and scaffold learners in learning these skills. This chapter is dedicated to such pedagogical tools. It presents several problem-solving strategies to address in the MTCS course together with appropriate activities that mediate them to the prospective computer science teachers by enabling them to experience the different strategies.
This is a preview of subscription content, log in via an institution to check access.
Access this chapter
Subscribe and save.
- Get 10 units per month
- Download Article/Chapter or eBook
- 1 Unit = 1 Article or 1 Chapter
- Cancel anytime
- Available as PDF
- Read on any device
- Instant download
- Own it forever
- Available as EPUB and PDF
- Compact, lightweight edition
- Dispatched in 3 to 5 business days
- Free shipping worldwide - see info
- Durable hardcover edition
Tax calculation will be finalised at checkout
Purchases are for personal use only
Institutional subscriptions
An algorithmic problem is defined by what is given – the initial conditions of the problem and its goals – the desired state, what should be accomplished. An algorithm problem can be solved with a series of actions formulated formally either by pseudo-code or a programming language. See Sect. 12.4.1 .
In advanced computer science classes, it is relevant to mention that in computer science, in addition to the development of problem-solving strategies, special emphasis is placed also on non-solvable problems (see Sect. 12.4.3 ).
Role of Variables in Python: http://www.cs.joensuu.fi/~saja/var_roles/stud_vers/stud_Python_eng.html
Roles of Variables with examples in Scratch: https://www.sisd.net/cms/lib/TX01001452/Centricity/domain/433/cse/1.1.5%20RolesOfVariables_UsedActivity1.2.4.pptx
The Roles of Variables home page ( http://saja.kapsi.fi/var_roles/ ) is a rich resource and contains different kinds of educational resources.
See http://www.cs.joensuu.fi/~saja/var_roles/role_intro.html
See http://www.cs.joensuu.fi/~saja/var_roles/try.html
See http://cs.uef.fi/~pgerdt/RAE/
See http://www.cs.joensuu.fi/~saja/var_roles/why_roles.html
See http://www.cs.joensuu.fi/~saja/var_roles/teaching.html
See http://saja.kapsi.fi/var_roles/literature.html
Ahrendt W, Bubel R, Hahnle R (2009) Integrated and tool-supported teaching of testing, debugging, and verification. In: Gibbons J, Oliveira JN (eds) Proceedings of the 2nd international conference on teaching formal methods (TFM ’09). Springer, Berlin/Heidelberg, pp 125–143
Google Scholar
Alqadi BS, Maletic JI (2017) An empirical study of debugging patterns among novices programmers. In: Proceedings of the 2017 ACM SIGCSE technical symposium on computer science education (SIGCSE ’17). ACM, New York, pp 15–20
Arshad N (2009) Teaching programming and problem solving to CS2 students using think-alouds. SIGCSE Bull 41(1):372–376
Astrachan O, Berry G, Cox L, Mitchener G (1998) Design patterns: an essential component of CS curricula. In: Proceeding of SIGCSE, pp 153–160
Batory D, Sarvela JN, Rauschmayer A (2004) Scaling stepwise refinement. IEEE Trans Softw Eng 30(6):355–371
Bauer A, Popović Z (2017) Collaborative problem solving in an open-ended scientific discovery game. In: Proceedings of the ACM Human-Computer Interaction 1, CSCW, Article 22 (December 2017)
Ben-Ari M, Sajaniemi J (2003) Roles of variables from the perspective of computer science educators. University of Joensuu, Department of Computer Science, Technical Report, Series A-2003–6
Börstler J, Hilburn TB (2016) Team projects in computing education. ACM Trans Comput Educ 16(2), Article 4 (March 2016)
Byckling P, Sajaniemi J (2006) Roles of variables and programming skills improvement. SIGCSE Bull 38(1):413–417
Carver S, McCoy (1988) Learning and transfer of debugging skills: applying task analysis to curriculum design and assessment. In Mayer RE (ed) Teaching and learning computer programming, multiple research perspectives. Lawrence Erlbaum Associates, Inc., Chapter 11
Celepkolu M, Boyer KE (2018) The importance of producing shared code through pair programming. In: Proceedings of the 49th ACM technical symposium on computer science education (SIGCSE ’18). ACM, New York, pp 765–770
Clancy MJ, Linn M C (1999) Patterns and pedagogy. In: Proceedings of the SIGCSE’99, pp 37–42
Cosmides L, Tooby J (1997) Evolutionary psychology: a primer. Retrieved 24 October 2004, from http://www.psych.ucsb.edu/research/cep/primer.html
Dijkstra EW (1976) A discipline of programming. Prentice-Hall, Englewood Cliffs
MATH Google Scholar
East JP, Thomas SR, Wallingford E, Beck W, Drake J (1996) Pattern-based programming instruction. In: Proceedings of ASEE annual conference and exposition, Washington, DC
Ginat D (2003) The greedy trap and learning from mistakes. SIGCSE Bull 35(1):11–15
Ginat D (2004) Algorithmic patterns and the case of the sliding delta. SIGCSE Bull 36(2):29–33
Ginat D (2008) Learning from wrong and creative algorithm design. SIGCSE Bull 40(1):26–30
Ginat D (2009) Interleaved pattern composition and scaffolded learning. In: Proceedings of the 14th Annual ACM SIGCSE Conference on Innovation and Technology in Computer Science Education – ITiCSE ‘09, Paris, France, pp 109–113
Ginat D, Shmalo R (2013) Constructive use of errors in teaching CS1. In: Proceedings of the 44th ACM technical symposium on Computer science education (SIGCSE ’13). ACM, New York, pp 353–358
Hasni TF, Lodhi F (2011) Teaching problem solving effectively. ACM Inroads 2(3):58–62
Hazzan O, Leron U (2006) Why do we resist testing? Syst Des Front Exclus Front Cover Syst Des 3(7):20–23
Johnson DW, Johanson RT (2017) Cooperative learning. Retrieved from: https://2017.congresoinnovacion.educa.aragon.es/documents/48/David_Johnson.pdf
Jonassen DH (2000) Toward a design theory of problem solving. Educ Technol Res Dev 48(4):63–85
Kiesmüller U (2009) Diagnosing learners’ problem-solving strategies using learning environments with algorithmic problems in secondary education. Trans Comput Educ 9(3), Article 17 (September 2009), 26 pages
Laakso MJ, Malmi L, Korhonen A, Rajala T, Kaila E, Salakoski T (2008) Using roles of variables to enhance novice’s debugging work. Issues Informing Sci Inf Technol 5:281–295
Lapidot T, Hazzan O (2005) Song debugging: merging content and pedagogy in computer science education. Inroads SIGCSE Bull 37(4):79–83
Lieberman H (1997) The debugging scandal and what to do about it (special section). Comm ACM 40(4):27–29
Lishinski A, Yadav A, Enbody R, Good J (2016) The influence of problem solving abilities on Students’ performance on different assessment tasks in CS1. In: Proceedings of the 47th ACM technical symposium on computing science education (SIGCSE ’16). ACM, New York, pp 329–334
Muller O (2005) Pattern oriented instruction and the enhancement of analogical reasoning. In: Proceedings of the first International Workshop on Computer Education Research ICER ‘05, Seattle, WA, USA, pp 57–67
Muller O, Haberman B, Averbuch H (2004) (An almost) pedagogical pattern for pattern-based problem solving instruction. In: Proceedings of the 9th Annual SIGCSE Conference on Innovation and Technology in Computer Science. Education, pp 102–106
Muller O, Ginat D, Haberman B (2007) Pattern-oriented instruction and its influence on problem decomposition and solution construction. ACM SIGCSE Bull 39(3):151–155
Murphy L, Lewandowski G, McCauley R, Simon B, Thomas L, Zander C (2008) Debugging: the good, the bad, and the quirky – a qualitative analysis of novices’ strategies. SIGCSE Bull 40(1):163–167
Nagvajara P, Taskin B (2007) Design-for-debug: a vital aspect in education. In: Proceedings of the 2007 IEEE international conference on microelectronic systems education (MSE ’07). IEEE Computer Society, Washington, DC, USA, pp 65–66
Papert S (1980) Mindstorms: children, computers and powerful ideas. Basic Books Inc, New York
Polya G (1957) How to solve it. Doubleday and Co., Inc, Garden City
Polya G (1981) Mathematical discovery on understanding learning and teaching problem solving. Wiley, New York
Popper KR (1992/1959) Logic of scientific discovery. Harper and Row, New York
Proulx VK (2000) Programming patterns and design patterns in the introductory computer science course. Proc SIGCSE 32(1):80–84
Ragonis N (2012) Integrating the teaching of algorithmic patterns into computer science teacher preparation programs. In: Proceedings of the 17th ACM annual conference on Innovation and technology in computer science education (ITiCSE ’12). ACM, New York, pp 339–344
Raman K, Svore KM, Gilad-Bachrach R, Burges CJC (2012) Learning from mistakes: towards a correctable learning algorithm. In: Proceedings of the 21st ACM international conference on information and knowledge management (CIKM ’12). ACM, New York, pp 1930–1934
Reed D (1999) Incorporating problem-solving patterns in CS1. J Comput Sci Edu 13(1):6–13
Reynolds RG, Maletic JI, Porvin SE (1992) Stepwise refinement and problem solving. IEEE Softw 9(5):79–88
Robins A, Rountree J, Rountree N (2003) Learning and teaching programming: a review and discussion. Comput Sci Edu 13(2):137–172
Sajaniemi J (2005) Roles of variables and learning to program. In: Jimoyiannis A (ed) Proceedings of the 3rd Panhellenic conference didactics of informatics, University of Peloponnese, Korinthos, Greece. http://cs.joensuu.fi/~saja/var_roles/abstracts/didinf05.pdf . Accessed 3 July 2010
Santos AL, Sousa J (2017) An exploratory study of how programming instructors illustrate variables and control flow. In: Proceedings of the 17th Koli calling international conference on computing education research (Koli Calling ’17). ACM, New York, pp 173–177
Schoenfeld AH (1983) Episodes and executive decisions in mathematical problem-solving. In: Lesh R, Landaue M (eds) Acquisition of mathematics concepts and processes. Academic Press Inc, New York
Schön DA (1983) The reflective practitioner. BasicBooks
Seta K, Kajino T, Umano M, Ikeda M (2006) An ontology based reflection support system to encourage learning from mistakes. In: Deved V (ed) Proceedings of the 24th IASTED international conference on artificial intelligence and applications (AIA’06). ACTA Press, Anaheim, pp 142–149
Soloway E (1986) Learning to program = learning to construct mechanisms and explanations. CACM 29(1):850–858
Spohrer JG, Soloway E (1986) Analyzing the high frequency bugs in novice programs. In: Soloway E, Iyengar S (eds) Empirical studies of programmers. Ablex, Norwood, pp 230–251
Stoeffler K, Rosen Y, von Davier A (2017) Exploring the measurement of collaborative problem solving using a human-agent educational game. In: Proceedings of the seventh international learning analytics & knowledge conference (LAK ‘17). ACM, New York, pp 570–571
Vainio V, Sajaniemi J (2007) Factors in novice programmers’ poor tracing skills. SIGCSE Bull 39(3):236–240
Vasconcelos J (2007) Basic strategy for algorithmic problem solving. http://www.cs.jhu.edu/~jorgev/cs106/ProblemSolving.html . Accessed 2 June 2010
Vírseda R d V, Orna EP, Berbis E, Guerrero S d L (2011) A logic teaching tool based on tableaux for verification and debugging of algorithms. In: Blackburn P, van Ditmarsch H, Soler-Toscano F, Manzano M (eds) Proceedings of the third international congress conference on tools for teaching logic (TICTTL’11). Springer, Berlin/Heidelberg, pp 239–248
Von Davier AA, Halpin PF (2013) Collaborative problem solving and the assessment of cognitive skills: psychometric considerations. ETS Res Rep Ser 2013(2):1–36
Wallingford E (1996) Toward a first course based on object-oriented patterns. In: Proceedings of the SIGCSE, pp 27–31
Wirth N (1971) Program development by stepwise refinement. CACM 14(4):221–227. http://sunnyday.mit.edu/16.355/wirth-refinement.html . Accessed 13 Nov 2010
Download references
Author information
Authors and affiliations.
Department of Education in Science & Technology, Technion–Israel Institute of Technology, Haifa, Israel
Orit Hazzan & Tami Lapidot
Faculty of Education, Beit Berl College, Doar Beit Berl, Israel
Noa Ragonis
You can also search for this author in PubMed Google Scholar
Rights and permissions
Reprints and permissions
Copyright information
© 2020 Springer Nature Switzerland AG
About this chapter
Hazzan, O., Ragonis, N., Lapidot, T. (2020). Problem-Solving Strategies. In: Guide to Teaching Computer Science. Springer, Cham. https://doi.org/10.1007/978-3-030-39360-1_8
Download citation
DOI : https://doi.org/10.1007/978-3-030-39360-1_8
Published : 06 August 2020
Publisher Name : Springer, Cham
Print ISBN : 978-3-030-39359-5
Online ISBN : 978-3-030-39360-1
eBook Packages : Computer Science Computer Science (R0)
Share this chapter
Anyone you share the following link with will be able to read this content:
Sorry, a shareable link is not currently available for this article.
Provided by the Springer Nature SharedIt content-sharing initiative
- Publish with us
Policies and ethics
- Find a journal
- Track your research
- Admiral “Amazing Grace” Hopper
Exploring the Intricacies of NP-Completeness in Computer Science
Understanding p vs np problems in computer science: a primer for beginners, understanding key theoretical frameworks in computer science: a beginner’s guide.

Learn Computer Science with Python
CS is a journey, not a destination
- Foundations
Understanding Algorithms: The Key to Problem-Solving Mastery

The world of computer science is a fascinating realm, where intricate concepts and technologies continuously shape the way we interact with machines. Among the vast array of ideas and principles, few are as fundamental and essential as algorithms. These powerful tools serve as the building blocks of computation, enabling computers to solve problems, make decisions, and process vast amounts of data efficiently.
An algorithm can be thought of as a step-by-step procedure or a set of instructions designed to solve a specific problem or accomplish a particular task. It represents a systematic approach to finding solutions and provides a structured way to tackle complex computational challenges. Algorithms are at the heart of various applications, from simple calculations to sophisticated machine learning models and complex data analysis.
Understanding algorithms and their inner workings is crucial for anyone interested in computer science. They serve as the backbone of software development, powering the creation of innovative applications across numerous domains. By comprehending the concept of algorithms, aspiring computer science enthusiasts gain a powerful toolset to approach problem-solving and gain insight into the efficiency and performance of different computational methods.
In this article, we aim to provide a clear and accessible introduction to algorithms, focusing on their importance in problem-solving and exploring common types such as searching, sorting, and recursion. By delving into these topics, readers will gain a solid foundation in algorithmic thinking and discover the underlying principles that drive the functioning of modern computing systems. Whether you’re a beginner in the world of computer science or seeking to deepen your understanding, this article will equip you with the knowledge to navigate the fascinating world of algorithms.
What are Algorithms?
At its core, an algorithm is a systematic, step-by-step procedure or set of rules designed to solve a problem or perform a specific task. It provides clear instructions that, when followed meticulously, lead to the desired outcome.
Consider an algorithm to be akin to a recipe for your favorite dish. When you decide to cook, the recipe is your go-to guide. It lists out the ingredients you need, their exact quantities, and a detailed, step-by-step explanation of the process, from how to prepare the ingredients to how to mix them, and finally, the cooking process. It even provides an order for adding the ingredients and specific times for cooking to ensure the dish turns out perfect.
In the same vein, an algorithm, within the realm of computer science, provides an explicit series of instructions to accomplish a goal. This could be a simple goal like sorting a list of numbers in ascending order, a more complex task such as searching for a specific data point in a massive dataset, or even a highly complicated task like determining the shortest path between two points on a map (think Google Maps). No matter the complexity of the problem at hand, there’s always an algorithm working tirelessly behind the scenes to solve it.
Furthermore, algorithms aren’t limited to specific programming languages. They are universal and can be implemented in any language. This is why understanding the fundamental concept of algorithms can empower you to solve problems across various programming languages.
The Importance of Algorithms
Algorithms are indisputably the backbone of all computational operations. They’re a fundamental part of the digital world that we interact with daily. When you search for something on the web, an algorithm is tirelessly working behind the scenes to sift through millions, possibly billions, of web pages to bring you the most relevant results. When you use a GPS to find the fastest route to a location, an algorithm is computing all possible paths, factoring in variables like traffic and road conditions, to provide you the optimal route.
Consider the world of social media, where algorithms curate personalized feeds based on our previous interactions, or in streaming platforms where they recommend shows and movies based on our viewing habits. Every click, every like, every search, and every interaction is processed by algorithms to serve you a seamless digital experience.
In the realm of computer science and beyond, everything revolves around problem-solving, and algorithms are our most reliable problem-solving tools. They provide a structured approach to problem-solving, breaking down complex problems into manageable steps and ensuring that every eventuality is accounted for.
Moreover, an algorithm’s efficiency is not just a matter of preference but a necessity. Given that computers have finite resources — time, memory, and computational power — the algorithms we use need to be optimized to make the best possible use of these resources. Efficient algorithms are the ones that can perform tasks more quickly, using less memory, and provide solutions to complex problems that might be infeasible with less efficient alternatives.
In the context of massive datasets (the likes of which are common in our data-driven world), the difference between a poorly designed algorithm and an efficient one could be the difference between a solution that takes years to compute and one that takes mere seconds. Therefore, understanding, designing, and implementing efficient algorithms is a critical skill for any computer scientist or software engineer.
Hence, as a computer science beginner, you are starting a journey where algorithms will be your best allies — universal keys capable of unlocking solutions to a myriad of problems, big or small.
Common Types of Algorithms: Searching and Sorting
Two of the most ubiquitous types of algorithms that beginners often encounter are searching and sorting algorithms.
Searching algorithms are designed to retrieve specific information from a data structure, like an array or a database. A simple example is the linear search, which works by checking each element in the array until it finds the one it’s looking for. Although easy to understand, this method isn’t efficient for large datasets, which is where more complex algorithms like binary search come in.
Binary search, on the other hand, is like looking up a word in the dictionary. Instead of checking each word from beginning to end, you open the dictionary in the middle and see if the word you’re looking for should be on the left or right side, thereby reducing the search space by half with each step.
Sorting algorithms, meanwhile, are designed to arrange elements in a particular order. A simple sorting algorithm is bubble sort, which works by repeatedly swapping adjacent elements if they’re in the wrong order. Again, while straightforward, it’s not efficient for larger datasets. More advanced sorting algorithms, such as quicksort or mergesort, have been designed to sort large data collections more efficiently.
Diving Deeper: Graph and Dynamic Programming Algorithms
Building upon our understanding of searching and sorting algorithms, let’s delve into two other families of algorithms often encountered in computer science: graph algorithms and dynamic programming algorithms.
A graph is a mathematical structure that models the relationship between pairs of objects. Graphs consist of vertices (or nodes) and edges (where each edge connects a pair of vertices). Graphs are commonly used to represent real-world systems such as social networks, web pages, biological networks, and more.
Graph algorithms are designed to solve problems centered around these structures. Some common graph algorithms include:
Dynamic programming is a powerful method used in optimization problems, where the main problem is broken down into simpler, overlapping subproblems. The solutions to these subproblems are stored and reused to build up the solution to the main problem, saving computational effort.
Here are two common dynamic programming problems:
Understanding these algorithm families — searching, sorting, graph, and dynamic programming algorithms — not only equips you with powerful tools to solve a variety of complex problems but also serves as a springboard to dive deeper into the rich ocean of algorithms and computer science.
Recursion: A Powerful Technique
While searching and sorting represent specific problem domains, recursion is a broad technique used in a wide range of algorithms. Recursion involves breaking down a problem into smaller, more manageable parts, and a function calling itself to solve these smaller parts.
To visualize recursion, consider the task of calculating factorial of a number. The factorial of a number n (denoted as n! ) is the product of all positive integers less than or equal to n . For instance, the factorial of 5 ( 5! ) is 5 x 4 x 3 x 2 x 1 = 120 . A recursive algorithm for finding factorial of n would involve multiplying n by the factorial of n-1 . The function keeps calling itself with a smaller value of n each time until it reaches a point where n is equal to 1, at which point it starts returning values back up the chain.
Algorithms are truly the heart of computer science, transforming raw data into valuable information and insight. Understanding their functionality and purpose is key to progressing in your computer science journey. As you continue your exploration, remember that each algorithm you encounter, no matter how complex it may seem, is simply a step-by-step procedure to solve a problem.
We’ve just scratched the surface of the fascinating world of algorithms. With time, patience, and practice, you will learn to create your own algorithms and start solving problems with confidence and efficiency.
Related Articles

Three Elegant Algorithms Every Computer Science Beginner Should Know
! |
CS for CA News & Updates
Computer science skills: computational thinking explained.
It’s a common misconception that computer science (CS) is only applicable to people working in a technology or STEM careers. However, skills learnt through CS are used in our everyday lives, and in a variety of subjects.
One of these skills is known as computational thinking (CT).
What is computational thinking?
There are many problem-solving skills involved in computer science, including those needed to design, develop, and debug software. Computational thinking is a way of describing these skills.
Computational thinking refers to the thought processes involved in defining a problem and its solution so that the solution can be expertly carried out by a computer. We don't need computers to engage in computational thinking, but CT can leverage the power of computers to solve a problem.
Computational thinking helps build these skills:
- Decomposition – the process of breaking down a complex problem into smaller parts that are more manageable, and helps us see problems as less overwhelming.
- Abstraction – identifying common features, recognizing patterns, and filtering out what we don’t need.
- Algorithmic Thinking – designing a set of steps to accomplish a specific task.
- Debugging and Evaluation – testing and refining a potential solution, and ensuring it’s the best fit for the problem.
These skills relate to critical thinking and problem solving skills across different subject matter, highlighting how concepts of computing can be combined with other fields of study to assist in problem-solving.
Computational thinking is a way of describing the many problem solving skills involved in computer science, including those needed to design, develop, and debug software. However, computer science is more than just skills, it also includes concepts about the Internet, networking, data, cybersecurity, artificial intelligence, and interfaces. Computational thinking can be relevant beyond computer science, overlapping with skills also used in other STEM subjects, as well as the arts, social sciences, and humanities.
Why is computational thinking important?
Computational thinking can apply these problem-solving techniques to a variety of subjects. For example, CT is established as one of the Science and Engineering Practices in the Next Generation Science Standards , and can also be found in several math state standards . Computational thinking also overlaps with skills used in other STEM subjects, as well as the arts, social sciences, and humanities. Computational thinking encourages us to use the power of computing beyond the screen and keyboard.
It can also allow us to advance equity in computer science education...
By centering the problem-solving skills that are at the heart of computer science, we can promote its integration with other subject areas, and expose more students to the possibilities of computer science.
Not only that, but computational thinking also opens the door for us to examine the limitations and opportunities of technology as it’s being developed. We’re able to analyze who is creating technology and why, as well as think critically about the ways in which it can impact society.
Want to learn more about computational thinking?
To learn more about computational thinking, check out the resources:
- This framework for CS for K-12 places CT at the core of its practices and is what the California standards are based on.
- Part of the British Computing Society, Computing at School put forth resources to assist teachers in the UK in embedding CT in their classrooms.
- This is one of the earliest definitions of CT for educators, and noteworthy for its inclusion of certain dispositions as being essential for effective CT.
- The developers of Scratch divide CT into concepts, practices, and perspectives, and focus on the expressive and creative nature of computing.
- Instead of focusing solely on standards for students, ISTE compiled a set of knowledge, skills, and mindsets needed for educators to be successful in integrating CT across the K-12 content areas and grade bands.
- Bebras began as an international competition to promote CT for students, regardless of programming experience. It is now increasingly being used as a form of CT assessment.
Explore Related Articles
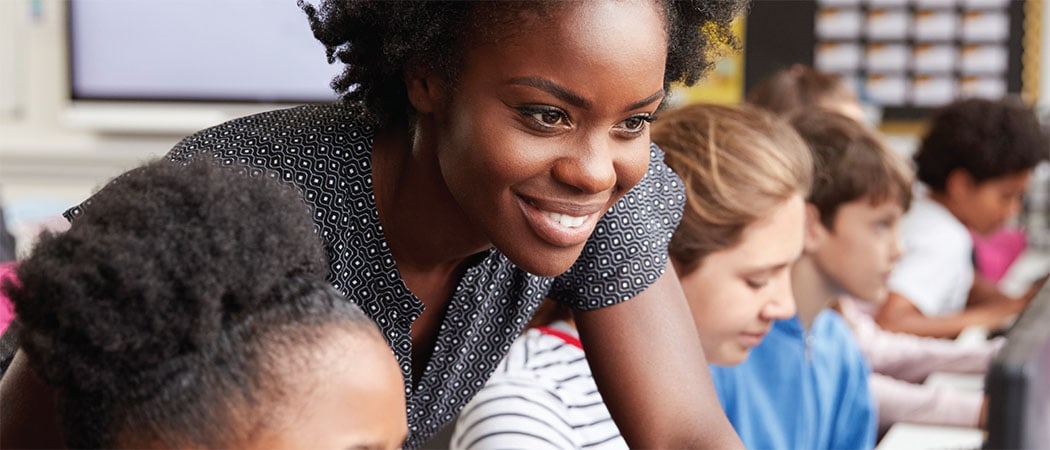
New Report on Equity in Computer Science Education in California’s Schools
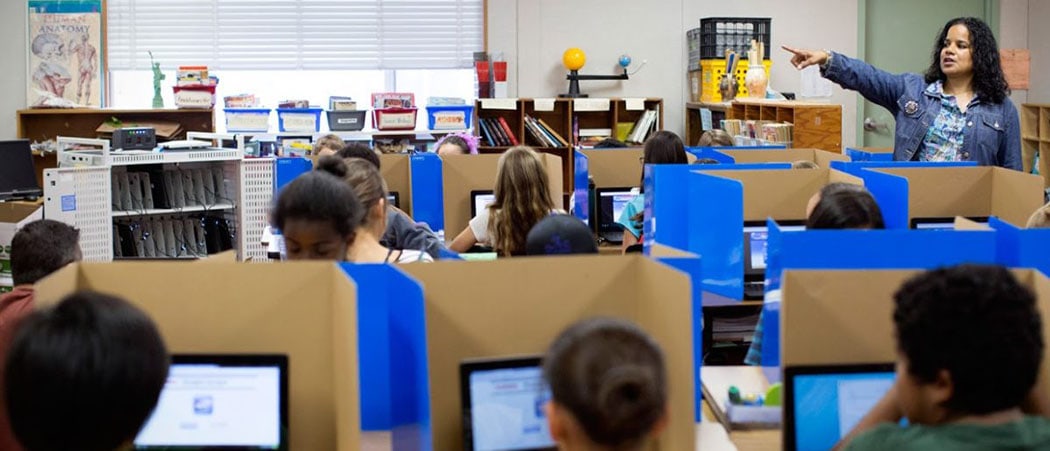
California Governor's Budget Supports Computer Science education
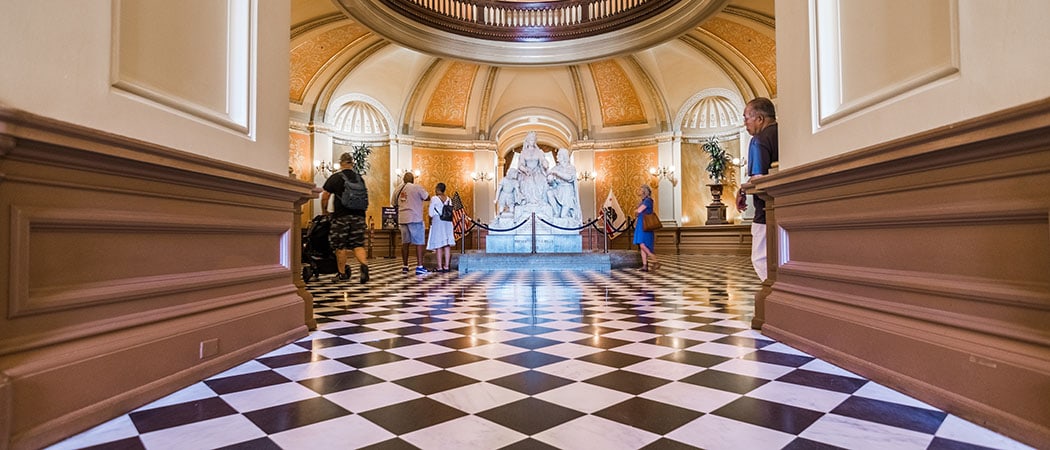
SBE Approves Computer Science Strategic Implementation Plan
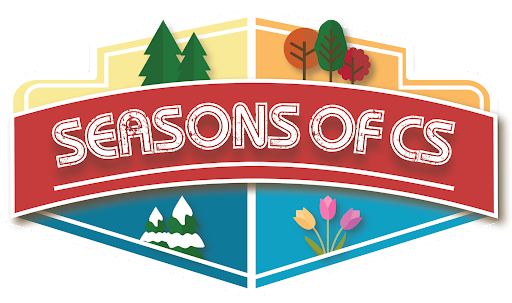
Unable to load Tweets
Popular Searches
Next generation science.
- Designing Challenge Based Science Learning
- Unit Library
What is Computational Thinking?
- Inclusive Integration of Computational Thinking
- Data Practices
- Creating Algorithms
- Understanding Systems with Computational Models
Computational thinking is an interrelated set of skills and practices for solving complex problems, a way to learn topics in many disciplines, and a necessity for fully participating in a computational world.
Many different terms are used when talking about computing, computer science, computational thinking, and programming. Computing encompasses the skills and practices in both computer science and computational thinking. While computer science is an individual academic discipline, computational thinking is a problem-solving approach that integrates across activities, and programming is the practice of developing a set of instructions that a computer can understand and execute, as well as debugging, organizing, and applying that code to appropriate problem-solving contexts. The skills and practices requiring computational thinking are broader, leveraging concepts and skills from computer science and applying them to other contexts, such as core academic disciplines (e.g. arts, English language arts, math, science, social studies) and everyday problem solving. For educators integrating computational thinking into their classrooms, we believe computational thinking is best understood as a series of interrelated skills and competencies.
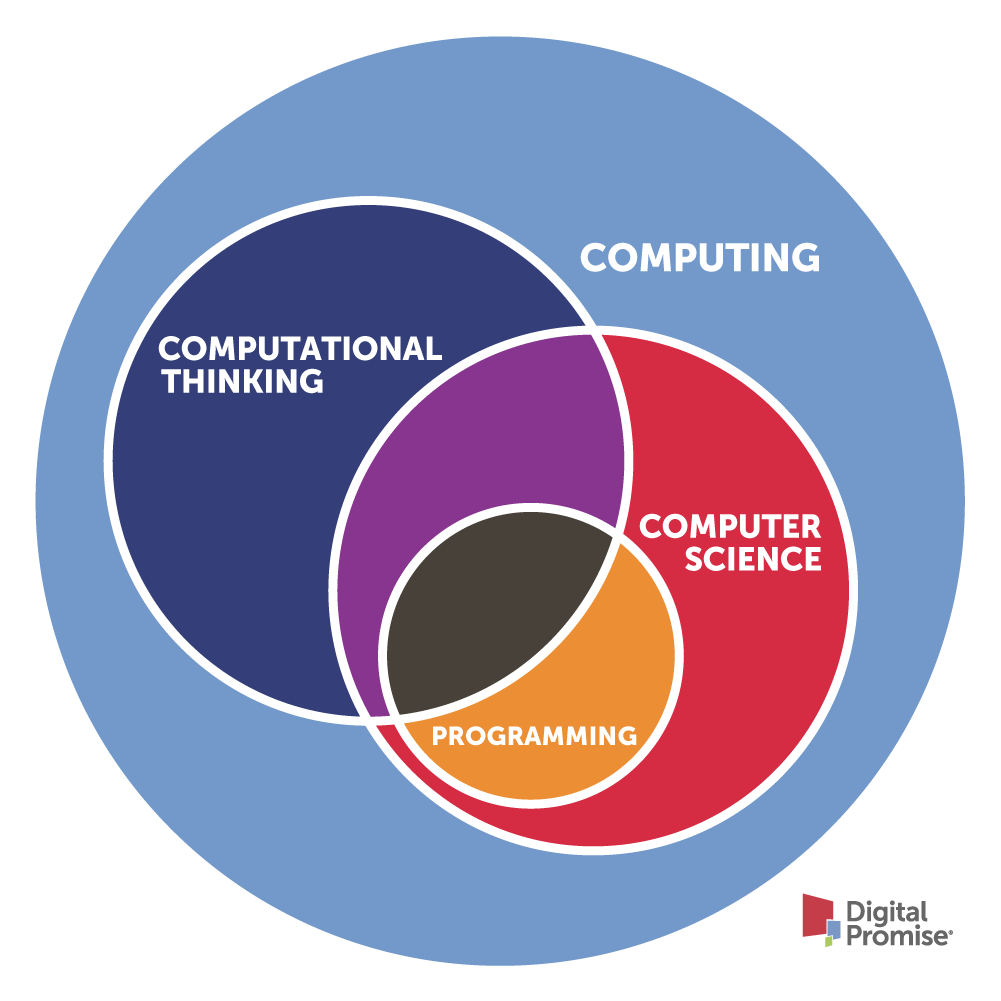
Figure 1. The relationship between computer science (CS), computational thinking (CT), programming and computing.
In order to integrate computational thinking into K-12 teaching and learning, educators must define what students need to know and be able to do to be successful computational thinkers. Our recommended framework has three concentric circles.
- Computational thinking skills , in the outermost circle, are the cognitive processes necessary to engage with computational tools to solve problems. These skills are the foundation to engage in any computational problem solving and should be integrated into early learning opportunities in K-3.
- Computational thinking practices , in the middle circle, combine multiple computational skills to solve an applied problem. Students in the older grades (4-12) may use these practices to develop artifacts such as a computer program, data visualization, or computational model.
- Inclusive pedagogies , in the innermost circle, are strategies for engaging all learners in computing, connecting applications to students’ interests and experiences, and providing opportunities to acknowledge, and combat biases and stereotypes within the computing field.
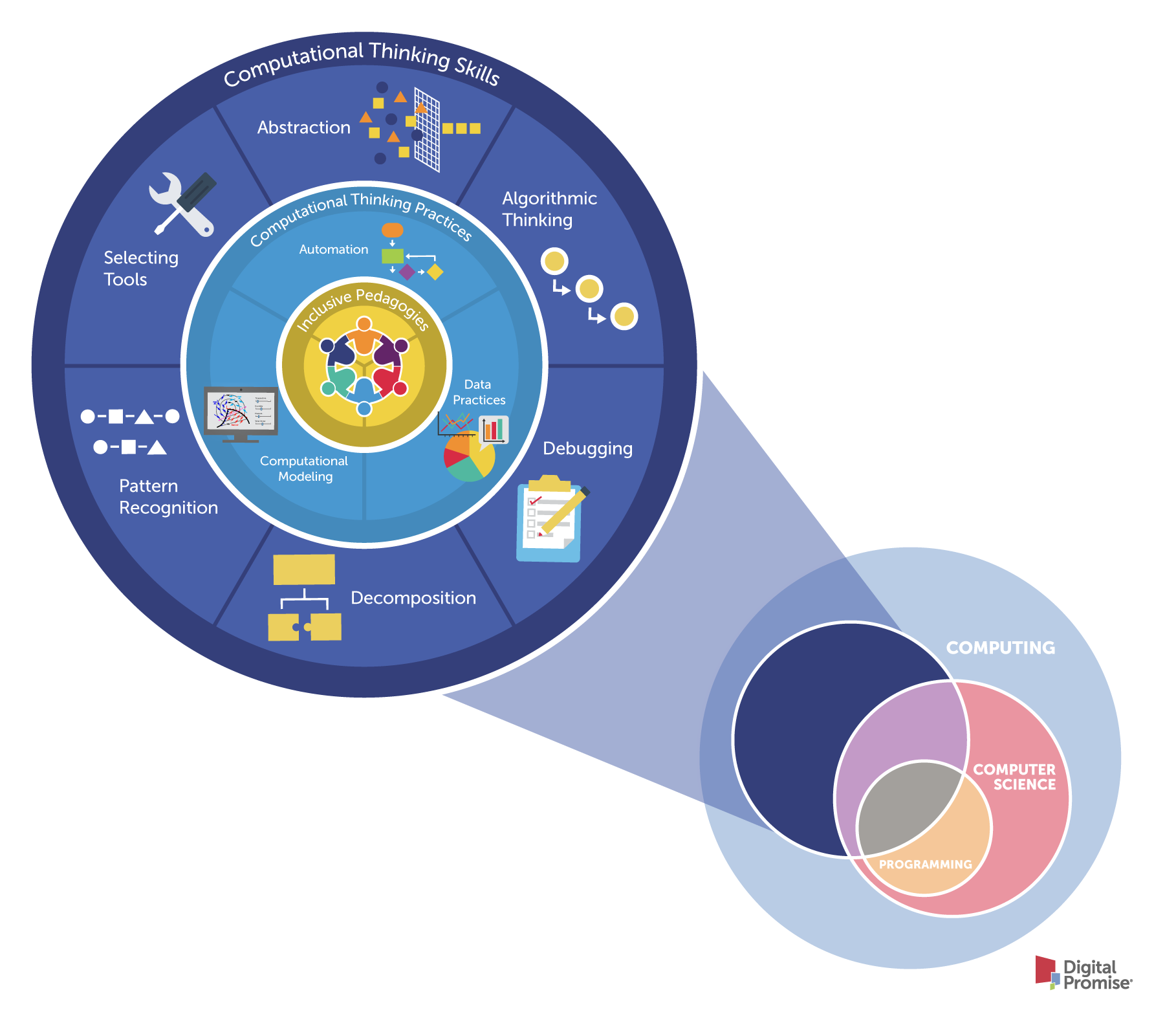
Figure 2. A framework for computational thinking integration.
What does inclusive computational thinking look like in a classroom? In the image below, we provide examples of inclusive computing pedagogies in the classroom. The pedagogies are divided into three categories to emphasize different pedagogical approaches to inclusivity. Designing Accessible Instruction refers to strategies teachers should use to engage all learners in computing. Connecting to Students’ Interests, Homes, and Communities refers to drawing on the experiences of students to design learning experiences that are connected with their homes, communities, interests and experiences to highlight the relevance of computing in their lives. Acknowledging and Combating Inequity refers to a teacher supporting students to recognize and take a stand against the oppression of marginalized groups in society broadly and specifically in computing. Together these pedagogical approaches promote a more inclusive computational thinking classroom environment, life-relevant learning, and opportunities to critique and counter inequalities. Educators should attend to each of the three approaches as they plan and teach lessons, especially related to computing.
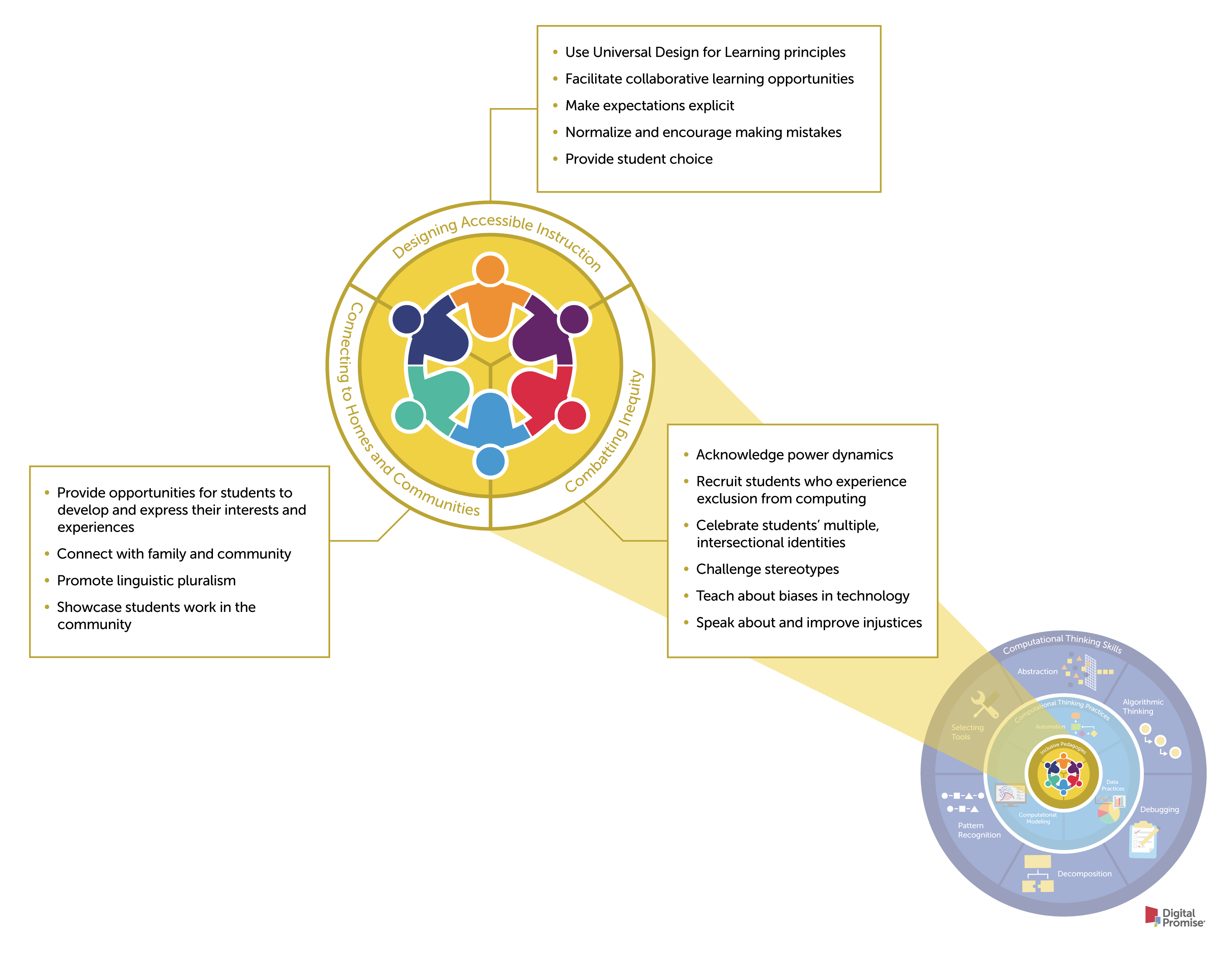
Figure 3. Examples of inclusive pedagogies for teaching computing in the classroom adapted from Israel et al., 2017; Kapor Center, 2021; Madkins et al., 2020; National Center for Women & Information Technology, 2021b; Paris & Alim, 2017; Ryoo, 2019; CSTeachingTips, 2021
Micro-credentials for computational thinking
A micro-credential is a digital certificate that verifies an individual’s competence in a specific skill or set of skills. To earn a micro-credential, teachers submit evidence of student work from classroom activities, as well as documentation of lesson planning and reflection.
Because the integration of computational thinking is new to most teachers, micro-credentials can be a useful tool for professional learning and/or credentialing pathways. Digital Promise has created micro-credentials for Computational Thinking Practices . These micro-credentials are framed around practices because the degree to which students have built foundational skills cannot be assessed until they are manifested through the applied practices.
Visit Digital Promise’s micro-credential platform to find out more and start earning micro-credentials today!
Sign up for updates!

3 Types of Computer Science Skills Every CS Professional Needs
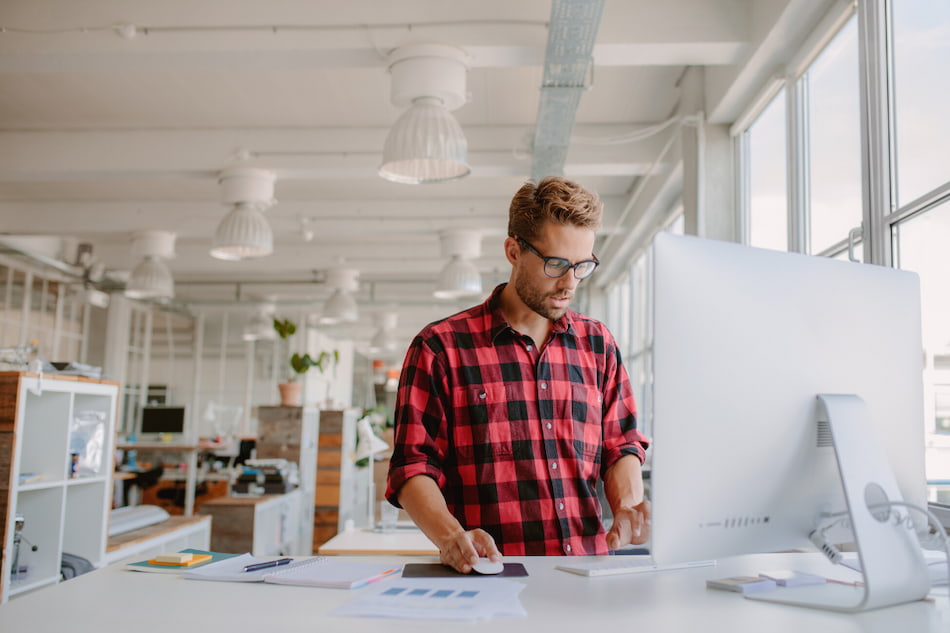
Industry Advice Computing and IT
Computer science is an increasingly popular field, and with good reason. Society has come to rely on the technology created by this industry to fulfill the functions of daily life, resulting in both exciting career opportunities and above-average salaries for individuals who choose to pursue a computer science career.
While the job outlook remains overwhelmingly positive , the evolution of the computer science field over the last few decades has created a demand for professionals with more than just basic coding skills. Now, professionals looking for success must have a strong combination of technical, interview, and soft skills unique to this specific sector.
Read on to explore what traits, skills, and subject matter fall into these three categories, and how a master’s degree from a university like Northeastern can help aspiring computer scientists hone them all.
Download Our Free Guide to Breaking into Computer Science
Whether you have a technical or non-technical background, here’s what you need to know.
DOWNLOAD NOW
Top Computer Science Skills to Learn
1. specific technical skills.
The most commonly acknowledged type of skills required among computer scientists are the practical abilities that allow them to develop software and digital tools. Some of the top skills in this category include:
- Programming: This incorporates deep knowledge of algorithms and data structures, and coding skills in languages like Java, C, Python, and JavaScript. Those hoping to improve their coding skills specifically should consider pursuing formal training in the form of a graduate degree or bootcamp .
- Software development: CS professionals should possess knowledge of software development and engineering principles, including the software development process from start to finish—writing programs using popular program languages, testing for usability, and ultimately implementing the programs.
- Mathematics : Alongside a comprehensive understanding of mathematical theory—which is equally as vital for aspiring CS professionals to obtain—knowledge of statistics, calculus, linear algebra, and other advanced mathematical techniques are mandatory for individuals in this field.
- Data Analysis: Basic data analysis skills are important for computer scientists, as data plays a key role in many advanced CS practices, including artificial intelligence (AI), predictive analysis , and more.
- Data Visualization: As crucial as it is to be able to analyze data effectively, it’s also essential that CS professionals can properly visualize data and results in a way that stakeholders can understand. This includes translating raw data into graphs, charts, and other visual tools to help communicate findings .
- Ethics: As technology continues to advance—especially with tools like artificial intelligence and machine learning—professionals in this field must develop an understanding of ethics and its impact on computer science.
The above list of skills includes only the baseline requirements for generic roles in the CS field. Those who wish to specialize in a certain niche area—such as AI , data science , cybersecurity , etc.—or plan to apply their computer science skills into a specific field will need to expand their knowledge in those areas, as well.
Honing Your Practical Skills With a Master’s Degree
One of the most efficient ways to gain these industry-specific skill sets is through the pursuit of a master’s degree in computer science from a top university like Northeastern. In a program like this, students participate in an array of courses designed to provide both the in-depth knowledge and hands-on abilities needed to thrive in this ever-changing industry.
“We really try to balance theory and practice [in our programs],” says Ian Gorton , director of the computer science master’s programs at Northeastern University—Seattle . “[We want] graduates to have a deep enough education theoretically so they can keep up with advances and changing technology, but also have the practical skills needed to get a job and thrive professionally.”
Northeastern’s MS in Computer Science curriculum is strategically designed to expose students to both computer science theory and practice. Courses like advanced software development, for example, cover advanced system design and solution-building, while simultaneously providing students the chance to practice explaining their solutions through “code walk-throughs.”
“[A code walk-through] is a common industry practice in which people stand up and explain their solution to an instructor…and then get questioned about it,” Gorton says. “This is obviously good interview practice, but also breeds a rigor in people that gets them thinking about how to solve problems.”
Learn More: Is a Master’s in Computer Science Worth the Investment?
Other courses focus on building software individually or as part of a small team to solve bigger problems. In these scenarios, students “work together to try to solve problems and build a solution,” Gorton says. Through this process, “they learn principles about how to split up a solution so that multiple people can work on it at the same time, and how they can plug the resulting components together and make it all work” the way they might as part of a future engineering team.
This type of hands-on learning also extends outside of the classroom in Northeastern’s programs. Through experiential learning opportunities, students get the chance to explore real-world problems for organizations that are a part of Northeastern’s expansive partner network.
“In our advanced software engineering course, we basically form a team of about 20 people, and we build a solution to a real problem…[for] an external stakeholder,” Gorton says. “It’s always a really tricky problem and requires teams to work across campuses to build a solution.”
Did You Know: Northeastern offers advanced computer science degrees at seven of our regional locations, including Arlington ; Boston ; Oakland ; Portland, Maine ; Seattle ; Silicon Valley ; and Vancouver .
Northeastern’s program also embraces the need for students in this field to have certain interdisciplinary skills that allow them to apply their technical knowledge to other industries. In fact, Northeastern’s Align MS in Computer Science program is specifically designed for students from a non-computer science background . In this program, students first learn the foundational CS skills they would have obtained in pursuing an undergraduate CS degree and then advance to the more complex topics covered in a master’s degree. These individuals graduate with advanced CS knowledge that complements their existing expertise in another sector and will allow them to change careers to computer science.
Students with or without a background in computer science also have the opportunity to declare a computer science specialization while enrolled in Northeastern’s master’s in CS programs. In doing so, they have the chance to learn an array of other relevant, practical skills that they can use in pursuit of specialized roles. For example, students who declare a specialization in AI might explore more advanced data science topics, as well as statistical modeling , natural language processing, machine learning, and more.
Gorton explains that, while specializations like these can be useful in setting professionals apart in competitive job markets, most will likely start their career in CS with a much broader role. For this reason, Northeastern strategically incorporates a balance between core skill sets and those explored in a specialization. “You have to have a good broad portfolio of skills and then have a specialization that you can kind of pull out of your pocket when you need to,” he says.
2. Relevant “Soft” Skills
Soft skills are considered those which are not tactical, technical, or tied directly to a specific career path. Emotional intelligence, leadership , and innovation are common examples of these kinds of traits, which are gained through hands-on experience and are valued across industries .
While technical skills were often thought of as the core of functioning workplaces in the past, businesses today now consider “soft skills” to be equally— if not more —relevant. Below, we explore the top “soft skills” that computer scientists should obtain.
- Critical Thinking: Being able to identify a problem, analyze the details of the situation, and then formulate an effective solution is an incredibly important aspect of computer science work.
- Attention to Detail: Effective computer scientists must be able to pay close attention to detail, as their work is often complex, demanding, and requires a keen eye.
- Creative Problem Solving: Solutions to many of the problems computer scientists are tasked with solving are not always obvious, and instead require these individuals to think outside of the box.
- Communication: Communication is a key soft skill in most industries , and computer science is no different. Professionals in this field must be able to communicate effectively with their teams , their bosses, and their stakeholders—including using data to tell stories and share insights.
- Listening : Tied to the need for proper communication skills is a need for computer scientists to be good listeners. Professionals should be able to listen to people’s problems and establish the necessary context from those conversations to solve them. This is especially important when CS specialists work with industries they’re not particularly familiar with.
- Collaboration: Despite common misconception, computer scientists very rarely work in isolation. In fact, more often than not, they are required to operate as part of a team, either working with other computer scientists to reach a solution or develop a product, or working with other members of a larger business team on a project. Either way, developing the skills needed to work as part of a larger group is essential to a computer scientist’s success.
Honing Your Soft Skills With a Master’s Degree
Taking the time to develop these soft skills can go a long way in setting aspiring computer scientists on a path toward success. Many of these abilities can be obtained through real-world experience or the pursuit of a Master of Science in Computer Science degree at a top university like Northeastern.
Many of the courses Gorton mentions have been designed to hone students’ practical skills in Northeastern’s CS programs also simultaneously provide opportunities for students to develop many of these integral skill sets. Courses that require students to stand up in front of their professors and peers and complete a code walk-through, for example, allow them to sharpen their communication skills. Similarly, courses in which students work as part of a team allow them to practice collaboration, listening, and leadership. By incorporating real-world problem solving into coursework early on—and providing countless opportunities for hands-on learning within functional workplaces—Northeastern also gives students the chance to practice critical thinking, creative problem solving, and more.
Graduate school provides a unique opportunity for aspiring computer scientists to acquire these vital skill sets alongside more technical ones, and develop themselves into well-rounded professionals.
3. Interview Skills
When pursuing a new role in any industry, applicants should work to develop their interview skills . This might include preparing for your interview by doing research on the company you’re interviewing with, prepping your answers to commonly asked questions , or even practicing maintaining eye-contact during a conversation.
In certain industries, however, there is more to an interview than a simple series of questions and answers. In the computer science field, for instance, an interview often includes an in-person presentation and a descriptive explanation of your work in which applicants answer in-depth questions about how they reached their solutions. An interview in this field may also require applicants to display certain examples of their live projects via a portfolio and to speak at length about the success of that work.
In order to prepare for this unique type of interview, Gorton identifies a few key skill sets that aspiring CS professionals should work to hone beforehand.
Presentation Skills
“There is a whole sort of ritual for a technical interview these days,” he says. “You have to not only be able to answer [an interviewer’s] questions, but you have to also know how to communicate your solution.”
In order to do this, an interviewee will be asked to write out a problem on a whiteboard and describe the thought process as they work to solve it. While the act of problem-solving should likely be second nature to those in this field, this interview task often feels more daunting to those without proper presentation skills. “You have to explain what you’re doing as you go along and engage the interviewer in a dialogue,” he says. “You don’t just want to turn around and start writing on the board for five minutes.”
To sharpen this skill, Gorton suggests practicing this process a few times through before each interview to ensure you are comfortable both with your actual mathematics work and your presentation abilities. “You’re probably not going to get it right the first time,” he says, emphasizing that practice makes perfect.
For this reason, Northeastern’s programs “spend a lot of time working with students on those sorts of technical problems and getting them not only to understand their solutions on a technical level, but [also] how to communicate them properly,” he says.
Portfolio Building
While computer scientists may not utilize a print or online portfolio to display their work the way professionals in other fields might, it is still important for them to know how to properly share their work in an interview if asked.
Gorton explains that prepared CS professionals will either link to live projects or show examples within code repositories online. “When we write solutions, we put them typically into something called GitHub , and you make your GitHub code or profile public so you can cite it on your resumé and show the projects you’ve contributed to.”
Setting You Up for Success: Having the ability to properly integrate coding examples into a resumé is an essential skill for computer scientists, but many computer scientists who are just starting out don’t have live examples of their work to share. To help build this portfolio of work early on, Northeastern’s master’s in computer science programs give students dozens of opportunities to practice their work hands-on through experiential learning opportunities, group projects, in-class work with real-world external stakeholders, and more.
Networking is a pivotal part of any interview process, no matter the industry. Today, 85 percent of all jobs are filled through this practice, and the computer science field is no different. The positive, professional connections aspiring CS professionals make through their time in graduate school or in the workforce have the ability to set them apart during interview processes—especially when it comes to applying to top tech companies like Google, Facebook, or Amazon.
For this reason, individuals need to take the time to learn how to properly make and maintain relationships with those in the industry. Northeastern’s graduate programs in computer science , for example, offer opportunities for students to attend university-hosted events, collaborate with industry professionals, and make real-world connections with those in their field through time spent working hands-on with leading organizations .
Pursuing a Master’s Degree from Northeastern
Whether you are looking to break into the computer science industry or are hoping to advance your career, a master’s degree from Northeastern University can help. Both the master’s in computer science and Align master’s in computer science programs are designed to help aspiring professionals obtain the technical, “soft,” and interview skills needed to thrive in this industry, all while under the guidance of leading industry professionals. Grow your network, build your resumé , and start your career off right with a master’s degree in computer science from Northeastern.
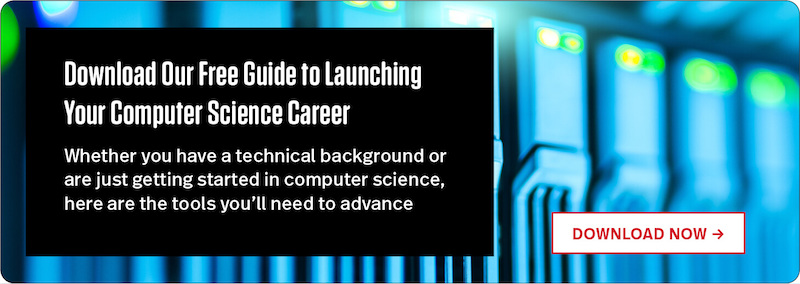
Subscribe below to receive future content from the Graduate Programs Blog.
About shayna joubert, related articles.
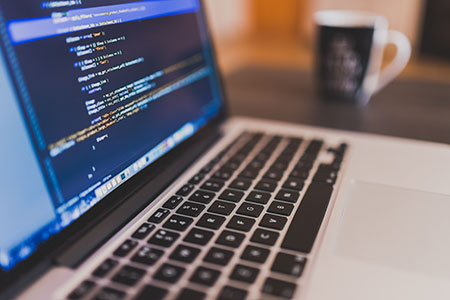
Why This Alum Decided to Earn His Master’s Degree in Computer Science
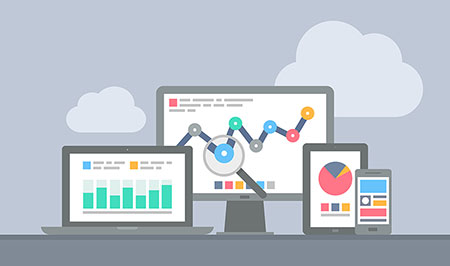
The Top 10 Highest-Paying Big Data Careers
Data scientist vs. data analyst: what’s the difference, did you know.
Computer science professionals see an average $30,000 salary increase after earning a master's degree. (Georgetown Center on Education)
Master of Science in Computer Science
Every company needs computer scientists. Launch your career today.
Most Popular:
Tips for taking online classes: 8 strategies for success, public health careers: what can you do with an mph, 7 international business careers that are in high demand, edd vs. phd in education: what’s the difference, 7 must-have skills for data analysts, in-demand biotechnology careers shaping our future, the benefits of online learning: 8 advantages of online degrees, how to write a statement of purpose for graduate school, the best of our graduate blog—right to your inbox.
Stay up to date on our latest posts and university events. Plus receive relevant career tips and grad school advice.
By providing us with your email, you agree to the terms of our Privacy Policy and Terms of Service.
Keep Reading:
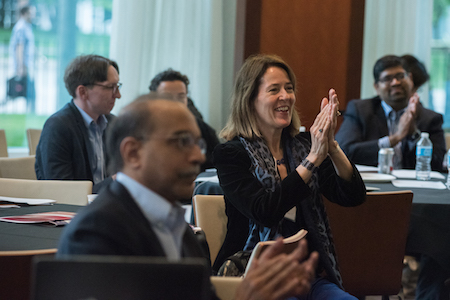
Top Higher Education Conferences To Attend in 2024
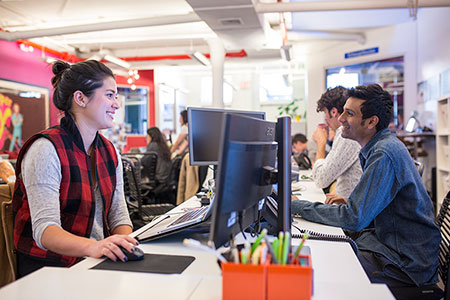
Grad School or Work? How To Balance Both
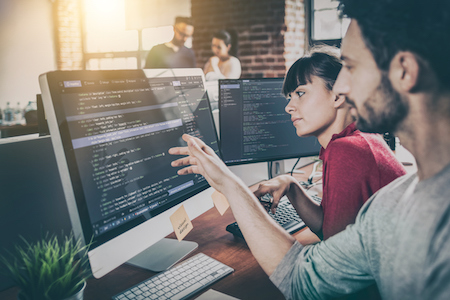
Is a Master’s in Computer Science Worth the Investment?
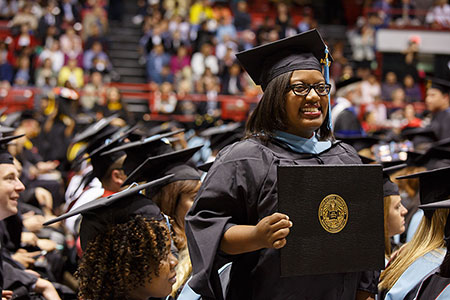
Should I Go to Grad School: 4 Questions To Consider
- IEEE CS Standards
- Career Center
- Subscribe to Newsletter
- IEEE Standards
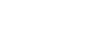
- For Industry Professionals
- For Students
- Launch a New Career
- Membership FAQ
- Membership FAQs
- Membership Grades
- Special Circumstances
- Discounts & Payments
- Distinguished Contributor Recognition
- Grant Programs
- Find a Local Chapter
- Find a Distinguished Visitor
- About Distinguished Visitors Program
- Find a Speaker on Early Career Topics
- Technical Communities
- Collabratec (Discussion Forum)
- My Subscriptions
- My Referrals
- Computer Magazine
- ComputingEdge Magazine
- Let us help make your event a success. EXPLORE PLANNING SERVICES
- Events Calendar
- Calls for Papers
- Conference Proceedings
- Conference Highlights
- Top 2024 Conferences
- Conference Sponsorship Options
- Conference Planning Services
- Conference Organizer Resources
- Virtual Conference Guide
- Get a Quote
- CPS Dashboard
- CPS Author FAQ
- CPS Organizer FAQ
- Find the latest in advanced computing research. VISIT THE DIGITAL LIBRARY
- Open Access
- Tech News Blog
- Author Guidelines
- Reviewer Information
- Guest Editor Information
- Editor Information
- Editor-in-Chief Information
- Volunteer Opportunities
- Video Library
- Member Benefits
- Institutional Library Subscriptions
- Advertising and Sponsorship
- Code of Ethics
- Educational Webinars
- Online Education
- Certifications
- Industry Webinars & Whitepapers
- Research Reports
- Bodies of Knowledge
- CS for Industry Professionals
- Resource Library
- Newsletters
- Women in Computing
- Digital Library Access
- Organize a Conference
- Run a Publication
- Become a Distinguished Speaker
- Participate in Standards Activities
- Peer Review Content
- Author Resources
- Publish Open Access
- Society Leadership
- Boards & Committees
- Local Chapters
- Governance Resources
- Conference Publishing Services
- Chapter Resources
- About the Board of Governors
- Board of Governors Members
- Diversity & Inclusion
- Open Volunteer Opportunities
- Award Recipients
- Student Scholarships & Awards
- Nominate an Election Candidate
- Nominate a Colleague
- Corporate Partnerships
- Conference Sponsorships & Exhibits
- Advertising
- Recruitment
- Publications
- Education & Career
How Computer Engineering Helps You Think Creatively
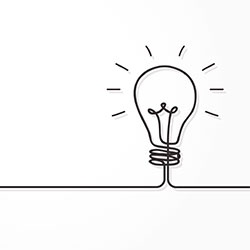
But in reality, learning the basics of computer science can help you think more critically and with more novel inspiration, ultimately helping you in other areas of your life.
Thinking of a career in computing? Our “Careers in Computing” blog will get you there
Apply Creative Problem Solving to Other Areas
Let’s start by explaining why the creative problem-solving skills you’ll learn in computer science can help you in everyday life:
- Novel solutions and new products. Being familiar with creating and polishing hardware and/or software can help you come up with ingenious solutions for your everyday life. You’re used to thinking about problems as solvable challenges, so you naturally come up with ways to address them. This applies to areas beyond computer science as well; for example, one former computer engineer used his creativity to engineer a pillow that reduces pressure on your face while sleeping .
- Lateral thinking and breaking patterns. Writing code and creating applications from scratch also incentivizes you to think laterally and break the patterns you’d otherwise fall into. Traditional lines of thinking just won’t work for some problems, so you’ll be forced to think in new, creative ways. That allows you to experiment with new approaches and keep trying until you find something that works.
- Seeing problems from other perspectives. As a computer engineer, you’ll be forced to see problems from other perspectives, whether you want to or not. That might mean reviewing code that someone else wrote, getting feedback from a client who has no familiarity with engineering, or imagining how an application might look to a user who’s never seen it before. In any case, you’ll quickly learn how to broaden your perspective, which means you’ll see problems in an entirely new light.
How Computer Engineering Improves Your Abilities
So how exactly does computer engineering improve your creative abilities in this way?
- Generating new ideas. You have to be creative if you’re going to generate new ideas . In some roles, you’ll be responsible for coming up with the ideas yourself—either designing your own apps for circulation, or making direct recommendations to your clients. In other scenarios, you’ll be responsible for coming up with novel ways to include a feature that might otherwise be impossible. In any case, you’ll be forced to come up with ideas constantly, which gets easier the more you practice it.
- Reviewing code. You’ll also be responsible for reviewing code—including code that you wrote and code that other people wrote. Reviewing your own code forces you to see it from an outsider’s perspective, and reviewing the code of others gives you insight into how they think. That diverse experience lends itself to imagining scenarios from different perspectives.
- Fixing bugs. Finding and fixing bugs is an important part of the job, and it’s one of the most creatively enlightening. To resolve the problem, you first have to understand why it’s happening. If you’ve written the code yourself, it’s easy to think the program will run flawlessly, so you’ll have to challenge yourself to start looking for the root cause of the problem. Sometimes, tinkering with the code will only result in more problems, which forces you to go back to the drawing board with a new angle of approach. It’s an ideal problem-solving exercise, and one you’ll have to undergo many times.
- Aesthetics and approachability. Finally, you’ll need to think about the aesthetics and approachability of what you’re creating. Your code might be perfectly polished on the backend, but if users have a hard time understanding the sequence of actions to follow to get a product to do what they want, you may need to rebuild it.
Latest career advice from our Career Round Table: With Demand for Data Scientists at an All-Time High, Top Experts Offer Sound Career Advice
Is Computer Science Worth Learning?
If you’re not already experienced in a field related to computer science, you might feel intimidated at the idea of getting involved in the subject. After all, people spend years, if not decades studying computer science to become professionals.
The good news is, you don’t need decades of experience to see the creative problem-solving benefits of the craft. Learning the basics of a programming language, or even familiarizing yourself with the type of logic necessary to code, can be beneficial to you in your daily life. Take a few hours and flesh out your skills; you’ll be glad you did.
Recommended by IEEE Computer Society
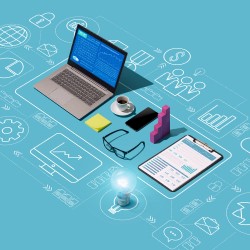
Amara's Law and Its Place in the Future of Tech
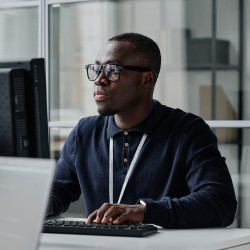
Cybersecurity Concerns for Coding Professionals
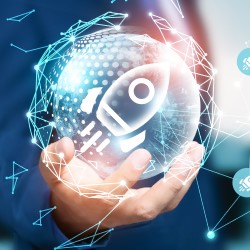
AI Has Arrived and Is Vital to Business Scalability

Gain An Edge On The Competition With Edge Computing

What is Green Coding and its Impact on Ethical Web Development?
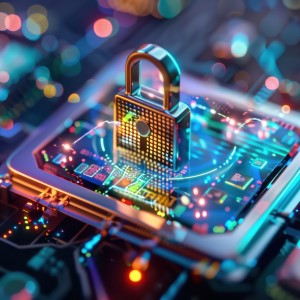
AI-Driven Data Security to Protect Against AI-Driven Data Leakage

Best Practices for Using Terraform: An In-depth Guide

The Integration of Amazon Redshift Cloud Data Warehouse
- QUICK LINKS
- How to enroll
- Career services
A list of essential computer science skills
This article was updated on December 13, 2023.
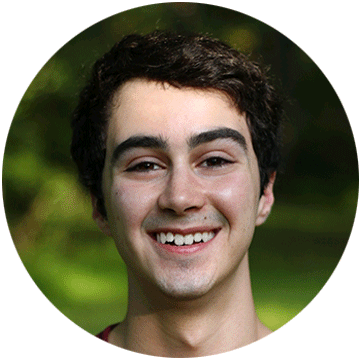
Written by Michael Feder
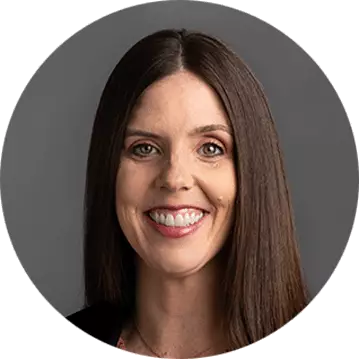
Reviewed by Kathryn Uhles , MIS, MSP, Dean, College of Business and IT
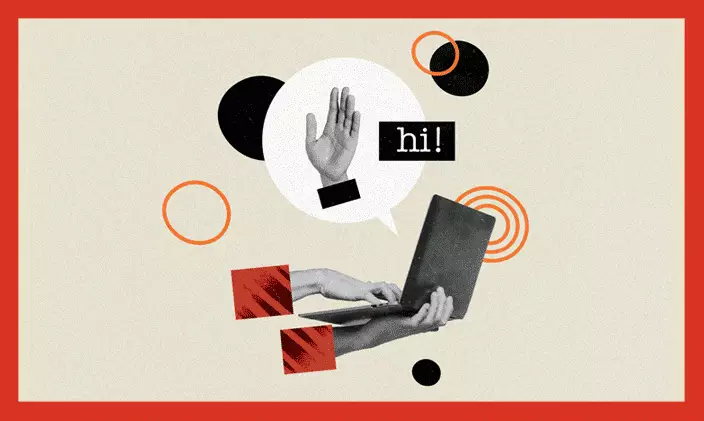
It may seem obvious that hard skills acquired through a degree are the most important factor of a strong job candidate. However, according to Ian Siegel, co-founder and CEO of ZipRecruiter, 93% of employers say soft skills play a key role in making a hiring decision. This means it’s important to highlight on your resumé a well-rounded set of skills.
However, knowing which mix of hard and soft skills to include can be tough. Do you emphasize the hard skills and include a few soft skills? Do you give equal balance to both? To better understand which approach is most likely to work, we’ll explore which hard and soft skills are essential within the computer science industry today, and we’ll look at why employers may be looking for applicants with these skills.
Hard skills in computer science
Careers in computer science rely on technical knowledge, which is also known as hard skills. These skills are directly related to job duties and can be learned and honed through professional development programs or advanced degree courses like a master’s degree in information systems . Here are some examples of common hard skills in computer science careers.
Coding languages
In many computer science roles , knowledge of common computer languages can be helpful. Even if your job doesn’t involve writing code, understanding it can empower you to troubleshoot problems or identify aspects of a computer system that can be improved.
Different languages have different applications, so knowing which languages your company uses can help narrow down the list of ones to include on your resumé, especially if you are fluent in several of them.
While coding languages differ quite a bit in syntax, philosophy and other structural elements, some programming concepts are virtually universal. Because of that, it doesn’t hurt to mention other languages you’re familiar with.
Data analysis
Some jobs in computer science may require you to be familiar with how to analyze data . This means knowing how to collect and organize data, and how to form conclusions based on that data.
Data analysis can play an important role in making applications more efficient. It can highlight bottlenecks as well as identify procedural areas in need of improvement. For instance, if the data shows that many users are experiencing similar computer issues while installing an application or signing up for an account, analysis may indicate there is a problem to solve with those processes.
Software development
Along with coding languages, it’s important to express your knowledge of software development . This demonstrates you understand the process of developing a software application, which involves developing big-picture ideas, coding and collaborating with team members cross-functionally.
Your resumé should emphasize what roles you have previously had in software development. With these details, employers can get a clearer understanding of your experience and how it fits in with their needs.
Technical writing
Often, professionals in computer science must not only perform technical duties. They may need to explain technical issues with processes or software too. This includes what the issue is, how it was found, the impacts of the issue and how to fix it. For this reason, it’s important to have skills in technical writing. IT professionals may find themselves providing documentation for how to perform processes like creating new accounts or connecting machines to a network. Technical writing skills are important so that these concepts are explained clearly and can be performed consistently.
Soft skills in computer science
Soft skills help make a resumé more well rounded, adding to the overall picture that prospective employers see when considering a candidate. The following skills are examples of the types of skills many employers find valuable in IT and beyond.
Problem-solving
Problem-solving is an essential skill for working in computer science and IT. If you have a natural curiosity to learn how to solve a problem, this may be an intriguing field to study and embark upon. It’s important to be able to also explain what problems may be uncovered and how to fix them.
Communication
Communication is important in just about every profession, and computer science jobs are no exception. Because of the work’s technical nature, it helps to be able to explain concepts clearly to people who work outside of IT. This is also true when warning team members of possible security risks.
Even within the technical sphere, good communication is helpful. Computer code is usually used and maintained by a team, for example, so concise documentation and comments within the code can help others quickly understand the program.
It is also vital to be able to explain potential issues to the rest of the team so they can work efficiently.
Time management
Being able to prioritize your time is essential for success in most careers, including computer science. Team members will not only depend on your work to do theirs, but your work could also potentially impact productivity throughout the company.
Within certain fields like software development, this skill is especially vital when quick coding is required. Additionally, there may be times when another team member’s task depends on yours being complete, so recognizing this and prioritizing these jobs is helpful.
Computer science professionals often work as a team, collaborating on code or orchestrating complex, companywide networks. Hiring managers value employees who can work well as part of a team.
Teamwork involves a variety of interpersonal skills, such as recognizing teammates’ strengths and delegating tasks effectively.
In a role like software development, this could include knowing when and whom to ask for coding advice on a specific branch of code. In a field like IT, this could mean knowing which team members have more experience with an aspect of the company computer system.
Eye for detail
Debugging code and troubleshooting computer systems can be difficult. Since so many small and large variables can have a considerable impact, it’s essential to be observant and meticulous. An error as simple as a misplaced decimal point in a code or an improperly configured network can cause significant issues.
While it is useful to be able to debug code by being observant, it is just as helpful to be able to reduce errors while writing code. Writing bug-free code may be virtually impossible , but writing code while being mindful of decisions concerning logic and syntax can help keep major bugs out of the picture.
Computer science and IT programs at University of Phoenix
If you’re interested in pursuing a job in computer information systems, the first step is to learn skills often taught in an information technology degree program or a related field. University of Phoenix offers bachelor’s degrees in the following fields of study.
- Bachelor of Science in Computer Science — This computer science degree teaches you how to apply information technology theory and principles to real-world business challenges. Advanced concepts in math, programming and computer architecture are covered.
- Bachelor of Science in Cybersecurity — This program teaches top skills such as security policies, network security, information systems security and cybersecurity.
- Bachelor of Science in Data Science — Gain fundamental skills and knowledge needed to analyze, manipulate and process data sets using statistical software. Learn ETL (extract, transform, load) processes for integrating data sets for business intelligence and more.
- Bachelor of Science in Information Technology — This program is designed to teach such skills as business process, cybersecurity, information systems, operations and systems analysis.
Learn more about these and other information technology degrees at University of Phoenix!

ABOUT THE AUTHOR
A graduate of Johns Hopkins University and its Writing Seminars program and winner of the Stephen A. Dixon Literary Prize, Michael Feder brings an eye for detail and a passion for research to every article he writes. His academic and professional background includes experience in marketing, content development, script writing and SEO. Today, he works as a multimedia specialist at University of Phoenix where he covers a variety of topics ranging from healthcare to IT.
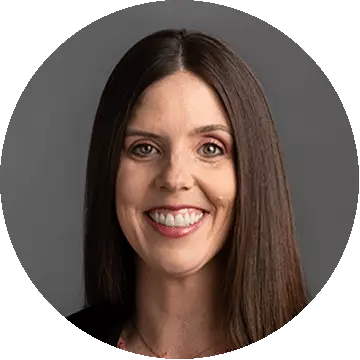
ABOUT THE REVIEWER
Currently Dean of the College of Business and Information Technology, Kathryn Uhles has served University of Phoenix in a variety of roles since 2006. Prior to joining University of Phoenix, Kathryn taught fifth grade to underprivileged youth in Phoenix.
This article has been vetted by University of Phoenix's editorial advisory committee. Read more about our editorial process.
Read more articles like this:
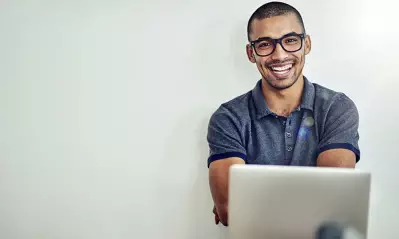
How Many Credits to Graduate College?
Tuition and financial aid.
May 23, 2022 • 13 minutes
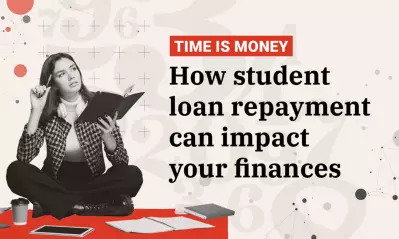
How Repaying Your Student Loans Can Impact Your Finances
April 28, 2023 • 7 minutes
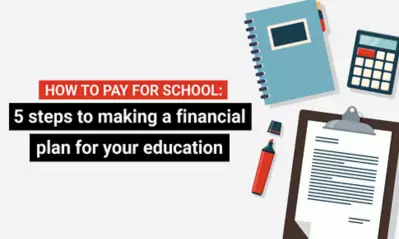
How to make a financial plan for your education
August 26, 2022 • 8 Minutes

Computer science and the art of problem-solvin g

Spirit of Performance 👻
Computer science is the study of problems, problem-solving, and the solutions that come out of the problem-solving process. Given a problem, a computer scientist’s goal is to develop an algorithm , a step-by-step list of instructions for solving any instance of the problem that might arise. Algorithms are finite processes that if followed will solve the problem. Algorithms are solutions.
Solving problems is the core of computer science. Programmers must first understand how a human solves a problem, then understand how to translate this “algorithm” into something a computer can do, and finally how to “write” the specific syntax (required by a computer) to get the job done. It is sometimes the case that a machine will solve a problem in a completely different way than a human.
Computer Programmers are problem solvers. In order to solve a problem on a computer, you must:
- Know how to represent the information (data) describing the problem.
- Determine the steps to transform the information from one representation into another.
Computer science can be thought of as the study of algorithms. However, we must be careful to include the fact that some problems may not have a solution. Although proving this statement is beyond the scope of this text, the fact that some problems cannot be solved is important for those who study computer science. We can fully define computer science, then, by including both types of problems and stating that computer science is the study of solutions to problems as well as the study of problems with no solutions.
It is also very common to include the word computable when describing problems and solutions. We say that a problem is computable if an algorithm exists for solving it. An alternative definition for computer science, then, is to say that computer science is the study of problems that are and that are not computable, the study of the existence and the non-existence of algorithms. In any case, you will note that the word “computer” did not come up at all. Solutions are considered independent of the machine.
Computer science, as it pertains to the problem-solving process itself, is also the study of abstraction . Abstraction allows us to view the problem and solution in such a way as to separate the so-called logical and physical perspectives.
Steps involved in problem-solving
- Analysing the problem
- Developing the algorithm
- Testing and debugging
Before we are ready to solve a problem, we must look over the question and understand it. By analysing it, we can figure out the outputs and inputs to be carried out. If we are not ready with what we have to solve, then we end up with the question and cannot find the answer as expected.
It is required to decide a solution before writing a program. The representation of solution in a natural language, called an algorithm. We must design, develop and decide the final approach after a number of trials and errors. Developing an algorithm captures and refines all the aspects of the desired solution.
Once we finalize the algorithm, we must convert the decided algorithm into a code. The code can be written in dedicated programming languages. So, that is understandable by the computer to find a desired solution. In this stage, a wide variety of programming language are used to convert the algorithm into code.
Designed and developed program undergoes several rigorous tests based on various real-time parameters. Industries and many companies follow some testing methods like system testing, component testing and acceptance testing. It must meet the user’s requirements, which have to respond with the required time and generate all expected outputs.
Problem solving flow
[ Right mindset ] --> [ Making right decisions ] - -> [ Keeping ideas on track ] --> [ Learning from feedbacks ] --> [ Asking questions ]
The way to approach problems is the key to improving the skills. To find a solution, a positive mindset helps to solve problems quickly . When we need to solve a problem, we must be clear with a solution. The perfect solution helps to get success in a shorter period. Ideas always help much in improving the skills. They also help to gain more knowledge and more command over things. A crucial part of learning is from the feedback. Mistakes help you gain more knowledge and have much growth. Questions are an incredible part of life. While searching for solutions, there are a lot of questions that arise in our minds.
Computer Programming is a step-by-step process of designing and developing various sets of computer programs to accomplish a specific computing outcome. The process comprises several tasks like analysis, coding, algorithm generation, checking accuracy and resource consumption of algorithms, etc. The purpose of computer programming is to find a sequence of instructions that solve a specific problem on a computer.
English is the most popular and well-known Human Language. The English language has its own set of grammar rules, which has to be followed to write in the English language correctly. Likewise, any other Human Languages (German, Spanish, Russian, etc.) are made of several elements like nouns, adjective, adverbs, propositions, and conjunctions, etc. So, just like English, Spanish or other human languages, programming languages are also made of different elements.
Just like human languages, programming languages also follow grammar called syntax . There are certain basic program code elements which are common for all the programming languages.
Computer programming is a set of written instructions that the computer follows. These instructions can be written in various languages. Each programming languages have their unique ways of organizing the commands, which are called syntax.
Multiple programming languages can help you solve the same programming problem. However, you need to select a language that you feel is relevant to perform your task. If you decide that a language does not suit your business requirement, you can always move on to a new language. Your skill in the chosen language will also be a deciding factor. Expected software system response time, a number of simultaneous users, security, maintains, compatibility with web, mobile, devices are few other factors to consider while choosing a language.
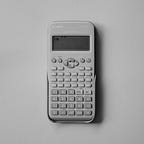
Written by Spirit of Performance 👻
Medium Corporation employees’ median income is US$221,563 per person per year. Medium founded to extend Twitter abilities. Twitter then sold for US$44 billion.
Text to speech
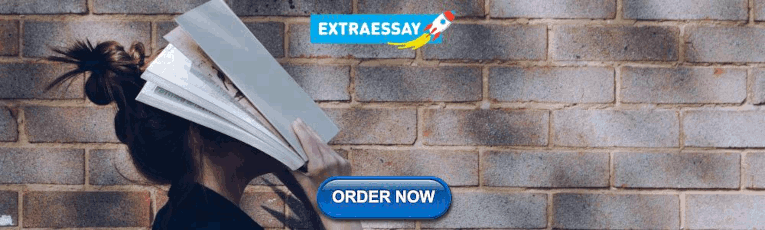
IMAGES
VIDEO
COMMENTS
So, computer science is all about taking in information and then performing some computations & analysis to solve a particular problem or produce a desired result, which depends on the application at hand. Computer science is similar to mathematics in that both are used as a means of defining and solving some problem. In fact, computer-based ...
How to think like a programmer — lessons in problem solving
Redefine the problem. 4. Relax one assumption at a time from the problem statement (to make the problem easier) Many problems are too hard to solve on their own. However, by solving a easier ...
Computational Thinking for Problem Solving
CS2104: Introduction to Problem Solving in Computer Science. This course introduces the student to a broad range of heuristics for solving problems in a range of settings. Emphasis on problem-solving techniques that aid programmers and computer scientists. Heuristics for solving problems ''in the small'' (classical math and word problems ...
What is Problem Solving? An Introduction - HackerRank Blog
Problem Solving Using Computational Thinking
3.2 Computer Science Fundamentals. Wrap your mind around computational thinking, from everyday tasks to algorithms. Computers use decision trees to turn many simple decisions into one big decision. Sometimes, the right way to solve a computational problem is by "brute force.". When Pierre the baker wants to get lots of things done, it helps ...
MIT OpenCourseWare is a web based publication of virtually all MIT course content. OCW is open and available to the world and is a permanent MIT activity
#Step 1 — Take time to understand the problem. The first step to solving any problem is to understand the problem being solved. This means you're able to articulate the problem fully in your own words. Don't get disheartened if you can't, it's part of the process of understanding.
Solving problems is the core of computer science. Programmers must first understand how a human solves a problem, then understand how to translate this "algorithm" into something a computer can do, and finally how to "write" the specific syntax (required by a computer) to get the job done. It is sometimes the case that a machine will solve a ...
One of the most important skills you learn in your computer science courses is how to problem solve. Although we cover some general problem solving paradigms in class, the best way to improve these skills is to get practice, practice, and more practice. ... Remember: problem solving is a creative process, which cannot be forced. Don't get ...
The centrality of the ability to solve a problem in computer science is widely delivered by schools and academic institutions which attempt to evaluate individuals' problem-solving skills as a measure of success in learning computer science (e.g., Lishinski et al. 2016). However, whereas the teaching of programming languages is usually well ...
I -- Catalogue Description. This course introduces the student to a broad range of heuristics for solving problems in a range of settings that are relevant to computation. Emphasis on problem-solving techniques that aid programmers and computer scientists. Heuristics for solving problems "in the small" (classical math and word problems ...
In the realm of computer science and beyond, everything revolves around problem-solving, and algorithms are our most reliable problem-solving tools. They provide a structured approach to problem-solving, breaking down complex problems into manageable steps and ensuring that every eventuality is accounted for.
Problem-solving skills are an ability that must be cultivated to equip students with the skills needed to deal with today's increasingly complex and volatile environment. ... He emphasizes the stages of the algorithm which correspond to the process of problem solving. In computer science, the term algorithm refers to a collection of ...
There are many problem-solving skills involved in computer science, including those needed to design, develop, and debug software. Computational thinking is a way of describing these skills. Computational thinking refers to the thought processes involved in defining a problem and its solution so that the solution can be expertly carried out by ...
What is Computational Thinking?
Below, we explore the top "soft skills" that computer scientists should obtain. Critical Thinking: Being able to identify a problem, analyze the details of the situation, and then formulate an effective solution is an incredibly important aspect of computer science work. Attention to Detail: Effective computer scientists must be able to pay ...
Apply Creative Problem Solving to Other Areas. Let's start by explaining why the creative problem-solving skills you'll learn in computer science can help you in everyday life: Novel solutions and new products. Being familiar with creating and polishing hardware and/or software can help you come up with ingenious solutions for your everyday ...
ABSTRACT. Background: Recent developments in STEM and computer science education put a strong emphasis on twenty-first-century skills, such as solving authentic problems. These skills typically transcend single disciplines. Thus, problem-solving must be seen as a multidisciplinary challenge, and the corresponding practices and processes need to be described using an integrated framework.
Soft skills in computer science. Soft skills help make a resumé more well rounded, adding to the overall picture that prospective employers see when considering a candidate. The following skills are examples of the types of skills many employers find valuable in IT and beyond. Problem-solving. Problem-solving is an essential skill for working ...
Apr 8, 2022. --. Computer science is the study of problems, problem-solving, and the solutions that come out of the problem-solving process. Given a problem, a computer scientist's goal is to develop an algorithm, a step-by-step list of instructions for solving any instance of the problem that might arise. Algorithms are finite processes that ...
Collaborative problem solving (CPS) is a critical competency for the modern workforce, as many of todays' problems require groups to come together to find innovative solutions to complex problems. This has motivated increased interest in work dedicated to assessing and developing CPS skills. However, there has been limited attention in prior CPS assessment research on potential differences in ...