IEEE Account
- Change Username/Password
- Update Address
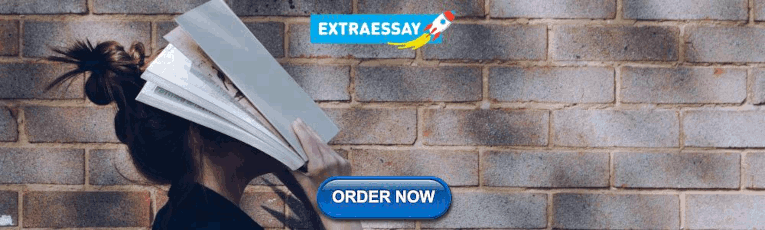
Purchase Details
- Payment Options
- Order History
- View Purchased Documents
Profile Information
- Communications Preferences
- Profession and Education
- Technical Interests
- US & Canada: +1 800 678 4333
- Worldwide: +1 732 981 0060
- Contact & Support
- About IEEE Xplore
- Accessibility
- Terms of Use
- Nondiscrimination Policy
- Privacy & Opting Out of Cookies
A not-for-profit organization, IEEE is the world's largest technical professional organization dedicated to advancing technology for the benefit of humanity. © Copyright 2024 IEEE - All rights reserved. Use of this web site signifies your agreement to the terms and conditions.
Academia.edu no longer supports Internet Explorer.
To browse Academia.edu and the wider internet faster and more securely, please take a few seconds to upgrade your browser .
Enter the email address you signed up with and we'll email you a reset link.
- We're Hiring!
- Help Center
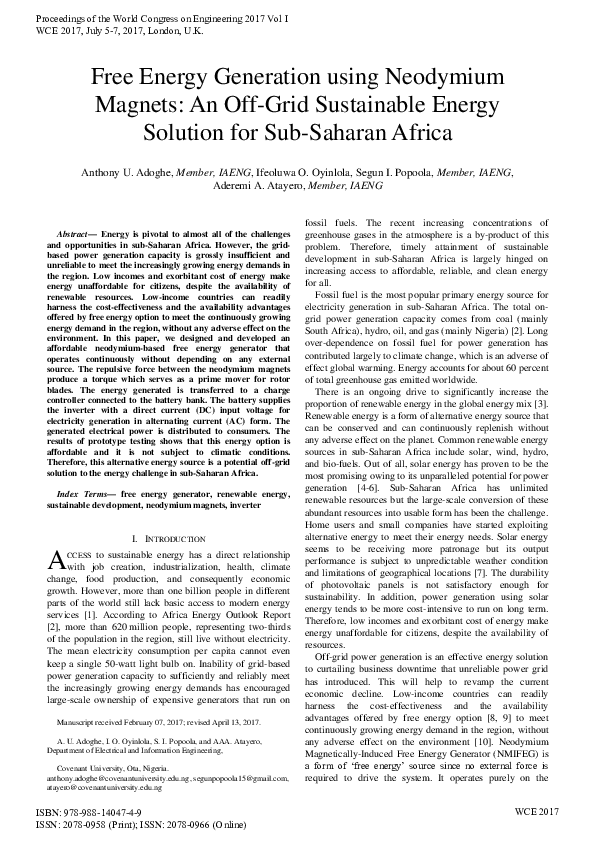
Free Energy Generation using Neodymium Magnets: An Off-Grid Sustainable Energy Solution for Sub-Saharan Africa

Energy is pivotal to almost all of the challenges and opportunities in sub-Saharan Africa. However, the grid-based power generation capacity is grossly insufficient and unreliable to meet the increasingly growing energy demands in the region. Low incomes and exorbitant cost of energy make energy unaffordable for citizens, despite the availability of renewable resources. Low-income countries can readily harness the cost-effectiveness and the availability advantages offered by free energy option to meet the continuously growing energy demand in the region, without any adverse effect on the environment. In this paper, we designed and developed an affordable neodymium-based free energy generator that operates continuously without depending on any external source. The repulsive force between the neodymium magnets produce a torque which serves as a prime mover for rotor blades. The energy generated is transferred to a charge controller connected to the battery bank. The battery supplies t...
Related Papers
Journal of Reasearch in Engineering and Applied Sciences
SURAJ AMBULKAR
Energy has become and plays the most significant part of a human's life. The term energy referred here is for electrical energy. Human is almost completely dependent on energy for his various purposes. Today there are several ways of generating electricity as per the requirement using several kinds of fuel like nuclear fuel, coal, gas, or hydro etc. But all these have their own dire consequences and detrimental effects on the environment, some even worst to have radiation threat if leaked. So as per the current scenario, there is a need to develop such a method which will generate electricity that will not be only eco-friendly but also cheap. This paper deals with the conceptual design of recent research going on developing such a technique that will not only give eco-friendly but also a cheap form of electrical energy. The mechanism and technique incorporate the application of magnets (Neodymium magnet) and its repulsive property of magnetic force used for generation of continu...
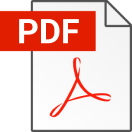
International Journal IJRITCC , Prof. Parag G Shewane
Neodymium (NdFeB) magnets have become most popular magnets in recent years and replaced. More advantages over the other types of magnet in many applications in modern products that require strong permanent magnets, such as motors in cordless tools, hard disk drives and magnetic fasteners. Neodymium magnets can be used to invent a new method of energy generation by using the magnetic field of magnet and convert the magnetic energy into kinetic energy without using any kind of fuel and overcoming the energy generation problem such as building a magnetic turbine. The main objective of the study was to study about the advantage of using NdFeB magnets over normal magnets, nature of different type of neodymium magnets and how it can be used to convert magnetic energy into kinetic energy.
IJERA Journal
In this paper construction of universal neodymium permanent magnetic rotor without any electromagnetic core, due to this we will reduce 38% of core losses and increase the stability of the system efficiency. Now a day's electricity generation from various sources like hydro, steam, solar, nuclear etc. but these sources have some merits & demerits. By these sources have more demerits as well as its have more losses, so we not fulfill the costumer/industrial requirements. For alternate arrangement we have been innovated this system. Keywords: 24 gauge copper wire, universal rotor (armature), Neodymium Permanent Magnets, 12V DC Generator, 12/20 cm wooden disk or plastic/non magnetic disk.
Albert Patrick David
The study for the search of renewable sources of energyis now a major concern worldwide as replacement to the high demand of fossil fuels. Majority of the electricity that is generated uses the Faraday's law, the electromagnetic induction. This law led to new technologies that even brought up the misconception of free energy. Energy only becomes free if we don't have to pay for the generation of it; hence we resort to abundant sources of energy that we can convert into electricity. The researcher used wind to generate power. It will continuously pass the blades of the fan causing it to rotate. The generator is mounted into a vehicle exposing the blades into turbulent wind, rotating the shaft to generate power and, is extracted and stored into a battery. The process also demonstrates the conversion of kinetic energy from the wind into mechanical power. Results on different settings are compared to identify the best scenario that will generate usable amount of energy and adjustments on the design of the prototype to meet the needs of the end users. The energy generated can be used in numerous applications such as powering and charging mobile devices, powering small light emitting diodes and bulbs. This study focuses on the construction of the generator and evaluating the device to identify its possible applicationsand future enhancements. This prototype may impact and attract future researchers to work more on the research of free energy or renewable energy.
Kashif Khan
IJSRP Journal
More than 90% world’s power is being generated using electromagnets based on the faraday’s law of electro-magnetic induction. Many new technologies were discovered with time which led a drastic change in the perception of electric energy. But at the same time there is misconception of FREE ENERGY. Energy becomes free only at a point after which we don’t have to pay for power generation after commissioning the unit. By using the magnetic force of magnets continuous motion (Energy) is generated.
International Journal of Scientific Research in Science, Engineering and Technology
International Journal of Scientific Research in Science, Engineering and Technology IJSRSET
Electricity is one of the most significant gifts that science has bestowed upon humanity. It has also become an integral part of modern life such that it is difficult to imagine a world without it. Electricity has numerous applications in our daily lives. Energy is usually produced by non-renewable sources such as petrol, Kerosene and nuclear which unfortunately create pollution. These methods are inconvenient in many ways. Burning of non renewable sources are hazardous to environment as it produces harmful gases. The batteries which are used to produce electricity is also manufactured using harmful substaces. Using high pressure steam and batteries are expensive and hard to maintain. The intention of this project is to producing energy without creating pollution and use it to power light bulbs, cell phones, laptops, and other small appliances. This project is great help to develop our engineering skills while learning about a clean way of generating electricity and satisfying our basic requirement. We are going to use the hard drive,magnet and inductive coil to generate electricity due to which our mobile phone will be charge and followed by ac to dc converter. This is totally clean way of generating energy. As fuel is not a renewable energy source and the prices are increasing day by day. It will not be affordable by a common man after some period. Here no fuel is required to generate electricity, so everybody can afford this method for power generation also it eliminates the emission of CO2 which will reduces the pollution. Conventional methods for generating electricity make use of dynamo and wind turbine, but they have disadvantage that they produce friction and reduces speed which require more efforts For the project to work we need strong electromagnets so we have used Neodymium magnets and also used.
Peter Egolf
International Journal of Research in Engineering and Technology
Ismail Khan
International Journal of Science Technology & Engineering
IJSTE - International Journal of Science Technology and Engineering
Imagine a motor that is propelled by magnets only. No electricity in; no petrol in; just torque out, to be used to turn a generator or a driveshaft. Science does not yet have models to describe how this works, largely because the scientific community at large does not believe it is possible. Notwithstanding academic snubbing, many thousands of individuals have chased such a dream, and some claim to have achieved eureka. Of all the free energy technologies, from solar and wind, to cold fusion and zero point energy, the magnet motor is probably the sexiest. There it is spinning away, in violation of known laws of physics, creating useful energy. What you have is a motor that you could plunk down just about anywhere (environmental conditions/protection depending), and it will run continuously, with no visible energy input, needing only occasional maintenance of the bearings and such. Heat is the enemy for such a system, because at higher temperatures, the magnetism of the magnets is lost. A magnetic motor (or magnet motor) is a device which converts power of or relating to or caused by magnetism (e.g., "magnetic forces") into mechanical force and motion, with no other input. It usually provides rotary mechanical motion. The machines that utilizes the properties of a magnet for mechanical energy.
Loading Preview
Sorry, preview is currently unavailable. You can download the paper by clicking the button above.
RELATED PAPERS
Computers & Structures
Jayesh Sodhani
Journal of Southwest Jiaotong University
Shabrina P U T R I Chairandy
YOGENDRAKUMAR LAHIR
Nano Energy
haotian chen
International journal of scientific research
Piyusha Sangave
International Journal of Innovations in Engineering and Science, ISSN:2456-3463 IJIES
International Journal of Non-linear Mechanics
Stefano Lenci
International Journal of Engineering Research and Technology (IJERT)
IJERT Journal
Peter Aspegren
nternational journal of advanced research in computer and communication engineering
Itika Tandon
IEEE Transactions on Energy Conversion
Paul Barendse
Marcin Szczygieł
Journal of Engineering and Technology
Mussa Mgwatu
Journal of Materials Engineering
Jayant Vaidya
Camelia Petrescu
IOSR Journals
2014 IEEE International Instrumentation and Measurement Technology Conference (I2MTC) Proceedings
Cleonilson Souza
IEEE Access
javad enayati
Journal of emerging technologies and innovative research
Shivam Dubey
Tresa Harsha P George
SANKARAN NAMPOOTHIRI KRISHNAN
Latin American Journal of Energy Research
eduarda carvalho
IECON 2007 - 33rd Annual Conference of the IEEE Industrial Electronics Society
David Dorrell
RELATED TOPICS
- We're Hiring!
- Help Center
- Find new research papers in:
- Health Sciences
- Earth Sciences
- Cognitive Science
- Mathematics
- Computer Science
- Academia ©2024
Electro-Magnetic Induction: Free Electricity Generator
15 Pages Posted: 26 Nov 2019
Albert Patrick David
Bulacan State University
Date Written: May 18, 2017
The study for the search of renewable sources of energy is now a major concern worldwide as replacement to the high demand of fossil fuels. Majority of the electricity that is generated uses the Faraday's law, the electromagnetic induction. This law led to new technologies that even brought up the misconception of free energy. Energy only becomes free if we don't have to pay for the generation of it; hence we resort to abundant sources of energy that we can convert into electricity. The researcher used wind to generate power. It will continuously pass the blades of the fan causing it to rotate. The generator is mounted into a vehicle exposing the blades into turbulent wind, rotating the shaft to generate power and, is extracted and stored into a battery. The process also demonstrates the conversion of kinetic energy from the wind into mechanical power. Results on different settings are compared to identify the best scenario that will generate usable amount of energy and adjustments on the design of the prototype to meet the needs of the end users. The energy generated can be used in numerous applications such as powering and charging mobile devices, powering small light emitting diodes and bulbs. This study focuses on the construction of the generator and evaluating the device to identify its possible applications and future enhancements. This prototype may impact and attract future researchers to work more on the research of free energy or renewable energy.
Keywords: electro-magnetic induction, wind energy, electricity, renewable energy
Suggested Citation: Suggested Citation
Albert Patrick David (Contact Author)
Bulacan state university ( email ).
Brgy. Guinhawa Malolos City, Bulacan 3000 Philippines +63 44 919 7800 (Phone)
HOME PAGE: http://https://bulsu.edu.ph
Do you have a job opening that you would like to promote on SSRN?
Paper statistics, related ejournals, economics of innovation ejournal.
Subscribe to this fee journal for more curated articles on this topic
Energy Engineering eJournal
Mechanical engineering ejournal, energy ejournal, materials for energy ejournal, computational materials science ejournal.
Thank you for visiting nature.com. You are using a browser version with limited support for CSS. To obtain the best experience, we recommend you use a more up to date browser (or turn off compatibility mode in Internet Explorer). In the meantime, to ensure continued support, we are displaying the site without styles and JavaScript.
- View all journals
- Explore content
- About the journal
- Publish with us
- Sign up for alerts
- Review Article
- Published: 13 January 2010
The free-energy principle: a unified brain theory?
- Karl Friston 1
Nature Reviews Neuroscience volume 11 , pages 127–138 ( 2010 ) Cite this article
77k Accesses
3877 Citations
666 Altmetric
Metrics details
- Control theory
- Neural encoding
Adaptive agents must occupy a limited repertoire of states and therefore minimize the long-term average of surprise associated with sensory exchanges with the world. Minimizing surprise enables them to resist a natural tendency to disorder.
Surprise rests on predictions about sensations, which depend on an internal generative model of the world. Although surprise cannot be measured directly, a free-energy bound on surprise can be, suggesting that agents minimize free energy by changing their predictions (perception) or by changing the predicted sensory inputs (action).
Perception optimizes predictions by minimizing free energy with respect to synaptic activity (perceptual inference), efficacy (learning and memory) and gain (attention and salience). This furnishes Bayes-optimal (probabilistic) representations of what caused sensations (providing a link to the Bayesian brain hypothesis).
Bayes-optimal perception is mathematically equivalent to predictive coding and maximizing the mutual information between sensations and the representations of their causes. This is a probabilistic generalization of the principle of efficient coding (the infomax principle) or the minimum-redundancy principle.
Learning under the free-energy principle can be formulated in terms of optimizing the connection strengths in hierarchical models of the sensorium. This rests on associative plasticity to encode causal regularities and appeals to the same synaptic mechanisms as those underlying cell assembly formation.
Action under the free-energy principle reduces to suppressing sensory prediction errors that depend on predicted (expected or desired) movement trajectories. This provides a simple account of motor control, in which action is enslaved by perceptual (proprioceptive) predictions.
Perceptual predictions rest on prior expectations about the trajectory or movement through the agent's state space. These priors can be acquired (as empirical priors during hierarchical inference) or they can be innate (epigenetic) and therefore subject to selective pressure.
Predicted motion or state transitions realized by action correspond to policies in optimal control theory and reinforcement learning. In this context, value is inversely proportional to surprise (and implicitly free energy), and rewards correspond to innate priors that constrain policies.
A free-energy principle has been proposed recently that accounts for action, perception and learning. This Review looks at some key brain theories in the biological (for example, neural Darwinism) and physical (for example, information theory and optimal control theory) sciences from the free-energy perspective. Crucially, one key theme runs through each of these theories — optimization. Furthermore, if we look closely at what is optimized, the same quantity keeps emerging, namely value (expected reward, expected utility) or its complement, surprise (prediction error, expected cost). This is the quantity that is optimized under the free-energy principle, which suggests that several global brain theories might be unified within a free-energy framework.
This is a preview of subscription content, access via your institution
Access options
Subscribe to this journal
Receive 12 print issues and online access
176,64 € per year
only 14,72 € per issue
Buy this article
- Purchase on SpringerLink
- Instant access to full article PDF
Prices may be subject to local taxes which are calculated during checkout
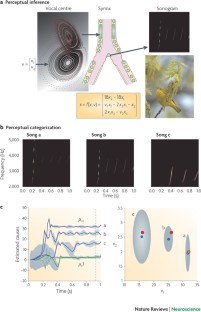
Similar content being viewed by others
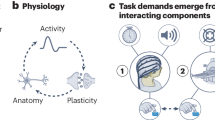
Centering cognitive neuroscience on task demands and generalization
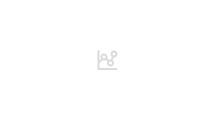
A brief history of heuristics: how did research on heuristics evolve?
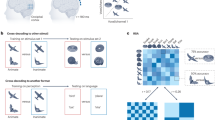
Testing cognitive theories with multivariate pattern analysis of neuroimaging data
Huang, G. Is this a unified theory of the brain? New Scientist 2658 , 30–33 (2008).
Article Google Scholar
Friston K., Kilner, J. & Harrison, L. A free energy principle for the brain. J. Physiol. Paris 100 , 70–87 (2006). An overview of the free-energy principle that describes its motivation and relationship to generative models and predictive coding. This paper focuses on perception and the neurobiological infrastructures involved.
Article PubMed Google Scholar
Ashby, W. R. Principles of the self-organising dynamic system. J. Gen. Psychol. 37 , 125–128 (1947).
Article CAS PubMed Google Scholar
Nicolis, G. & Prigogine, I. Self-Organisation in Non-Equilibrium Systems (Wiley, New York, 1977).
Google Scholar
Haken, H. Synergistics: an Introduction. Non-Equilibrium Phase Transition and Self-Organisation in Physics, Chemistry and Biology 3rd edn (Springer, New York, 1983).
Book Google Scholar
Kauffman, S. The Origins of Order: Self-Organization and Selection in Evolution (Oxford Univ. Press, Oxford, 1993).
Bernard, C. Lectures on the Phenomena Common to Animals and Plants (Thomas, Springfield, 1974).
Applebaum, D. Probability and Information: an Integrated Approach (Cambridge Univ. Press, Cambridge, UK, 2008).
Evans, D. J. A non-equilibrium free energy theorem for deterministic systems. Mol. Physics 101 , 15551–11554 (2003).
Article CAS Google Scholar
Crauel, H. & Flandoli, F. Attractors for random dynamical systems. Probab. Theory Relat. Fields 100 , 365–393 (1994).
Feynman, R. P. Statistical Mechanics: a Set of Lectures (Benjamin, Reading, Massachusetts, 1972).
Hinton, G. E. & von Cramp, D. Keeping neural networks simple by minimising the description length of weights. Proc. 6th Annu. ACM Conf. Computational Learning Theory 5–13 (1993).
MacKay. D. J. C. Free-energy minimisation algorithm for decoding and cryptoanalysis. Electron. Lett. 31 , 445–447 (1995).
Neal, R. M. & Hinton, G. E. in Learning in Graphical Models (ed. Jordan, M. I.) 355–368 (Kluwer Academic, Dordrecht, 1998).
Itti, L. & Baldi, P. Bayesian surprise attracts human attention. Vision Res. 49 , 1295–1306 (2009).
Friston, K., Daunizeau, J. & Kiebel, S. Active inference or reinforcement learning? PLoS ONE 4 , e6421 (2009).
Article PubMed PubMed Central CAS Google Scholar
Knill, D. C. & Pouget, A. The Bayesian brain: the role of uncertainty in neural coding and computation. Trends Neurosci. 27 , 712–719 (2004). A nice review of Bayesian theories of perception and sensorimotor control. Its focus is on Bayes optimality in the brain and the implicit nature of neuronal representations.
von Helmholtz, H. in Treatise on Physiological Optics Vol. III 3rd edn (Voss, Hamburg, 1909).
MacKay, D. M. in Automata Studies (eds Shannon, C. E. & McCarthy, J.) 235–251 (Princeton Univ. Press, Princeton, 1956).
Neisser, U. Cognitive Psychology (Appleton-Century-Crofts, New York, 1967).
Gregory, R. L. Perceptual illusions and brain models. Proc. R. Soc. Lond. B Biol. Sci. 171 , 179–196 (1968).
Gregory, R. L. Perceptions as hypotheses. Philos. Trans. R. Soc. Lond. B Biol. Sci. 290 , 181–197 (1980).
Ballard, D. H., Hinton, G. E. & Sejnowski, T. J. Parallel visual computation. Nature 306 , 21–26 (1983).
Kawato, M., Hayakawa, H. & Inui, T. A forward-inverse optics model of reciprocal connections between visual areas. Network: Computation in Neural Systems 4 , 415–422 (1993).
Dayan, P., Hinton, G. E. & Neal, R. M. The Helmholtz machine. Neural Comput. 7 , 889–904 (1995). This paper introduces the central role of generative models and variational approaches to hierarchical self-supervised learning and relates this to the function of bottom-up and top-down cortical processing pathways.
Lee, T. S. & Mumford, D. Hierarchical Bayesian inference in the visual cortex. J. Opt. Soc. Am. A Opt. Image Sci. Vis. 20 , 1434–1448 (2003).
Kersten, D., Mamassian, P. & Yuille, A. Object perception as Bayesian inference. Annu. Rev. Psychol. 55 , 271–304 (2004).
Friston, K. J. A theory of cortical responses. Philos. Trans. R. Soc. Lond. B Biol. Sci. 360 , 815–836 (2005).
Article PubMed PubMed Central Google Scholar
Beal, M. J. Variational Algorithms for Approximate Bayesian Inference . Thesis, University College London (2003).
Efron, B. & Morris, C. Stein's estimation rule and its competitors – an empirical Bayes approach. J. Am. Stats. Assoc. 68 , 117–130 (1973).
Kass, R. E. & Steffey, D. Approximate Bayesian inference in conditionally independent hierarchical models (parametric empirical Bayes models). J. Am. Stat. Assoc. 407 , 717–726 (1989).
Zeki, S. & Shipp, S. The functional logic of cortical connections. Nature 335 , 311–317 (1988). Describes the functional architecture of cortical hierarchies with a focus on patterns of anatomical connections in the visual cortex. It emphasizes the role of functional segregation and integration (that is, message passing among cortical areas).
Felleman, D. J. & Van Essen, D. C. Distributed hierarchical processing in the primate cerebral cortex. Cereb. Cortex 1 , 1–47 (1991).
Mesulam, M. M. From sensation to cognition. Brain 121 , 1013–1052 (1998).
Sanger, T. Probability density estimation for the interpretation of neural population codes. J. Neurophysiol. 76 , 2790–2793 (1996).
Zemel, R., Dayan, P. & Pouget, A. Probabilistic interpretation of population code. Neural Comput. 10 , 403–430 (1998).
Paulin, M. G. Evolution of the cerebellum as a neuronal machine for Bayesian state estimation. J. Neural Eng. 2 , S219–S234 (2005).
Ma, W. J., Beck, J. M., Latham, P. E. & Pouget, A. Bayesian inference with probabilistic population codes. Nature Neurosci. 9 , 1432–1438 (2006).
Friston, K., Mattout, J., Trujillo-Barreto, N., Ashburner, J. & Penny, W. Variational free energy and the Laplace approximation. Neuroimage 34 , 220–234 (2007).
Rao, R. P. & Ballard, D. H. Predictive coding in the visual cortex: a functional interpretation of some extra-classical receptive field effects. Nature Neurosci. 2 , 79–87 (1998). Applies predictive coding to cortical processing to provide a compelling account of extra-classical receptive fields in the visual system. It emphasizes the importance of top-down projections in providing predictions, by modelling perceptual inference.
Mumford, D. On the computational architecture of the neocortex. II. The role of cortico-cortical loops. Biol. Cybern. 66 , 241–251 (1992).
Friston, K. Hierarchical models in the brain. PLoS Comput. Biol. 4 , e1000211 (2008).
Murray, S. O., Kersten, D., Olshausen, B. A., Schrater, P. & Woods, D. L. Shape perception reduces activity in human primary visual cortex. Proc. Natl Acad. Sci. USA 99 , 15164–15169 (2002).
Article CAS PubMed PubMed Central Google Scholar
Garrido, M. I., Kilner, J. M., Kiebel, S. J. & Friston, K. J. Dynamic causal modeling of the response to frequency deviants. J. Neurophysiol. 101 , 2620–2631 (2009).
Sherman, S. M. & Guillery, R. W. On the actions that one nerve cell can have on another: distinguishing “drivers” from “modulators”. Proc. Natl Acad. Sci. USA 95 , 7121–7126 (1998).
Angelucci, A. & Bressloff, P. C. Contribution of feedforward, lateral and feedback connections to the classical receptive field center and extra-classical receptive field surround of primate V1 neurons. Prog. Brain Res. 154 , 93–120 (2006).
Grossberg, S. Towards a unified theory of neocortex: laminar cortical circuits for vision and cognition. Prog. Brain Res. 165 , 79–104 (2007).
Grossberg, S. & Versace, M. Spikes, synchrony, and attentive learning by laminar thalamocortical circuits. Brain Res. 1218 , 278–312 (2008).
Barlow, H. in Sensory Communication (ed. Rosenblith, W.) 217–234 (MIT Press, Cambridge, Massachusetts, 1961).
Linsker, R. Perceptual neural organisation: some approaches based on network models and information theory. Annu. Rev. Neurosci. 13 , 257–281 (1990).
Oja, E. Neural networks, principal components, and subspaces. Int. J. Neural Syst. 1 , 61–68 (1989).
Bell, A. J. & Sejnowski, T. J. An information maximisation approach to blind separation and blind de-convolution. Neural Comput. 7 , 1129–1159 (1995).
Atick, J. J. & Redlich, A. N. What does the retina know about natural scenes? Neural Comput. 4 , 196–210 (1992).
Optican, L. & Richmond, B. J. Temporal encoding of two-dimensional patterns by single units in primate inferior cortex. III Information theoretic analysis. J. Neurophysiol. 57 , 132–146 (1987).
Olshausen, B. A. & Field, D. J. Emergence of simple-cell receptive field properties by learning a sparse code for natural images. Nature 381 , 607–609 (1996).
Simoncelli, E. P. & Olshausen, B. A. Natural image statistics and neural representation. Annu. Rev. Neurosci. 24 , 1193–1216 (2001). A nice review of information theory in visual processing. It covers natural scene statistics and empirical tests of the efficient coding hypothesis in individual neurons and populations of neurons.
Friston, K. J. The labile brain. III. Transients and spatio-temporal receptive fields. Philos. Trans. R. Soc. Lond. B Biol. Sci. 355 , 253–265 (2000).
Bialek, W., Nemenman, I. & Tishby, N. Predictability, complexity, and learning. Neural Comput. 13 , 2409–2463 (2001).
Lewen, G. D., Bialek, W. & de Ruyter van Steveninck, R. R. Neural coding of naturalistic motion stimuli. Network 12 , 317–329 (2001).
Laughlin, S. B. Efficiency and complexity in neural coding. Novartis Found. Symp. 239 , 177–187 (2001).
CAS PubMed Google Scholar
Tipping, M. E. Sparse Bayesian learning and the Relevance Vector Machine. J. Machine Learn. Res. 1 , 211–244 (2001).
Paus, T., Keshavan, M. & Giedd, J. N. Why do many psychiatric disorders emerge during adolescence? Nature Rev. Neurosci. 9 , 947–957 (2008).
Gilestro, G. F., Tononi, G. & Cirelli, C. Widespread changes in synaptic markers as a function of sleep and wakefulness in Drosophila . Science 324 , 109–112 (2009).
Roweis, S. & Ghahramani, Z. A unifying review of linear Gaussian models. Neural Comput. 11 , 305–345 (1999).
Hebb, D. O. The Organization of Behaviour (Wiley, New York, 1949).
Paulsen, O. & Sejnowski, T. J. Natural patterns of activity and long-term synaptic plasticity. Curr. Opin. Neurobiol. 10 , 172–179 (2000).
von der Malsburg, C. The Correlation Theory of Brain Function . Internal Report 81–82, Dept. Neurobiology, Max-Planck-Institute for Biophysical Chemistry (1981).
Singer, W. & Gray, C. M. Visual feature integration and the temporal correlation hypothesis. Annu. Rev. Neurosci. 18 , 555–586 (1995).
Bienenstock, E. L., Cooper, L. N. & Munro, P. W. Theory for the development of neuron selectivity: orientation specificity and binocular interaction in visual cortex. J. Neurosci. 2 , 32–48 (1982).
Abraham, W. C. & Bear, M. F. Metaplasticity: the plasticity of synaptic plasticity. Trends Neurosci. 19 , 126–130 (1996).
Pareti, G. & De Palma, A. Does the brain oscillate? The dispute on neuronal synchronization. Neurol. Sci. 25 , 41–47 (2004).
Leutgeb, S., Leutgeb, J. K., Moser, M. B. & Moser, E. I. Place cells, spatial maps and the population code for memory. Curr. Opin. Neurobiol. 15 , 738–746 (2005).
Durstewitz, D. & Seamans, J. K. Beyond bistability: biophysics and temporal dynamics of working memory. Neuroscience 139 , 119–133 (2006).
Anishchenko, A. & Treves, A. Autoassociative memory retrieval and spontaneous activity bumps in small-world networks of integrate-and-fire neurons. J. Physiol. Paris 100 , 225–236 (2006).
Abbott, L. F., Varela, J. A., Sen, K. & Nelson, S. B. Synaptic depression and cortical gain control. Science 275 , 220–224 (1997).
Yu, A. J. & Dayan, P. Uncertainty, neuromodulation and attention. Neuron 46 , 681–692 (2005).
Doya, K. Metalearning and neuromodulation. Neural Netw. 15 , 495–506 (2002).
Chawla, D., Lumer, E. D. & Friston, K. J. The relationship between synchronization among neuronal populations and their mean activity levels. Neural Comput. 11 , 1389–1411 (1999).
Fries, P., Womelsdorf, T., Oostenveld, R. & Desimone, R. The effects of visual stimulation and selective visual attention on rhythmic neuronal synchronization in macaque area V4. J. Neurosci. 28 , 4823–4835 (2008).
Womelsdorf, T. & Fries, P. Neuronal coherence during selective attentional processing and sensory-motor integration. J. Physiol. Paris 100 , 182–193 (2006).
Desimone, R. Neural mechanisms for visual memory and their role in attention. Proc. Natl Acad. Sci. USA 93 , 13494–13499 (1996). A nice review of mnemonic effects (such as repetition suppression) on neuronal responses and how they bias the competitive interactions between stimulus representations in the cortex. It provides a good perspective on attentional mechanisms in the visual system that is empirically grounded.
Treisman, A. Feature binding, attention and object perception. Philos. Trans. R. Soc. Lond. B Biol. Sci. 353 , 1295–1306 (1998).
Maunsell, J. H. & Treue, S. Feature-based attention in visual cortex. Trends Neurosci. 29 , 317–322 (2006).
Spratling, M. W. Predictive-coding as a model of biased competition in visual attention. Vision Res. 48 , 1391–1408 (2008).
Reynolds, J. H. & Heeger, D. J. The normalization model of attention. Neuron 61 , 168–185 (2009).
Schroeder, C. E., Mehta, A. D. & Foxe, J. J. Determinants and mechanisms of attentional modulation of neural processing. Front. Biosci. 6 , D672–D684 (2001).
Hirayama, J., Yoshimoto, J. & Ishii, S. Bayesian representation learning in the cortex regulated by acetylcholine. Neural Netw. 17 , 1391–1400 (2004).
Edelman, G. M. Neural Darwinism: selection and reentrant signaling in higher brain function. Neuron 10 , 115–125 (1993).
Knobloch, F. Altruism and the hypothesis of meta-selection in human evolution. J. Am. Acad. Psychoanal. 29 , 339–354 (2001).
Friston, K. J., Tononi, G., Reeke, G. N. Jr, Sporns, O. & Edelman, G. M. Value-dependent selection in the brain: simulation in a synthetic neural model. Neuroscience 59 , 229–243 (1994).
Sutton, R. S. & Barto, A. G. Toward a modern theory of adaptive networks: expectation and prediction. Psychol. Rev. 88 , 135–170 (1981).
Montague, P. R., Dayan, P., Person, C. & Sejnowski, T. J. Bee foraging in uncertain environments using predictive Hebbian learning. Nature 377 , 725–728 (1995). A computational treatment of behaviour that combines ideas from optimal control theory and dynamic programming with the neurobiology of reward. This provided an early example of value learning in the brain.
Schultz, W. Predictive reward signal of dopamine neurons. J. Neurophysiol. 80 , 1–27 (1998).
Daw, N. D. & Doya, K. The computational neurobiology of learning and reward. Curr. Opin. Neurobiol. 16 , 199–204 (2006).
Redgrave, P. & Gurney, K. The short-latency dopamine signal: a role in discovering novel actions? Nature Rev. Neurosci. 7 , 967–975 (2006).
Berridge, K. C. The debate over dopamine's role in reward: the case for incentive salience. Psychopharmacology (Berl.) 191 , 391–431 (2007).
Sella, G. & Hirsh, A. E. The application of statistical physics to evolutionary biology. Proc. Natl Acad. Sci. USA 102 , 9541–9546 (2005).
Rescorla, R. A. & Wagner, A. R. in Classical Conditioning II: Current Research and Theory (eds Black, A. H. & Prokasy, W. F.) 64–99 (Appleton Century Crofts, New York, 1972).
Bellman, R. On the Theory of Dynamic Programming. Proc. Natl Acad. Sci. USA 38 , 716–719 (1952).
Watkins, C. J. C. H. & Dayan, P. Q-learning. Mach. Learn. 8 , 279–292 (1992).
Todorov, E. in Advances in Neural Information Processing Systems (eds Scholkopf, B., Platt, J. & Hofmann T.) 19 , 1369–1376 (MIT Press, 2006).
Camerer, C. F. Behavioural studies of strategic thinking in games. Trends Cogn. Sci. 7 , 225–231 (2003).
Smith, J. M. & Price, G. R. The logic of animal conflict. Nature 246 , 15–18 (1973).
Nash, J. Equilibrium points in n-person games. Proc. Natl Acad. Sci. USA 36 , 48–49 (1950).
Wolpert, D. M. & Miall, R. C. Forward models for physiological motor control. Neural Netw. 9 , 1265–1279 (1996).
Todorov, E. & Jordan, M. I. Smoothness maximization along a predefined path accurately predicts the speed profiles of complex arm movements. J. Neurophysiol. 80 , 696–714 (1998).
Tseng, Y. W., Diedrichsen, J., Krakauer, J. W., Shadmehr, R. & Bastian, A. J. Sensory prediction-errors drive cerebellum-dependent adaptation of reaching. J. Neurophysiol. 98 , 54–62 (2007).
Bays, P. M. & Wolpert, D. M. Computational principles of sensorimotor control that minimize uncertainty and variability. J. Physiol. 578 , 387–396 (2007). A nice overview of computational principles in motor control. Its focus is on representing uncertainty and optimal estimation when extracting the sensory information required for motor planning.
Shadmehr, R. & Krakauer, J. W. A computational neuroanatomy for motor control. Exp. Brain Res. 185 , 359–381 (2008).
Verschure, P. F., Voegtlin, T. & Douglas, R. J. Environmentally mediated synergy between perception and behaviour in mobile robots. Nature 425 , 620–624 (2003).
Cohen, J. D., McClure, S. M. & Yu, A. J. Should I stay or should I go? How the human brain manages the trade-off between exploitation and exploration. Philos. Trans. R. Soc. Lond. B Biol. Sci. 362 , 933–942 (2007).
Ishii, S., Yoshida, W. & Yoshimoto, J. Control of exploitation-exploration meta-parameter in reinforcement learning. Neural Netw. 15 , 665–687 (2002).
Usher, M., Cohen, J. D., Servan-Schreiber, D., Rajkowski, J. & Aston-Jones, G. The role of locus coeruleus in the regulation of cognitive performance. Science 283 , 549–554 (1999).
Voigt, C. A., Kauffman, S. & Wang, Z. G. Rational evolutionary design: the theory of in vitro protein evolution. Adv. Protein Chem. 55 , 79–160 (2000).
Freeman, W. J. Characterization of state transitions in spatially distributed, chaotic, nonlinear, dynamical systems in cerebral cortex. Integr. Physiol. Behav. Sci. 29 , 294–306 (1994).
Tsuda, I. Toward an interpretation of dynamic neural activity in terms of chaotic dynamical systems. Behav. Brain Sci. 24 , 793–810 (2001).
Jirsa, V. K., Friedrich, R., Haken, H. & Kelso, J. A. A theoretical model of phase transitions in the human brain. Biol. Cybern. 71 , 27–35 (1994). This paper develops a theoretical model (based on synergetics and nonlinear oscillator theory) that reproduces observed dynamics and suggests a formulation of biophysical coupling among brain systems.
Breakspear, M. & Stam, C. J. Dynamics of a neural system with a multiscale architecture. Philos. Trans. R. Soc. Lond. B Biol. Sci. 360 , 1051–1074 (2005).
Bressler, S. L. & Tognoli, E. Operational principles of neurocognitive networks. Int. J. Psychophysiol. 60 , 139–148 (2006).
Werner, G. Brain dynamics across levels of organization. J. Physiol. Paris 101 , 273–279 (2007).
Pasquale, V., Massobrio, P., Bologna, L. L., Chiappalone, M. & Martinoia, S. Self-organization and neuronal avalanches in networks of dissociated cortical neurons. Neuroscience 153 , 1354–1369 (2008).
Kitzbichler, M. G., Smith, M. L., Christensen, S. R. & Bullmore, E. Broadband criticality of human brain network synchronization. PLoS Comput. Biol. 5 , e1000314 (2009).
Rabinovich, M., Huerta, R. & Laurent, G. Transient dynamics for neural processing. Science 321 48–50 (2008).
Tschacher, W. & Hake, H. Intentionality in non-equilibrium systems? The functional aspects of self-organised pattern formation. New Ideas Psychol. 25 , 1–15 (2007).
Maturana, H. R. & Varela, F. De máquinas y seres vivos (Editorial Universitaria, Santiago, 1972). English translation available in Maturana, H. R. & Varela, F. in Autopoiesis and Cognition (Reidel, Dordrecht, 1980).
Fiorillo, C. D., Tobler, P. N. & Schultz, W. Discrete coding of reward probability and uncertainty by dopamine neurons. Science 299 , 1898–1902 (2003).
Niv, Y., Duff, M. O. & Dayan, P. Dopamine, uncertainty and TD learning. Behav. Brain Funct. 1 , 6 (2005).
Fletcher, P. C. & Frith, C. D. Perceiving is believing: a Bayesian approach to explaining the positive symptoms of schizophrenia. Nature Rev. Neurosci. 10 , 48–58 (2009).
Phillips, W. A. & Silverstein, S. M. Convergence of biological and psychological perspectives on cognitive coordination in schizophrenia. Behav. Brain Sci. 26 , 65–82 (2003).
Friston, K. & Kiebel, S. Cortical circuits for perceptual inference. Neural Netw. 22 , 1093–1104 (2009).
Download references
Acknowledgements
This work was funded by the Wellcome Trust. I would like to thank my colleagues at the Wellcome Trust Centre for Neuroimaging, the Institute of Cognitive Neuroscience and the Gatsby Computational Neuroscience Unit for collaborations and discussions.
Author information
Authors and affiliations.
The Wellcome Trust Centre for Neuroimaging, University College London, Queen Square, London, WC1N 3BG, UK
Karl Friston
You can also search for this author in PubMed Google Scholar
Ethics declarations
Competing interests.
The author declares no competing financial interests.
Supplementary information
Supplementary information s1 (box).
The entropy of sensory states and their causes (PDF 522 kb)
Supplementary information S2 (box)
Variational free energy (PDF 576 kb)
Supplementary information S3 (box)
The free-energy principle and infomax (PDF 422 kb)
Supplementary information S4 (box)
Value and surprise (PDF 572 kb)
Supplementary information S5 (box)
Policies and cost (PDF 561 kb)
An information theory measure that bounds or limits (by being greater than) the surprise on sampling some data, given a generative model.
The process whereby an open or closed system regulates its internal environment to maintain its states within bounds.
The average surprise of outcomes sampled from a probability distribution or density. A density with low entropy means that, on average, the outcome is relatively predictable. Entropy is therefore a measure of uncertainty.
(Surprisal or self information.) The negative log-probability of an outcome. An improbable outcome (for example, water flowing uphill) is therefore surprising.
(A term from statistical mechanics.) Deals with the probability that the entropy of a system that is far from the thermodynamic equilibrium will increase or decrease over a given amount of time. It states that the probability of the entropy decreasing becomes exponentially smaller with time.
A set to which a dynamical system evolves after a long enough time. Points that get close to the attractor remain close, even under small perturbations.
(Or information divergence, information gain or cross entropy.) A non-commutative measure of the non-negative difference between two probability distributions.
(Or 'approximating conditional density'.) An approximate probability distribution of the causes of data (for example, sensory input). It is the product of inference or inverting a generative model.
A probabilistic model (joint density) of the dependencies between causes and consequences (data), from which samples can be generated. It is usually specified in terms of the likelihood of data, given their causes (parameters of a model) and priors on the causes.
(Or posterior density.) The probability distribution of causes or model parameters, given some data; that is, a probabilistic mapping from observed data to causes.
The probability distribution or density of the causes of data that encodes beliefs about those causes before observing the data.
A measure of salience based on the Kullback-Leibler divergence between the recognition density (which encodes posterior beliefs) and the prior density. It measures the information that can be recognized in the data.
The idea that the brain uses internal probabilistic (generative) models to update posterior beliefs, using sensory information, in an (approximately) Bayes-optimal fashion.
Any strategy (in speech coding) in which the parameters of a signal coder are evaluated by decoding (synthesizing) the signal and comparing it with the original input signal.
Possibly the first theory for why top-down influences (mediated by backward connections in the brain) might be important in perception and cognition.
A prior induced by hierarchical models; empirical priors provide constraints on the recognition density in the usual way but depend on the data.
Quantities that are sufficient to parameterize a probability density (for example, mean and covariance of a Gaussian density).
(Or Laplace approximation or method.) A saddle-point approximation of the integral of an exponential function, that uses a second-order Taylor expansion. When the function is a probability density, the implicit assumption is that the density is approximately Gaussian.
A tool used in signal processing for representing a signal using a linear predictive (generative) model. It is a powerful speech analysis technique and was first considered in vision to explain lateral interactions in the retina.
An optimization principle for neural networks (or functions) that map inputs to outputs. It says that the mapping should maximize the Shannon mutual information between the inputs and outputs, subject to constraints and/or noise processes.
Governed by random effects.
An attentional effect mediated by competitive interactions among neurons representing visual stimuli; these interactions can be biased in favour of behaviourally relevant stimuli by both spatial and non-spatial and both bottom-up and top-down processes.
Reciprocal message passing among neuronal groups.
An area of machine learning concerned with how an agent maximizes long-term reward. Reinforcement learning algorithms attempt to find a policy that maps states of the world to actions performed by the agent.
An optimization method (based on the calculus of variations) for deriving an optimal control law in a dynamical system. A control problem includes a cost function that is a function of state and control variables.
(Or dynamic programming equation.) Named after Richard Bellman, it is a necessary condition for optimality associated with dynamic programming in optimal control theory.
(Or game theory.) An area of applied mathematics concerned with identifying the values, uncertainties and other constraints that determine an optimal decision.
(Or method of steepest ascent.) A first-order optimization scheme that finds a maximum of a function by changing its arguments in proportion to the gradient of the function at the current value. In short, a hill-climbing scheme. The opposite scheme is a gradient descent.
An optimal policy has the property that whatever the initial state and initial decision, the remaining decisions must constitute an optimal policy with regard to the state resulting from the first decision.
Involves a balance between exploration (of uncharted territory) and exploitation (of current knowledge). In reinforcement learning, it has been studied mainly through the multi-armed bandit problem.
An area of applied mathematics that describes the behaviour of complex (possibly chaotic) dynamical systems as described by differential or difference equations.
Concerns the self-organization of patterns and structures in open systems far from thermodynamic equilibrium. It rests on the order parameter concept, which was generalized by Haken to the enslaving principle: that is, the dynamics of fast-relaxing (stable) modes are completely determined by the 'slow' dynamics of order parameters (the amplitudes of unstable modes).
Referring to the fundamental dialectic between structure and function.
Refers to a device or scheme that uses a generative model to furnish a recognition density and learns hidden structures in data by optimizing the parameters of generative models.
Rights and permissions
Reprints and permissions
About this article
Cite this article.
Friston, K. The free-energy principle: a unified brain theory?. Nat Rev Neurosci 11 , 127–138 (2010). https://doi.org/10.1038/nrn2787
Download citation
Published : 13 January 2010
Issue Date : February 2010
DOI : https://doi.org/10.1038/nrn2787
Share this article
Anyone you share the following link with will be able to read this content:
Sorry, a shareable link is not currently available for this article.
Provided by the Springer Nature SharedIt content-sharing initiative
This article is cited by
Sex modulation of faces prediction error in the autistic brain.
- Adeline Lacroix
- Sylvain Harquel
- Marie Gomot
Communications Biology (2024)
Bayesianism and wishful thinking are compatible
- David E. Melnikoff
- Nina Strohminger
Nature Human Behaviour (2024)
Cerebellar Purkinje cells combine sensory and motor information to predict the sensory consequences of active self-motion in macaques
- Omid A. Zobeiri
- Kathleen E. Cullen
Nature Communications (2024)
The generative grammar of the brain: a critique of internally generated representations
- George Dragoi
Nature Reviews Neuroscience (2024)
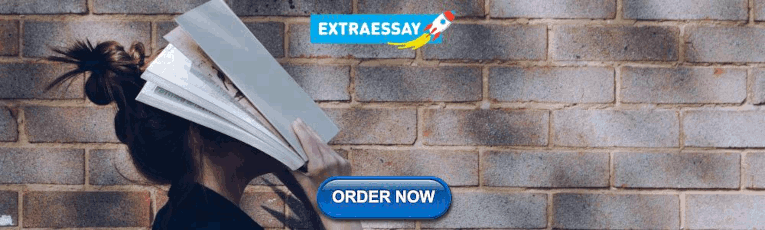
Tactile shape discrimination for moving stimuli
- Nicolas Pélegrin
- Mahiko Konishi
- Jean-Christophe Sarrazin
Scientific Reports (2024)
Quick links
- Explore articles by subject
- Guide to authors
- Editorial policies
Sign up for the Nature Briefing newsletter — what matters in science, free to your inbox daily.

Information
- Author Services
Initiatives
You are accessing a machine-readable page. In order to be human-readable, please install an RSS reader.
All articles published by MDPI are made immediately available worldwide under an open access license. No special permission is required to reuse all or part of the article published by MDPI, including figures and tables. For articles published under an open access Creative Common CC BY license, any part of the article may be reused without permission provided that the original article is clearly cited. For more information, please refer to https://www.mdpi.com/openaccess .
Feature papers represent the most advanced research with significant potential for high impact in the field. A Feature Paper should be a substantial original Article that involves several techniques or approaches, provides an outlook for future research directions and describes possible research applications.
Feature papers are submitted upon individual invitation or recommendation by the scientific editors and must receive positive feedback from the reviewers.
Editor’s Choice articles are based on recommendations by the scientific editors of MDPI journals from around the world. Editors select a small number of articles recently published in the journal that they believe will be particularly interesting to readers, or important in the respective research area. The aim is to provide a snapshot of some of the most exciting work published in the various research areas of the journal.
Original Submission Date Received: .
- Active Journals
- Find a Journal
- Proceedings Series
- For Authors
- For Reviewers
- For Editors
- For Librarians
- For Publishers
- For Societies
- For Conference Organizers
- Open Access Policy
- Institutional Open Access Program
- Special Issues Guidelines
- Editorial Process
- Research and Publication Ethics
- Article Processing Charges
- Testimonials
- Preprints.org
- SciProfiles
- Encyclopedia
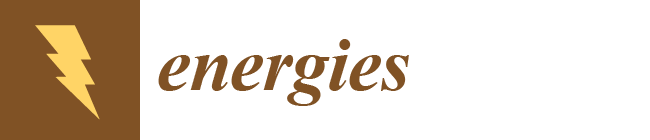
Article Menu

- Subscribe SciFeed
- Recommended Articles
- Google Scholar
- on Google Scholar
- Table of Contents
Find support for a specific problem in the support section of our website.
Please let us know what you think of our products and services.
Visit our dedicated information section to learn more about MDPI.
JSmol Viewer
Positive energy districts: fundamentals, assessment methodologies, modeling and research gaps.
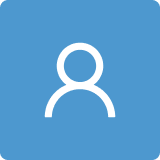
1. Introduction
State of the art on positive energy districts, 2. methodology.
- Setting: a café-like environment with small, round tables, tablecloths, colored pens, sticky notes and any interaction tool available.
- Welcome and Introduction: the host offers a welcome, introduces the World Café process, and sets the context.
- Small-Group Rounds: three or more twenty-minute rounds of conversations occur in small groups. Participants switch tables after each round, with one person optionally remaining as the “table host” to brief newcomers.
- Questions: each round starts with a context-specific question. Questions may remain constant or be built upon each other to guide the discussion.
- Harvest: participants share their discussion insights with the larger group, often visually represented through graphic recording.
- Objectives of the workshop and preparation. The first step of the World Café approach is to identify the main objectives. For this workshop, there was the need to investigate the current landscape of PED research, as well as to have a benchmark and collect feedback on the current research activities within Annex 83. Questions were structured in order to frame the current state-of-the-art understanding of the topic. A mapping of the potential different stakeholders in the PED design and implementation process was carried out at this stage. As a result, municipalities, community representatives, energy contractors, real estate companies and commercial facilitators, as well as citizens, were identified as main target groups. Later, the follow-up discussions were built around these main actors. Further, the mapping of the stakeholders’ involvement was carried out for better understanding the complexity of relationships, roles and synergies as well as the impact on the design, implementation and operation stages of PEDs.
- Positive Energy Districts’ definitions and fundamentals ( Section 3.1 ).
- Quality-of-life indicators in Positive Energy Districts ( Section 3.2 ).
- Technologies in Positive Energy Districts: development, use and barriers ( Section 3.3 ).
- Positive Energy Districts modeling: what is further needed to model PEDs? ( Section 3.4 ).
- Sustainability assessment of Positive Energy Districts ( Section 3.5 ).
- Stakeholder engagement within the design process ( Section 3.6 ).
- Tools and guidelines for PED implementation ( Section 3.7 ).
3.1. Positive Energy Districts Definitions and Fundamentals
3.2. quality-of-life indicators in positive energy districts, 3.3. technologies in positive energy districts: development, use and barriers, 3.4. positive energy districts modeling: what is further needed to model peds, 3.5. sustainability assessment of positive energy districts, 3.6. stakeholder engagement within the design process, 3.7. tools and guidelines for ped implementation, 4. conclusions, author contributions, data availability statement, acknowledgments, conflicts of interest.
- European Commission; Directorate-General for Research and Innovation. 100 Climate-Neutral Cities by 2030—By and for the Citizens ; European Commission: Luxembourg, 2020. [ Google Scholar ]
- Department of Economic and Social Affairs United Nations. 68% of the World Population Projected to Live in Urban Areas by 2050, Says UN. Available online: https://www.un.org/development/desa/en/news/population/2018-revision-of-world-urbanization-prospects.html (accessed on 24 June 2024).
- Fatima, Z.; Vacha, T.; Swamygowda, K.; Qubailat, R. Getting Started with Positive Energy Districts: Experience until Now from Maia, Reykjavik, Kifissia, Kladno and Lviv. Sustainability 2022 , 14 , 5799. [ Google Scholar ] [ CrossRef ]
- OECD. Managing Environmental and Energy Transitions for Regions and Cities ; OECD: Paris, France, 2020; Available online: https://www.oecd.org/en/about/projects/managing-environmental-and-energy-transitions-for-regions-and-cities.html (accessed on 24 June 2024).
- Pierce, S.; Pallonetto, F.; De Donatis, L.; De Rosa, M. District energy modelling for decarbonisation strategies development—The case of a University campus. Energy Rep. 2024 , 11 , 1256–1267. [ Google Scholar ] [ CrossRef ]
- U.N. SDGs Goal 11|Make Cities and Human Settlements Inclusive, Safe, Resilient and Sustainable. Available online: https://sdgs.un.org/goals/goal11 (accessed on 24 June 2024).
- Shahmohammad, M.; Salamattalab, M.M.; Sohn, W.; Kouhizadeh, M.; Aghamohmmadi, N. Opportunities and obstacles of blockchain use in pursuit of sustainable development goal 11: A systematic scoping review. Sustain. Cities Soc. 2024 , 112 , 105620. [ Google Scholar ] [ CrossRef ]
- Koch, F.; Ahmad, S. How to Measure Progress Towards an Inclusive, Safe, Resilient and Sustainable City? Reflections on Applying the Indicators of Sustainable Development Goal 11 in Germany and India. In Urban Transformations. Future City ; Kabisch, S., Koch, F., Gawel, E., Haase, A., Knapp, S., Krellenberg, K., Nivala, J., Zehnsdorf, A., Eds.; Springer: Cham, Switzerland, 2017; Volume 10. [ Google Scholar ] [ CrossRef ]
- Sarvari, H.; Mehrabi, A.; Chan, D.W.M.; Cristofaro, M. Evaluating urban housing development patterns in developing countries: Case study of Worn-out Urban Fabrics in Iran. Sustain. Cities Soc. 2021 , 70 , 102941. [ Google Scholar ] [ CrossRef ]
- Gondeck, M.; Triebel, M.-A.; Steingrube, A.; Albert-Seifried, V.; Stryi-Hipp, G. Recommendations for a positive energy district framework—Application and evaluation of different energetic assessment methodologies. Smart Energy 2024 , 15 , 100147. [ Google Scholar ] [ CrossRef ]
- Gohari, S.; Castro Silvia, S.; Ashrafian, T.; Konstantinou, T.; Giancola, E.; Prebreza, B.; Aelenei, L.; Murauskaite, L.; Liu, M. Unraveling the implementation processes of PEDs: Lesson learned from multiple urban contexts. Sustain. Cities Soc. 2024 , 106 , 105402. [ Google Scholar ] [ CrossRef ]
- Sassenou, L.-N.; Olivieri, L.; Olivieri, F. Challenges for positive energy districts deployment: A systematic review. Renew. Sustain. Energy Rev. 2024 , 191 , 114152. [ Google Scholar ] [ CrossRef ]
- Good, N.; Martínez Ceseña, E.A.; Mancarella, P. Chapter Two—Energy Positivity and Flexibility in Districts. In Energy Positive Neighborhoods and Smart Energy Districts ; Monti, A., Pesch, D., Ellis, K.A., Mancarella, P., Eds.; Academic Press: Cambridge, MA, USA, 2017; pp. 7–30. ISBN 9780128099513. [ Google Scholar ] [ CrossRef ]
- Derkenbaeva, E.; Halleck Vega, S.; Hofstede, G.J.; van Leeuwen, E. Positive Energy Districts: Mainstreaming Energy Transition in Urban Areas. Renew. Sustain. Energy Rev. 2022 , 153 , 111782. [ Google Scholar ] [ CrossRef ]
- Bruck, A.; Díaz Ruano, S.; Auer, H. One Piece of the Puzzle towards 100 Positive Energy Districts (PEDs) across Europe by 2025: An Open-Source Approach to Unveil Favourable Locations of PV-Based PEDs from a Techno-Economic Perspective. Energy 2022 , 254 , 124152. [ Google Scholar ] [ CrossRef ]
- Vandevyvere, H.; Ahlers, D.; Wyckmans, A. The Sense and Non-Sense of PEDs—Feeding Back Practical Experiences of Positive Energy District Demonstrators into the European PED Framework Definition Development Process. Energies 2022 , 15 , 4491. [ Google Scholar ] [ CrossRef ]
- Natanian, J.; Magyari, A.; Brunetti, A.; Reith, A.; Guarino, F.; Manapragada, N.; Cellura, S.; de Luca, F.; Naboni, E. Ten Questions on Tools and Methods for Positive Energy Districts. Build. Environ. 2024 , 255 , 111429. [ Google Scholar ] [ CrossRef ]
- Perera, A.T.D.; Javanroodi, K.; Wang, Y.; Hong, T. Urban Cells: Extending the Energy Hub Concept to Facilitate Sector and Spatial Coupling. Adv. Appl. Energy 2021 , 3 , 100046. [ Google Scholar ] [ CrossRef ]
- Aparisi-Cerdá, I.; Ribó-Pérez, D.; Cuesta-Fernandez, I.; Gómez-Navarro, T. Planning Positive Energy Districts in Urban Water Fronts: Approach to La Marina de València, Spain. Energy Convers. Manag. 2022 , 265 , 115795. [ Google Scholar ] [ CrossRef ]
- Sareen, S.; Albert-Seifried, V.; Aelenei, L.; Reda, F.; Etminan, G.; Andreucci, M.-B.; Kuzmic, M.; Maas, N.; Seco, O.; Civiero, P.; et al. Ten Questions Concerning Positive Energy Districts. Build. Environ. 2022 , 216 , 109017. [ Google Scholar ] [ CrossRef ]
- Mihailova, D.; Schubert, I.; Burger, P.; Fritz, M.M.C. Exploring modes of sustainable value co-creation in renewable energy communities. J. Clean. Prod. 2022 , 330 , 129917. [ Google Scholar ] [ CrossRef ]
- Mihailova, D.; Schubert, I.; Martinez-Cruz, A.L.; Hearn, A.X.; Sohre, A. Preferences for configurations of Positive Energy Districts—Insights from a discrete choice experiment on Swiss households. Energy Policy 2022 , 163 , 112824. [ Google Scholar ] [ CrossRef ]
- Working Group SET. Plan Temporary SET-Plan ACTION n 3.2 Implementation Plan: Europe to Become a Global Role Model in Integrated, Innovative Solutions for the Planning, Deployment, and Replication of Positive Energy Districts ; 2018. Available online: https://jpi-urbaneurope.eu/wp-content/uploads/2021/10/setplan_smartcities_implementationplan-2.pdf (accessed on 24 June 2024).
- JPI Urban Europe/SET Plan Action 3.2. In White Paper on PED Reference Framework for Positive Energy Districts and Neighbourhoods ; 2020. Available online: https://jpi-urbaneurope.eu/wp-content/uploads/2020/04/White-Paper-PED-Framework-Definition-2020323-final.pdf (accessed on 24 June 2024).
- ATELIER. Atelier—Positive Energy Districts. Available online: https://smartcity-atelier.eu/ (accessed on 24 June 2024).
- EURAC Smart-BEEjS—Human-Centric Energy Districts: Smart Value Generation by Building Efficiency and Energy Justice for Sustainable Living. Available online: https://www.eurac.edu/en/institutes-centers/institute-for-renewable-energy/projects/smart-beejs (accessed on 24 June 2024).
- Making City Making City—Energy Efficient Pathway for the City Transformation. Available online: https://makingcity.eu/ (accessed on 24 June 2024).
- +CityxChange. Available online: https://cityxchange.eu/ (accessed on 24 June 2024).
- Uspenskaia, D.; Specht, K.; Kondziella, H.; Bruckner, T. Challenges and Barriers for Net-Zero/Positive Energy Buildings and Districts—Empirical Evidence from the Smart City Project SPARCS. Buildings 2021 , 11 , 78. [ Google Scholar ] [ CrossRef ]
- Hedman, Å.; Rehman, H.U.; Gabaldón, A.; Bisello, A.; Albert-Seifried, V.; Zhang, X.; Guarino, F.; Grynning, S.; Eicker, U.; Neumann, H.-M.; et al. IEA EBC Annex83 Positive Energy Districts. Buildings 2021 , 11 , 130. [ Google Scholar ] [ CrossRef ]
- Gabaldón Moreno, A.; Vélez, F.; Alpagut, B.; Hernández, P.; Sanz Montalvillo, C. How to Achieve Positive Energy Districts for Sustainable Cities: A Proposed Calculation Methodology. Sustainability 2021 , 13 , 710. [ Google Scholar ] [ CrossRef ]
- Aghamolaei, R.; Shamsi, M.H.; Tahsildoost, M.; O’Donnell, J. Review of district-scale energy performance analysis: Outlooks towards holistic urban frameworks. Sustain. Cities Soc. 2018 , 41 , 252–264. [ Google Scholar ] [ CrossRef ]
- Bottecchia, L.; Gabaldón, A.; Castillo-Calzadilla, T.; Soutullo, S.; Ranjbar, S.; Eicker, U. Fundamentals of Energy Modelling for Positive Energy Districts. In Sustainability in Energy and Buildings ; Littlewood, J.R., Howlett, R.J., Jain, L.C., Eds.; Springer: Singapore, 2022; Volume 263, Smart Innovation, Systems and Technologies. [ Google Scholar ] [ CrossRef ]
- Zhang, S.; Ma, M.; Zhou, N.; Yan, J.; Feng, W.; Yan, R.; You, K.; Zhang, J.; Ke, J. Estimation of Global Building Stocks by 2070: Unlocking Renovation Potential. Nexus 2024 , 1 , 100019. [ Google Scholar ] [ CrossRef ]
- Xiang, X.; Zhou, N.; Ma, M.; Feng, W.; Yan, R. Global transition of operational carbon in residential buildings since the millennium. Adv. Appl. Energy 2023 , 11 , 100145. [ Google Scholar ] [ CrossRef ]
- Yan, R.; Ma, M.; Zhou, N.; Feng, W.; Xiang, X.; Mao, C. Towards COP27: Decarbonization patterns of residential building in China and India. Appl. Energy 2023 , 352 , 122003. [ Google Scholar ] [ CrossRef ]
- Yuan, H.; Ma, M.; Zhou, N.; Xie, H.; Ma, Z.; Xiang, X.; Ma, X. Battery electric vehicle charging in China: Energy demand and emissions trends in the 2020s. Appl. Energy 2024 , 365 , 123153. [ Google Scholar ] [ CrossRef ]
- Orehounig, K.; Evins, R.; Dorer, V. Integration of Decentralized Energy Systems in Neighbourhoods Using the Energy Hub Approach. Appl. Energy 2015 , 154 , 277–289. [ Google Scholar ] [ CrossRef ]
- Pan, Z.; Guo, Q.; Sun, H. Interactions of District Electricity and Heating Systems Considering Time-Scale Characteristics Based on Quasi-Steady Multi-Energy Flow. Appl. Energy 2016 , 167 , 230–243. [ Google Scholar ] [ CrossRef ]
- Zhou, Y.; Cao, S.; Hensen, J.L.M. An energy paradigm transition framework from negative towards positive district energy sharing networks—Battery cycling aging, advanced battery management strategies, flexible vehicles-to-buildings interactions, uncertainty and sensitivity analysis. Appl. Energy 2021 , 288 , 116606. [ Google Scholar ] [ CrossRef ]
- Marrasso, E.; Martone, C.; Pallotta, G.; Roselli, C.; Sasso, M. Assessment of energy systems configurations in mixed-use Positive Energy Districts through novel indicators for energy and environmental analysis. Appl. Energy 2024 , 368 , 123374. [ Google Scholar ] [ CrossRef ]
- Bruck, A.; Díaz Ruano, S.; Auer, H. A Critical Perspective on Positive Energy Districts in Climatically Favoured Regions: An Open-Source Modelling Approach Disclosing Implications and Possibilities. Energies 2021 , 14 , 4864. [ Google Scholar ] [ CrossRef ]
- Ali, U.; Shamsi, M.H.; Hoare, C.; Mangina, E.; O’Donnell, J. Review of Urban Building Energy Modeling (UBEM) Approaches, Methods and Tools Using Qualitative and Quantitative Analysis. Energy Build. 2021 , 246 , 111073. [ Google Scholar ] [ CrossRef ]
- Brunetti, A.; Cellura, S.; Guarino, F.; Longo, S.; Mistretta, M.; Reda, F.; Rincione, R. Development of an Early Design Tool for the Sustainability Assessment of Positive Energy Districts: Methodology, Implementation and Case-Studies. J. Phys. Conf. Ser. 2023 , 2600 , 82020. [ Google Scholar ] [ CrossRef ]
- Trulsrud, T.H.; Van der Leer, J. Towards a positive energy balance: A comparative analysis of the planning and design of four positive energy districts and neighbourhoods in Norway and Sweden. Energy Build. 2024 , 318 , 114429. [ Google Scholar ] [ CrossRef ]
- Guasselli, F.; Vavouris, A.; Stankovic, L.; Stankovic, V.; Didierjean, S.; Gram-Hanssen, K. Smart energy technologies for the collective: Time-shifting, demand reduction and household practices in a Positive Energy Neighbourhood in Norway. Energy Res. Soc. Sci. 2024 , 110 , 103436. [ Google Scholar ] [ CrossRef ]
- Aparisi-Cerdá, I.; Ribó-Pérez, D.; Gómez-Navarro, T.; García-Melón, M.; Peris-Blanes, J. Prioritising Positive Energy Districts to achieve carbon neutral cities: Delphi-DANP approach. Renew. Sustain. Energy Rev. 2024 , 203 , 114764. [ Google Scholar ] [ CrossRef ]
- Castillo-Calzadilla, T.; Garay-Martinez, R.; Martin Andonegui, C. Holistic fuzzy logic methodology to assess positive energy district (PathPED). Sustain. Cities Soc. 2023 , 89 , 104375. [ Google Scholar ] [ CrossRef ]
- Derkenbaeva, E.; Jan Hofstede, G.; van Leeuwen, E.; Halleck Vega, S.; Wolfers, J. ENERGY Pro: Spatially explicit agent-based model on achieving positive energy districts. MethodsX 2024 , 12 , 102779. [ Google Scholar ] [ CrossRef ]
- Eicker, U. Introduction: The Challenges of the Urban Energy Transition. In Urban Energy Systems for Low-Carbon Cities ; Eicker, U., Ed.; Academic Press: Cambridge, MA, USA, 2019; pp. 1–15. ISBN 978-0-12-811553-4. [ Google Scholar ]
- Jepsen, B.K.H.; Haut, T.W.; Design, M.J. Modelling and Performance Evaluation of a Positive Energy District in a Danish Island. Future Cities Environ. 2022 , 8 , 1. [ Google Scholar ] [ CrossRef ]
- Guarino, F.; Bisello, A.; Frieden, D.; Bastos, J.; Brunetti, A.; Cellura, M.; Ferraro, M.; Fichera, A.; Giancola, E.; Haase, M.; et al. State of the Art on Sustainability Assessment of Positive Energy Districts: Methodologies, Indicators and Future Perspectives. Sustain. Energy Build. 2021 , 2021 , 479–492. [ Google Scholar ]
- Hearn, A.X.; Sohre, A.; Burger, P. Innovative but Unjust? Analysing the Opportunities and Justice Issues within Positive Energy Districts in Europe. Energy Res. Soc. Sci. 2021 , 78 , 102127. [ Google Scholar ] [ CrossRef ]
- Haarstad, H.; Wathne, M.W. Are Smart City Projects Catalyzing Urban Energy Sustainability? Energy Policy 2019 , 129 , 918–925. [ Google Scholar ] [ CrossRef ]
- Gouveia, J.P.; Seixas, J.; Palma, P.; Duarte, H.; Luz, H.; Cavadini, G.B.C. Positive Energy District: A Model for Historic Districts to Address Energy Poverty. Front. Sustain. Cities 2021 , 3 , 648473. [ Google Scholar ] [ CrossRef ]
- Bossi, S.; Gollner, C.; Theierling, S. Towards 100 Positive Energy Districts in Europe: Preliminary Data Analysis of 61 European Cases. Energies 2020 , 13 , 6083. [ Google Scholar ] [ CrossRef ]
- Rankinen, J.-A.; Lakkala, S.; Haapasalo, H.; Hirvonen-Kantola, S. Stakeholder Management in PED Projects: Challenges and Management Model. Int. J. Sustain. Energy Plan. Manag. 2022 , 34 , 91–106. [ Google Scholar ] [ CrossRef ]
- Schiele, H.; Krummaker, S.; Hoffmann, P.; Kowalski, R. The “research world café” as method of scientific enquiry: Combining rigor with relevance and speed. J. Bus. Res. 2022 , 140 , 280–296. [ Google Scholar ] [ CrossRef ]
- Vandevyvere, H.; Ahlers, D.; Alpagut, B.; Cerna, V.; Cimini, V.; Haxhija, S.; Hukkalainen, M.; Kuzmic, M.; Livik, K.; Padilla, M.; et al. Positive Energy Districts Solution Booklet ; European Union: Luxembourg, 2020. [ Google Scholar ]
- Évora—POCITYF. Available online: https://pocityf.eu/city/evora/ (accessed on 24 June 2024).
- Commission, E.; European Climate, I.; Agency, E.E.; Alpagut, B.; Zhang, X.; Gabaldon, A.; Hernandez, P. Digitalization in Urban. Energy Systems—Outlook 2025, 2030 and 2040 ; Publications Office of the European Union: Luxembourg, 2022. [ Google Scholar ]
- Waglé, S.; Singh, J.; Shah, P. Citizen Report Card Surveys: A Note on the Concept and Methodology (English) ; World Bank: Washington, DC, USA, 2004. [ Google Scholar ]
- Cimini, V.; Giglio, F.; Carbonari, G. D2.4: Report on Bankability of the Demonstrated Innovations. In +CityxChange—Work Package 2 Task 2.7 ; 2019. Available online: https://cityxchange.eu/wp-content/uploads/2020/03/D2.4-Report-on-Bankability-of-the-Demonstrated-Innovations_v3.0-final.pdf (accessed on 24 June 2024).
- Cordis—Horizon 2020 Results in Brief. A Collaborative Approach Promotes Net Zero Energy Settlements. In Achieving near Zero and Positive Energy Settlements in Europe Using Advanced Energy Technology ; 2021. Available online: https://cordis.europa.eu/article/id/430390-a-collaborative-approach-promotes-net-zero-energy-settlements (accessed on 24 June 2024).
- Krangsås, S.G.; Steemers, K.; Konstantinou, T.; Soutullo, S.; Liu, M.; Giancola, E.; Prebreza, B.; Ashrafian, T.; Murauskaitė, L.; Maas, N. Positive Energy Districts: Identifying Challenges and Interdependencies. Sustainability 2021 , 13 , 10551. [ Google Scholar ] [ CrossRef ]
- Prina, M.G.; Manzolini, G.; Moser, D.; Nastasi, B.; Sparber, W. Classification and Challenges of Bottom-up Energy System Models—A Review. Renew. Sustain. Energy Rev. 2020 , 129 , 109917. [ Google Scholar ] [ CrossRef ]
- Allegrini, J.; Orehounig, K.; Mavromatidis, G.; Ruesch, F.; Dorer, V.; Evins, R. A Review of Modelling Approaches and Tools for the Simulation of District-Scale Energy Systems. Renew. Sustain. Energy Rev. 2015 , 52 , 1391–1404. [ Google Scholar ] [ CrossRef ]
- Lyden, A.; Pepper, R.; Tuohy, P.G. A Modelling Tool Selection Process for Planning of Community Scale Energy Systems Including Storage and Demand Side Management. Sustain. Cities Soc. 2018 , 39 , 674–688. [ Google Scholar ] [ CrossRef ]
- Tozzi, P.; Jo, J.H. A Comparative Analysis of Renewable Energy Simulation Tools: Performance Simulation Model vs. System Optimization. Renew. Sustain. Energy Rev. 2017 , 80 , 390–398. [ Google Scholar ] [ CrossRef ]
- Hall, L.M.H.; Buckley, A.R. A Review of Energy Systems Models in the UK: Prevalent Usage and Categorisation. Appl. Energy 2016 , 169 , 607–628. [ Google Scholar ] [ CrossRef ]
- Ringkjøb, H.-K.; Haugan, P.M.; Solbrekke, I.M. A Review of Modelling Tools for Energy and Electricity Systems with Large Shares of Variable Renewables. Renew. Sustain. Energy Rev. 2018 , 96 , 440–459. [ Google Scholar ] [ CrossRef ]
- Neumann, H.M.; Garayo, S.D.; Gaitani, N.; Vettorato, D.; Aelenei, L.; Borsboom, J.; Etminan, G.; Kozlowska, A.; Reda, F.; Rose, J.; et al. Qualitative Assessment Methodology for Positive Energy District Planning Guidelines. In Smart Innovation, Systems and Technologies ; Springer Science and Business Media Deutschland GmbH: Berlin/Heidelberg, Germany, 2022; Volume 263, pp. 507–517. [ Google Scholar ]
- Li, L.; Lange, K.W. Planning Principles for Integrating Community Empowerment into Zero-Net Carbon Transformation. Smart Cities 2023 , 6 , 100–122. [ Google Scholar ] [ CrossRef ]
- Turci, G.; Alpagut, B.; Civiero, P.; Kuzmic, M.; Pagliula, S.; Massa, G.; Albert-Seifried, V.; Seco, O.; Soutullo, S. A Comprehensive PED-Database for Mapping and Comparing Positive Energy Districts Experiences at European Level. Sustainability 2022 , 14 , 427. [ Google Scholar ] [ CrossRef ]
- PED DB: Map—PED-EU-NET|COST ACTION CA19126. Available online: https://pedeu.net/map/ (accessed on 15 January 2024).
- European Union. New European Bauhaus. Available online: https://new-european-bauhaus.europa.eu/about/dashboard_en (accessed on 30 June 2024).
- Volpe, R.; Gonzalez Alriols, M.; Martelo Schmalbach, N.; Fichera, A. Optimal design and operation of distributed electrical generation for Italian positive energy districts with biomass district heating. Energy Convers. Manag. 2022 , 267 , 115937. [ Google Scholar ] [ CrossRef ]
- Bilić, B.; Šmit, K. Evaluation of the New European Bauhaus in Urban Plans by Land Use Occurrence Indicators: A Case Study in Rijeka, Croatia. Buildings 2024 , 14 , 1058. [ Google Scholar ] [ CrossRef ]
- Fichera, A.; Pluchino, A.; Volpe, R. Local Production and Storage in Positive Energy Districts: The Energy Sharing Perspective. Front. Sustain. Cities 2021 , 3 , 690927. [ Google Scholar ] [ CrossRef ]
- Cellura, M.; Fichera, A.; Guarino, F.; Volpe, R. Sustainable Development Goals and Performance Measurement of Positive Energy District: A Methodological Approach. Conference paper-Book Chapter. In Sustainability in Energy and Buildings ; Springer: Singapore, 2021; Volume 263, pp. 519–527. [ Google Scholar ]
Click here to enlarge figure
Question #1 | Question #2 | Question #3 | |
---|---|---|---|
| What are the essential PED DNAs? Can generic PED archetypes be created based on them? | What are the categories of quality-of-life indicators relevant for PED development? | How would you use a database tool to learn about PED development process (e.g., using static information for dynamic decision-making)? |
| Which future technologies would you expect to be adopted in PEDs and cities? | What can be the challenges and the barriers in the future (regarding e.g., control, smart solutions, modeling, technologies) to PED development and diffusion? | What is your expectation for urban and district energy modeling? How can models help to shape PEDs and cities? |
| What is the impact of stakeholders in the PED design/decision process, what are their interests and how are stakeholders likely to be involved in the overall process? | What costs do you expect to bear and what revenues do you expect to realize from the PED implementation? Which aspects should be included in the organizational/business models? | What would you prioritize in terms of energy aspects or efficiency and social implications of living in a PED? Which aspects are more relevant for you? |
| Annex 83 together with other PED initiatives is developing a database of PEDs and PED-Labs: what would be your main interest in consulting the database? | Having the outcomes from PED guidelines analysis, what information would be the most interesting for you to see? | Who can benefit from the PED research studies and Annex 83 results? Which stakeholders are interested? |
Categories | Key Characteristics |
---|---|
Facts and Figures | Physical sizes/population size |
Geographical location | |
Climate | |
Density | |
Built form | |
Land use | |
Energy demand | |
Renewable energy potential | |
Technologies | Renewable energy supplies |
Energy-efficiency measures | |
Energy distribution (e.g., co-generation, district network) | |
Energy storage | |
Mobility solutions | |
Quality of Life | User comfort |
Social-economic conditions | |
Health impacts (e.g., air pollution, noise pollution) | |
Accessibility to green space | |
Accessibility to services (e.g., bike lane, public transportation) | |
Local value/sense of community | |
Others | Regulations/Policies |
Stakeholder involvement | |
Local targets and ambitions | |
Local challenges | |
Impacts of PEDs |
Type | Quality Categories | |
---|---|---|
Tangible | Indoor and outdoor environmental quality | Physical quality and comfort of the environment |
Security and safety | ||
Level and accessibility of servicing | Public and active transport facilities including walkability, energy services (access to affordable energy including access to energy efficiency), sustainable waste management | |
Access to daily life amenities including education, culture, sports, coworking and study places, provisions for children, but even common gardens or community kitchens | ||
Aesthetic quality | ||
Functional mix | ||
Future-proofness | ||
Acceptable cost of life (affordability, inclusivity) | ||
Equity and just transition | ||
Functional links to realizing circularity and reducing emissions | ||
Citizen engagement | Involvement in decision-making | |
Social diversity in participation | ||
Access to greenery | The possibility to reconnect with nature | |
Sufficient open space | ||
Information flow | From creating awareness over enhancing knowledge and literacy up to capacity of control | |
Transparency on energy flows and information for the end prosumer | ||
Insight in applicable PED solutions and in healthy lifestyles | ||
Intangible | Sense of well-being | |
Quality of social connections | ||
Sense of personal achievement | ||
Level of self-esteem | ||
Sense of community | ||
Degree of cooperation and engagement for the common interest | ||
Time spent with friends (outdoor) | ||
Budget available at the end of the month to spend freely | ||
Not being aware or realizing of living in a PED |
Technology Groups | Solutions | |
---|---|---|
Energy efficiency | New energy-efficient buildings and building retrofitting. | |
Nature-based solutions (natural sinks) and carbon capture solutions (CCS) | ||
Efficient resource management | ||
Efficient water systems for agriculture (smart agriculture, hydroponics, agrivoltaics, etc.) | ||
Organic photovoltaics and a circular approach (second life materials, like batteries) | ||
Energy flexibility | Hardware | Storage (long-term and short-term) |
Monitoring systems (sensors, smart meters, PLCs *, energy management systems, etc.) | ||
Vehicle to grid | ||
Heat pumps | ||
Electronic devices like IoT * technologies | ||
Buildings fully automated with real time monitoring behind-the-meter and automated actions | ||
Cybersecurity, data rights and data access | ||
Demand management and remote control of devices | ||
Software | Edge computing | |
Machine learning | ||
Blockchain | ||
Digital twins | ||
5G | ||
City management platform and platforms for city planning (space, refurbishment, climate change, etc.) | ||
E-mobility | Promotion of shared vehicles over individual car use, lift sharing, and alternative ways (like micromobility) to collective transports | |
Soft mobility | Promotion of a lifestyle that require less use of cars, i.e., “soft mobility” solutions like low emission zones or banning the entrance of some type of car (e.g., Singapore and Iran have policies in place to allow only certain car groups to drive freely in certain periods) | |
E-vehicle charging stations and vehicle-to-grid solutions | ||
Low-carbon generation | Photovoltaics | |
Energy communities | ||
Electrification of heating and cooling (H&C) using heat pumps, district heating networks utilizing waste heat, or solar thermal technologies | ||
Virtual production | ||
Fusion technology |
Challenges and Barriers | Key Topics |
---|---|
Capacity building and policy issues | Political and legal barriers |
Regulatory frameworks and policy constraints | |
Tailored legislation | |
Bridging the knowledge gap | |
Inadequate data sharing practices | |
Securing sufficient financial resources | |
Lack of clear regulations defining PED classification | |
Active involvement of policymakers | |
Widespread dissemination of knowledge | |
Collaborative data-sharing efforts | |
Securing adequate funding | |
Establishing supportive policies and regulations | |
Social challenges and considerations | Cultural barriers |
Access to affordable and sustainable energy for all | |
Building social agreements and fostering collaboration | |
Energy literacy | |
Addressing personal behavior acceptance | |
Transition strategy for inclusivity | |
Social inclusion and trust-building | |
Data sharing and privacy concerns | |
Overcoming public opposition and promoting knowledge dissemination | |
Financial barriers | Long-term storage investment and space competition |
Insufficient investment | |
High upfront costs | |
Allocation of costs among stakeholders | |
Incentives for participation | |
Addressing investment challenges for different stakeholders | |
Accounting for battery costs | |
Data management | Data standardization |
Data security measures and protocols | |
Sustainability and maintenance of data infrastructure | |
Privacy regulations and data anonymization techniques | |
Sustainable business models and ownership structures | Standardization of control technologies and replication strategies |
Grid management approaches | |
Deep penetration of sustainable technologies | |
Implementation of predictive models Long-term maintenance activities and resident data collection | |
Balancing diverse requirements | |
Addressing grid operation challenges | |
Managing multiple independent energy districts | |
Inclusivity strategies for digital technology reliance | |
Managing production peaks and defining the role of buildings and districts | |
Effective management strategies for grid congestion and stability |
Categories of Innovation | Innovation Types | Possible Revenues/Advantages in PED Business Model/Governance | Possible Costs/Drawbacks in PED Business Model/Governance |
---|---|---|---|
Configuration | Profit Model | Providing thermal comfort instead of a certain amount of thermal energy to inhabitants | Misconducts or rebound effect |
Network | Inclusion of the PED into larger projects and international networks, possibility of co-financing and knowledge sharing | Misalignment or delay of the PED project to the original timeline due to constrains related to international activities and networking | |
Structure | Participation of the real estate companies/investors in the development and management of the energy infrastructure and EV mobility services as well as building management | Lack of knowledge, involvement in activities out of the usual business of investors | |
Free or almost free thermal energy supply from “waste energy” sources | Failure of the network due to unliteral decisions of a member in ceasing the provision of energy | ||
Process | Involvement of future inhabitants in the design phase of the energy community since the early stage, to share the sense of belonging and ownership | Reluctancy of inhabitants to participate in additional expenses or being involved in “entrepreneurial” activities or bored by the participation in boards and governance structures | |
Offering | Product Performance | Investors and companies involved in the PED development take profit from their role of frontrunner placing them before the competitors or entering in new market niches | Hi-tech BA and BEM systems may result costly in O&M, because of digital components, cloud and computing services, rapid aging of technology |
Product System | Including EV available for PED users may generate new incomes and reduce the need of individual cars. The integration of EV in the energy system may offer “flexibility services” | Lack of knowledge, involvement in activities out of the usual business of investors/real estate companies. Low interest of users in participating to the flexibility market, because of discomfort (unexpected empty battery of the EV) | |
Experience | Services | Provision of high tech and high-performance buildings, with outstanding energy performances (lower heating/cooling costs) and sophisticated Building Automation and Energy Management systems | Sophisticated Building Automation and Energy Management systems may result “invasive” to users, asking for continuous interaction with complicate systems, or leaving them not enough freedom to choose (e.g., opening the windows is not possible to achieve some energy performance) |
Channel | The PED is promoted as a rewarding sustainable investment, this allows the city to attract more clean investments (public funds, investment funds, donors), speeding up the energy transition | The communication of the characteristics of the PED is not done in the proper way | |
Brand | Gold class rated buildings may have an increased value on the market, resulting in higher selling and rental costs, occupancy rate. The high architectural quality is appreciated by the market | The Branding/certification of the PED is not recognized by the market as an added value. | |
The development of the PED takes longer as expected. Technology failures during the implementation or operation phase create a bad reputation and discourage future similar activities | |||
Customer Engagement | The PED is available as a digital twin, users are engaged via a dedicated app, allowing interaction, communication, reporting, monitoring of bills, etc. | The PED is perceived by users (e.g., social housing tenants) as a hassle and not responding to their needs, because they have not been involved in the identification of peculiar traits since the beginning |
Category | Beneficiaries |
---|---|
Citizens and communities | Citizens, inhabitants, residents, general public, local communities and neighborhoods, municipalities and provinces, energy communities, and socially disadvantaged groups. |
City decision-makers and planners | City decision-makers, city planners, local authorities, policy-makers, public administrations, politicians, local and national governments. |
Research | Scientists, publishers, and research organizations. |
Private companies and technology developers | Private companies of RES technologies, ICT companies, start-ups and new companies, entrepreneurs, technology developers and other companies involved in local development (tech development and evaluation). |
Energy providers | Energy providers, grid operators. |
Education stakeholders | Students and teachers. |
Non-governmental organizations (NGOs) | NGOs and other civil society groups |
Category | Comments |
---|---|
Strategies | Most comments dealt with the strategies on how to achieve PEDs, that should focus on success factors of PED initiatives, technologies and stakeholders rather than a standardized approach |
References | Useful information, special attention to Liwen Li, planning principles for integrating community empowerment into zero-carbon transformation |
Definitions | Help to reduce uncertainty |
Boundaries | Energy balance calculations, mobility, definition (of buildings) |
Finance | Financial mechanisms, support schemes |
Citizen engagement | From engagement to empowerment |
Management | Process management, organizing involvement, information provision |
Policy | Incentives, regional policies |
Flexibility/Grid interaction | Timesteps, credit system |
Form | Dissemination through video and other forms (not only written information) |
Category | Comments |
---|---|
Lessons learned | Special reference to real life implementation |
Results | Data analysis and potential research on the field |
Metadata as the useful information that can the real goal of consultation | |
Benchmarking to compare PEDs | |
Need to normalize results depending on a number of factors (size, location…) to really compare different initiatives | |
Privacy and data protection | |
Sets of technologies and solutions | - |
Economic parameters | As a way to benchmark the different PED technologies |
Citizen engagement | Energy poverty |
Prosumers | |
From engagement to empowerment | |
Definition and boundaries | Need to standardize and have a reference framework to establish the energy balance |
Contact persons | It is very valuable to have a contact address to ask more about the initiative |
Regulatory framework | Drivers and Enablers |
The statements, opinions and data contained in all publications are solely those of the individual author(s) and contributor(s) and not of MDPI and/or the editor(s). MDPI and/or the editor(s) disclaim responsibility for any injury to people or property resulting from any ideas, methods, instructions or products referred to in the content. |
Share and Cite
Kozlowska, A.; Guarino, F.; Volpe, R.; Bisello, A.; Gabaldòn, A.; Rezaei, A.; Albert-Seifried, V.; Alpagut, B.; Vandevyvere, H.; Reda, F.; et al. Positive Energy Districts: Fundamentals, Assessment Methodologies, Modeling and Research Gaps. Energies 2024 , 17 , 4425. https://doi.org/10.3390/en17174425
Kozlowska A, Guarino F, Volpe R, Bisello A, Gabaldòn A, Rezaei A, Albert-Seifried V, Alpagut B, Vandevyvere H, Reda F, et al. Positive Energy Districts: Fundamentals, Assessment Methodologies, Modeling and Research Gaps. Energies . 2024; 17(17):4425. https://doi.org/10.3390/en17174425
Kozlowska, Anna, Francesco Guarino, Rosaria Volpe, Adriano Bisello, Andrea Gabaldòn, Abolfazl Rezaei, Vicky Albert-Seifried, Beril Alpagut, Han Vandevyvere, Francesco Reda, and et al. 2024. "Positive Energy Districts: Fundamentals, Assessment Methodologies, Modeling and Research Gaps" Energies 17, no. 17: 4425. https://doi.org/10.3390/en17174425
Article Metrics
Article access statistics, further information, mdpi initiatives, follow mdpi.
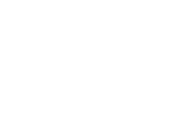
Subscribe to receive issue release notifications and newsletters from MDPI journals
Escaping the Energy Poverty Trap: Policy Assessment
- Open access
- Published: 02 September 2024
Cite this article
You have full access to this open access article
- Elisenda Jové-Llopis ORCID: orcid.org/0000-0001-6145-0230 1 &
- Elisa Trujillo-Baute ORCID: orcid.org/0000-0002-6328-3242 2
3 Altmetric
Climate change and the ongoing energy transition can increase energy poverty rates. To date, the main tool employed to alleviate energy poverty has involved income transfers to vulnerable households. However, measures that seek to improve a home’s energy efficiency have recently gained increasing relevance. In this study we assess the effectiveness of these two types of policy, assuming universal coverage and optimal behaviour. Results points that income transfers and energy efficiency measures have the potential to decrease the proportion of households in energy poverty; however, the magnitude of their respective effects differs greatly. The average impact of energy efficiency measures provides for a greater reduction in energy poverty rates than income transfer policies. Although the greatest reduction in energy poverty is obtained by combining both measures, this combination of tools leads to overlapping effects with income transfers making only a marginal contribution once total retrofit have been implemented.
Similar content being viewed by others
Assessing Ecobonus as Energy Poverty Mitigation Policy: Is Energy Efficiency for All?
Energy poverty and just transformation in greece.
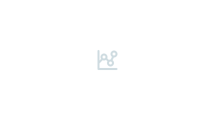
Energy financing, energy projects retrofit and energy poverty: a scenario-analysis approach for energy project cost estimation and energy price determination
Avoid common mistakes on your manuscript.
1 Introduction
Although guaranteeing access to ‘affordable, reliable, sustainable, and modern energy’ is a priority target of one of the sustainable development goals adopted by the United Nations, the number of energy poor around the world remains alarming high (Guan et al. 2023 ; Pachauri et al. 2021 ). For example, in 2022 in the European Union, around 42 million households were estimated to be vulnerable to energy poverty, i.e., they faced difficulties keeping their homes at comfortably warm. In these developed countries energy poverty is conditioned by issues of affordability (Vandyck et al. 2023 ); however, in the developing world energy poverty is more a matter of access to, and the availability of, modern energy services (Pachauri et al. 2021 ). Regardless of this distinction, energy poverty at the individual level has traditionally been impacted by factors from the triangle of on income, energy efficiency, and energy prices with serious implications for the well-being, health, and social inclusion of affected households (Dobbins et al. 2019 ; Oliveras et al. 2021 ). However, the emerging debate is expanding from this traditional triangle to examine the links between energy poverty and new policy areas resulting from climate change and the energy transition (Stojilovska et al. 2022 ). These problems need to be addressed urgently considering also other elements within the broader energy system (Bessa and Gouveia 2022 ) especially now that the world finds itself immersed in an unprecedented energy crisis and having to contend with the impact of the COVID-19 pandemic (Carfora et al. 2021 ; Guan et al. 2023 ). Failure to address this problem adequately could greatly hinder the achievement of global targets on energy, climate, poverty, and health according to the 2030 Agenda for Sustainable Development (IEA 2023 ).
To date, and because of the multidimensional nature of energy poverty (Meyer et al. 2018 ; Halkos and Gkampoura 2021 ) most of the academic literature has focused its attention on quantifying the phenomenon (Boardman 1991 ; Faiella and Lavecchia 2021 ) with a particular focus on the type of indicator being employed (Tirado Herrero 2017 ; Romero et al. 2018 ). Recently, academia has begun to show a concern for identifying the determinants of energy poverty (Legendre and Ricci 2015 ; Costa-Campi et al. 2019 ), on the understanding that a knowledge of the causes of energy poverty contributes to the proposal of better solutions (Bouzarovski et al. 2012 ; Stojilovska et al. 2022 ). Yet, and despite the importance of being able to assess the effectiveness of public policies aimed at reducing energy poverty in the context of the just energy transition, economic evaluations remain scarce. In any case, existing analyses offer only a partial approach to the problem by evaluating a single type of policy (Alvarez and Tol 2021 ; McCoy and Kotsch 2020 ; Bagnoli and Bertoméu-Sánchez 2022 ), overlooking, for the time being, any potential complementarities or overlaps between multiple types of policy. Moreover, recent studies emphasised that siloed approaches to the design of energy poverty policies are not sufficient to address the full scope of this problem (Stojilovska et al. 2022 ). Here, we seek to further previous studies by examining policy action mechanisms and their interactions in an effort at maximizing their impact on the vulnerable. In the empirical exercise we report here, we assess the hypothetical effectiveness of two types of policies adopted in Spain to reduce energy poverty in terms of both their costs and benefits: we evaluate, on the one hand, policies that act via disposable income, and on a recurring basis, on the bono social —subsidised rates—of electricity and heating and, on the other, policies that act via expenditure, and on a one-off basis, on energy efficiency.
To increase our understanding of the potential effects of these two types of policy action, we drew on existing microdata from the household budget survey (HBS) conducted by Spain’s National Institute of Statistics. To assess the effectiveness of existing energy poverty policies, the magnitude of the phenomena is quantified under scenarios that include alternative policies both individually and in combination.
We find that, on average, the implementation of policies that act via income (i.e. the bono social of electricity and heating) have reduced the number of energy poor households; however, the magnitude of the effect is quite modest, with only 9% of households escaping from the energy poverty trap (i.e. being in a state of energy poverty). In contrast, policies that act via expenditure (i.e. energy efficiency measures) have the potential to minimise between 8%—when targeting more efficient lighting—and 64% of households from energy poverty—when including all the retrofit measures in the dwellings of energy poor households. We further show that when implementing both types of policy (i.e. energy efficiency—total retrofit—and the bono social ), it is possible to minimise 67.4% of households from energy poverty. In other words, it is apparent that implementing both policies together results in negligible gains (64% with total retrofit vs. 67.4% with total retrofit + bono social ).
This study makes several contributions. One of the standout features of this analysis is the improvement in the line of creating evidence to support the policymaking process. We contribute to the scarce literature on the effectiveness of policy instruments tackling energy poverty (Alvarez and Tol 2021 ; Bagnoli and Bertoméu-Sánchez 2022 ). Second, the literature to date has been particularly reliant on evaluating a single type of policy rather than examining potential complementarities between multiple types of policy. However, by leveraging data from Spain, this article enhances previous research by considering jointly income and expenditure policy proposals and their potential impacts in the country. Evaluating the cost-effectiveness of these policies, the paper makes important contributions to the field gaining insights on which support schemes obtain both the highest energy reduction and the highest number of lifted out of energy poverty, especially in times of constrained public budgets. It is worth emphasizing that we assess the theoretical impact of these policies, under the assumption of optimal behaviour and universal coverage for energy-poor households (Al Shawa 2024 ; Berger and Höltl 2019 ).
The remainder of the paper is structured as follows. Section 2 consists of a panoramic overview of the energy poverty policies framework. Section 3 presents the methods and Sect. 4 shows our main findings. The last section presents our conclusions and some policy recommendations.
2 Literature Review: Energy Poverty Policy Framework
We analyse the energy poverty policies based on the conceptual framework that classifies policies depending on the mechanism through which these affects the energy poor household. Specifically, there are three main mechanisms: disposable income, energy expenditure, and consumption behaviour (Pye et al. 2017 ). Traditionally, the main measure used to minimise energy poverty has consisted of income transfers to vulnerable households. Interventions of this type are short-term and palliative in nature, as they seek to provide financial relief to the most vulnerable consumers (in Spain, the so-called bono social falls into this type, given its aim and short-term effects). Indeed, it is evident that countries begin by implementing income measures, as they are the fastest way to address the problem of energy poverty (Kyprianou et al. 2019 ). The recent increase in energy prices and the impact of the COVID-19 crisis have, once more, shown that the measures implemented to address their effects have been short-term, essentially acting via income (Mastropietro et al. 2020 ; Hesselman et al. 2021 ). However, to have a long-term impact, a structural solution to the multidimensional problem is required, one that targets the underlying causes of energy expenditure within the family unit. Indeed, expenditure and behavioural policies with long-term effects are now beginning to gain impetus (Dong et al. 2021 ; Zhao et al. 2022 ). For example, in the proposal for a European Union directive on energy efficiency, it is recognised that energy efficiency measures should be given priority when they can alleviate energy poverty (European Commission 2021 ).
The European Union has set itself the unequivocal goal of achieving an inclusive and fair energy transition (European Commission 2019 ); however, the specification of the tools to lead the fight against energy poverty remains in the hands of the Member States (Dobbins et al. 2019 ). Spain is one of the European Union members that has launched the most initiatives (Bouzarovski et al. 2019 ). Acting through the disposable income of energy poor households, in 2009, the Spanish Government introduced the bono social —a subsidised rate—of electricity, which constitutes a discount applied directly to the consumer’s bill of the so-called voluntary price for the small consumer (or PVPC) tariff. More recently, in 2018, the bono social for heating was introduced as a complementary mechanism to aid vulnerable consumers. This takes the form of a single payment made into the beneficiary’s bank account for the use of heating and hot water (see Methods ).
On the expenditure side, Spain implements the PREE Program (Building Energy Rehabilitation), which channels aid to promote action aimed at reducing carbon dioxide emissions, through energy saving, energy efficiency and renewable thermal energies in existing buildings. The program includes improvements to the thermal envelope, the improvement of the energy efficiency of thermal installations (i.e. replacing fossil fuel-based thermal generation facilities with those based on renewable sources and improving the energy efficiency of generation subsystems such as the heat pump), as well as the improved energy efficiency of lighting. A key aspect of the PREE Program is its social scope, with particular attention being given to the granting of aid to carry out rehabilitation actions in buildings that host vulnerable groups or affected by energy poverty. Hence, following the adoption of Spain’s National Strategy against Energy Poverty 2019–2024 (Government of Spain 2019 ), additional aid is now granted for actions carried out in housing whose owners receive the bono social for electricity.
In general, under the PREE Program, energy efficiency improvements undertaken in entire buildings for all types of reform are granted a subsidy of 35% of the eligible costs, with the exception of lighting installations, for which the subsidy is 15%. Higher subsidised rates are available; for instance, if the household is defined as vulnerable according to the regulations governing the bono social for electricity it qualifies for a 15% higher subsidy, and if the rehabilitation work combines simultaneously two or more elements, one of them being involving the thermal envelope, the household qualifies for a 20% higher subsidy. An additional 15% subsidy can also be obtained in those cases where the efficiency target is met, that is, when a dwelling achieves an A or B energy rating.
Depending on the type of policy implemented—income vs. expenditure—the effect on the group trapped in energy poverty is likely to differ. According to the expenditure-based indicator of low income-high costs (LIHC) (Hills 2012 ), a household is defined as energy poor if its income falls below a certain poverty threshold (i.e. 60% of the median income) and its energy expenses climb above a certain energy threshold (the equivalent median of energy expenditure calculated on the total number of households) (see Fig. 1 ). Thus, if a household is in the lower left quadrant, it will be considered energy poor. This household can escape from energy poverty in one of three ways: (1) by increasing the disposable income, for example by means of transfers from Spain’s bono social for electricity or heating, (2) by decreasing energy costs thanks to the operation of an expenditure policy that improves the energy efficiency of the house, or (3) by both increasing income and decreasing energy costs thanks to combination of both income and expenditure policies. The LIHC indicator has been selected to evaluate the impact of the policies on the energy poverty rate (see Sect. 3.4).
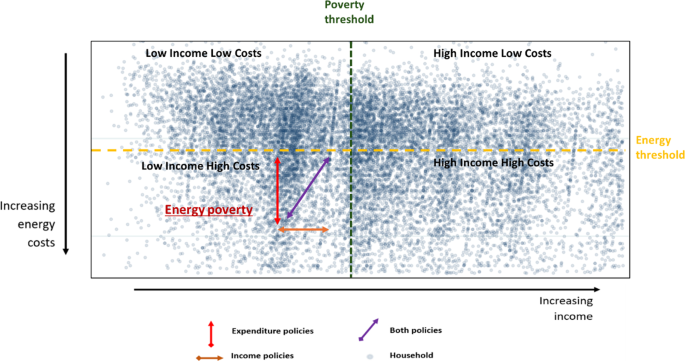
Source : Based on Hills ( 2012 )
Definition of energy poverty and sample distribution (pre-policies).
In what follows, we explain how the various policies targeting energy poverty are replicated in the 19,868 households of 2019 HBS database. This database is carried out by the Spanish National Statistics Institute and provides data at the household level on energy expenditure and a wide variety of socioeconomic variables. Our aim is to exploit the household characteristics available in that database to simulate policy implementation and to assess their potential impacts at the household level. As discussed, we consider two types of policy: income versus expenditure.
3.1 Income Policy—Bono Social for Electricity (BSE) Measure
Here, we describe the replication of the bono social for electricity (henceforth BSE). The first step involves the identification of ‘vulnerable’ and ‘severely vulnerable’ households in the microdata. The identification of these target households is based primarily on household income levels, but other elements must also be considered. Income thresholds are defined in reference to the Indicador Público de Renta de Efectos Múltiples or IPREM, an indictor used in Spain as a benchmark for the granting of aid, subsidies, or unemployment benefit (for example, in 2019 the IPREM index for 14 payments was 1520€ per year). More specifically, the income thresholds that define a ‘vulnerable’ household are:
1.5 * the IPREM index of 14 payments, in the event that it is not part of a family unit or there is no minor in the family unit;
2 * the IPREM index of 14 payments, in the event that there is a minor in the family unit; and
2.5 * the IPREM index of 14 payments, in the event that there are two minors in the family unit.
The BSE definition of a ‘vulnerable’ household incorporates two extra conditions, independent of the those defined by income thresholds:
Being recognised as a familia numerosa or large family, understood to be one or two parents living with three or more children, whether or not the children are common to both parents.
The signatory of the electricity contract (or all the household members that have an income but who are not receiving other income whose annual aggregate amount exceeds 500 euros) is a pensioner of the social security system. There are many variations of the minimum pension level depending on the household composition. We employ the minimum retirement income by category and identify households according to their composition.
We also identify those households that meet the stricter requirements for being recognised as ‘severely vulnerable’ households. A ‘severely vulnerable’ household is one that receives an annual income below 50% of the threshold established for being considered a ‘vulnerable’ consumer. If the household constitutes a familia numerosa, the family unit must be in receipt of an annual income ≤ 2 × IPREM of 14 payments. Additionally, if all the members who have income in the family unit receive a minimum pension, and do not receive other income whose annual aggregate amount exceeds 500 euros, the household unit must receive an annual income ≤ 1 × IPREM of 14 payments to be considered ‘severely vulnerable’.
Finally, less stringent income criteria are reserved for special cases. Particularly, if special circumstances are met, the established limits are increased by 0.5 IPREM points. Special cases include criteria that we have been able to replicate in the database—for example, single-parent families—and others that are impossible to replicate due to data limitations—for example, victims of gender violence or terrorism, and the disabled.
The procedure outlined above allows us to identify the ‘vulnerable’ households that meet the requirements to benefit from the policy; however, the actual number of BSE beneficiaries is significantly lower. The main reasons for this are that BSE is only for consumers under the regulated tariff (PVPC), many households have either not been informed about the BSE, are unaware they have the right to access the subsidy, or do not know how to complete the bureaucratic process to become a beneficiary. In our empirical evaluation, to assess the full potential of policies tackling energy poverty, we assume that the measure reaches all vulnerable households.
Having identified the ‘vulnerable’ and ‘severely vulnerable’ households, we applied the income transfer (equivalent to the corresponding discount) to their electricity bills—i.e. a 25% discount for vulnerable households and a 40% discount for ‘severely vulnerable’ households. Thus, we calculate (Eq. 1 ) the income transfer from the BSE in each household i as:
The last element of the BSE replicated in our assessment is ensuring that the income transfer is limited to certain levels of electricity consumption. For instance, families without children have a consumption limit of 1380 kWh per year, and any consumption above this limit is not discounted (at 25 or 40% depending on the case). The data show that in 2019, 10.65% of ‘vulnerable’ consumers exceeded the established consumption limits and that, therefore, these limits were binding for the total consumption of these households. To replicate these limits in our calculations, the consumption thresholds were transformed from kWh to EUR based on retail electricity price data and applied to the BSE discounts.
3.2 Income Policy—Bono Social for Heating Measure
The bono social for heating is assigned automatically when a household is a recipient of the BSE. Footnote 1 Thus, in order to identify the target households, we use the same requirements as outlined above for the BSE. However, the amount transferred is determined according to the climate zone in which the household is located (see Fig. 7 ). The implementation of these climate zones is the main challenge faced when seeking to replicate this measure in our database. While the regulations state that climate zones are assigned at a municipal level, due to database limitations, we are obliged to assign the climate zones by the corresponding Autonomous Community or regional NUTS-2 level (Eurostat 2016 ). Specifically, for each Community we calculate the average bono social for heating according to the climate zones present in each territory and in this way, we determine the amount transferred to each household ( \({Heatingbonus}_{i})\) .
Finally, to analyse energy poverty rates before and after the application of these policies, we have to compute respective household income levels (Eq. 2 ). The household income before policy implementation is expressed as \({(Inc}_{i}^{o})\) , while household income after the bono social is applied is expressed as \({(Inc}_{i}^{b})\) , where:
3.3 Expenditure Policy—Energy Efficiency Measures
Here we provide detailed information about the use of HBS data (specifically as regards dwelling characteristics) to replicate the impact of different rehabilitation measures (that is, those included in the energy efficiency measure) in terms of household costs and savings.
The methodology used to calculate theoretical energy efficiency improvements at the household level is highly detailed and because we only have information about housing type (apartment, townhouse, etc.), surface area ( \({m}^{2}\) ) and the number of rooms in the dwelling, we are only able to replicate this in part in our database. We build on the findings of a previous technical document (Capdevila et al. 2012 ). This allows us to characterize, in a comparable and consistent fashion, a wide set of Institute for the Diversification and Saving of Energy (IDAE)-PREE Program eligible retrofit works with the limited information on dwelling characteristics in the database. To the best of our knowledge, this is the only study or technical document available to meet our purposes here, with the added advantage of providing retrofit results tailored to Spanish dwellings and taking into consideration the wide climate variations in Spain, along with their impact on energy efficiency. Specifically, we use the sustainability parameters for a set of energy efficiency rehabilitation works for Spanish dwellings. For each energy efficiency work type, we use the improvement in energy usage in kWh/m 2 year of final energy consumption to calculate the current value of future gains from the energy efficiency Footnote 2 —denoted as \({Eff\_gains}_{i}^{w}\left(\frac{\text{kWh}}{{\text{m}}^{2}}\right)\) , and the cost of implementation (€/m 2 )—denoted as \({Cost}^{W}\left(\frac{EUR}{{\text{m}}^{2}}\right)\) . The retrofit works considered refer to the rehabilitation of the envelope (facade insulation, cover insulation and insulation holes), equipment (condensation boiler, efficient boiler, solar thermal and heat pump including cooling and heating technologies), and lighting.
We calculate the final annual investment cost after subsidies for the different energy efficiency works that each household undertakes. First, in order to calculate the annual investment cost of each energy efficiency intervention, we include the lifespan of the different works and equipment.Lifespan corresponds to the typical lifespan of the given equipment (see Nägeli et al. 2019 ; Košičan et al. 2021 ). We assume the following lifespans (in years): facade and gaps—30, roof—20, solar thermal—20, other equipment—12.5, and lighting—10. Then, we define the final annual cost (in €) of the energy efficiency work \(w\) for the household \(i\) as \({Cost}_{i}^{w}\) . All the different retrofit improvements are considered individually and also in combination. The interventions are evaluated for ‘vulnerable’ and ‘non-vulnerable’ consumers. The percentage of eligible costs depends on the type of intervention and is assigned according to the standards established in the program design (as detailed in the measure description in the “ Bono social vs. energy efficiency” section).
Mathematically, our costs and savings model can be summarised in the following equations (Eq. 3.1 and Eq. 3.2 ). The following investment costs are assigned at the household level for the different interventions.
For lighting retrofits only, the \({Cost}_{i}^{w}\) is equal to:
Otherwise \({Cost}_{i}^{w}\) is equal to:
The mean annual investment costs of the different rehabilitation measures, obtained by application to our database (as detailed above), are summarised in Table 1 .
The energy efficiency gains (in €, Eq. 4 ) resulting from the energy efficiency work \(w\) for household \(i\) , are defined as:
Therefore, the monetary savings (Eq. 5 ) obtained from the efficiency work \(w\) by household \(i\) , are:
Finally, we subtract the savings from the household’s energy expenditures to know the impact of the measure in terms of energy expenditure. Where \({EE}_{i}^{o}\) is the energy expenditure of household \(i\) before any measure has been applied, and \({EE}_{i}^{w}\) is the energy expenditure of household \(i\) after the energy efficiency measure \(w\) has been applied.
In short, we simulated the impact of the energy efficiency retrofit measure \(w\) on the household’s energy consumption. Starting with a household’s energy consumption, we subtract the energy efficiency gains obtained thanks to the retrofit work and add the annual cost of the retrofit after applying the PREE Program subsidies.
3.4 Impact of Policies on Energy Poverty
The final step in our methodology is to evaluate the impact of the policies on the energy poverty rate. To characterize energy poverty, we use the LIHC indicator, which defines a household as energy poor when its energy costs are above the national median and its residual income after energy expenditure is below 60% of the median residual income or the poverty line. Footnote 3 Following Romero et al. ( 2018 ), who took their lead from Hills ( 2012 ), the median of energy expenditure is subtracted from the median household income to be consistent with the first term of the equation. In addition, with this formulation, we also overcome the main criticism made by Robinson et al. ( 2018 ) regarding the consideration of the median fuel cost instead of 60% of the median as in the case of income.
The household energy expenditure and income data come from the Spanish HBS. They are treated in order that the UC can be considered. These data are used in the following variables:
\(EE_{i}\) is the observed energy expenditure of household i
\(\widetilde{EE}_{{}} {\text{ is the}}\) observed country median energy expenditure
\(Inc_{i}\) is the observed income of household
\(\widetilde{Inc}_{{}}\) is the observed country median income
Using the survey information, we can calculate energy poverty before the benefits of the measure are felt by households.
Hence, before the bono social uses or energy efficiency policies have been applied, a household is energy poor if:
Our goal is to understand the impact of the bono social on Spanish energy poverty. As explained above, we have defined household income before the bono as \({Inc}_{it}^{o}\) while \({Inc}_{it}^{b}\) represents household income after the selected household receive the bono social . Hence, after the bono has been received, a household is energy poor if:
Analogously, based on energy expenditure before policies as \({EE}_{i}^{o}\) , we can define the energy expenditure of household \(i\) in period \(t\) after the energy retrofit measure w has been applied as \({EE}_{i}^{w}\) . Hence, a household \(i\) in period \(t\) is poor after retrofit work \(w\) if
Hence, if both the bono social and the retrofit policies are applied, energy poverty is defined as:
As explained before, we use the LIHC indicator of energy poverty for our analysis. This methodological choice is deemed suitable, given the nature of the study with an individual approach to, both, defining the problem and performing the policy assessment, and also given the availability of relevant data. The ideal empirical approach to the research question we are facing would potentially imply the use alternative indicators of energy poverty. Also, it could be of interest to incorporate broader definition of energy poverty to consider factors beyond the individual ones in defining the energy poverty situation and contextualize it within the broader energy system, where relevant elements, like the energy prices, could be directly incorporated into the analysis. Although combining indicators is desirable to measure broader aspects, it implies challenges such as database availability and comparability.
At the moment, in spite of there not being any absolute consensus about the best metric for the analysis of energy poverty, since no measurement is perfect (Sareen et al. 2020), it is possible to affirm that there is a subset of indicators that, from the perspective of income and/or expenditure, allows the problem to be robustly quantified, and therefore, ultimately, solutions to be put forward for public policy. This subset is made up of the indicators that are most frequently used in the literature, and which have been those favoured mainly by the United Kingdom government, and in recent years they have also gained ground in research based in the EU (Legendre and Ricci 2015 ; Bouzarovski and Tirado Herrero 2017 ).
Measurement through the LIHC is not free of criticisms. One of the issues frequently pointed out is that, since the indicator defines poverty as those who earn 60% of median income in combination with the median energy cost (instead of 60% of the median energy cost), this approach excludes single person households (Broadman 2012 ; Robinson et al. 2018 ). In our analysis this limitation is overcome by using the 60% of the median for both, income and energy expenditure. It is also claimed that the LIHC indicator tends to prioritise energy efficiency as a solution to fuel poverty, distracting from other drivers more related to affordability (Middlemiss 2016 ). This is not a major source of concern given that the nature of our study is beyond the simple statistical analysis of the indicator, with the assessment of the potential effects from alternatives policies, including both income and expenditure side of the analysis.
No indicator of energy poverty is likely to be perfect, but the LIHC includes those on the margin of poverty who are pushed to energy poverty by their high energy requirement, hence considering the problem from both income and cost perspectives (Hills 2011 ). Grounded on the above but acknowledging the limitations of using the LIHC as a single measurement, this study relies on a modified LIHC indicator to quantify the energy poverty in Spain.
Finally, it is relevant to mention that structural elements of the energy model which may contribute to energy poverty could be relevant to contextualize the phenomenon and enrich the analysis. However, the policy assessment performed in this study is based on simulations using data for a single year, in a system with uniform energy pricing. This implies that, in the policy simulations, the only changes faced by energy poor households are those coming from the policy itself, while the structural elements are assumed to remain constant, i.e. ceteris paribus . While this approach could be considered too narrow and ideally additional elements could be incorporated to provide a more comprehensive view of the energy poverty problem, actually allows to provide a straightforward answer to the research question on policies acting though alternatives mechanism, directly affecting the energy poverty condition at the household level (income and energy expenditure).
4 Results: Impact Assessment
The richness of our composition of the data makes it possible to design scenarios that consider the households’ socioeconomic characteristics, and to simulate policy implementation and their impacts at the household level. The policy evaluation presented is based on results using data for the year 2019, which included the bono social applied before the pandemic crisis, given that temporary modifications were introduced with the onset of the pandemic.
We analyse the results with regards to two specific dimensions: first, in terms of the resultant fall in energy expenditure (and its equivalent in the case of an income transfer); and second, in terms of the households lifted out of the energy poverty trap. Table 2 provides details on both energy poor households and all households in the sample (19,868 households), about the pre-policy (original) values and the outcomes following the implementation of the different measures (energy efficiency and bono social ). Regarding pre-policy values, our data show that 6.8% of the total sample of households experienced energy poverty, according to the LIHC indicator. Moreover, the average yearly income and energy expenditure per household of the overall population stood at 25,139€ and 1076€, while the comparative figures for the energy poor households stood at 10,421€ and 1481€, respectively.
Regarding the evaluation of policies in terms of their impact on energy expenditure, the results for the effects of the energy efficiency measure highlight that, in the case of actions impacting the thermal envelope, all leads to the similar reduction in energy expenditure, decreasing outlay by an average 6% in the case of energy poor households and by 7% when applied to all households in the sample. In the case of actions to improve equipment, the installation of a condensation boiler leads to the greatest reduction in energy expenditure, with an average 16% decrease in poor households and 20% in all households. Lighting is the energy efficiency measure that has the lowest impact on expenditure, being responsible for a 3 and 4% fall when installed in poor households and in all households, respectively. Finally, combining retrofit solutions leads to lower levels of energy expenditure than implementing individual solutions (thus, all thermal envelope actions lead to a 17% reduction, all equipment actions to a 23% reduction, and total retrofit reduces expenditure by 39% in energy poor households). Overall, our results show that the energy efficiency gains are always smaller for energy poor households, which would appear to reflect the smaller size of these dwellings in the sample, giving them a lower potential gain. However, in relative terms, when considering the saving with respect to the average income, the energy poor households would obtain the highest potential gains. According to our results, the bono social measure leads to an increase in average disposable income of 280€ with respect to the pre-policy income of energy poor households. This transfer means their disposable income is 2.6% higher, which—if spent on energy is equivalent to a 19% reduction of the energy expenditure. If we combine this income transfer and all the energy efficiency measures (i.e. total retrofit), then the impact is equivalent to a 57% decrease in the energy expenditure of energy poor households.
In addition to evaluating these policies in terms of their impact on energy expenditure, it is also critical to assess their effectiveness in terms of the proportion of households that escape the energy poverty trap. In this regard, our estimates show that improvements to the thermal envelope have the potential of saving 16% of households from energy poverty in the case of facade insulation, cover insulation and insolation of holes, leading to shares of energy poverty after the measure of 5.7%. Meanwhile, improvements to equipment have the potential of saving 36% of households from the poverty trap when the condensation boiler is replaced and 8% with more efficient lighting, leading to shares of energy poverty after the measure of 4.3% and 6.2%, respectively (see Fig. 2 ).
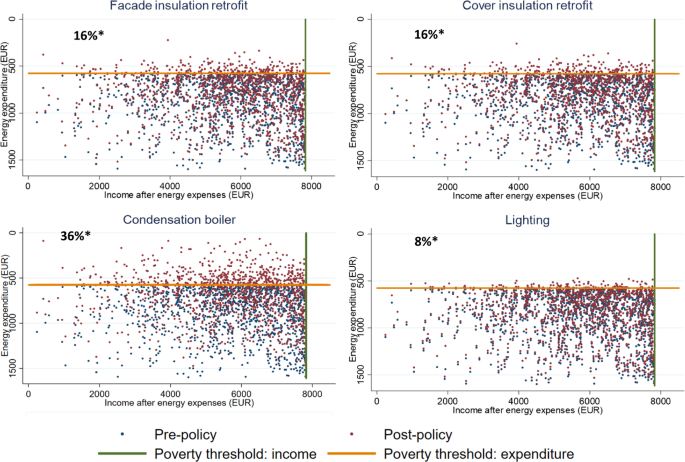
Impact of envelope and equipment expenditure measures on energy poverty. Annual energy expenditure and income after energy expenses per household in euros. * % of energy poor households minimised from energy poverty
One of the most relevant results from this simulation is found when under the energy efficiency measure all retrofit options are combined (see Fig. 3 ). Thus, when energy poor households adopt all the retrofit measures, 64% can potentially be lifted out from the energy poverty trap, leading to an energy poverty share of only 2.4% after the measure is implemented.
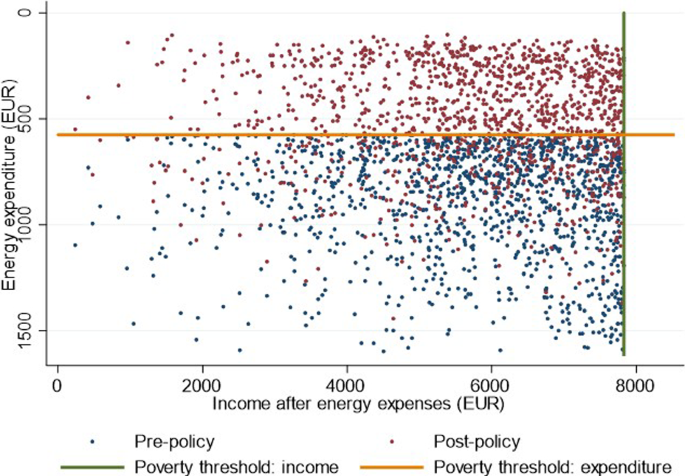
Impact of total retrofit measure on energy poverty. Annual energy expenditure and income after energy expenses per household in euros. 64% of energy poor households minimised from energy poverty
If we compare all the effects of energy efficiency measures combined with an income policy on the proportion of households that escape the energy poverty trap, our outcomes for the bono social are considerably inferior. Indeed, bono social of electricity and heating only have the potential of saving 9% of households from energy poverty, with a share of energy poverty after the policy equals to 6.2% (similar to pre-policy share of 6.8%) (see Fig. 4 ).
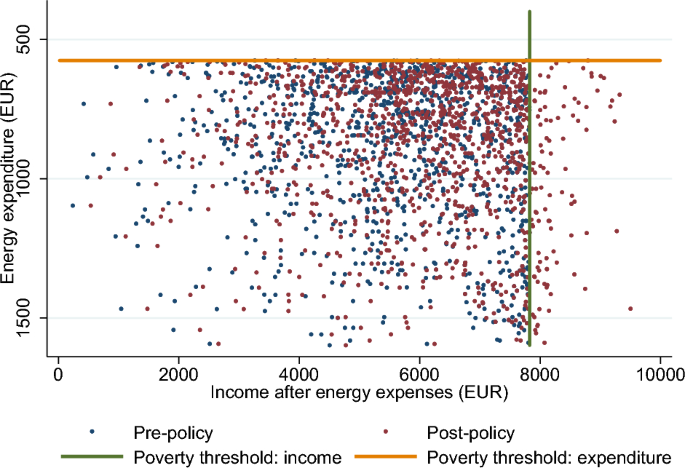
Impact of the bono social on energy poverty. Annual energy expenditure and income after energy expenses per household in euros. 9% of energy poor households minimised from energy poverty
Finally, when the energy poor households implement all possible policies considered in this study, including, that is, all the energy efficiency (total retrofit) measures and the bono social , our results indicate that this has the potential of saving 67.4% households from the energy poverty trap, achieving a share of energy poverty after the policy of only 2.2% (see Fig. 5 ). If we compare this result with that of the impact of a total retrofit, it is apparent that the gain from also introducing the bonos , in terms of the reduction in energy poverty, is extremely small (64% with total retrofit vs. 67.4% with total retrofit + bonos ), leading to similar shares of energy poverty (2.4% with total retrofit vs. 2.2% with total retrofit + bonos ). Energy efficiency is widely recognized within the European Union as a transformative solution to alleviate energy poverty. However, it is important to consider potential obstacles to the effectiveness of building renovation and thermal insulation efforts, such as the well-documented rebound effect (see Berkhout et al. 2000 ; Sorrell and Dimitropoulos 2008 ). The energy efficiency literature has studied this effect on energy-poor consumers, indicating that the benefits of efficiency interventions can be diminished by behavioural responses aimed at increasing thermal comfort (Berger and Höltl 2019 ; Milne and Boardman 2000 ). To mitigate these challenges, energy efficiency measures must be complemented by behavioural initiatives that aim to modify energy consumption habits through training and personalized advice. The overarching goal is to transition from established pre-retrofit practices and associated energy use patterns to new, more efficient practices across various aspects such as lighting, appliance usage, heating systems, and more (Rau et al. 2020 ).
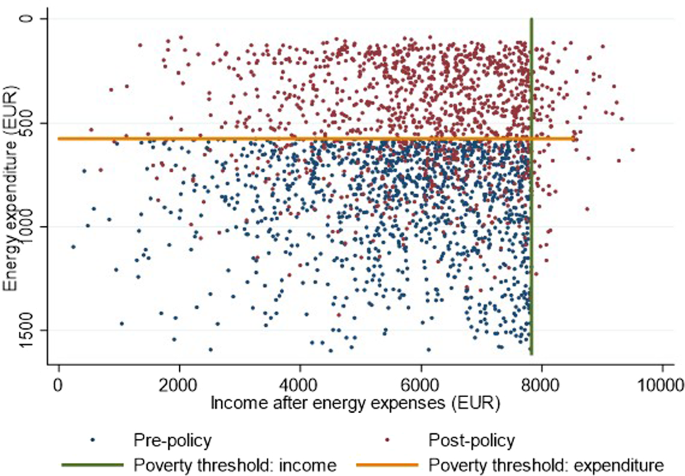
Impact of energy efficiency and bonus policies on energy poverty. Annual energy expenditure and income after energy expenses per household in euros. 67.4% of energy poor households minimised from energy poverty
Additional simulations were conducted to explore the sensitivity of our results with respect to the sample year used and the LIHC threshold. Firstly, we analysed results using data from the year 2019, as this included the bono social applied before the pandemic crisis, avoiding the temporary modifications introduced to address this shock. We also performed simulations with data from the most recent year available, 2022, when the energy poverty rate increased to 7.7% (up from 6.8% in 2019). While the bono social discounts in 2019 were 25% or 40%, depending on the level of vulnerability, these discounts increased to 65% and 80% following the implementation of the post-pandemic social shield. Results for the impact of energy efficiency in 2022 align with those from 2019, and as expected, there are considerable differences in terms of the bono social impact. Specifically, the extensive bono social for electricity and heating has the potential to save 24.7% of households out of energy poverty, resulting in a post-policy energy poverty rate of 5.8% (compared to 9% of households saved of energy poverty in 2019, with a post-policy rate of 6.2%). Given that this extensive measure is planned to be removed by the end of 2024, the results for 2019, which reflect a more stable context, remain the focus of our analysis.
Secondly, following previous studies, we used the energy poverty measure defined by the LIHC, with the poverty threshold set at 60% of the median income. Despite being a standard, this threshold can be considered somewhat arbitrary, and a sensitivity analysis helps to understand the significance of this convention. While the results are slightly sensitive to changes in the threshold, the main conclusions remain unaffected, as the order of magnitude stays consistent across different policies implemented. Figure 6 presents the simulation results in terms of the percentage of households lifted out of energy poverty after the policies, considering three alternative LIHC thresholds.
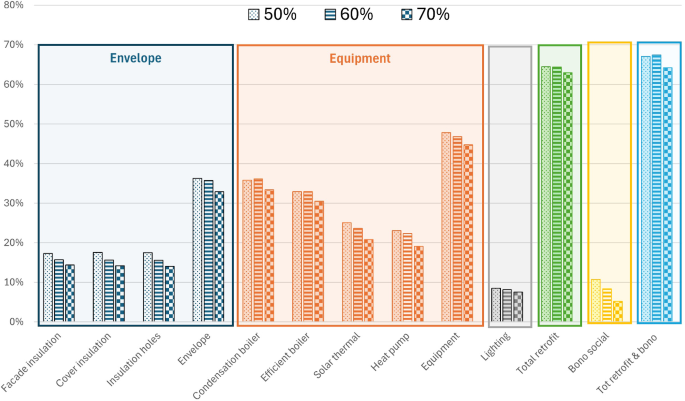
Households escaped from energy poverty after policies (% with different LIHC thresholds)
5 Conclusions
Our analysis provides new insights into how the energy poverty level might be alleviated by combining policies based on income ( bono social ) and expenditure (energy efficiency) tools. The results of our empirical evaluation have a number of highly relevant policy considerations. First, both income and expenditure policies have the potential to reduce the proportion of energy poor households in an economy; however, the magnitude of their respective effects differs greatly. The average impact of energy efficiency measures provides for a greater reduction in the number of energy poor households than the resource transfer measures, where the effect is quite modest. Yet, our results show the outcomes of energy efficiency measures to be highly heterogeneous depending on the type of actions implemented, with the installation of efficient lighting having the smallest impact and the rehabilitation of a building’s thermal envelope having the greatest impact.
Second, and of considerable relevance to our discussion here, is the finding that the combination of energy efficiency measures has better outcomes than the implementation of single energy efficiency measures. Third, the greatest reduction in energy poverty is obtained when income and expenditure measures are jointly applied. However, the incremental effect of the income policy once total retrofit measures has been implemented is highly marginal, reducing the energy poverty level achieved by less than one per cent.
Our outcomes provide useful arguments for the debate regarding society’s policy costs to combat energy poverty, we contribute to this by comparing the total annual expenditure of each measure. The total expenditure of the bono social , assuming universal coverage, is calculated by adding the annual cost of the corresponding benefit to each energy poor household. Analogously, the expenditure of total retrofit, is the result of adding the annualised cost of implementing the measures, assuming universality among the poor household. As a result, when implementing income policies with the amount of EUR 936 million each year, we find that only 9% of households would escape the energy poverty trap. These estimates are an order of magnitude higher than the estimated EUR 926 million of annual average expenditure needed to implement full retrofit measures, which would potentially allow 64% of households to escape the poverty trap, assuming optimal behaviour of consumers and access to finance.
Overall, we can conclude that, while both types of policy are effective at reducing the number of energy poor households, income policies do not break the vicious cycle of energy poverty. Rather, placing energy efficiency programs at the heart of policies to combat energy poverty constitutes a better long-term solution. However, policymakers should be aware that there are factors may influence the effectiveness of these policies. For example, while the bono social of electricity in Spain has shown significant improvements since its inception, there are areas that still require attention. Specifically, it is crucial to simplify the application process further (including necessary documentation and adjusting language to enhance understanding for interested groups). In addition, we still have much to learn about how energy efficiency measures can reach the most vulnerable households. This will clearly require greater commitment and research on how best to address the financial obstacles to the uptake of energy efficiency measures, especially for low-income households. While financial barriers are significant, there are other obstacles to consider, such as the lack of awareness about renovation policies, split incentives between owners and tenants, mistrust of renovation providers, and the presence of cumbersome and slow administrative processes for aids, particularly affecting low-income families with limited education. Therefore, energy efficiency policies must be complemented by the creation of one-stop shops that offer not only financing solutions but also personalized technical advice, and support to households throughout all the renovation process.Please note that the Figure 2 has been changed to figure 7, Figure 3 has been changed to figure 2, Figure 4 has been changed to figure 3, Figure 5 has been changed to figure 4, Figure 6 has been changed to figure 5, Figure 7 has been changed to figure 6 and also the citations are changed in text. Please check and confirm.The new numbering of the figures creates confusion for the reader. It is possible that the figure appearing in the appendix has the following name Fig. A.1 or similar.
The preceding analysis has provided useful insights into the factors influencing energy poverty and the potential impacts of different policy interventions. However, we should mention a number of limitations that future research might address. First, to our ambitious goal, although we could only explain two policies in detail, we focus on the most relevant policies in the Spanish context. We are aware that the narrow scope of the study means that important insights may be missed by not considering a wider range of policies, for instance, behavioural policies, but they are not directly comparable (heterogeneous in terms of targets, resources, and mechanisms). In order to do that, public authorities should extend data availability about actions related with energy poverty in order to better measure the incidence and better design policy recommendations. Second, the energy efficiency policies analysed still fail to cover the costs for numerous vulnerable families, who must pay the costs upfront while financial aid is often only provided afterwards. This creates barriers for households seeking to access these measures. Further research should be conducted to understand in the financing strategy and the total costs of the policy in order to provide a more comprehensive evaluation process.
Given that the bono social is made up two bundled elements, and in fact, the thermal bono is a transfer (in a strict sense), the methodological decision was to treat both as a single transfer. This is because, in practical terms, they allow for a greater disposable income.
Following the recommendations by ECEEE and Ecofys ( 2015 ) on discount rates for energy efficiency projects by households, we use a 3% discount rate for the net present value computed to obtain the current value of future gains from energy efficiency.
Another relevant indicator that aligns with our analysis is the Low Income Low Energy Efficiency (LILEE) indicator, commonly used in the United Kingdom. However, due to data availability, it is not feasible to calculate this indicator for our Spanish sample (Government of United Kingdom 2024 ).
Al Shawa B (2024) Keeping the rebound and energy poverty at bay: a novel tariff structure for the United Kingdom’s residential sector. Energy Res Soc Sci 112:103508. https://doi.org/10.1016/j.erss.2024.103508
Article Google Scholar
Alvarez GG, Tol RSJ (2021) The impact of the bono social de electricidad on energy poverty in Spain. Energy Econ 103:105554. https://doi.org/10.1016/j.eneco.2021.105554
Bagnoli L, Bertoméu-Sánchez S (2022) How effective has the electricity social rate been in reducing energy poverty in Spain? Energy Econ 106:105792. https://doi.org/10.1016/j.eneco.2021.105792
Berger T, Höltl A (2019) Thermal insulation of rental residential housing: do energy poor households benefit? A case study in Krems, Austria. Energy Policy 127:341–349. https://doi.org/10.1016/j.enpol.2018.12.018
Berkhout PH, Muskens JC, Velthuijsen JW (2000) Defining the rebound effect. Energy Policy 28(6–7):425–432. https://doi.org/10.1016/S0301-4215(00)00022-7
Bessa S, Gouveia JP (2022) A framework for policy mix analysis: assessing energy poverty policies. J Environ Econ Policy. https://doi.org/10.1080/21606544.2022.2153744
Boardman B (1991) Fuel poverty: from cold homes to affordable warmth. Belhaven Press, London
Google Scholar
Boardman B (2012) Fuel poverty synthesis: lessons learnt, actions needed: fuel poverty comes of age: commemorating 21 years of research and policy. Energy Policy 49:143–148. https://doi.org/10.1016/j.enpol.2012.02.035
Bouzarovski S, Tirado Herrero S (2017) The energy divide: Integrating energy transitions, regional inequalities and poverty trends in the European Union. Eur Urban Reg Stud 24(1):69–86. https://doi.org/10.1177/0969776415596449
Bouzarovski S, Petrova S, Sarlamanov R (2012) Energy poverty policies in the EU: a critical perspective. Energy Policy 49:76–82. https://doi.org/10.1016/j.enpol.2012.01.033
Bouzarovski S, Thomson H, Cornelis M, Rogulj I, Campuzano M, Goermaere S (2019) Transforming energy poverty policies in the European Union: second annual report of the European Union Energy Poverty Observatory. EU Energy Poverty Observatory: Manchester, 20–01
Buzar S (2016) Energy poverty in Eastern Europe: hidden geographies of deprivation. Routledge, London
Book Google Scholar
Capdevila I, Linares E, Folch R (2012) Eficiencia energética en la rehabilitación de edificios. Energía y medio ambiente 25. Fundación Gas Natural Fenosa
Carfora A, Scandurra G, Thomas A (2021) Forecasting the COVID-19 effects on energy poverty across EU member states. Energy Policy. https://doi.org/10.1016/j.enpol.2021.112597
Costa-Campi MT, Jové-Llopis E, Trujillo-Baute E (2019) Energy poverty in Spain: an income approach analysis. Energy Sources Part B Econ Plan Policy 14(7–9):327–340. https://doi.org/10.1080/15567249.2019.1710624
Dobbins A, Fuso Nerini F, Deane P, Pye S (2019) Strengthening the EU response to energy poverty. Nat Energy 4(1):2–5. https://doi.org/10.1038/s41560-018-0316-8
Dong K, Ren X, Zhao J (2021) How does low-carbon energy transition alleviate energy poverty in China? A nonparametric panel causality analysis. Energy Econ 103:105620. https://doi.org/10.1016/j.eneco.2021.105620
ECEEE and Ecofys (2015) Evaluating our future. The crucial role of discount rates in European Commission energy system modelling. European Council for an Energy Efficient Economy. www.eceee.org/policy-areas/discount-rates/
European Commission (2019) Communication from the Commission to the European Parliament, the European Council, the Council, the European Economic and Social Committee and the Committee of the Regions. The European Green Deal. Brussels, 11.12.2019. COM(2019) 640 final. Retrieved from https://eur-lex.europa.eu/legal-content/EN/TXT/?uri=CELEX%3A52019DC0640 . Accessed Dec 2021
European Commission (2021) Proposal for a Directive of the European Parliament and of the Council on energy efficiency (recast). Brussels, 14.7.2021. COM(2021) 558 final. 2021/0203(COD). https://eur-lex.europa.eu/legal-content/EN/TXT/?uri=CELEX%3A52021PC0558 . Accessed Dec 2021
Eurostat (2016) Statistics Illustrated-NUTS classification (Nomenclature of territorial units for statistics) according to NUTS 2016. https://ec.europa.eu/eurostat/web/nuts/statistics-illustrated . Accessed May 2022
Faiella I, Lavecchia L (2021) Energy poverty. How can you fight it, if you can’t measure it? Energy Build 233:110692. https://doi.org/10.1016/j.enbuild.2020.110692
Government of Spain (2019) Ministry for the ecological transition and the demographic challenge (MITECO).national strategy against energy poverty 2019–2024. https://www.miteco.gob.es/es/prensa/estrategianacionalcontralapobrezaenergetica2019-2024_tcm30-496282.pdf . Accessed June 2022
Government of United Kingdom (2024) Fuel poverty methodology handbook (Low Income Low Energy Efficiency). https://assets.publishing.service.gov.uk/media/65ccf6341d9395000c9466a7/fuel-poverty-methodology-handbook-2024.pdf . Accessed April 2024
Guan Y, Yan J, Shan Y, Zhou Y, Hang Y, Li R, Liu Y, Liu B, Nie Q, Bruckner B, Feng K, Hubacek K (2023) Burden of the global energy price crisis on households. Nat Energy 8:304–316. https://doi.org/10.1038/s41560-023-01209-8
Halkos GE, Gkampoura EC (2021) Evaluating the effect of economic crisis on energy poverty in Europe. Renew Sustain Energy Rev 144:110981. https://doi.org/10.1016/j.rser.2021.110981
Hesselman M, Varo A, Guyet R, Thomson H (2021) Energy poverty in the COVID-19 era: mapping global responses in light of momentum for the right to energy. Energy Res Soc Sci 81:102246. https://doi.org/10.1016/j.erss.2021.102246
Hills J (2011) Fuel poverty: the problem and its measurement. Centre for Analysis of SocialExclusion, London
Hills J (2012) Getting the measure of fuel poverty: Final Report of the Fuel Poverty Review. CASEreport, 72. Centre for Analysis of Social Exclusion, London School of Economics and Political Science, London
IEA (2023) Tracking SDG7: The energy progress report, 2023, IEA, IRENA, UNSD, World Bank, WHO, Paris. https://www.iea.org/reports/tracking-sdg7-the-energy-progress-report-2023 . Accessed April 2024
Košičan J, Pardo Picazo MÁ, Vilčeková S, Košičanová D (2021) Life cycle assessment and economic energy efficiency of a solar thermal installation in a family house. Sustainability 13:2305. https://doi.org/10.3390/su13042305
Kyprianou I, Serghides DK, Varo A, Gouveia JP, Kopeva D, Murauskaite L (2019) Energy poverty policies and measures in 5 EU countries: a comparative study. Energy Build 196:46–60. https://doi.org/10.1016/j.enbuild.2019.05.003
Legendre B, Ricci O (2015) Measuring fuel poverty in France: which households are the most fuel vulnerable? Energy Econ 49:620–628. https://doi.org/10.1016/j.eneco.2015.01.022
Mastropietro P, Rodilla P, Batlle C (2020) Emergency measures to protect energy consumers during the Covid-19 pandemic: a global review and critical analysis. Energy Res Soc Sci 68:101678. https://doi.org/10.1016/j.erss.2020.101678
McCoy D, Kotsch RA (2020) Quantifying the distributional impact of energy efficiency measures. Energy J 42(6):121–144
Meyer S, Laurence H, Bart D, Middlemiss L, Maréchal K (2018) Capturing the multifaceted nature of energy poverty: lessons from Belgium. Energy Res Soc Sci 40:273–283. https://doi.org/10.1016/j.erss.2018.01.017
Middlemiss L (2016) A critical analysis of the new politics of fuel poverty in England. Crit Soc Policy 37(3):425–443. https://doi.org/10.1177/0261018316674851
Milne G, Boardman B (2000) Making cold homes warmer: the effect of energy efficiency improvements in low-income homes A report to the energy action grants agency charitable trust. Energy Policy 28(6–7):411–424. https://doi.org/10.1016/S0301-4215(00)00019-7
Comisión Nacional de los Mercados y la Competencia (CNMC) (2020) Boletín de indicadores eléctricos de abril 2020. Publishing CNMC. https://www.cnmc.es/listado/sucesos_energia_mercado_electrico_boletines_de_indicadores_electricos/block/250 Accessed April 2020
Nägeli C, Farahani A, Österbring M, Dalenbäck JO, Wallbaum H (2019) A service-life cycle approach to maintenance and energy retrofit planning for building portfolios. Build Environ 160:106212. https://doi.org/10.1016/j.buildenv.2019.106212
Oliveras L, Peralta A, Palència L, Gotsens M, López MJ, Artazcoz L, Borell C, Marí-Dell’Olmo M (2021) Energy poverty and health: trends in the European Union before and during the economic crisis, 2007–2016. Heal Place 67:102294. https://doi.org/10.1016/j.healthplace.2020.102294
Pachauri S, Poblete-Cazenave M, Aktas A, Gidden MJ (2021) Access to clean cooking services in energy and emission scenarios after COVID-19. Nat Energy 6(11):1067–1076. https://doi.org/10.1038/s41560-021-00911-9
Pye S, Dobbins A, Baffert C, Brajković J, Deane P, De Miglio R (2017) Energy poverty across the EU: analysis of policies and measures. In: Welsch M (ed) Europe’s energy transition: insights for policy making. Academic Press, London, pp 261–280. https://doi.org/10.1016/B978-0-12-809806-6.00030-4
Chapter Google Scholar
Rau H, Moran P, Manton R, Goggins J (2020) Changing energy cultures? Household energy use before and after a building energy efficiency retrofit. Sustain Cities Soc 54:101983. https://doi.org/10.1016/j.scs.2019.101983
Robinson C, Bouzarovski S, Lindley S (2018) Getting the measure of fuel poverty: the geography of fuel poverty indicators in England. Energy Res Soc Sci 36:79–93. https://doi.org/10.1016/j.erss.2017.09.035
Romero JC, Linares P, López X (2018) The policy implications of energy poverty indicators. Energy Policy 115:98–108. https://doi.org/10.1016/j.enpol.2017.12.054
Sorrell S, Dimitropoulos J (2008) The rebound effect: microeconomic definitions, limitations and extensions. Ecoll Econ 65(3):636–649. https://doi.org/10.1016/j.ecolecon.2007.08.013
Stojilovska A, Guyet R, Mahoney K, Gouveia JP, Castaño-Rosa R, Živčič L, Barbosa R, Tkalec T (2022) Energy poverty and emerging debates: beyond the traditional triangle of energy poverty drivers. Energy Policy 169:113181. https://doi.org/10.1016/j.enpol.2022.113181
Tirado Herrero S (2017) Energy poverty indicators: a critical review of methods. Indoor Built Environ 26(7):1018–1031. https://doi.org/10.1177/1420326X17718054
Vandyck T, Della Valle N, Temursho U, Weitzel M (2023) EU climate action through an energy poverty lens. Sci Rep 13(1):6040. https://doi.org/10.1038/s41598-023-32705-2
Zhao J, Dong K, Dong X, Shahbaz M (2022) How renewable energy alleviate energy poverty? A global analysis. Renew Energy 186:299–311. https://doi.org/10.1016/j.renene.2022.01.005
Download references
Acknowledgements
The authors acknowledge support from project PID2022-140546OB-I00 funded by MCIU/AEI/ https://doi.org/10.13039/501100011033 and ERDF, EU; from project 2021SGR00355 funded by the Departament de Recerca i Universitat de la Generalitat de Catalunya , and from the Chair of Energy Sustainability (IEB, Universitat de Barcelona ).
Open Access funding provided thanks to the CRUE-CSIC agreement with Springer Nature.
Author information
Authors and affiliations.
Department of Economics, Chair of Energy Sustainability and Barcelona Institute of Economics (IEB), Universitat de Barcelona, Barcelona, Spain
Elisenda Jové-Llopis
Department of Economics and Business, Chair of Energy Sustainability (IEB-UB), University of Lleida, Lleida, Spain
Elisa Trujillo-Baute
You can also search for this author in PubMed Google Scholar
Corresponding author
Correspondence to Elisenda Jové-Llopis .
Ethics declarations
Conflict of interest.
The authors have no competing interests to declare that are relevant to the content of this article.
Additional information
Publisher's note.
Springer Nature remains neutral with regard to jurisdictional claims in published maps and institutional affiliations.
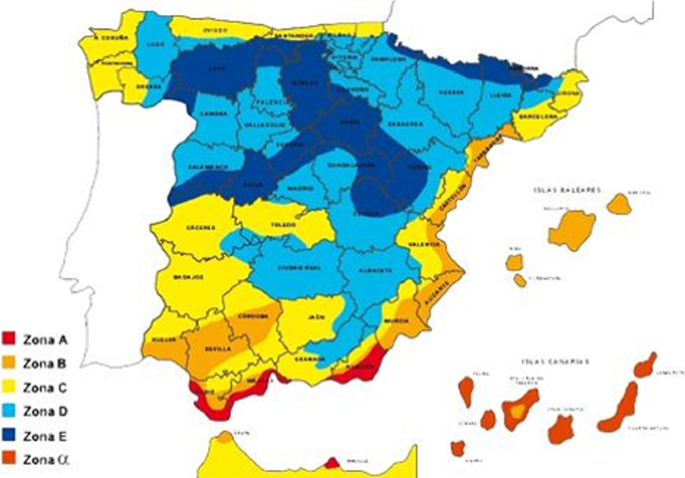
Climatic zones of bono social for heating
Rights and permissions
Open Access This article is licensed under a Creative Commons Attribution 4.0 International License, which permits use, sharing, adaptation, distribution and reproduction in any medium or format, as long as you give appropriate credit to the original author(s) and the source, provide a link to the Creative Commons licence, and indicate if changes were made. The images or other third party material in this article are included in the article's Creative Commons licence, unless indicated otherwise in a credit line to the material. If material is not included in the article's Creative Commons licence and your intended use is not permitted by statutory regulation or exceeds the permitted use, you will need to obtain permission directly from the copyright holder. To view a copy of this licence, visit http://creativecommons.org/licenses/by/4.0/ .
Reprints and permissions
About this article
Jové-Llopis, E., Trujillo-Baute, E. Escaping the Energy Poverty Trap: Policy Assessment. Environ Resource Econ (2024). https://doi.org/10.1007/s10640-024-00918-2
Download citation
Accepted : 15 August 2024
Published : 02 September 2024
DOI : https://doi.org/10.1007/s10640-024-00918-2
Share this article
Anyone you share the following link with will be able to read this content:
Sorry, a shareable link is not currently available for this article.
Provided by the Springer Nature SharedIt content-sharing initiative
- Energy poverty
- Vulnerable households
- Energy expenditure
- Energy efficiency
- Policy evaluation
JEL Classification
- Find a journal
- Publish with us
- Track your research
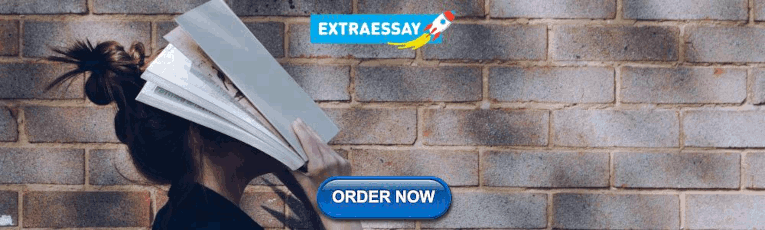
IMAGES
VIDEO
COMMENTS
Magnet Engine free energy generator is an easy device to. generate electrical energy. It works on the principle of. Neodymium Magnets. In a simple motor, magnetic field is. created by the electric ...
Abstract and Figures. The aim of our project is to generate free energy using flywheel. A mains motor of two horsepower capacity is used to drive a series of belt and pulley drive which form a ...
This low-cost energy generator operates by assist source to run the free energy generator without mechanical input and it produces a large amount of power. Published in: 2020 International Conference on Computer, Control, Electrical, and Electronics Engineering (ICCCEEE) Date of Conference: 26 February 2021 - 01 March 2021.
Free energy generators can be used to tap into the available free energy. ... He received the prestigious Best Research Paper Award from Elsevier Computer Communications Journal for the period 2015-2018, in addition to the Top Researcher Award for 2018 at Antalya Bilim University, Turkey. He has led several international symposia and workshops ...
In this paper, we designed and developed an affordable neodymium-based free energy generator that operates continuously without depending on any external source. The repulsive force between the ...
The free energy generator can be fitted on the sides of a 2 wheeler in such a way that as the vehicle moves the fan start rotating and produces fee energy. 3. With 4 wheeler (car) In the same manner the fan can also be fitted on the 4 wheeler and produce free energy. 4.
Related Papers. Journal of Reasearch in Engineering and Applied Sciences. A Conceptual Research on Free Energy Magnetric Generator 1 2. 2016 • ... Free energy generator offers great advantages of cost-effectiveness and availability for the underserved population of sub-Saharan Africa to meet their energy needs. ISBN: 978-988-14047-4-9 ISSN ...
The study for the search of renewable sources of energy is now a major concern worldwide as replacement to the high demand of fossil fuels. Majority of the electricity that is generated uses the Faraday's law, the electromagnetic induction. This law led to new technologies that even brought up the misconception of free energy.
The change of the free energy and chemical potential along this pathway is then given by the integral of the expectation value of the derivative of the Hamiltonian dH( λ) /d λ: 1 dH( λ) d. λ, μ = 0 d λ λ. (1) where means evaluation of the expectation value. using λ an ensemble created by the Hamiltonian at coupling λ.
This paper provides a concise description of the free energy principle, starting from a formulation of random dynamical systems in terms of a Langevin equation and ending with a Bayesian mechanics that can be read as a physics of sentience. It rehearses the key steps using standard results from statistical physics. These steps entail (i) establishing a particular partition of states based upon ...
1. Introduction. It is said that the free energy principle is dificult to understand. This is ironic on three counts. First, the free energy principle (FEP) is so simple that it is (almost) tautological. Indeed, philosophical accounts compare its explanandum to a desert landscape, in the sense of Quine [1].
Free energy: a user's guide Page 13 of 35 33. A simple model of selection. In our model xand zare the inputs and outputs of the agent. The set {x,z} is called the agent's Markov blanket. This term is derived from Judea Pearl's work on statistical inference using Bayesian networks (Pearl 1988).
Over the last fifteen years, an ambitious explanatory framework has been proposed to unify explanations across biology and cognitive science. Active inference, whose most famous tenet is the free energy principle, has inspired excitement and confusion in equal measure. Here, we lay the ground for proper critical analysis of active inference, in three ways. First, we give simplified versions of ...
International Journal of Energy Research - Wiley Online Library. International Journal of Energy Research is dedicated to providing a multidisciplinary, unique platform for researchers, scientists, engineers, technology developers, planners, and policy makers to present research results and findings in a compelling manner on novel energy ...
The use of free energy was noticed in this research paper by using a magnet, and a few satisfactory ... This free Energy can be used to charge small Li-Ion batteries of high voltage & low current, which are used for other applications. 6. For Small wattage bulb: The Free energy generator can be used to power up ...
The Free Energy Principle (FEP) is currently one of the most promising frameworks with which to address a unified explanation of life-related phenomena. With powerful formalism that embeds a small set of assumptions, it purports to deal with complex adaptive dynamics ranging from barely unicellular organisms to complex cultural manifestations. The FEP has received increased attention in ...
This prototype may impact and attract future researchers to work more on the research of free energy or renewable energy. Discover the world's research. 25+ million members;
The free-energy principle (Box 1) says that any self-organizing system that is at equilibrium with its environment must minimize its free energy 2. The principle is essentially a mathematical ...
This research paper revolves around the construction, working and applications of free energy generator & its future enhancements. Here, Disc shaped neodymium ... Free energy generator is used to generate these types of energy. This is a mechanical device which uses the flywheel to store energy in the form of inertia. Let us explain all the system.
Abstract. This paper provides a concise description of the free energy principle, starting from a formulation of random dynamical systems in terms of a Langevin equation and ending with a Bayesian mechanics that can be read as a physics of sentience. It rehearses the key steps using standard results from statistical physics.
Free energy surfaces as a function of V and Q4 are shown for three temperatures, and the average potential energy for local minima of V contributing to the free energy minima is indicated by blue arrows from the disconnectivity graph for kBT /ε = 0.26 and 0.28. A representative structure is also shown above each free energy minimum.
The free energy principle 6 Part Two: Section 5 considers the very small in terms of quantum mechanics. This section derives the Schrödinger wave equation using the relationship between a particle's flow and the NESS density established in the first section. The trick here is to express or factorise the NESS density in terms of (complex ...
The definition, characterization and implementation of Positive Energy Districts is crucial in the path towards urban decarbonization and energy transition. However, several issues still must be addressed: the need for a clear and comprehensive definition, and the settlement of a consistent design approach for Positive Energy Districts. As emerged throughout the workshop held during the fourth ...
Abstract. The free energy principle and its corollary, the active inference framework, serve as theoretical foundations in the domain of neuroscience, explaining the genesis of intelligent behavior. This principle states that the processes of perception, learning, and decision making—within an agent—are all driven by the objective of "minimizing free energy," evincing the following ...
Climate change and the ongoing energy transition can increase energy poverty rates. To date, the main tool employed to alleviate energy poverty has involved income transfers to vulnerable households. However, measures that seek to improve a home's energy efficiency have recently gained increasing relevance. In this study we assess the effectiveness of these two types of policy, assuming ...